Noticias
Investigating students’ programming behaviors, interaction qualities and perceptions through prompt-based learning in ChatGPT
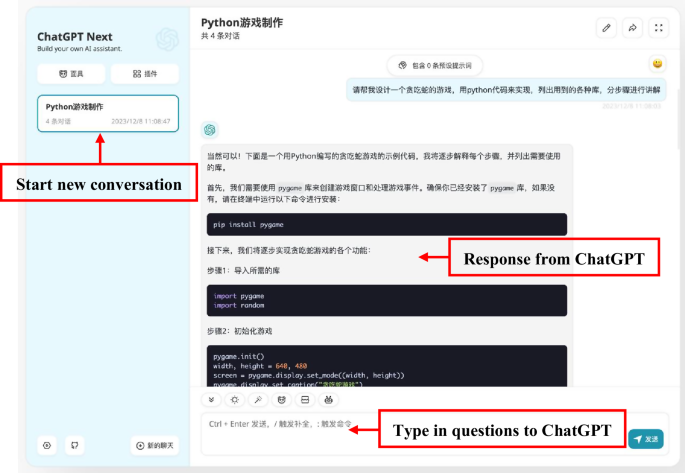
Ahmed MA (2023) ChatGPT and the EFL classroom: Supplement or substitute in Saudi Arabia’s eastern region. Inf Sci Lett 12(7):2727–2734. https://doi.org/10.18576/isl/120704
Google Scholar
Andersen R, Mørch AI, Litherland KT (2022) Collaborative learning with block-based programming: investigating human-centered artificial intelligence in education. Behav Inf Technol 41(9):1830–1847. https://doi.org/10.1080/0144929x.2022.2083981
Google Scholar
Avila-Chauvet L, Mejía D, & Acosta Quiroz CO (2023) Chatgpt as a support tool for online behavioral task programming. Retrieved 20 Feburary, 2024, from https://elkssl50f6c6d9a165826811b16ce3d7452cf8elksslauthserver.casb.hznu.edu.cn/abstract=4329020
Babe HM, Nguyen S, Zi Y, Guha A, Feldman MQ, Anderson CJ (2023) StudentEval: A benchmark of student-written prompts for large language models of code. Cornell University Library, arXiv.org, Ithaca, https://doi.org/10.48550/arxiv.2306.04556
Google Scholar
Balanskat A, Engelhardt K (2015) Computing our future. Computer programming and coding – Priorities, school curricula and initiatives across Europe–update 2015. European Schoolnet, Brussels
Biswas S (2022) Role of ChatGPT in Computer Programming. Mesopotamian J Comput Sci 8–16. https://doi.org/10.58496/mjcsc/2022/004
Brown T, Mann B, Ryder N, Subbiah M, Kaplan J. D, Dhariwal P, Neelakantan A, Shyam P, Sastry G, Askell A, Agarwal S, Herbert-Voss A, Krueger G, Henighan T, Child R, Ramesh A, Ziegler D, Wu J, Winter C, Hesse C, Chen M, Sigler E, Litwin M, Gray S, Chess B, Clark J, Berner C, McCandlish S, Radford A, Sutskever I, & Amodei D (2020). Language models are few-shot learners. In H Larochelle, M Ranzato, R Hadsell, MF Balcan, and H Lin eds. Advances in NeurIPS, (v 33), (pp. 1877–1901). Curran Associates, Inc., URL https://elksslc6aca02ee3bce85ceac323fb2b0abf87elksslauthserver.casb.hznu.edu.cn/paper/2020/file/1457c0d6bfcb4967418bfb8ac142f64a-Paper.pdf
Cooper G (2023) Examining science education in ChatGPT: An exploratory study of generative artificial intelligence. J Sci Educ Technol 32(3):444–452
Google Scholar
Davis FD (1989) Perceived usefulness, perceived ease of use and user acceptance of information technology. MIS Quartely 13(3):319–340
Google Scholar
DeepLearning.AI. (2023) Retrieved 20 Feburary, 2024, from https://elksslc767dbb71ea7c8497a1d1906bf794435elksslauthserver.casb.hznu.edu.cn/short-courses/chatgpt-prompt-engineering-for-developers/
Dengler K, Matthes B (2018) The impacts of digital transformation on the labour market: Substitution potentials of occupations in Germany. Technol Forecast Soc Change 137:304–316. https://doi.org/10.1016/j.techfore.2018.09.024
Google Scholar
Denny P, Leinonen J, Prather J, Luxton-Reilly A, Amarouche T, Becker BA, Reeves BN (2023) Promptly: Using prompt problems to teach learners how to effectively utilize AI code generators. Cornell University Library, arXiv.org, Ithaca, 10.48550/arxiv.2307.16364
Dwivedi YK, Kshetri N, Hughes L, Slade EL, Jeyaraj A, Kar AK, Baabdullah AM, Koohang A, Raghavan V, Ahuja M, Albanna H, Albashrawi MA, Al-Busaidi AS, Balakrishnan J, Barlette Y, Basu S, Bose I, Brooks L, Buhalis D, Wright R (2023) So what if ChatGPT wrote it?” multidisciplinary perspectives on opportunities, challenges and implications of generative conversational AI for research, practice and policy. Int J Inf Manag 71:102642. https://doi.org/10.1016/j.ijinfomgt.2023.102642
Google Scholar
Eason C, Huang R, Han-Shin C, Tseng Y, Liang-Yi L (2023) GPTutor: A ChatGPT-powered programming tool for code explanation. Cornell University Library, arXiv.org, Ithaca, 10.48550/arxiv.2305.01863
Ekin S (2023) Prompt engineering for ChatGPT: A quick guide to techniques, tips, and best practices. Retrieved Jan 10, 2024 from https://doi.org/10.36227/techrxiv.22683919
Faraone SV, Dorfman DD (1987) Lag sequential analysis: Robust statistical methods. Psychol Bull 101(2):312–323. https://doi.org/10.1037/0033-2909.101.2.312
Google Scholar
Filva DA, Forment MA, Garcıa-Penalvo FJ, Escudero DF, Casan MJ (2019) Clickstream for learning analytics to assess students’ behavior with scratch. Future Gener Computer Syst 93:673–686. https://doi.org/10.1016/j.future.2018.10.057
Google Scholar
Gayed JM, Carlon MKJ, Oriola AM, Cross JS (2022) Exploring an AI-based writing assistant’s impact on English language learners. Computers Educ: Artif Intell 3:100055. https://doi.org/10.1016/j.caeai.2022.100055
Google Scholar
ICOURSE (2023) retrieved from 28 July, 2023 from https://elkssl0e848bfdddb2460a97621c537a9f3f13elksslauthserver.casb.hznu.edu.cn/course/BIT-268001?from=searchPage&outVendor=zw_mooc_pcssjg_
Jeon J, Lee S (2023) Large language models in education: A focus on the complementary relationship between human teachers and ChatGPT. Edu Info Technol 1–20. https://doi.org/10.1007/s10639-023-11834-1
Kashefi A, Mukerji T (2023) ChatGPT for programming numerical methods. J Mach Learn Modeling Comput 4(2):1–74. https://doi.org/10.1615/jmachlearnmodelcomput.2023048492
Google Scholar
Kohnke L, Moorhouse BL, Zou D (2023) ChatGPT for language teaching and learning. RELC J 54(2):537–550. https://doi.org/10.1177/00336882231162868
Google Scholar
Krupp L, Steinert S, Kiefer-Emmanouilidis M, Avila KE, Lukowicz P, Kuhn J, Küchemann S, Karolus J (2023) Unreflected acceptance – investigating the negative consequences of ChatGPT-assisted problem solving in physics education. Cornell University Library, arXiv.org, Ithaca, 10.48550/arxiv.2309.03087
Liu J, Li Q, Sun X, Zhu Z, Xu Y (2021) Factors influencing programming self-efficacy: an empirical study in the context of Mainland China. Asia Pac J Educ 43(3):835–849. https://doi.org/10.1080/02188791.2021.1985430
Google Scholar
Liu P, Yuan W, Fu J, Jiang Z, Hayashi H, Neubig G (2023) Pre-train, prompt, and predict: A systematic survey of prompting methods in natural language processing. ACM Comput Surv 55(9):1–35
Google Scholar
Liu G, Ma C (2023) Measuring EFL learners’ use of ChatGPT in informal digital learning of English based on the technology acceptance mode, Innov Lang Learn Teach (online), 1–19. https://doi.org/10.1080/17501229.2023.2240316
Liu I-F, Hung H-C, Liang C-T (2023) A Study of Programming Learning Perceptions and Effectiveness under a Blended Learning Model with Live Streaming: Comparisons between Full-Time and Working Students. Interactive Learn Environ (online), 1–15, https://doi.org/10.1080/10494820.2023.2198586
Lu OHT, Huang JCH, Huang AYQ, Yang SJH (2017) Applying learning analytics for improving students engagement and learning outcomes in an MOOCs enabled collaborative programming course. Interact Learn Environ 25(2):220–234. https://doi.org/10.1080/10494820.2016.1278391
Google Scholar
Mayer RE (2004) Should there be a three-strikes rule against pure discovery learning? The case for guided methods of instruction. Am Psychologist 59(1):14–19
Google Scholar
McTear M, Varghese Marokkie S, Bi Y (2023) A comparative study of chatbot response generation: Traditional approaches versus large language models.In: Jin Z, Jiang Y, Buchmann RA, Bi Y, Ghiran AM, Ma W (eds) Lecture Notes in Computer Science. Knowledge Science, Engineering and Management. KSEM 2023. Springer, Cham 14118. https://doi.org/10.1007/978-3-031-40286-9_7
Mollick E, Mollick L (2023) Assigning AI: Seven approaches for students, with prompts. Cornell University Library, arXiv.org, Ithaca, 10.48550/arxiv.2306.10052
Nouri J, Zhang L, Mannila L, Noren E (2020) Development of computational thinking, digital competence and 21st century skills when learning programming in K-9. Educ Inq 11(1):1–17
Google Scholar
Ofosu-Ampong K, Acheampong B, Kevor M-O, Amankwah-Sarfo F (2023) Acceptance of artificial intelligence (ChatGPT-4) in education: Trust, innovativeness and psychological need of students. Info Knowledge Manag 13(4). https://doi.org/10.7176/ikm/13-4-03
Ou Q, Liang W, He Z, Liu X, Yang R, Wu X (2023) Investigation and analysis of the current situation of programming education in primary and secondary schools. Heliyon 9(4):e15530. https://doi.org/10.1016/j.heliyon.2023.e15530
Google Scholar
Ouh EL, Benjamin KSG, Shim KJ, Wlodkowski S (2023) ChatGPT, can you generate solutions for my coding exercises? an evaluation on its effectiveness in an undergraduate java programming course. Cornell University Library, arXiv.org, Ithaca, https://doi.org/10.48550/arxiv.2305.13680
Google Scholar
Ouyang F, Dai X (2022) Using a three-layered social-cognitive network analysis framework for understanding online collaborative discussions. Australas J Educ Technol 38(1):164–181. https://doi.org/10.14742/ajet.7166
Google Scholar
Ouyang L, Wu J, Xu J, Almeida D, Wainwright CL, Mishkin P, Zhang C, Agarwal S, Slama K, Ray A, Schulman J, Hilton J, Kelton F, Miller L, Simens M, Askell A, Welinder P, Christiano P, Leike J, Lowe R (2022) Training language models to follow instructions with human feedback. Cornell University Library, arXiv.org, Ithaca, https://doi.org/10.48550/arxiv.2203.02155
Google Scholar
Phung T, Pădurean V, Cambronero J, Gulwani S, Kohn T, Majumdar R, Singla A, Soares G (2023) Generative AI for programming education: Benchmarking ChatGPT, GPT-4, and human tutors. Cornell University Library, arXiv.org, Ithaca, https://doi.org/10.48550/arxiv.2306.17156
Google Scholar
Pryzant R, Iter D, Li J, Lee YT, Zhu C, Zeng M (2023) Automatic prompt optimization with “gradient descent” and beam search. Cornell University Library, arXiv.org, Ithaca, https://doi.org/10.48550/arxiv.2305.03495
Google Scholar
Rawas S (2023) ChatGPT: Empowering lifelong learning in the digital age of higher education. Educ Info Technol (online), 1–14. https://doi.org/10.1007/s10639-023-12114-8
Sánchez RA, Hueros AD (2010) Motivational factors that influence the acceptance of Moodle using TAM. Computers Hum Behav 26(6):1632–1640. https://doi.org/10.1016/j.chb.2010.06.011
Google Scholar
Schick T, Schütze H (2021) Exploiting cloze questions for few shot text classification and natural language inference. Cornell University Library, arXiv.org, Ithaca, https://doi.org/10.48550/arxiv.2001.07676
Google Scholar
Shaffer DW, Collier W, Ruis AR (2016) A tutorial on epistemic network analysis: analyzing the structure of connections in cognitive, social, and interaction data. J Learn Analytics 3(3):9–45
Google Scholar
Strzelecki A (2023) To use or not to use ChatGPT in higher education? A study of students’ acceptance and use of technology. Interactive Learning Environments, (online), 1–14. https://doi.org/10.1080/10494820.2023.2209881
Sun D, Boudouaia A, Zhu C et al. (2024a) Would ChatGPT-facilitated programming mode impact college students’ programming behaviors, performances, and perceptions? An empirical study Int J Edu Technol Higher Educ 21:14. https://doi.org/10.1186/s41239-024-00446-5
Google Scholar
Sun D, Ouyang F, Li Y, Zhu C (2021b) Comparing learners’ knowledge, behaviors, and attitudes between two instructional modes of computer programming in secondary education. Int J STEM Educ 8(1):54–54. https://doi.org/10.1186/s40594-021-00311-1
Google Scholar
Sun L, Guo Z, Zhou D (2022) Developing K-12 students’ programming ability: A systematic literature review. Educ Inf Technol 27:7059–7097. https://doi.org/10.1007/s10639-022-10891-2
Google Scholar
Sun M, Wang M, Wegerif R, Jun P (2022) How do students generate ideas together in scientific creativity tasks through computer-based mind mapping? Computers Educ 176:104359. https://doi.org/10.1016/j.compedu.2021.104359
Google Scholar
Sun D, Looi C, Li Y, Zhu C, Zhu C, Cheng M (2024b) Block-based versus text-based programming: A comparison of learners’ programming behaviors, computational thinking skills and attitudes toward programming. Educ Technol Res Develop, online. https://doi.org/10.1007/s11423-023-10328-8
Sun D, Ouyang F, Li Y, Zhu C, Zhou Y (2024c) Using multimodal learning analytics to understand effects of block-based and text-based modalities on computer programming. J Comput Assisted Learn, (online), 1 Int J Edu Technol Higher Edu14. https://doi.org/10.1111/jcal.12939
Sun L, Hu L, Zhou D (2021a) Which way of design programming activities is more effective to promote K-12 students’ computational thinking skills? A meta-analysis. J Comput Assist Learn (online), 1Int J Edu Technol Higher Edu 15. https://doi.org/10.1111/jcal.12545
Surameery NMS, Shakor MY (2023) Use chatgpt to solve programming bugs. Int J Inf Technol Computer Eng 3(1):17–22
Tam A (2023) What are large language models. Retrieved Jan 10, 2024 from https://elkssl6ed619682db9e40376a67ef6f6364a3belksslauthserver.casb.hznu.edu.cn/what-are-large-languagemodels/#:~:text=There%20are%20multiple%20large%20language,language%20and%20can%20generate%20text
Thongkoo, K Daungcharone, J & Thanyaphongphat. (2020). Students’ acceptance of digital learning tools in programming education course using technology acceptance model. In Proceedings of Joint International Conference on Digital Arts, Media and Technology with ECTI Northern Section Conference on Electrical, Electronics, Computer and Telecommunications Engineering (pp. 377–380)
Tian H, Lu W, Li TO, Tang X, Cheung S, Klein J, Bissyandé TF (2023) Is ChatGPT the ultimate programming assistant – how far is it? Cornell University Library, arXiv.org, Ithaca, https://doi.org/10.48550/arxiv.2304.11938
Google Scholar
Venkatesh V, Davis FD (2000) A theoretical extension of the technology acceptance model: Four longitudinal field studies. Manag Sci 46(2):186–204. https://doi.org/10.1287/mnsc.46.2.186.11926
Google Scholar
Vukojičić, M, & Krstić, J (2023) ChatGPT in programming education: ChatGPT as a programming assistant. Journal for Contemporary Education, (online), 7-13
Wei J, Bosma M, Zhao VY, Guu K, Adams WY, Lester B, Du N, Dai AM, Le QV (2021) Finetuned language models are zero-shot learners. Cornell University Library, arXiv.org, Ithaca, https://doi.org/10.48550/arxiv.2109.01652
Google Scholar
White J, Fu Q, Hays S, Sandborn M, Olea C, Gilbert H, Elnashar A, Spencer-Smith J, Schmidt DC (2023) A prompt pattern catalog to enhance prompt engineering with ChatGPT. Cornell University Library, arXiv.org, Ithaca, https://doi.org/10.48550/arxiv.2302.11382
Google Scholar
Xie Y, Boudouaia A, Xu J, AL-Qadri AH, Khattala A, Li Y, Aung YM (2023) A study on teachers’ continuance intention to use technology in English instruction in western China junior secondary schools. Sustainability 15(5):4307. https://doi.org/10.3390/su15054307
Google Scholar
Xu L, Chen Y, Cui G, Gao H, Liu Z (2022) Exploring the universal vulnerability of prompt-based learning paradigm. Cornell University Library, arXiv.org, Ithaca, https://doi.org/10.48550/arxiv.2204.05239
Google Scholar
Yi YJ, You S, Bae BJ (2016) The influence of smartphones on academic performance. Libr Hi Tech 34(3):480–499. https://doi.org/10.1108/lht-04-2016-0038
Google Scholar
Yilmaz R, Karaoglan Yilmaz FG (2023b) The effect of generative artificial intelligence (AI)-based tool use on students’ computational thinking skills, programming self-efficacy and motivation. Computers Educ Artif Intell 4:100147. https://doi.org/10.1016/j.caeai.2023.100147
Google Scholar
Yilmaz R, Karaoglan Yilmaz FG (2023a) Augmented intelligence in programming learning: Examining student views on the use of ChatGPT for programming learning. Computers Hum Behav: Artif Hum 1(2):100005. https://doi.org/10.1016/j.chbah.2023.100005
Google Scholar
Yin, W, Hay, J, and Roth, D (2019) Benchmarking zero-shot text classification: Datasets, evaluation and entailment approach. Proceedings of the Conference on Empirical Methods in Natural Language Processing and the 9th International Joint Conference on Natural Language Processing (EMNLP-IJCNLP’19), in: Kentaro Inui, Jing Jiang, Vincent Ng, and Xiaojun Wan (Eds.). Association for Computational Linguistics, 3912–3921. https://doi.org/10.18653/v1/D19-1404