Noticias
10 worst players in NBA history according to ChatGPT
Published
3 meses agoon
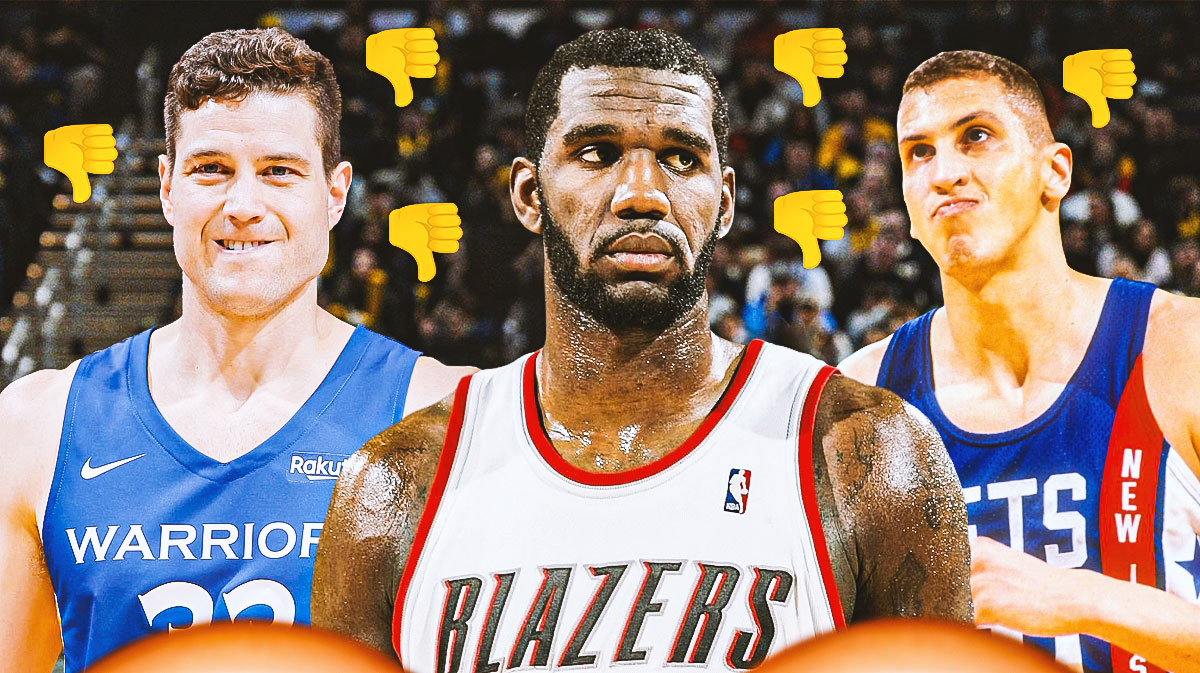
Defining “the worst NBA players ever” certainly isn’t easy. It is hard to say what is worse, an NBA Draft bust who was given a shot to produce, but never amounted to much, or a player who simply didn’t see much time on the court at all, and therefore, never even had a chance to prove himself or put up any resemblance of statistics.
We here at ClutchPoints have looked at the biggest NBA Draft busts ever before. We’ve also looked at players who barely even saw court time. But because of our predicament, we decided to ask ChatGPT who the worst NBA players in history are.
So, check out the gallery to see what artificial intelligence has to say. ChatGPT provided a brief summary justifying its answers, and we went in and provided analysis from our perspective.
1. Michael Olowokandi
ChatGPT summary: Olowokandi was drafted first overall in the 1998 NBA Draft, ahead of future Hall of Famers like Dirk Nowitzki, Paul Pierce, and Vince Carter. Unfortunately, he never lived up to the hype, and despite his size and athleticism, he was often criticized for his poor basketball IQ, lack of work ethic, and inability to dominate in the post. His career numbers are underwhelming, and his lack of impact on winning teams solidified his place in “bust” territory.
ClutchPoints’ analysis: Michael Olowokandi was a high-risk, high-reward draft prospect, but unfortunately, he landed on the wrong side of that equation. The freak athlete with unquestionable measurables was never able to develop the necessary skills to thrive in the NBA game.
The Kandi Man only shot 43.5% from the field despite standing over seven feet tall, which is an incredibly poor mark for a big man. Olowokandi averaged 8.3 points per game over his career, but he only did so because he was forced onto the court due to the Los Angeles Clippers taking him first-overall.
2. Anthony Bennett
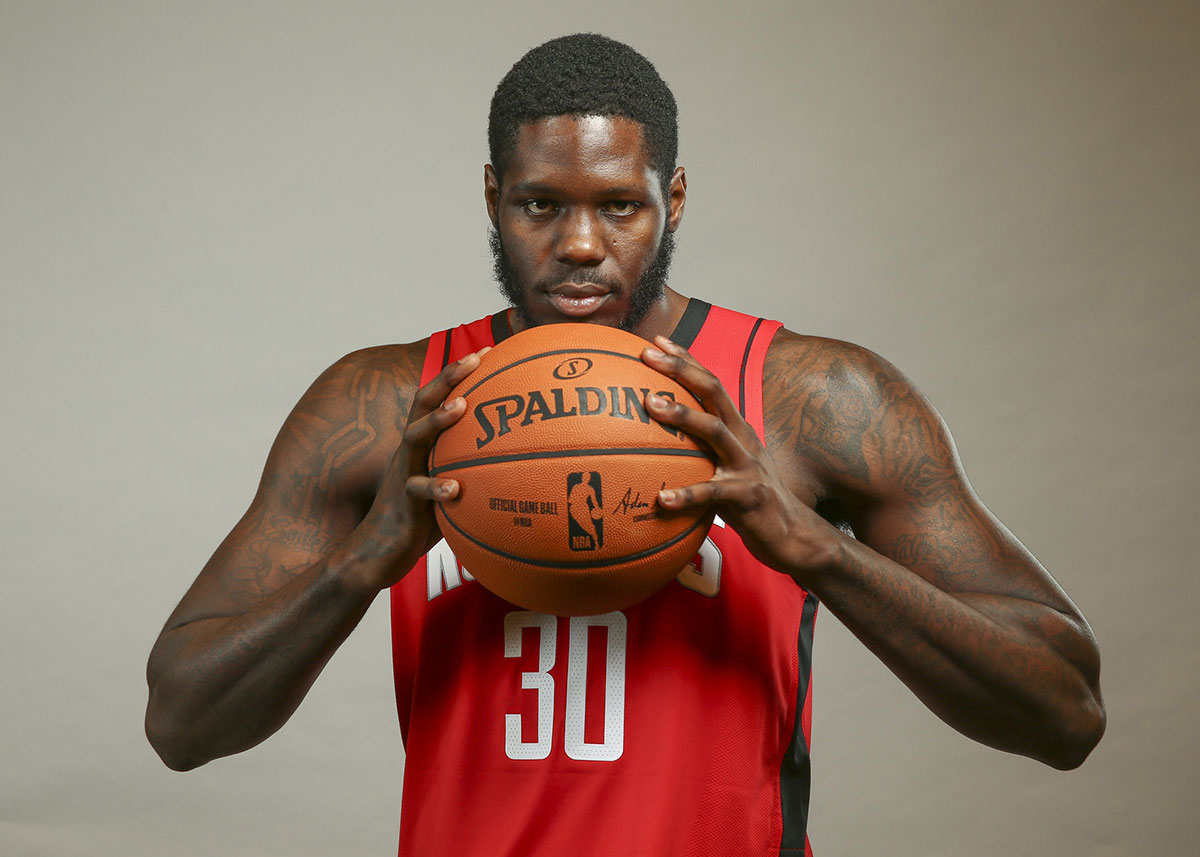
ChatGPT summary: Bennett’s selection as the first overall pick in 2013 remains one of the biggest draft mistakes in NBA history. He was a project player with an inconsistent jumper, no defined role, and questionable conditioning. His rookie season was disastrous, and he struggled to find a place in the league after his brief stint with the Cavaliers. Bennett’s career flamed out quickly, and he bounced around multiple teams before being out of the NBA entirely.
ClutchPoints’ analysis: Our vote for the biggest NBA Draft bust ever was Anthony Bennett, so it makes sense that he shows up here as one of the worst NBA players in history. Bennett was a massive failure, but it was almost easy to see it coming. His 2013 NBA Draft class was viewed as one of the worst draft classes ever, and even then it was looked at as a massive reach when the Cleveland Cavaliers took him first overall.
Bennett, who was supposed to be drafted late in the lottery, was a tweener without a real calling card. It showed on the basketball court, and he was even traded after only one season. Overall, Bennett lasted four seasons in the NBA, each of which was with a different team.
He averaged 4.4 points per game and only started in four total games. Despite the benefits that come with being picked early, no team was really willing to give Bennett a chance because the talent level was just clearly not there.
3. Darko Milicic
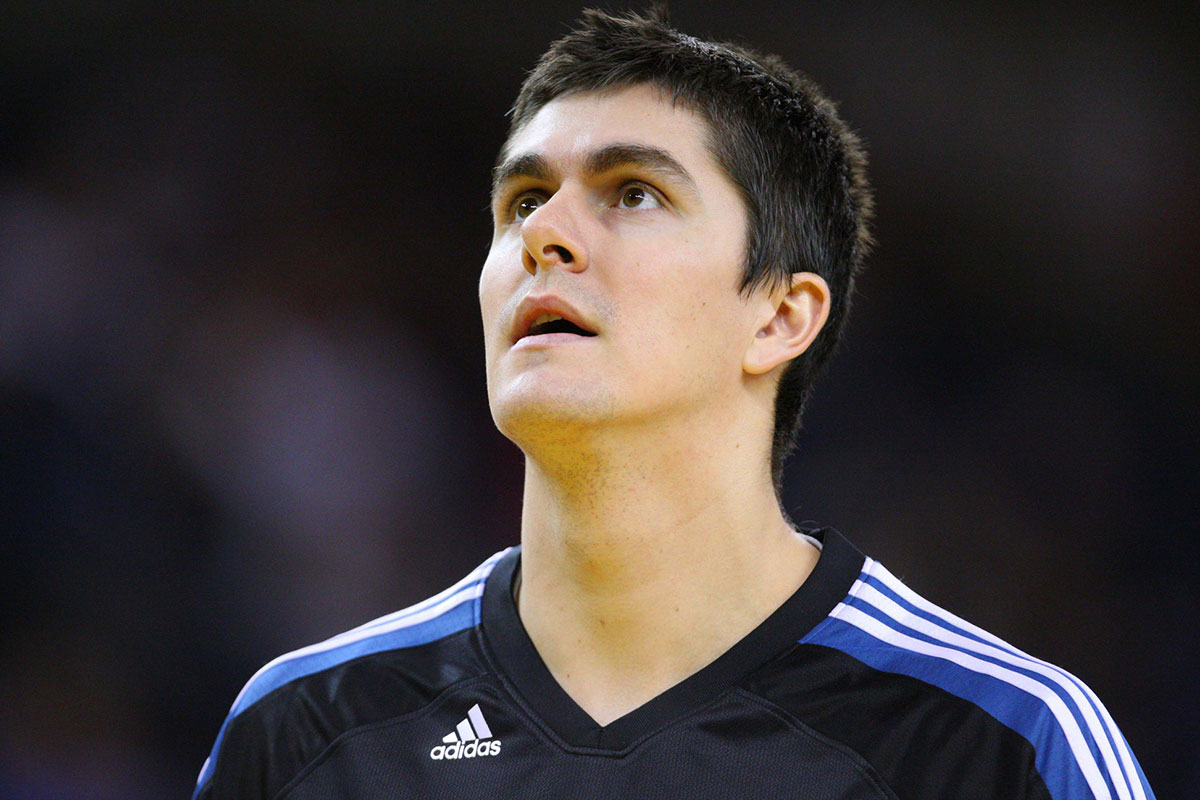
ChatGPT summary: Darko Miličić’s selection as the second overall pick in the 2003 NBA Draft is often regarded as one of the biggest draft busts in history. While the Pistons won a championship during his rookie season (with him barely playing), Darko never lived up to his potential as a skilled 7-footer. He struggled with consistency, effort, and lack of development. Players like Carmelo Anthony, Dwyane Wade, and Chris Bosh were selected after him, making his selection all the more infamous.
ClutchPoints’ analysis: The 2003 NBA Draft class is one of the best ever, as LeBron James, Carmelo Anthony, Dwyane Wade, and Chris Bosh all went inside of the top five. Darko Milicic casts a shadow over the class as the number two pick, though. Milicic joined a great Detroit Pistons team (that he won a championship with his rookie season), but he was incredibly raw due to his status as one of the youngest NBA players ever.
He was never able to develop, and the big man ended his career as a bench warmer and draft bust. His failures were compounded by the stars drafted around him, many of which are still playing or played until recently. Meanwhile, Milicic is now a farmer in Serbia.
4. Sam Bowie
ChatGPT summary: Sam Bowie’s career is largely remembered for being selected ahead of Michael Jordan in the 1984 NBA Draft. While injuries did hamper his career and kept him from reaching his potential, the fact remains that the Blazers passed on one of the greatest players of all time. Bowie had a solid, albeit unspectacular career, but his selection is often cited as a massive blunder in NBA draft history.
ClutchPoints’ analysis: Like Milicic, Sam Bowie is punished in NBA dialogue because of the players drafted before and after him. Hakeem Olajuwon was taken first overall, and Michael Jordan – the greatest NBA player ever – was selected third, while the Portland Trail Blazers ended up with Bowie second overall because team’s prioritized centers over guards in the early ’80s.
As is the trend with high Trail Blazers draft picks, Bowie struggled with injuries, and that prevented him from ever reaching his potential. He was a career 10.9 point-per-game scorer, and he played 511 games in his career (349 starts), so we think it is probably unfair to call him one of the worst NBA players ever, even though it is evident he is one of the worst NBA Draft picks ever.
5. Kwame Brown
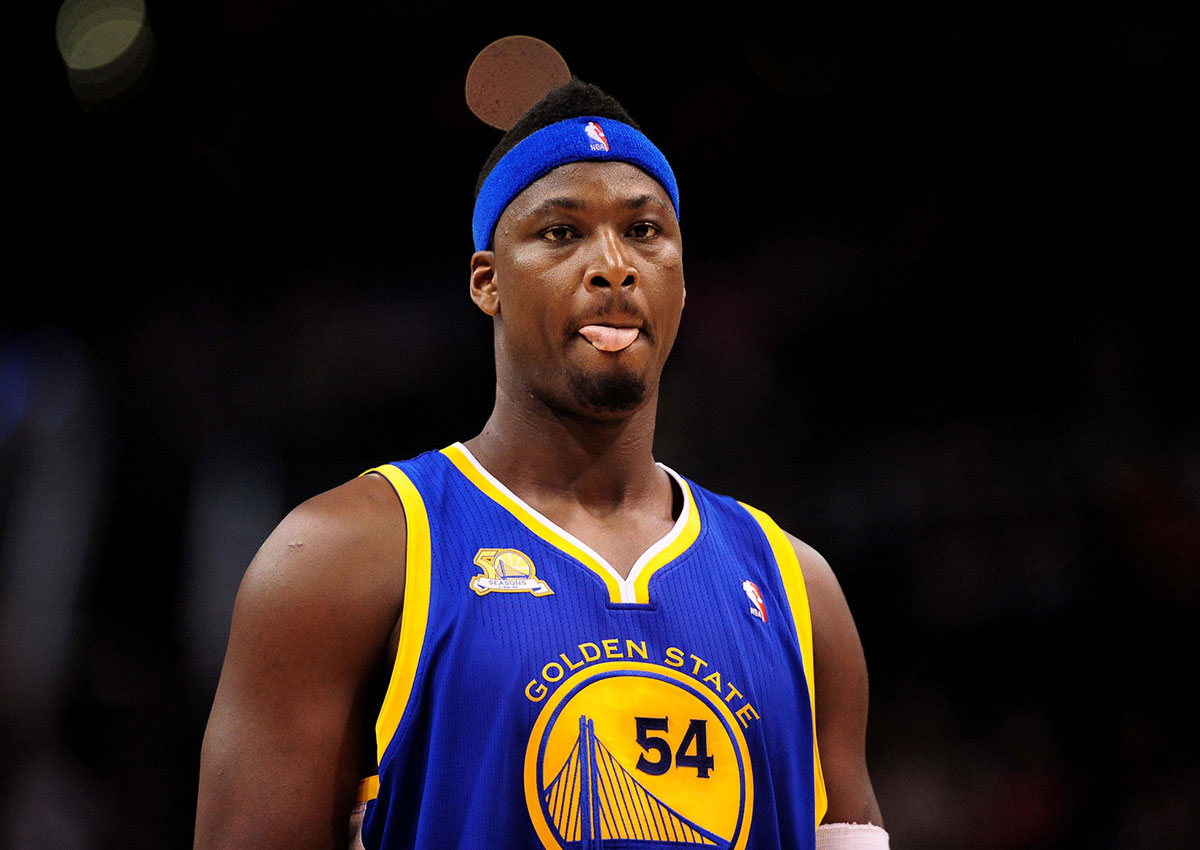
ChatGPT summary: Kwame Brown is the classic example of a player who struggled with the weight of being the top overall pick. After being chosen by the Washington Wizards ahead of other highly regarded prospects, he never lived up to expectations. Brown was often criticized for his lack of skill development, low basketball IQ, and inability to impact games. Despite a long career, his lack of growth made him a punchline in the basketball world.
ClutchPoints’ analysis: In addition to being one of the worst NBA players ever, Kwame Brown is certainly one of the worst first overall picks in NBA history. Brown came to the league straight out of high school, and his failures demonstrated the risk of taking such a young player. Immaturity issues were prevalent with the center, as he never showed a willingness to get better at what he needed to improve upon.
Brown actually did last 12 years in the league, but he was only a double-digit scorer once, and he was limited to a bench role more often than not.
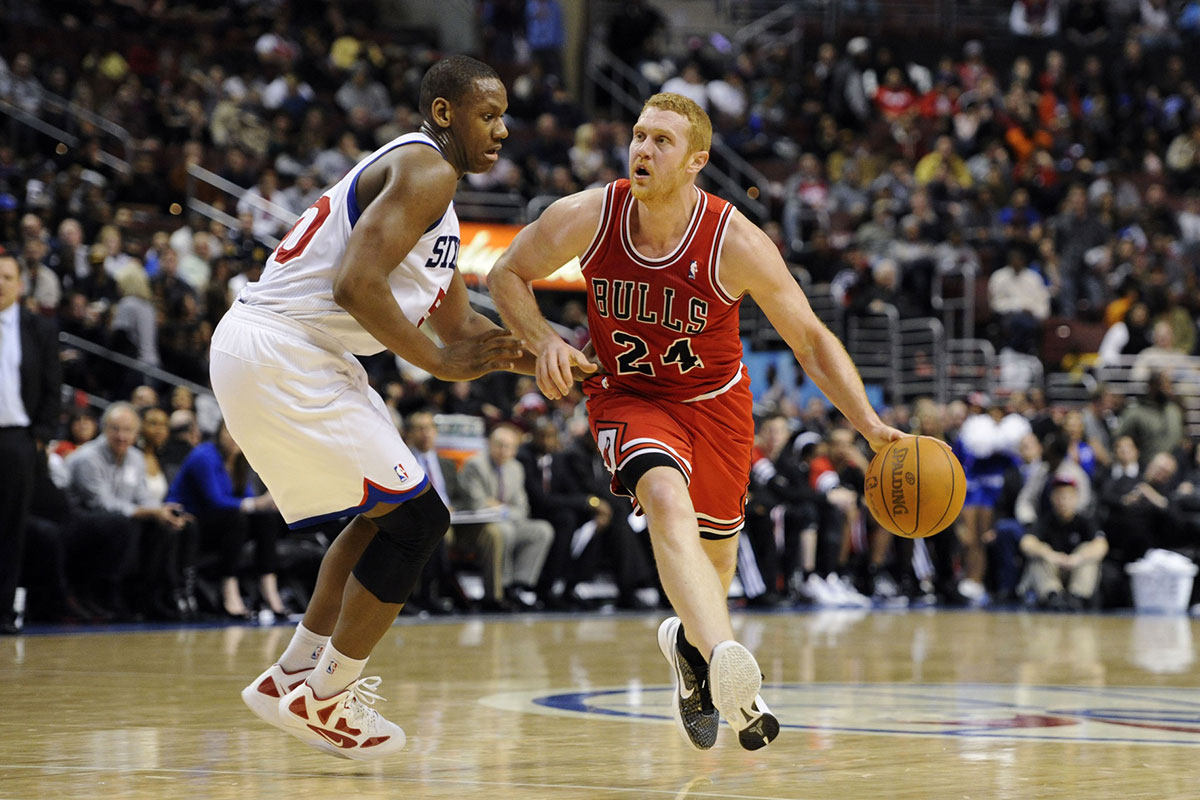
ChatGPT summary: While Brian Scalabrine became a fan favorite and was often referred to as the “White Mamba” due to his enthusiasm and attitude, his actual on-court production was underwhelming. Scalabrine was a journeyman who spent most of his career as a benchwarmer, but his personality and role in the Boston Celtics’ championship run in 2008 gave him a bit of a cult following. In reality, though, his skill set did not justify his NBA career length.
ClutchPoints’ analysis: A lot of the players on this list were big draft busts: Players who were drafted high and were expected to do great, but did not live up to expectations. Brian Scalabrine is here for a different reason, as he was only a second-round pick and was never expected to be much more than a depth piece.
Related NewsArticle continues below
Scalabrine is here because he gained notoriety as a goofy bench player on some pretty good teams. While he was glued to the bench, he actually won a championship with the Boston Celtics in 2008. Scalabrine only averaged 3.1 points per game, but he is famous for saying “I’m closer [in skill] to LeBron than you are to me.” The quote is true and goes to show just how great NBA players are, even the very worst ones.
Scalabrine is the poster boy of a fan-favorite bench-warmer, but considering he lasted 11 years in the NBA and doesn’t qualify as a draft bust, and we aren’t sure that he belongs on this list.
7. Robert Traylor
ChatGPT summary: Known as “Tractor,” Robert Traylor was drafted by the Bucks, but his career never reached the expected level. He was traded to the Cleveland Cavaliers in a deal that eventually involved the selection of Dirk Nowitzki. Traylor’s poor conditioning, inability to stay on the court, and underwhelming performances led to a rather forgettable NBA career. He never made much of an impact.
ClutchPoints’ analysis: Robert Traylor was traded for Dirk Nowitzki, which will make him remembered as a key part in one of the most lopsided trades ever. Despite his hulking frame, Traylor struggled to score with efficiency inside. Additionally, he struggled with stamina and conditioning issues.
Traylor was a career 4.8 point-per-game scorer, and he was out of the league after seven seasons despite being a former number six draft pick. Unfortunately, Traylor passed away in 2011 after suffering a heart attack.
8. Greg Oden
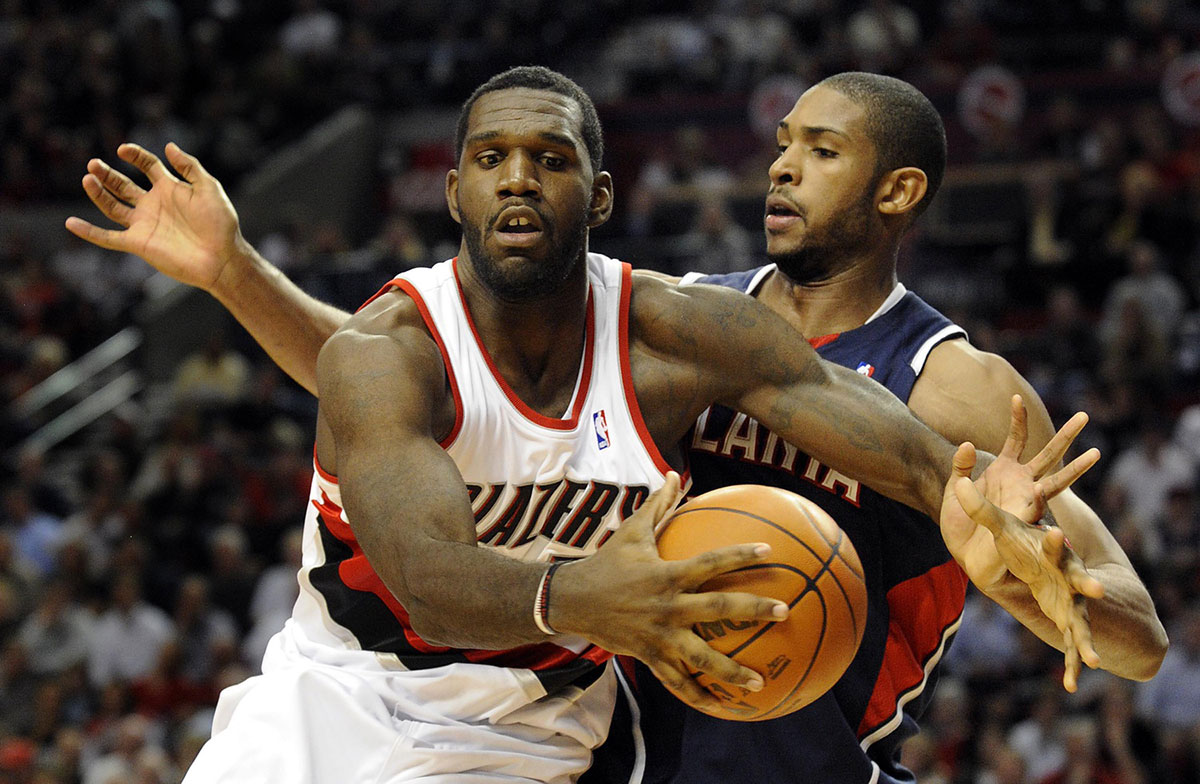
ChatGPT summary: Greg Oden was a highly touted prospect after dominating at Ohio State, but injuries tragically derailed his career. He suffered multiple knee surgeries that kept him from ever reaching his potential. While Oden was solid when he played, the fact that he was picked ahead of Kevin Durant in the 2007 Draft has led to him being remembered as one of the biggest “what ifs” in NBA history.
ClutchPoints’ analysis: Injuries were the demise of Greg Oden. The 2007 first overall pick, who was selected before Kevin Durant, only played in 105 total games, and the 66 games that he started in were fewer than a complete NBA season. Oden missed the entirety of what would have been his rookie season, as well as three straight years from 2010 to 2013. He only once played more than 23 games in a season once, and even then he missed 21 games.
Oden was a talented player, as evidenced by his decent scoring figures (8.9 points and 11.1 points in 2008-09 and 2009-10, respectively) in limited minutes. Being another injury-prone big who was taken before an all-time NBA great will make him infamous as one of the worst NBA players ever, though.
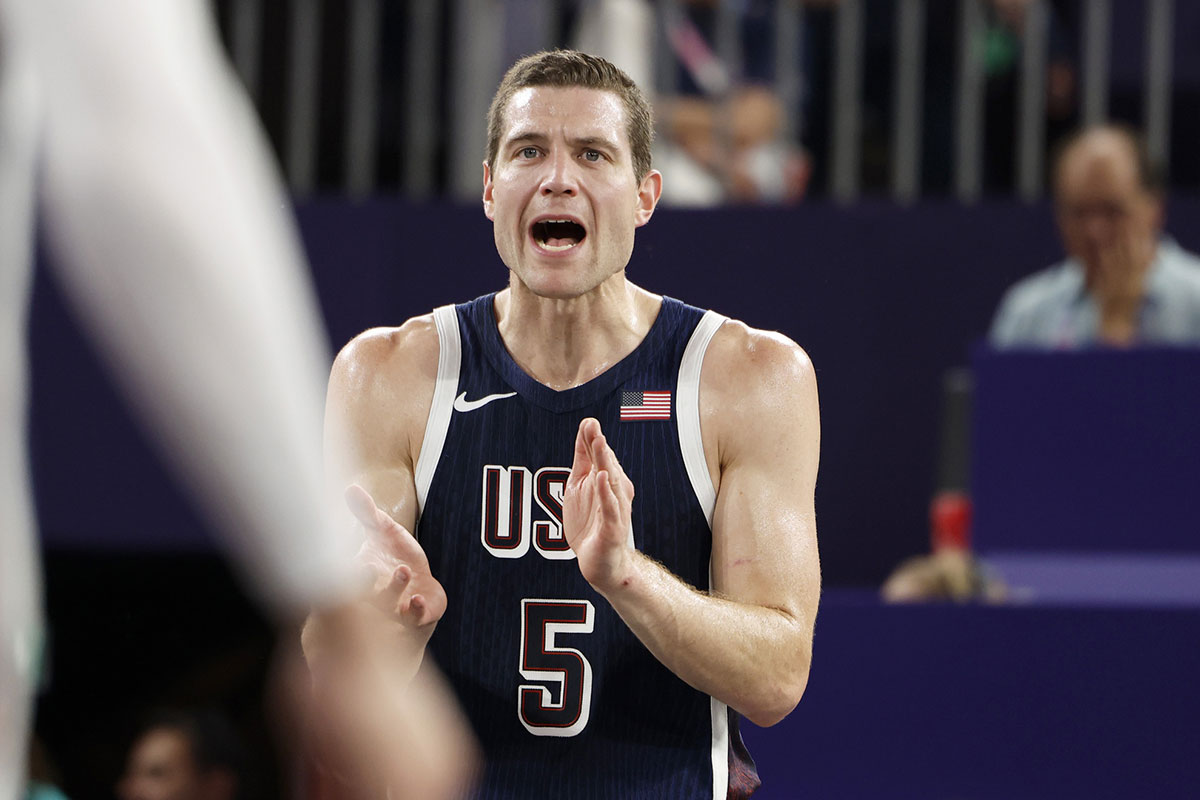
ChatGPT summary: Fredette was a college sensation at BYU, known for his scoring ability, particularly his three-point shooting. However, when he transitioned to the NBA, his inability to guard anyone and his lack of playmaking ability limited his effectiveness. Fredette’s lack of athleticism and inability to adapt to the NBA’s higher level of competition led to a very short career.
ClutchPoints’ analysis: Jimmer Fredette is arguably one of the best college basketball players ever, and certainly one of the best of the 21st century, but the same can’t be said about his NBA career. Fredette has looked good throughout his professional career, including when playing overseason and during his time with Team USA playing 3×3.
Every time he has made an attempt at the NBA, though, he has failed. Fredette can score/shoot with the best of them, but he is undersized to play the shooting guard position in the NBA, and he doesn’t have the playmaking knack to thrive as a lead guard. The step up in competition has proven to be too much for Fredette, especially when it comes to trying to defend anybody.
Fredette’s career six points per game mark is disappointing when you consider that he is a three-time CBA All-Star and a Greek League champion.
10. Nikolaz Tskitishvili
ChatGPT summary: Tskitishvili’s selection as the 5th pick in the 2002 NBA Draft was a puzzling one at the time and only grew more perplexing as his career unfolded. He had an impressive international resume but struggled with the speed and strength of the NBA. Tskitishvili’s inability to adjust, along with a lack of shooting consistency and toughness, made him one of the most infamous draft busts in NBA history.
ClutchPoints’ analysis: Nikolaz Tskitishvili might not have the name recognition of some of the other players on this list, but he was certainly an NBA failure. Foreign big men were coveted in the early 2000s because of the success of Dirk Nowitzki, which led to the Denver Nuggets drafting Tsikishvili fifth overall in 2002.
After only averaging 3.9 point per game as a rookie, though, the Nuggets were forced to find a new franchise cornerstone, and they selected Carmelo Anthony in the following draft. Somehow, that rookie season was Tskitshvili’s best, as he never started another game in the NBA, and he was out of the league after only 1,946 total minutes of court time. Despite standing seven feet tall, Tskitshvili’s career field goal percentage was only 30.4%, as he wasn’t made for the physicality and athleticism of the NBA game.
You may like
Noticias
Operai golpea a Elon Musk con contador • El registro
Published
35 minutos agoon
10 abril, 2025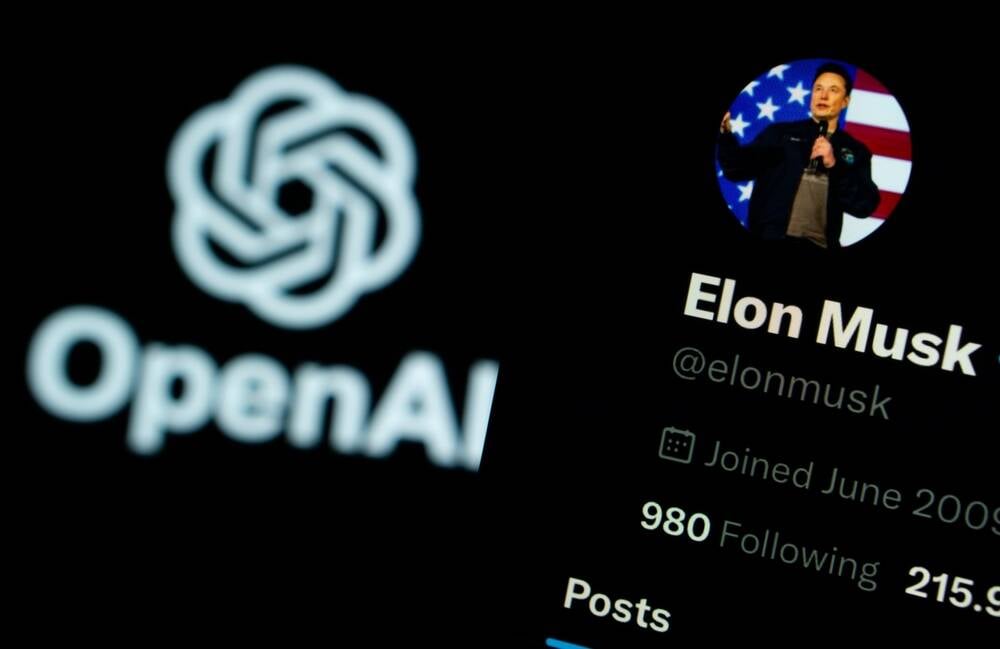
Operai ha contrarrestado al cofundador Elon Musk, acusándolo de tácticas ilegales e injustas para descarrilar sus planes de reestructuración y exigir que un juez lo responsabilice por el daño presuntamente infligido en la AI Super-Lab.
El contador considerable [PDF] y la respuesta a las afirmaciones de Musk se presentó ayer en el Tribunal Federal de California. Si bien acusa al magnate de Tesla de una amplia gama de comportamientos destinados a socavar las operaciones de OpenAI, incluido “acoso, interferencia y información errónea”, las dos reclamaciones de alivio de la Contadora se concentran en el intento de febrero de Musk para comprar el fabricante de ChatGPT por $ 97.375 mil millones. Si bien el equipo de Musk ha retratado la oferta como genuina, los abogados de OpenAi lo llaman algo completamente diferente.
En lugar de una oferta de adquisición seria, OpenAI afirma que la medida de Musk fue una “simulada” diseñada “para interferir con la reestructuración corporativa contemplada de OpenAI”. Musk ya no está involucrado en OpenAi y dirige un atuendo de inteligencia artificial rival, Xai, entre otros negocios.
“La carta no incluía evidencia de financiamiento para pagar el precio de compra de casi $ 100 mil millones”, dijo Openai en su presentación de contadores, y agregó que ninguno de los inversores enumerados en la carta de intención de Musk había hecho ninguna diligencia debida. Más tarde, un inversor admitió, según los registros de la corte, que la intención de Musk era obtener acceso a los materiales internos de Openi a través de los procedimientos legales y “detrás de la pared” en el Super Lab de respaldo de Microsoft.
“Aunque OpenAi reconoció la oferta como una finta, su mera existencia, y la tormenta de fuego de los medios que lo rodean, requirió OpenAi para gastar recursos significativos en la respuesta”, dijo el gigante de la IA.
Es ese esfuerzo, y la llamada “oferta simulada”, lo que llevó a OpenAi a acusar a Musk de prácticas comerciales injustas y fraudulentas, así como una interferencia tortuosa con prospectivo ventaja económica (es decir, cuando un tercero interrumpe un posible acuerdo en detrimento del demandante).
Operai está buscando un alivio cautelar para detener la supuesta interferencia y restitución de Musk por los recursos que, según los que afirma, respondieron a su oferta.
Le preguntamos a OpenAi qué esperaba lograr, y nos dirigió a la presentación de la corte y a sus comentarios realizados en la X de Musk, donde el negocio AI dijo que el contador estaba destinado a detener sus “tácticas de mala fe para reducir la velocidad de OpenAi y aprovechar el control de las innovaciones principales de la IA para su beneficio personal”.
[Musk] Intenté confiscar el control de OpenAi y fusionarlo con Tesla como un fin de lucro: sus propios correos electrónicos lo demuestran. Cuando no se salió con la suya, se quedó
“Elon nunca ha sido sobre la misión. Siempre ha tenido su propia agenda”, continuó Openai. “Trató de confiscar el control de OpenAi y fusionarlo con Tesla como una con fines de lucro: sus propios correos electrónicos lo demuestran. Cuando no se salió con la suya, se fue”.
La muy breve historia de una disputa multimillonaria
Para aquellos que han hecho todo lo posible para ignorar la disputa del jefe de Musk y Operai, Sam Altman, puede ser necesaria un poco de historia.
Musk fue uno de los cofundadores de OpenAi, pero se asaltó en 2018 luego de desacuerdos internos sobre el control y la dirección estratégica. Operai alega que el Oligarch SpaceX propuso fusionarse OpenAi con Tesla (que tiene objetivos autónomos impulsados por IA) o buscó un control total, que el equipo de Altman rechazó, lo que llevó a su salida.
En un momento, el liderazgo de Openi temía que Musk se convertiría en un “dictador” de AGI, o poderosa inteligencia general artificial, si se le permitiera un control completo sobre el laboratorio, a juzgar por correos electrónicos surgió durante esta batalla legal.
“Usted declaró que no desea controlar el AGI final, pero durante esta negociación, nos ha demostrado que el control absoluto es extremadamente importante para usted”, escribió Musk, cofundador y mega-boffin Ilya Sutskever. “El objetivo de OpenAi es hacer el futuro el futuro y evitar una dictadura AGI”.
En marzo de 2024, Musk demandó a Openai y Altman alegando incumplimiento de contrato, prácticas comerciales injustas y fallas fiduciarias relacionadas con la estrecha asociación de OpenAI con Microsoft y el establecimiento de una subsidiaria con fines de lucro. (Openai comenzó como una organización sin fines de lucro).
Musk retiró esta demanda en junio del año pasado sin proporcionar una razón pública, pero presentó una casi idéntica un par de meses después. Afirmó el cambio de OpenAi hacia un modelo con fines de lucro contradecía su misión original de desarrollar IA en beneficio de la humanidad.
El equipo legal de Openai describió la queja de Musk como “Lurch[ing] De la teoría a la teoría, distorsione[ing] sus propias exhibiciones y comercio[ing] De principio a fin en conclusiones sin hechos y a menudo ad hominem “.
Operai niega que se esté convirtiendo en una empresa única con fines de lucro, afirmando en su contratación que su plan de reestructuración solo vería que su subsidiaria con fines de lucro se convirtió en una corporación de beneficios público. Ese movimiento es necesario, afirmado Openai, para permitir que el equipo compita mejor por el capital “al servicio de la misión de desarrollar AGI en beneficio de la humanidad”. Dicho esto, Operai continúa recaudando decenas de miles de millones de dólares en fondos, $ 40 mil millones tan recientemente como finales de marzo.
Un portavoz de Operai le dijo además El registro No tenía intención de abandonar su núcleo sin fines de lucro.
“Nuestra junta ha sido muy clara de que tenemos la intención de fortalecer la organización sin fines de lucro para que pueda cumplir su misión a largo plazo”, nos dijo Openai. “No lo estamos vendiendo, estamos duplicando su trabajo”.
Operai también nos señaló el anuncio de la semana pasada de una comisión que comprende expertos en salud, ciencia, educación y servicios públicos para guiar la evolución planificada de las ORG.
“Esperamos los aportes y los consejos de los líderes que tienen experiencia en organizaciones comunitarias sobre cómo podemos ayudarlos a lograr sus misiones”, dijo Openai en un comunicado enviado por correo electrónico.
Sin embargo, OpenAi tiene que completar su transición a una entidad con fines de lucro a fines de 2025 para asegurar que los $ 40 mil millones mencionados anteriormente en fondos dirigidos por SoftBank.
Es probable que la demanda de Musk solo desacelere, especialmente porque el juicio, según una orden previa al juicio esta semana, no se debe comenzar hasta marzo de 2026.
Ni Musk, famoso ahora, la grasa Eminence del presidente Trump, ni su equipo legal respondieron a preguntas para esta historia. ®
Noticias
La generación de imágenes en chatgpt se ha mejorado mucho
Published
49 minutos agoon
10 abril, 2025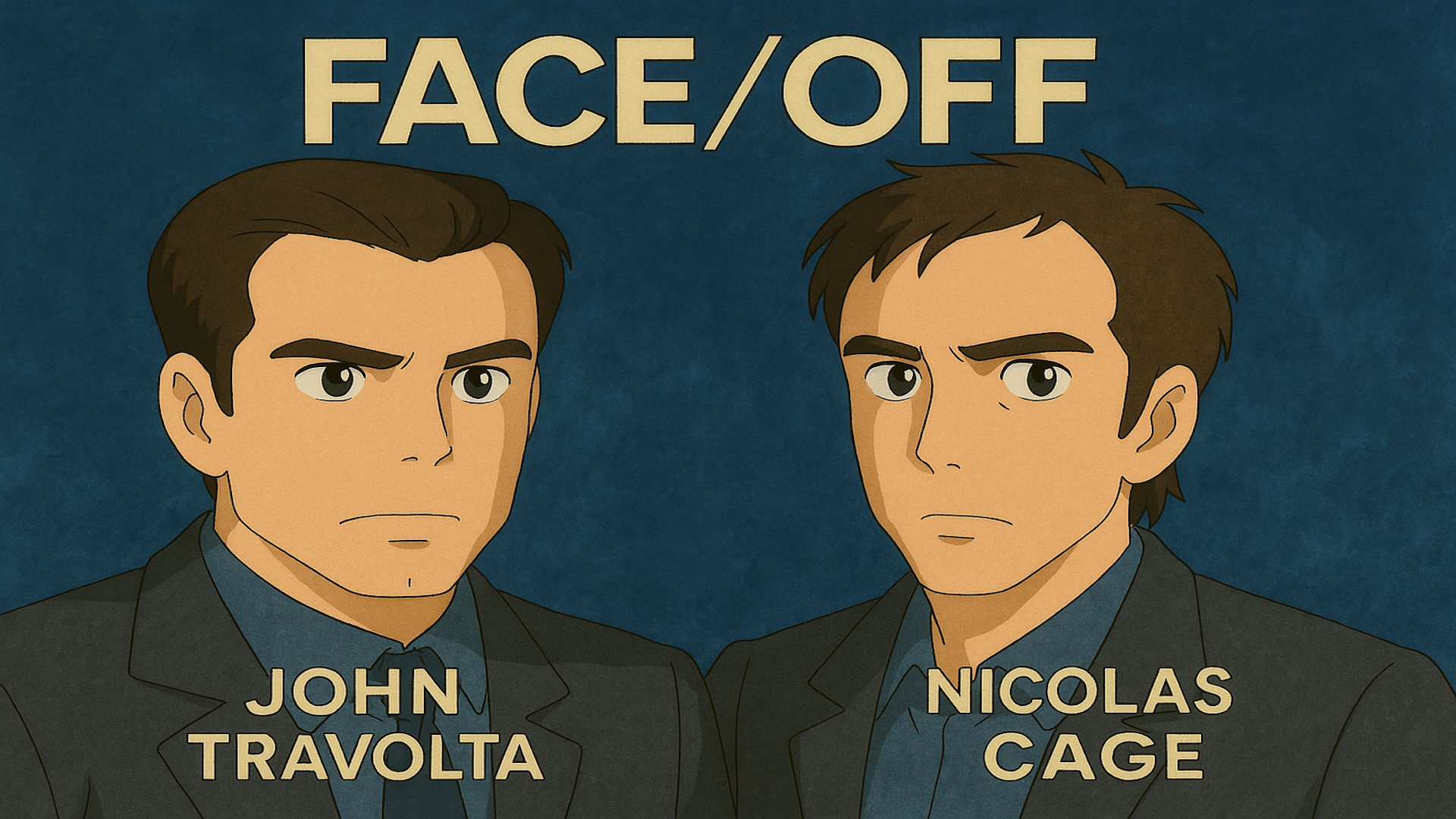
Resumen
-
La generación de imágenes 4O en ChatGPT ofrece imágenes fotorrealistas con una consistencia mejorada y sigue con precisión las instrucciones.
-
Los usuarios pueden convertir imágenes en diferentes estilos y refinarlas a través de indicaciones.
-
Las imágenes cargadas se pueden emplear como referencias, o ChatGPT puede utilizar su propia base de conocimiento.
Cuando Operai deja una nueva característica, a menudo hay una pequeña cantidad de zumbido entre las personas interesadas, pero rara vez rompe Internet. Sin embargo, con el lanzamiento de un modelo actualizado de generación de imágenes, ChatGPT hizo exactamente eso.
La generación de imágenes 4O ha reemplazado a Dall-E como la herramienta de generación de imágenes predeterminada en ChatGPT, y los resultados son muy impresionantes. Ha llevado a que las personas inundan Internet con imágenes que han generado utilizando la herramienta, y su popularidad parece haber tomado por sorpresa por sorpresa.
La generación de imágenes 4O está integrada en GPT-4O
Como su nombre indica, 4O Generación de imágenes está integrada en el modelo GPT-4O. Mientras esté utilizando ese modelo, no necesita hacer nada más que pedirle a ChatGPT que cree una imagen, y la generación de imágenes 4O llegará a funcionar. Algunos modelos, como O1, no le permiten crear imágenes, pero parece que 4O Generation de imágenes no se limita a GPT-4O. Intenté crear una imagen en GPT-4, y todavía usaba la generación de imágenes 4O en lugar del modelo Dall-E que se usaba anteriormente.
Si prefiere usar Dall-E por algún motivo, todavía hay un Dall-E GPT dedicado disponible en la tienda pública GPT. Puede usar esto para generar imágenes utilizando el modelo más antiguo y menos capaz. Hay poco uso para eso ahora, aparte de ver cuán mejor se ha vuelto la generación de imágenes.
Crear excelentes imágenes fotorrealistas
Una de las mejoras más obvias sobre Dall-E es que la generación de imágenes 4O puede producir algunas imágenes fotorrealistas excelentes, sin que tenga que preocuparse demasiado por la elaboración rápida. Mientras que las imágenes tardan un poco en generarse y revelan lentamente de arriba hacia abajo de una manera que recuerda cómo las imágenes se solucionan lentamente sobre el acceso telefónico, los resultados son muy superiores a lo que Dall-E podría producir.
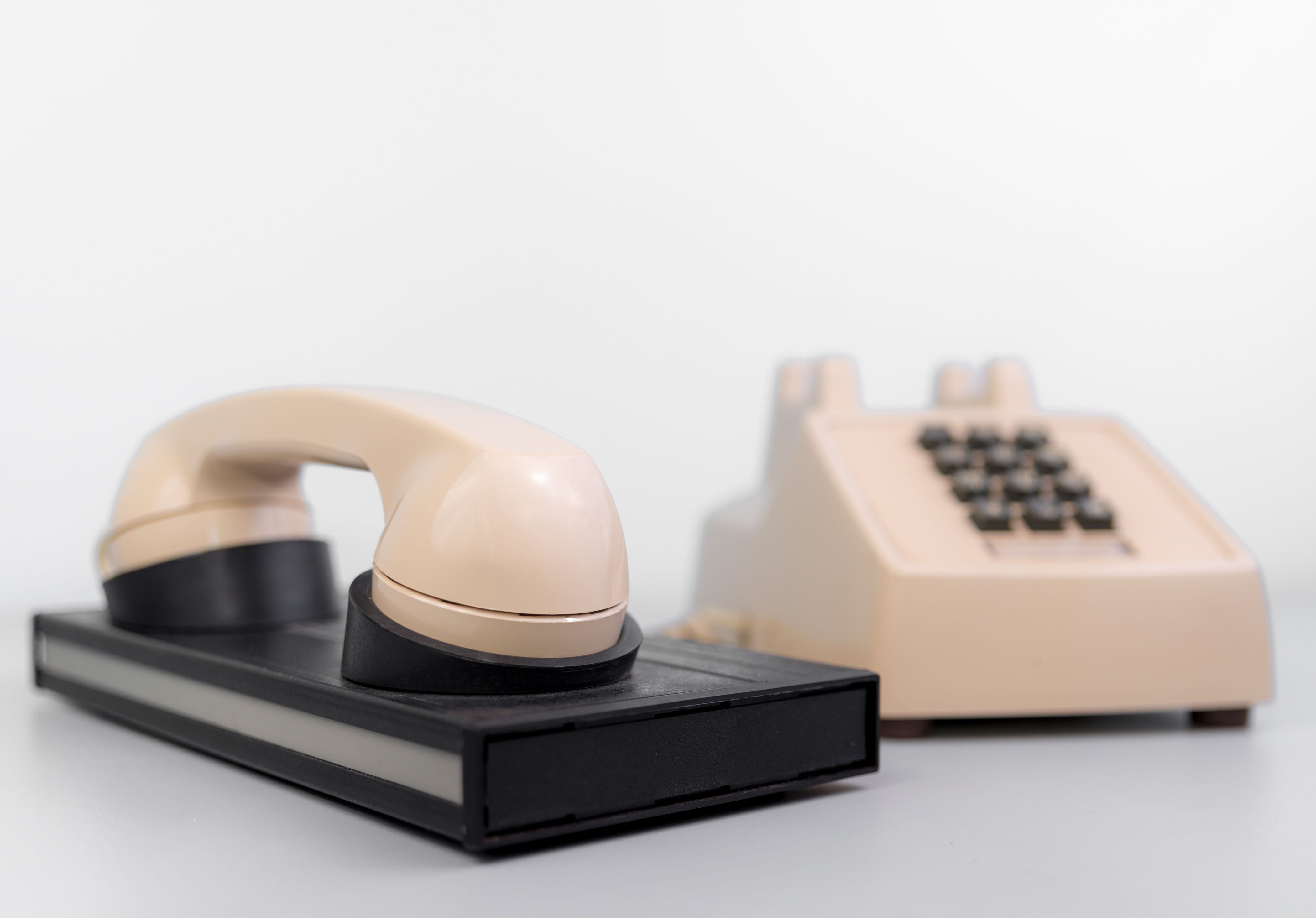
Relacionado
La nueva generación de imágenes de Chatgpt se siente como un acceso telefónico de nuevo
Ver mis imágenes aparecer lentamente de arriba hacia abajo me lleva de regreso a los viejos tiempos.
Le pedí a Dall-E una imagen fotorrealista de un mono con un sombrero de copa, y esto es lo que me dio:
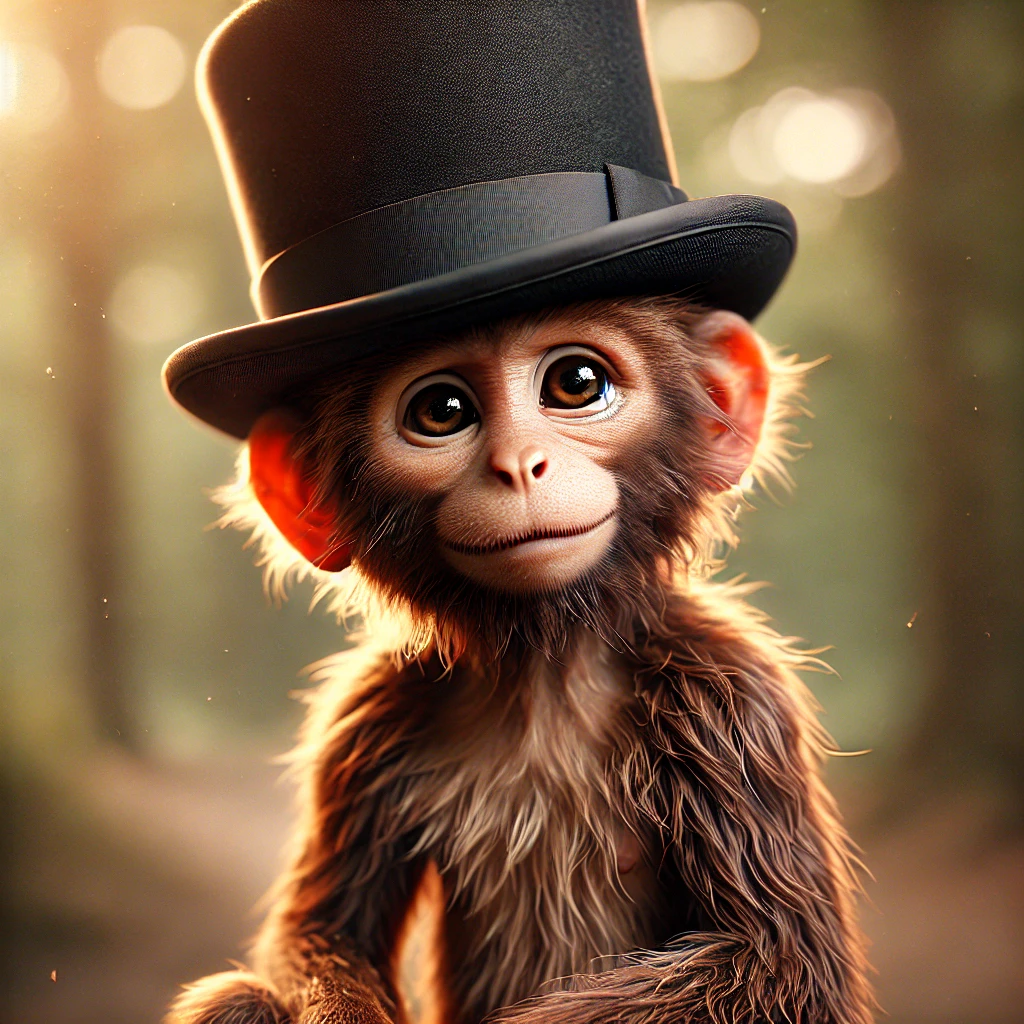
Esta es una imagen generada por la generación de imágenes 4O utilizando el mismo aviso:
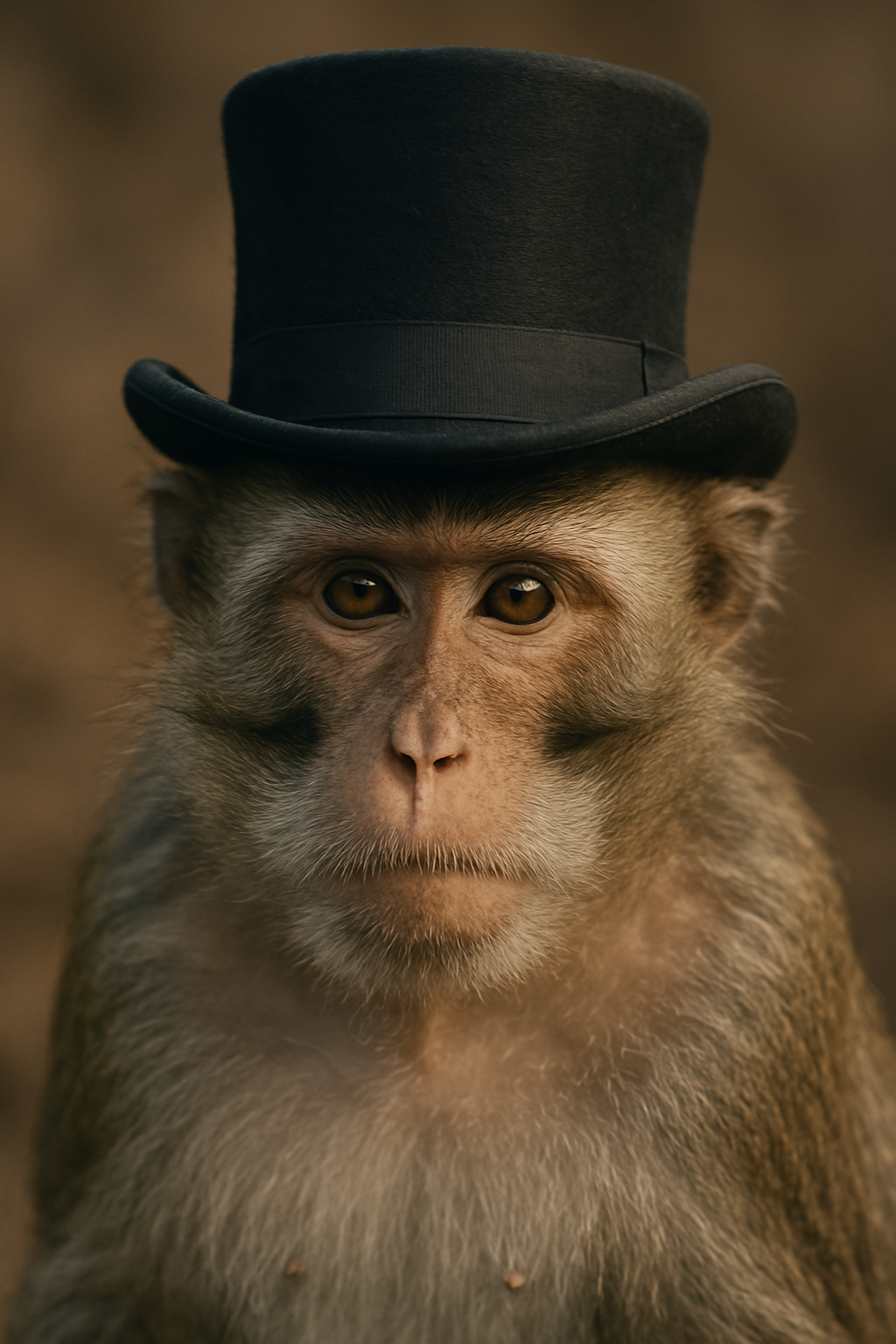
La diferencia es asombrosa y, francamente, un poco aterradora. Hasta ahora, generalmente es posible saber si una imagen era generada por AI si parecía lo suficientemente fuerte para dedos adicionales o texto destrozado. Sin embargo, las imágenes que genera ChatGPT son muy difíciles de distinguir de lo real, y como se dice comúnmente sobre los nuevos desarrollos de IA, este es lo peor que jamás sean.
Puedes convertir imágenes en diferentes estilos
Una de las cosas que ha incorporado Internet desde el lanzamiento de 4O Generation de imágenes es la capacidad de pedirle a ChatGPT que convierta sus imágenes en diferentes estilos. Por ejemplo, puede subir una foto tuya y pedirle a ChatGPT que la cambie al estilo de Van Gogh. Esto no es algo nuevo, pero la calidad de los resultados es un gran paso adelante de Dall-E.
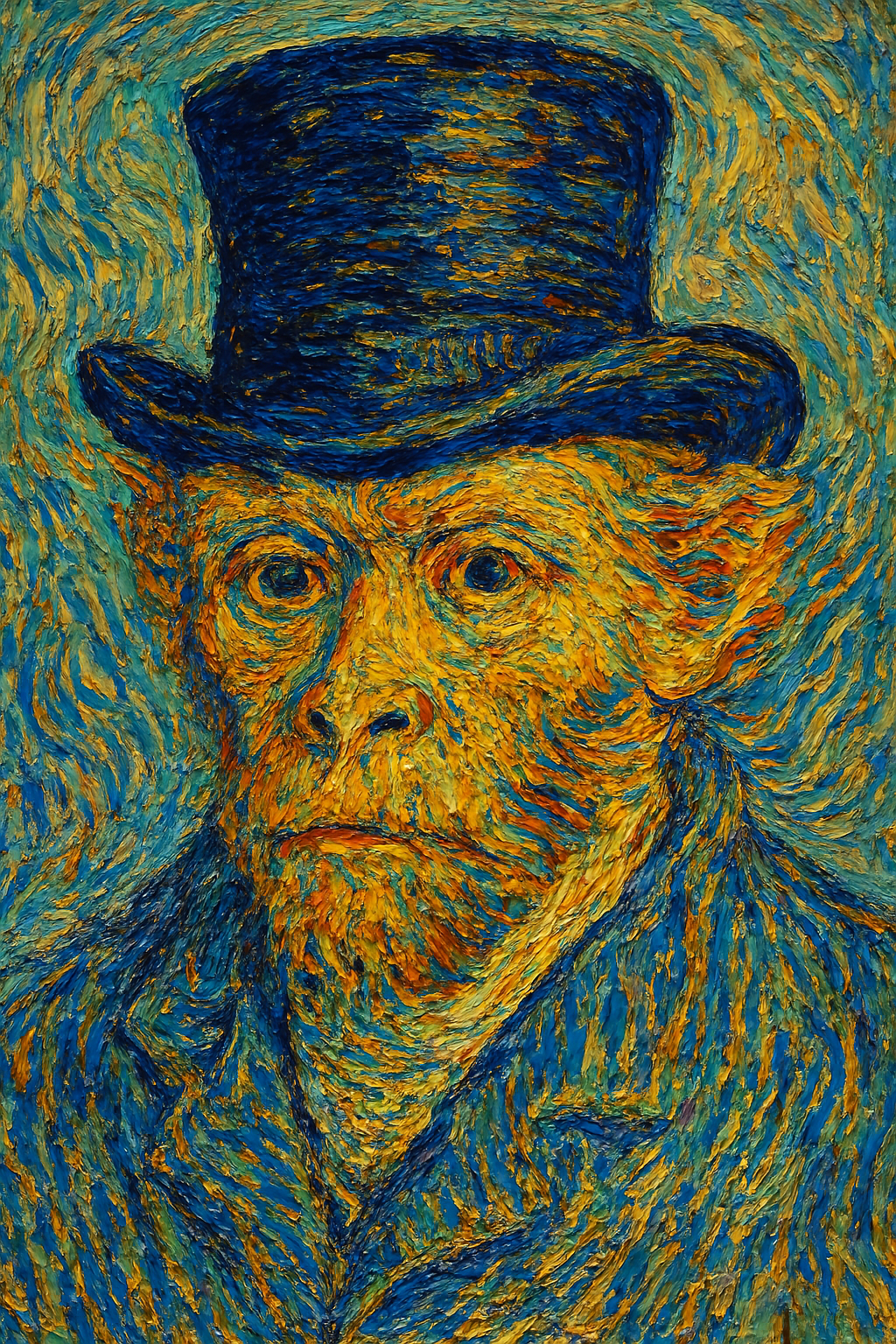
Esto hizo que muchas personas comenzaran a subir imágenes de sí mismas o de la cultura popular que se habían transformado en el estilo de Studio Ghibli, el popular estudio de animación detrás de películas clásicas como Enérgico y Mi vecino Totoro. Los resultados suelen ser impresionantes, pero provocó un debate en línea sobre cuán ético es usar AI para robar esencialmente el estilo de un artista sin su permiso. En el momento de escribir, sin embargo, todavía podía hacer imágenes al estilo de Studio Ghibli sin problemas.
Es fácil refinar imágenes a través de indicaciones
Otra mejora importante es que la generación de imágenes 4O tiene una excelente consistencia. Esto significa que si hay una pequeña cosa mal con su imagen, puede pedirle a ChatGPT que lo arregle, y dejará solo el resto de la imagen. Dall-E a menudo hará cambios importantes en el resto de la imagen cuando intente arreglar una parte de ella.
Esto hace que sea mucho más fácil obtener la imagen exacta que desea, que a menudo es una gran fuente de frustración con Dall-E. Tendría que probar varias veces incluso para acercarse a la imagen que quería, y a veces fallaría por completo. Ahora, por ejemplo, puede pedir tener el sombrero de copa del mono en un ángulo diferente, y el sombrero cambiará, pero el resto de la imagen permanecerá igual.
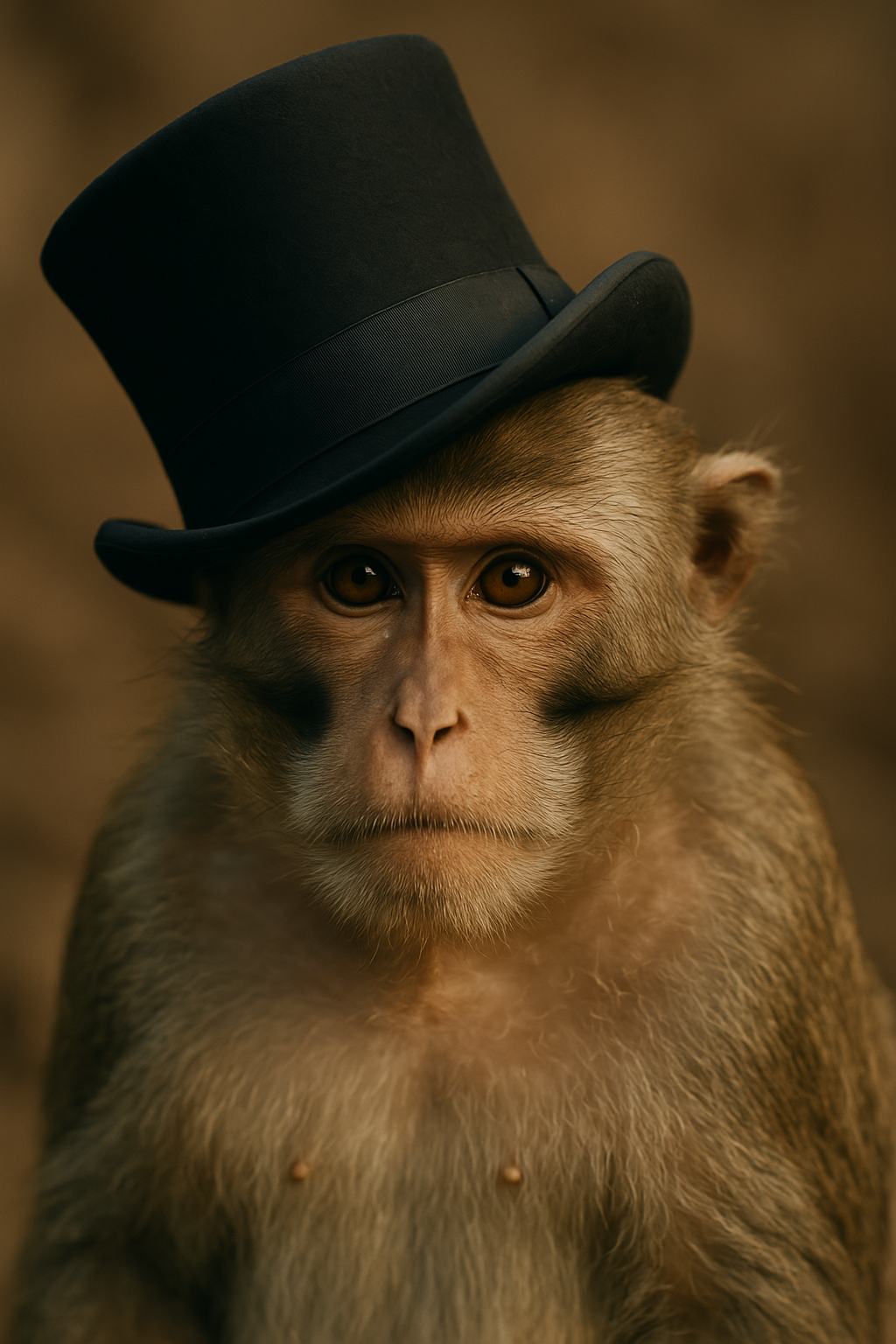
Esta consistencia también lo hace excelente para producir múltiples imágenes de la misma persona o carácter. Puede pedir que el mismo personaje aparezca en una configuración diferente, y ChatGPT preservará la apariencia del personaje en su nueva imagen.
Chatgpt finalmente puede manejar el texto
Este es uno de los mayores cambios en la generación de imágenes 4O. Dall-E podría agregar texto a las imágenes, pero realmente, realmente luchó por hacerlo. Por lo general, recibirías un mensaje de texto que se parecía principalmente a las palabras que querías, pero que estaban muy ligeramente apagados. Suficiente para arruinar sus imágenes, al menos. Usando la generación de imágenes 4O, puede crear el texto exacto que desea, y genera sin problemas.
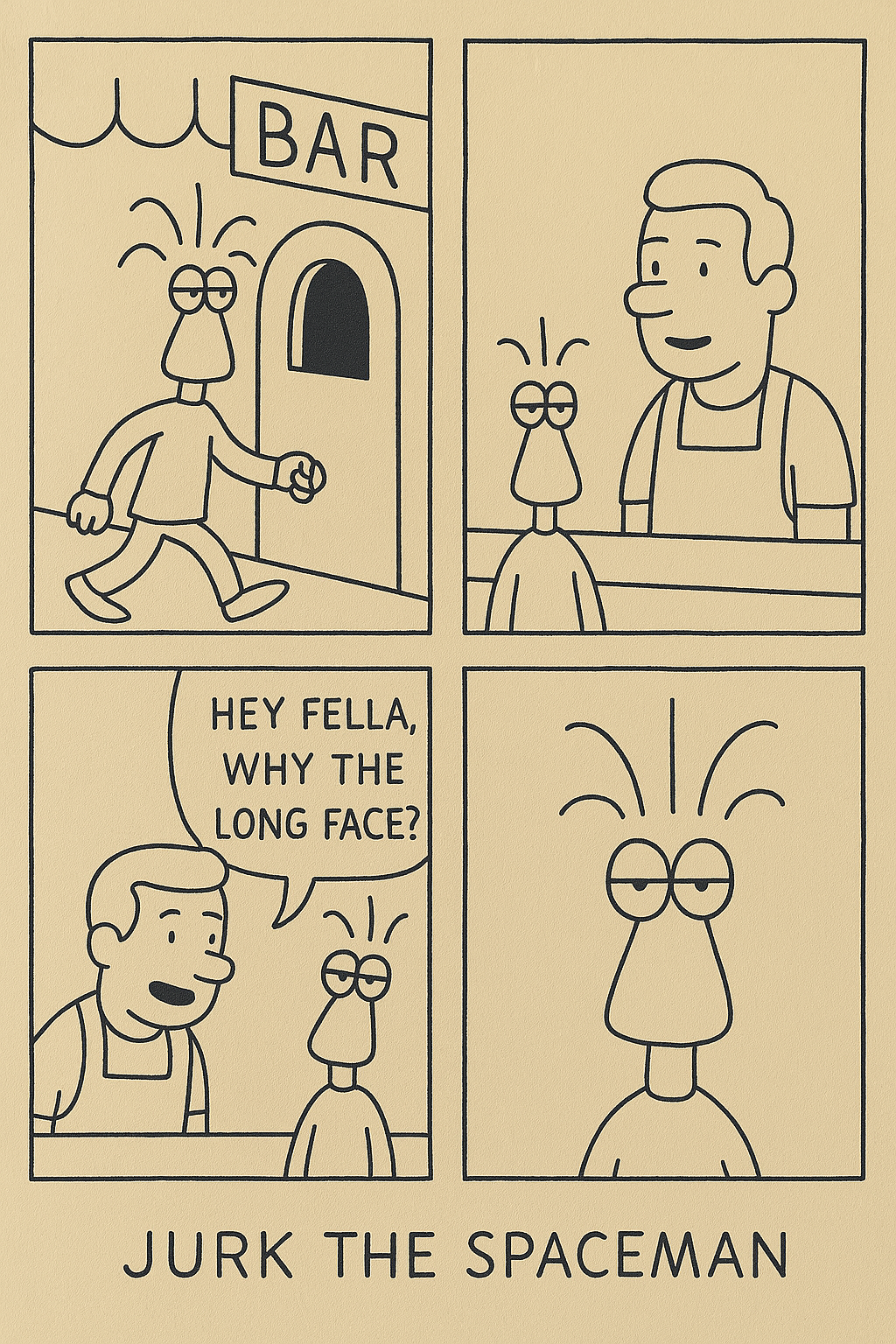
Esto, combinado con la consistencia mejorada, significa que puede crear cosas usando la generación de imágenes 4O que simplemente no eran posibles antes. Bosquejé un terrible dibujo de un alienígena de dibujos animados y pude crear una caricatura de cuatro paneles que usó ese personaje, completo con burbujas de habla con texto perfecto. Tomó más tiempo escribir el aviso que para generar mi dibujos animados completos.
4O La generación de imágenes seguirá las instrucciones
Esto es enorme. Uno de los mayores problemas que tuve con Dall-E es que a menudo se negaría a seguir una instrucción, especialmente si esa instrucción involucraba un negativo. Pasé horas tratando de generar una imagen de Santa con bigote pero sin barba (solo para ver cómo se vería, obviamente), y no importa lo que intentara, obtendría una barba completa cada vez.
La única forma en que logré acercarme al éxito fue pedirle que generara una imagen de Hercule Poirot disfrazada de Santa, e incluso entonces, tomó múltiples intentos antes de obtener una imagen sin la barba y un bigote blanco. Ahora, sin embargo, puedo obtener una imagen de Santa sin barba en el primer intento.
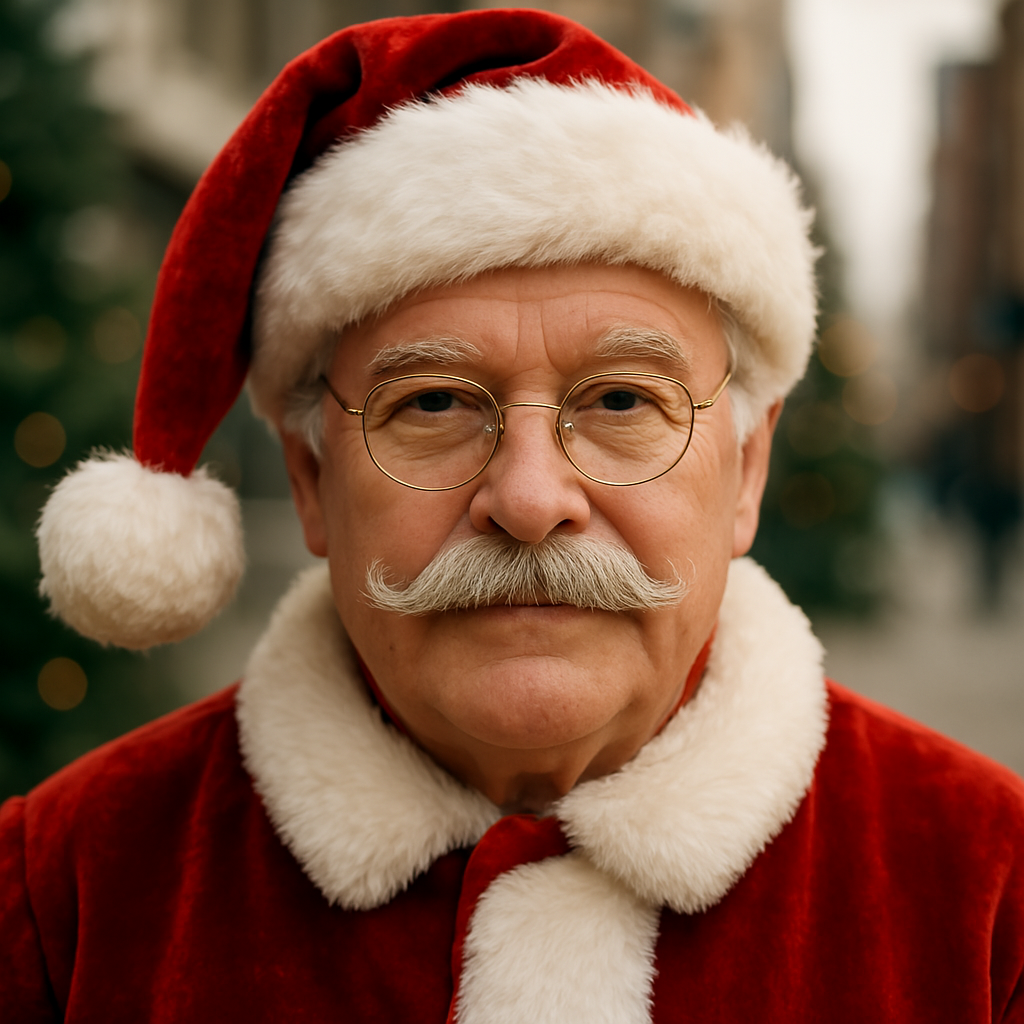
Sin embargo, la adhesión de instrucciones es aún más impresionante. Puede definir hasta 20 objetos diferentes, describiendo cada uno, y la generación de imágenes 4O seguirá las instrucciones para cada objeto. El ejemplo que ofrece OpenAI es para una cuadrícula 4×4 de emoji con formas y colores específicos, y ChatGPT puede crear una imagen con los 16 emoji exactamente como se describe.
Puede usar imágenes cargadas como referencias
Una desventaja de generar imágenes a partir de indicaciones es que describir lo que desea en una imagen puede ser difícil, pero describir el estilo de la imagen puede ser aún más difícil. Decirle a ChatGPT que produzca el aspecto exacto que tiene en la cabeza no siempre es tan fácil.
Afortunadamente, no solo necesitas usar texto. Puede cargar imágenes para indicar el tipo de estilo que desea para sus imágenes. CHATGPT usará estas imágenes para informar la imagen final que genera a partir de su mensaje.
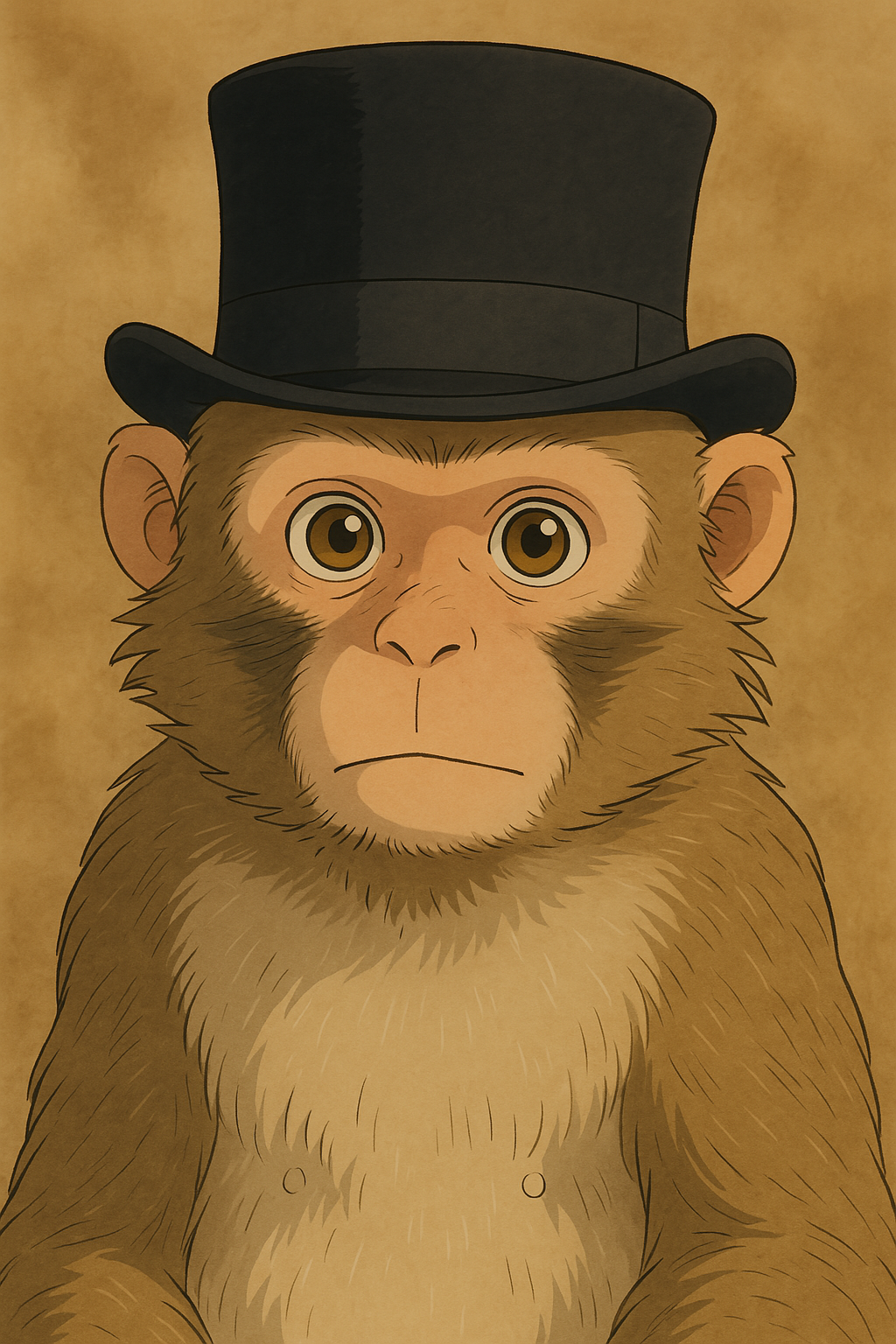
Si desea un elemento específico en su imagen, por ejemplo, puede cargar una imagen de él en ChatGPT. Si desea que las personas se encuentren en una pose específica, puede subir una imagen de personas que se encuentran en esa pose. Si encuentra una ilustración que desea que fuera una imagen fotorrealista, puede cargarla y pedirle a ChatGPT que lo convierta en una fotografía.
Incluso puede dibujar un bosquejo aproximado de cómo quiere que se vea la imagen, tomar una foto y subirlo a Chatgpt. Luego puede generar una imagen fotorrealista basada en su terrible boceto. Hace que sea mucho más fácil generar la imagen exacta que desea.
Las imágenes pueden llamar al conocimiento de Chatgpt.
La generación de imágenes 4O no se limita a la información en su mensaje o los archivos que carga. GPT-4O tiene su propia base de conocimiento a la que puede recurrir, para ayudarlo a crear las imágenes que desea. Las imágenes Studio Ghibli son un excelente ejemplo; No necesitas explicar cómo se ve Studio Ghibli Animation; Chatgpt ya lo sabe.
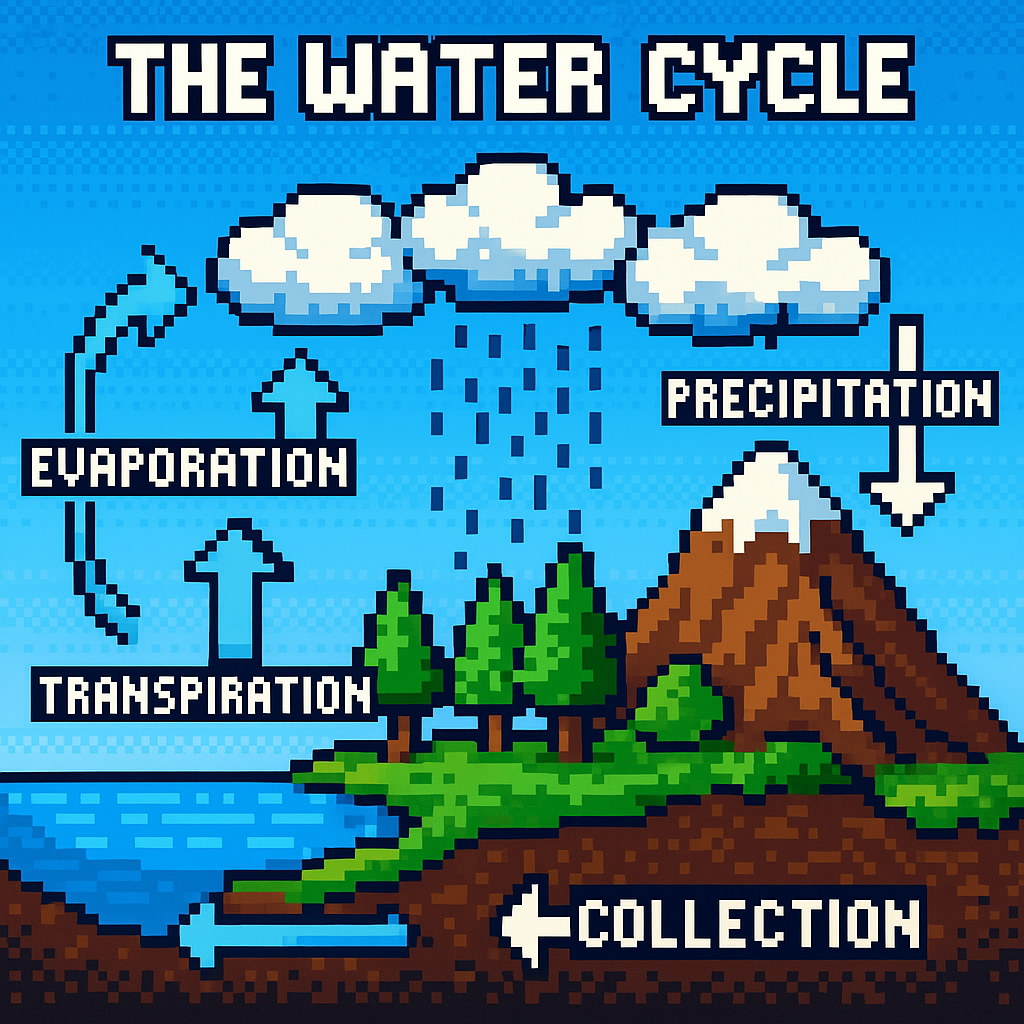
Sin embargo, esto va mucho más allá de conocer diferentes estilos artísticos. Cualquier conocimiento que ChatGPT tenga se puede aplicar a sus imágenes. Por ejemplo, puede solicitar un diagrama que explique el ciclo del agua, y no necesita explicar cuál es el ciclo del agua; ChatGPT extraerá la información clave de su propio conocimiento.
4O La generación de imágenes no es perfecta (todavía)
4O La generación de imágenes es increíblemente buena. De hecho, es tan bueno que Sam Altman, el CEO de OpenAI, tuviera que agregar límites de tarifa porque las GPU de la compañía comenzaban a derretirse.
Inicialmente, puede crear tantas imágenes como quisiera, pero ahora a menudo verá un mensaje que le dice que debe esperar unos minutos antes de crear otra imagen. No es el único problema que puede encontrar con la generación de imágenes 4O.
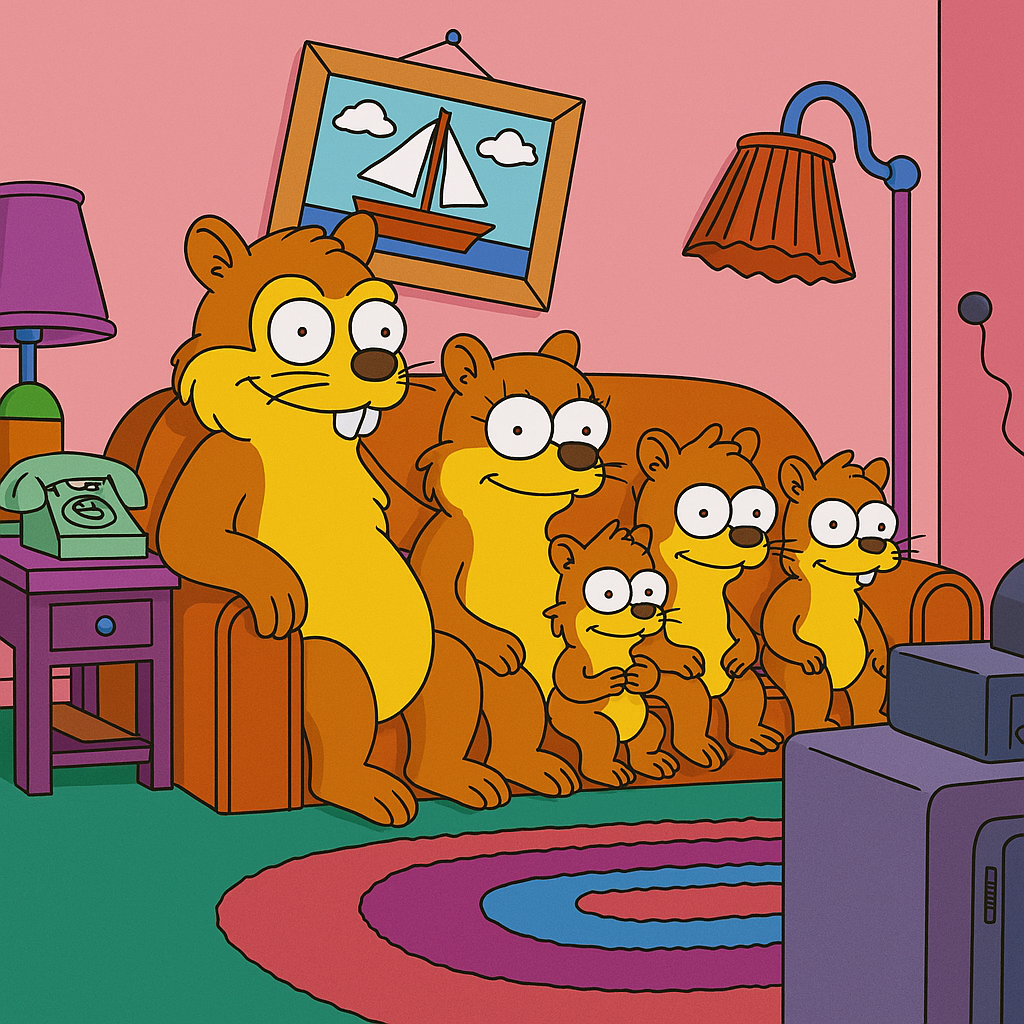
También hay limitaciones en la creación de ciertos tipos de contenido. En teoría, al menos, no debería poder generar nada ofensivo o inapropiado. Si intenta crear imágenes con caracteres con derechos de autor, ChatGPT también puede rechazar. Las líneas están un poco borrosas aquí. Por lo general, puede crear personajes de un estilo similar, si no los personajes en sí, o evitar las restricciones utilizando indicaciones ligeramente vagas.
El seguimiento de las instrucciones no siempre funciona perfectamente, y todavía ocasionalmente también tengo problemas con el texto. Ahora es muy raro, pero ocasionalmente, arrojará una letra adicional, especialmente si agregar esa letra todavía hace que el texto sea una palabra válida. Sin embargo, generalmente puede corregir fácilmente estos errores con la próxima generación.
La generación de imágenes 4O es un salto considerable en la generación de imágenes de IA, con un fotorrealismo mejorado, una mejor consistencia y una instrucción significativamente mejor. Ahora es increíblemente fácil crear imágenes fotorrealistas que se ven exactamente como quieres.
Sin embargo, hay muchas preguntas éticas que esto plantea. Si eres un diseñador gráfico o un fotógrafo, esta actualización enviará escalofríos por la columna vertebral. Lo que no se puede negar es que esta actualización ha hecho que sea mucho más fácil para los usuarios de ChatGPT crear imágenes muy impresionantes, sean cuales sean los dilemas éticos.
Noticias
‘Genere un ejercicio indicador para el niño neurodivergente’
Published
1 hora agoon
10 abril, 2025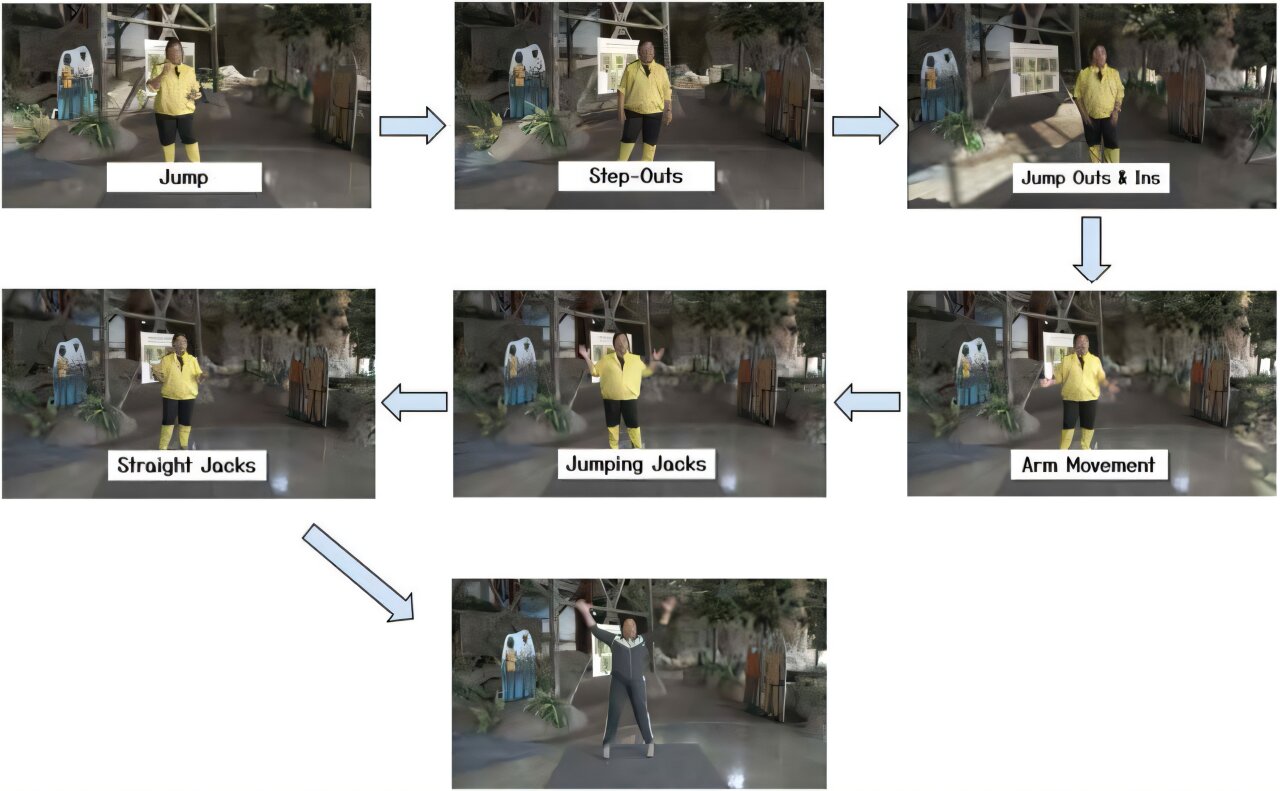
Capturas de pantalla de cada uno de los videos en la Unidad 1, que conducen a gatos rectos (una versión escalada de Jumping Jacks). La última imagen muestra el ejercicio fundamental básico de la Unidad 1, saltando con gatos, sirviendo como el calentamiento para los videos de la Unidad 2. Crédito: Fronteras en fisiología (2025). Doi: 10.3389/fphys.2024.1496114
Un equipo de investigación de la Universidad de Michigan ha utilizado la IA para ayudar a entregar “bocadillos” de ejercicio a los niños con autismo.
El equipo de investigación, dirigido por la fisióloga del ejercicio UM Rebecca Hasson, revisó un programa que tenía como objetivo ayudar a los niños a hacer ejercicio cuando las familias tuvieron que pivotar al aprendizaje en línea durante la pandemia Covid-19. Ahora, un equipo de investigadores de la Escuela de Kinesiología utilizó ChatGPT para revisar las instrucciones para los 132 videos de ejercicios del programa, adaptándolos para satisfacer mejor las necesidades de los niños neurodivergentes. Sus resultados se publican en la revista Fronteras en fisiología.
En 2012, durante “¡Movernos” de Michelle Obama! Campaña para combatir la obesidad infantil, Hasson comenzó a trabajar en formas de incorporar la actividad física en la vida cotidiana de los niños. Ella y otros investigadores desarrollaron formas de integrar la actividad física en los días escolares de los niños sin interrupciones importantes, y utilizaron la idea de “ejercicios de bocadillos”, períodos de ejercicio de aproximadamente tres a cuatro minutos.
Llamaron al programa Inpact, o interrumpieron la sesión prolongada con actividad. El programa se ha implementado en 25 escuelas de Michigan hasta la fecha.
“Tuvimos estas grandes oportunidades de capacitación, los maestros se sentían apoyados, los niños se movían más, y luego teníamos la pandemia”, dijo Hasson. “La casa se convirtió en el aula, y la actividad física de los niños cayó significativamente. Así que tuvimos que descubrir cómo adaptar lo que estábamos haciendo en el aula al entorno local”.
Los investigadores se pusieron inmediatamente a trabajar adaptando Inpact para el entorno doméstico. Para llegar a los niños que podrían no tener acceso a Internet, los investigadores trabajaron con el canal de aprendizaje de Michigan de PBS y el Departamento de Educación para transmitir el programa de actividades físicas en la televisión. En seis meses, el programa de televisión tenía 15,000-20,000 espectadores todos los días.
Si bien el programa fue un éxito, el investigador Haylie Miller, psicólogo del desarrollo y profesor asistente de ciencia del movimiento, notó que los niños neurodivergentes podrían tener dificultades para seguir las instrucciones en los videos del ejercicio, ya que fueron diseñados para la forma en que los niños neurotípicos aprendieron. Miller estudia cómo las personas neurodivergentes usan información visual para planificar, ejecutar y modificar el movimiento.
El equipo decidió adaptar en colaboración el programa INPACT para satisfacer mejor las necesidades de acceso de los niños neurodivergentes.
“Las personas neurodivergentes procesan información sensorial de manera diferente a las personas neurotípicas, lo que puede hacer que la actividad física sea incómoda, difícil o intimidante”, dijo Miller. “Las personas neurodivergentes también tienen diferencias en la conciencia corporal y el procesamiento del lenguaje, y pueden necesitar más o diferentes indicaciones para sentirse cómodos realizando una actividad”.
Por ejemplo, dice que si una persona tiene dificultades para el equilibrio, la coordinación de sus extremidades y realiza una tarea de varios pasos, es probable que necesite más apoyo físico o instructivo para tener éxito en un movimiento complejo como un gato de salto.
El equipo comenzó a examinar los 132 videos de ejercicios desarrollados para el programa INPACT, cada uno de los cuales incluía múltiples ejercicios. La estudiante de pregrado Tania Sapre tuvo la tarea de comenzar a adaptar las instrucciones de ejercicio de los videos para ser más inclusivos a las personas neurodivergentes.
“Comencé a jugar con ChatGPT para inspirarme sobre cómo debería formatear mis instrucciones cuando de repente me di cuenta de que ChatGPT podría ayudar a llenar el vacío de conocimiento y la sobrecarga de datos que estaba experimentando”, dijo Sapre.
“Pensé que si pudiera perfeccionar el uso de ChatGPT para mis instrucciones, podría crear un proceso simple que podría ser replicado por otros investigadores, maestros y familias en el hogar para abordar ejercicios novedosos que nuestro programa no cubrió, ayudando a los niños a mantenerse activos en todas partes”.
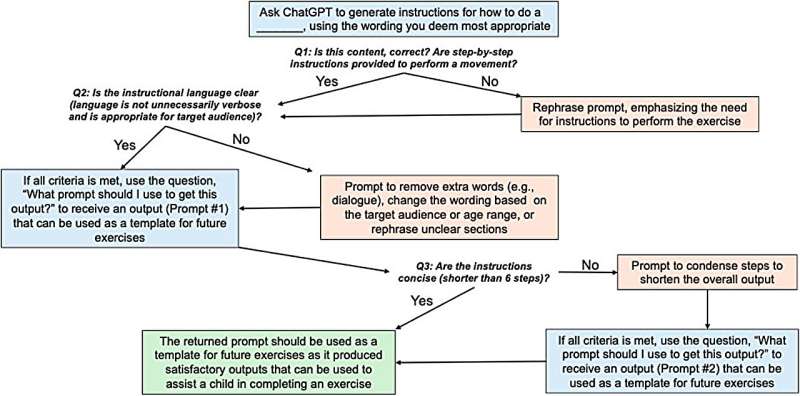
El árbol de decisión utilizado para generar inicialmente las indicaciones de ChatGPT que devuelven instrucciones de ejercicio satisfactorias. Crédito: Fronteras en fisiología (2025). Doi: 10.3389/fphys.2024.1496114
Primero, el equipo organizó su contenido de video para poder formar consultas para enviar a ChatGPT. Desde los 132 videos Inpact en Home, los investigadores identificaron más de 500 actividades. Luego categorizaron estas actividades en grupos de habilidades principales: saltos, núcleo, lateral, deporte, parte superior del cuerpo, parte inferior del cuerpo y movimientos compuestos.
Luego desarrollaron un aviso para obtener un conjunto de instrucciones para un ejercicio particular de ChatGPT. Por ejemplo, los investigadores le pidieron a ChatGPT que “proporcionara instrucciones simplificadas paso a paso para un gato de salto, adecuado para un niño neurodivergente”. Según la respuesta de ChatGPT a esa pregunta, los investigadores le pidieron a la herramienta AI que “condensara las instrucciones paso a paso para un gato de salto, adecuado para un niño neurodivergente”.
El equipo revisó cada conjunto de instrucciones para garantizar que las instrucciones generadas por IA fueran elaboradas correctamente. Los investigadores también aseguraron que las instrucciones siguieron a un principio central de su programa de ejercicios, las “tres C”: consistencia, concisión y claridad.
“El equipo de Inpact ya estaba en el camino correcto hacia el diseño universal mediante el uso de modelado de video y indicaciones verbales. Tener múltiples medios de representación realmente puede ayudar a una persona neurodivergente a comprender la actividad sin tener que adivinar”, dijo Miller.
“Nos hemos basado en esta sólida base al simplificar el lenguaje utilizado para describir cada movimiento, dividir los movimientos en sus componentes de manera más explícita y usar una redacción constante en todas las actividades para reducir la ambigüedad”.
Alanna Price es coordinadora de salud regional para su distrito de Michigan. Supervisa la educación en la salud y la educación del conductor, y ayudó a revisar las instrucciones para los estudiantes neurodivergentes.
“La educación física adaptativa es necesaria porque asegura que todos los niños puedan participar en actividades físicas modificadas a sus habilidades. Esto promueve la salud física, las habilidades sociales y el bienestar emocional”, dijo Price. “Los programas APE modifican la educación física tradicional para satisfacer las necesidades únicas de todos los estudiantes, ayudándoles a desarrollar habilidades motoras, fuerza y coordinación.
“Las direcciones de ejercicio modificadas para niños neurodivergentes, como aquellos con autismo o TDAH, pueden mejorar su desarrollo físico y cognitivo. Estas modificaciones a menudo incluyen movimientos simplificadores y palabras de indicación, utilizando ayudas visuales e incorporando actividades sensoriales”.
El equipo también ha comenzado a desarrollar un “paquete de inicio” de tarjetas de juego de actividades para personas con mayores necesidades de apoyo que puedan necesitar desarrollar más habilidades fundamentales antes de sumergirse en la experiencia completa de Inpact, dijo Miller.
“Durante la pandemia, hubo menos recursos para los padres que tuvieron hijos que aprenden de manera diferente. En el futuro, esperamos ser más proactivos en lugar de reactivos, pero esta ha sido una gran experiencia de aprendizaje para todos nosotros, y creo que habla del valor de estar abierto a las críticas”, dijo Hasson.
“Si su misión realmente es avanzar en las oportunidades para que todos los niños estén activos, entonces estar dispuesto a asociarse con otros para que eso suceda es realmente importante”.
Además del paquete de inicio, los investigadores esperan tener sus videos traducidos al español y el árabe, los dos idiomas hablados con mayor frecuencia en Michigan después del inglés.
Más información:
Tania Sapre et al, mejorando la actividad física en el hogar para niños neurodivergentes: adaptando el programa Inpact at Home con IA y diseño universal, Fronteras en fisiología (2025). Doi: 10.3389/fphys.2024.1496114
Proporcionado por la Universidad de Michigan
Citación: Hola, Chatgpt: ‘Genere un mensaje de ejercicio para el niño neurodivergente’ (2025, 10 de abril) Consultado el 10 de abril de 2025 de https://medicalxpress.com/news/2025-04-chatgpt-generate-prompt-neurodivergent-child.html
Este documento está sujeto a derechos de autor. Además de cualquier trato justo con el propósito de estudio o investigación privada, no se puede reproducir ninguna parte sin el permiso por escrito. El contenido se proporciona solo para fines de información.
Related posts
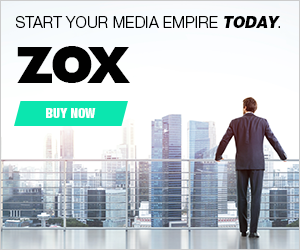
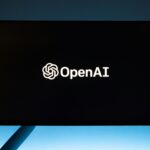
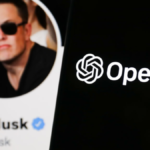
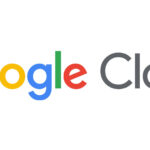
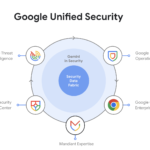
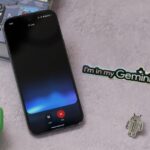
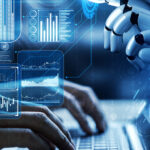
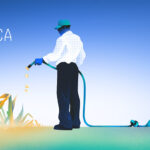
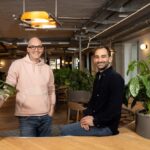
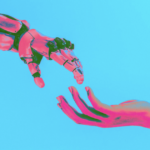
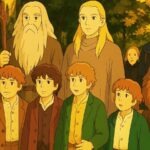
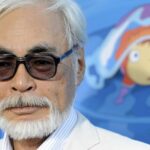
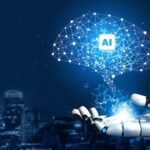
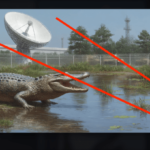
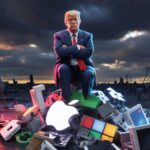
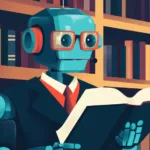
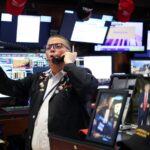
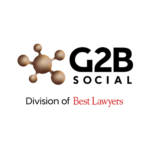
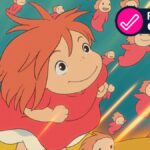
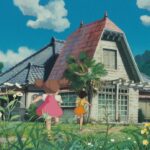
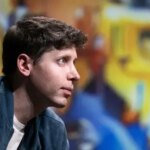
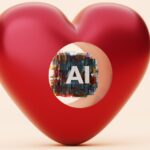
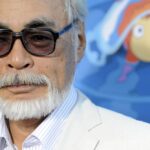
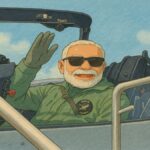
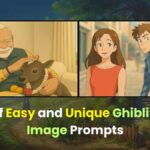
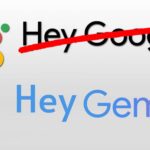
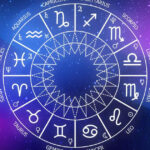
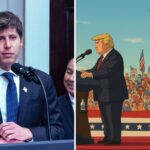
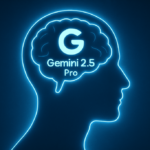
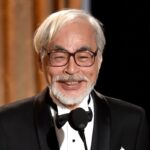
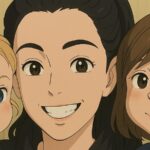
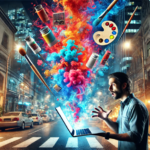
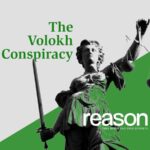
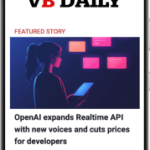
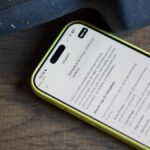
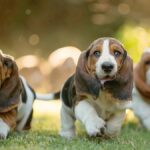
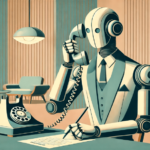
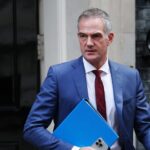
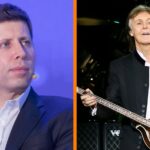
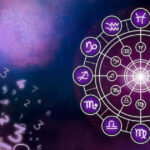
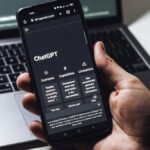
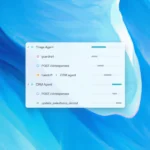
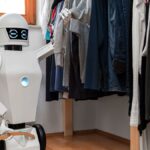
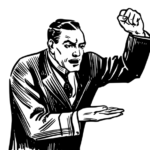
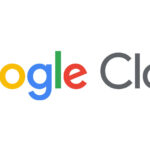
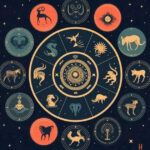
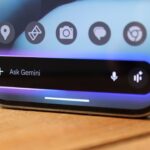
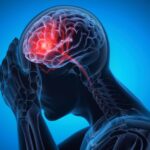
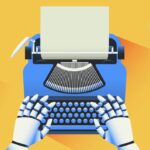
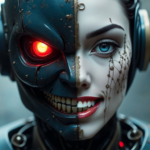
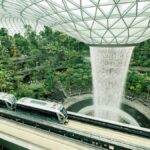
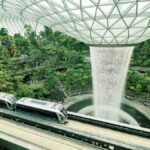
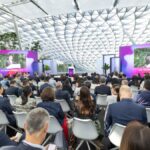
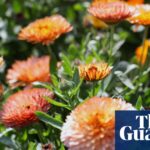

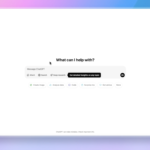
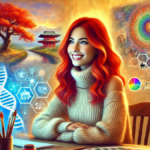
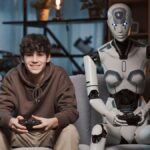
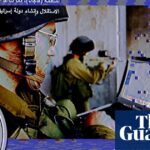
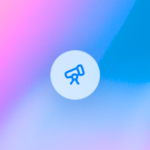
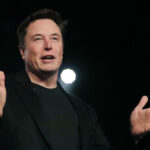
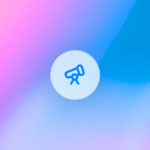
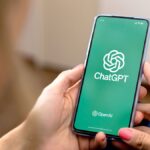
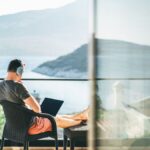
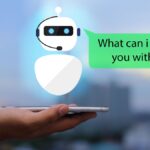
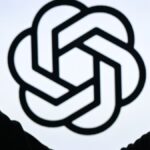
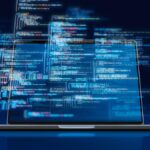
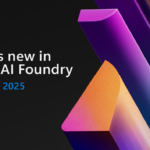
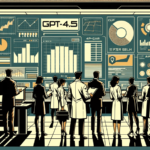
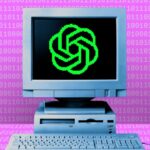
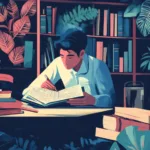
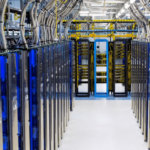
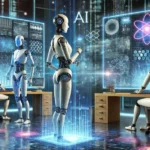
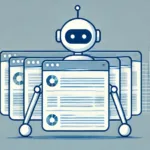
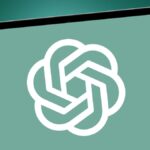
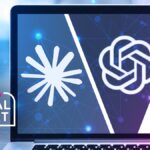
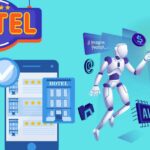
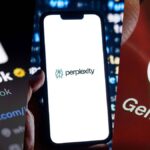
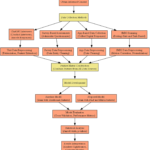
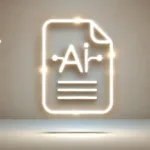
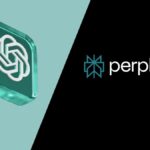
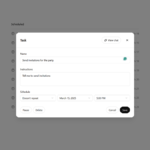
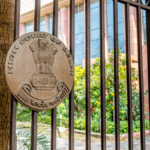
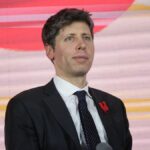
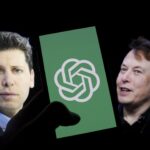
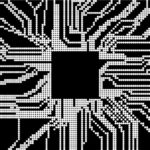
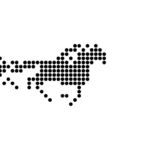
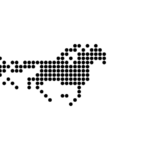
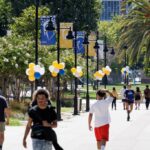
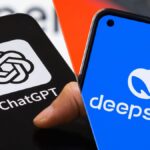
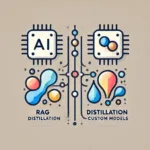
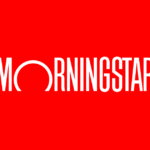
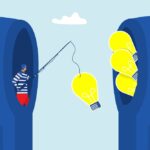
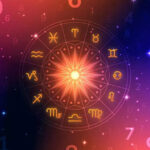
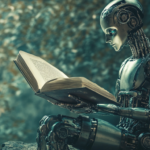
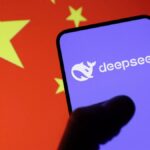
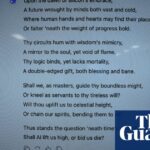
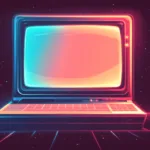

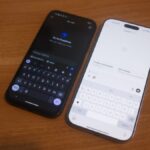
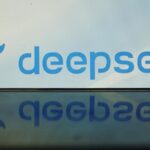

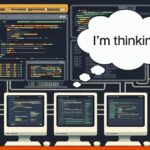
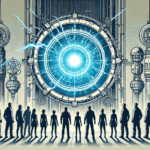
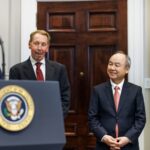
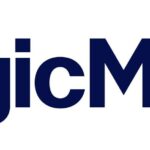
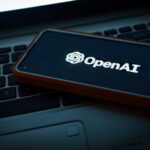

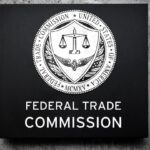
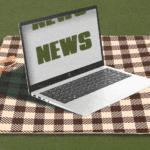
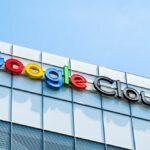
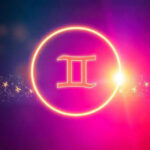
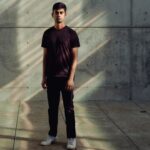
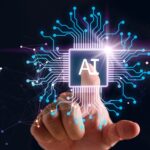
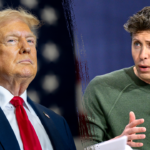
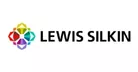
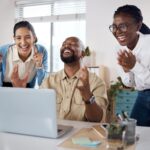
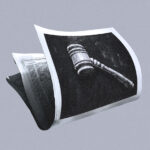
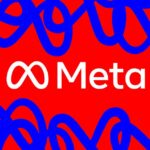

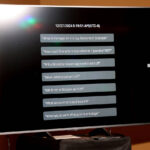

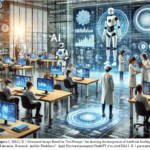
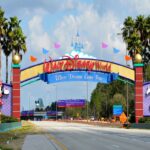
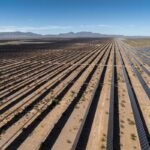
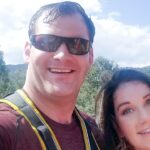
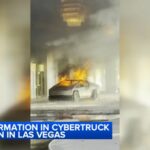
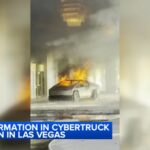
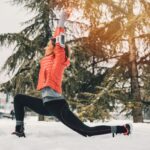
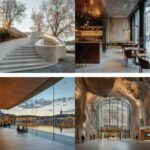
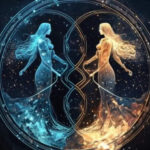
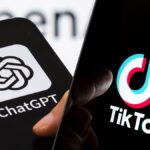
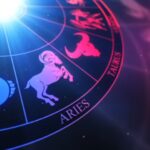
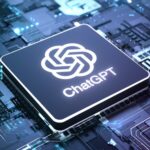
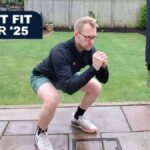
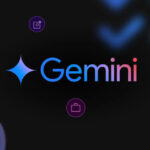
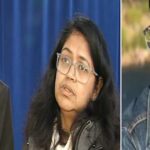
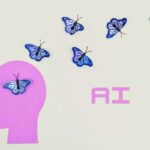
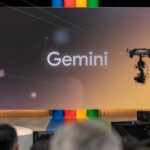
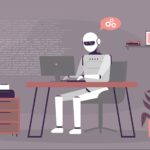
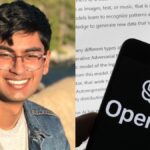
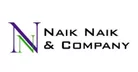

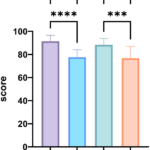
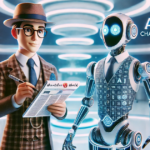
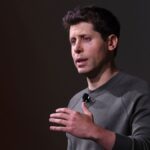
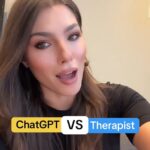
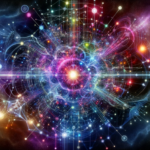
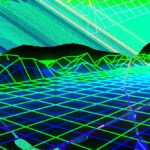
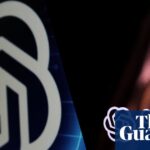
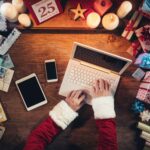
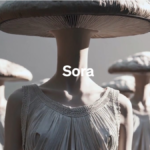
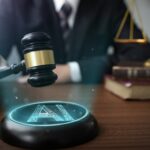
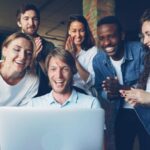
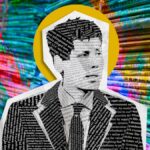

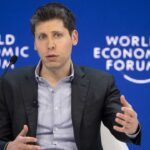
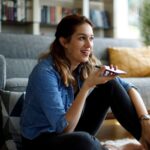
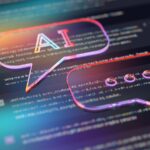
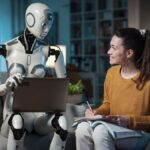
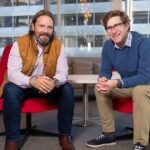
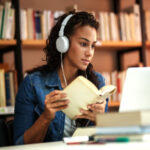
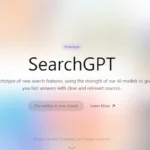
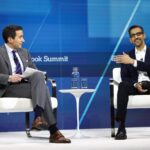
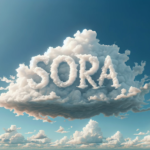
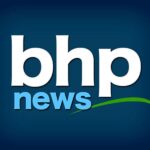
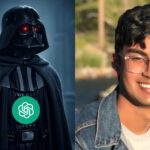
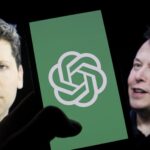
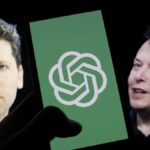
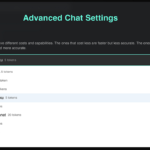
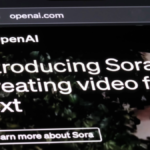
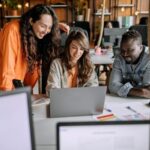
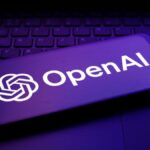
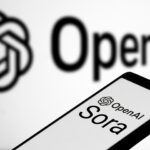
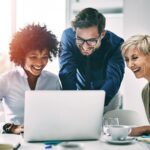
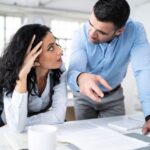
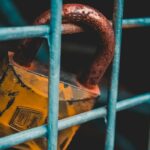
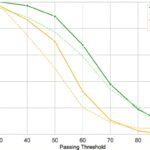
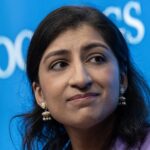
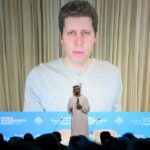
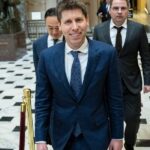
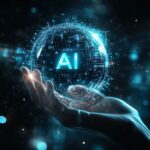
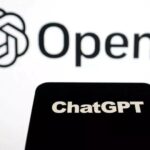
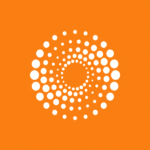
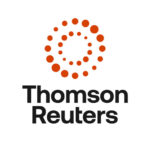
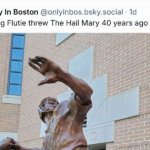

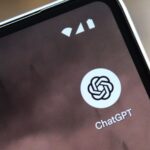
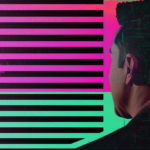
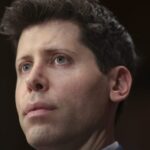
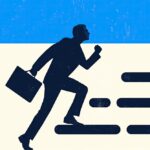
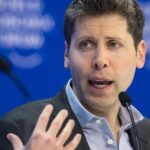
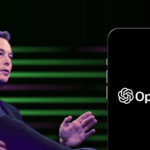
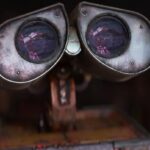
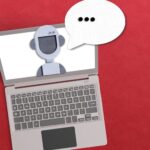
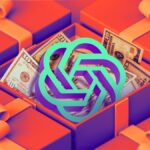
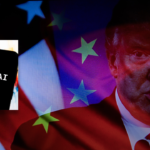
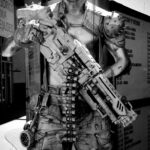
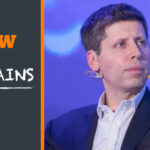
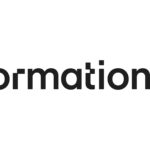
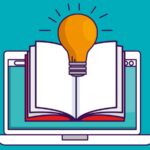
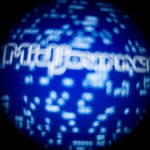
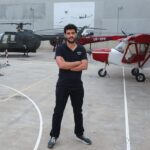
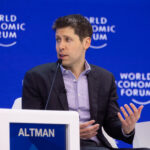
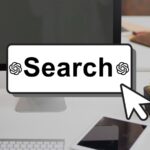
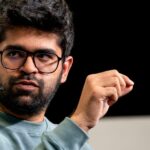
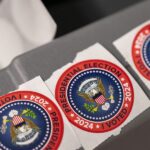
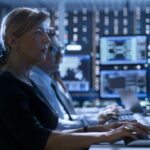
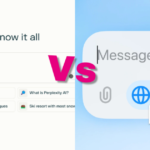
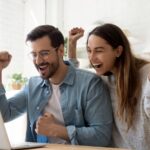
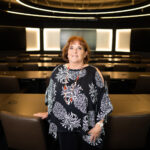
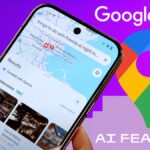
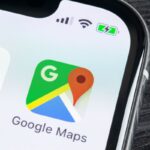
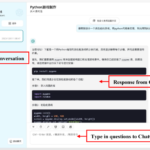
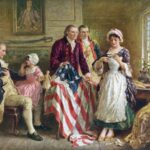
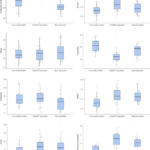

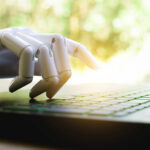
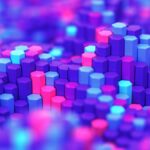
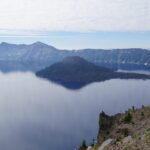
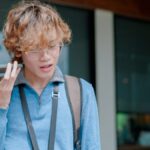
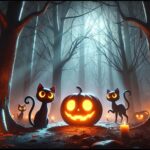
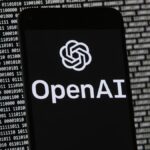
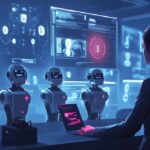
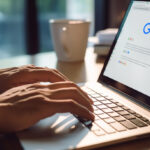
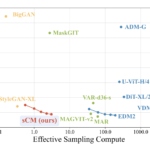
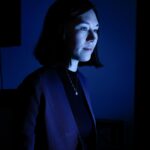
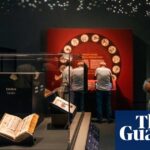
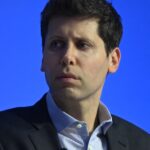
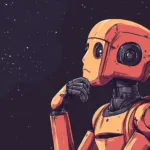
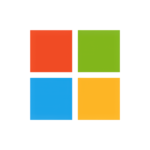
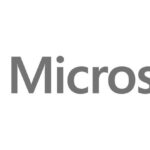
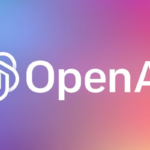
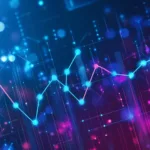
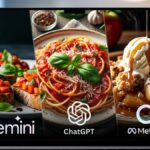
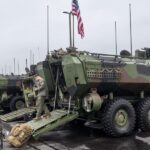

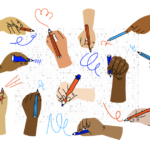
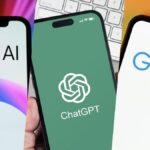
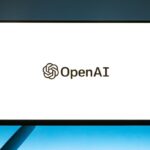
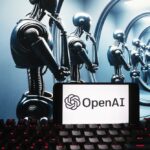
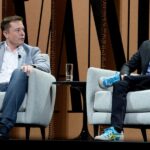
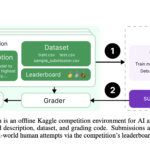
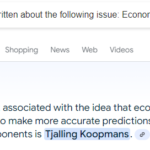
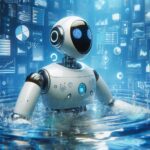
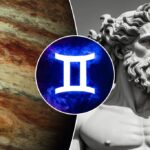
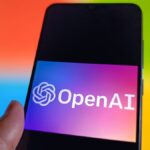
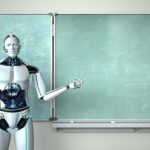
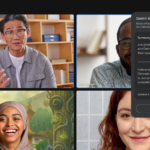
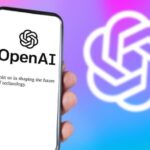
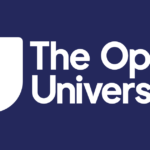
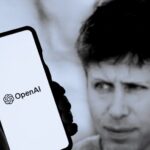
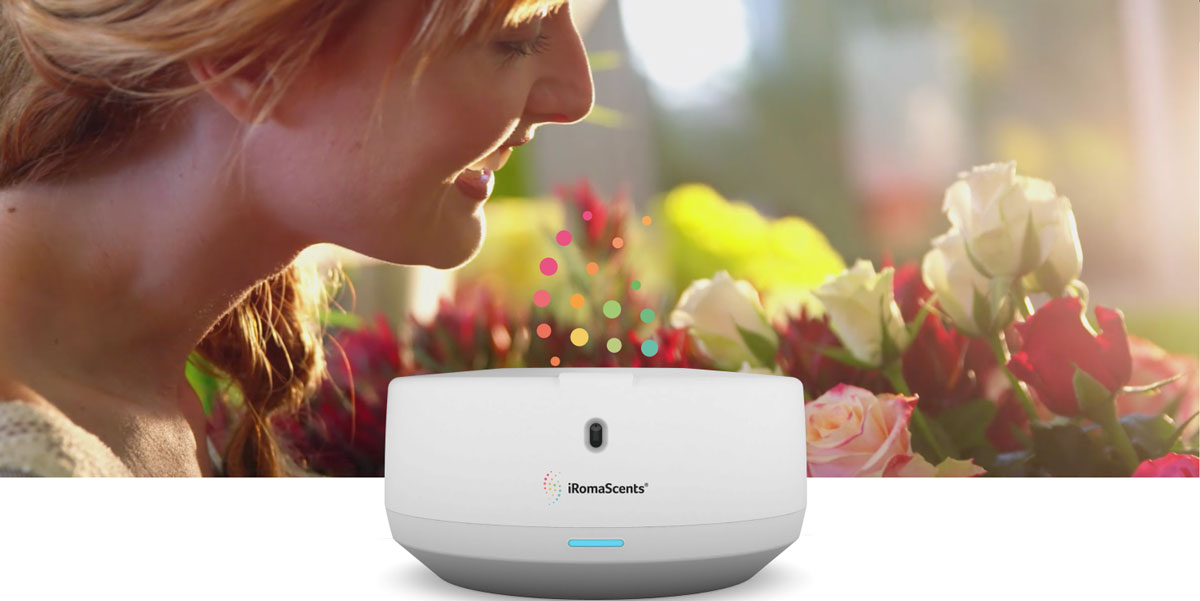

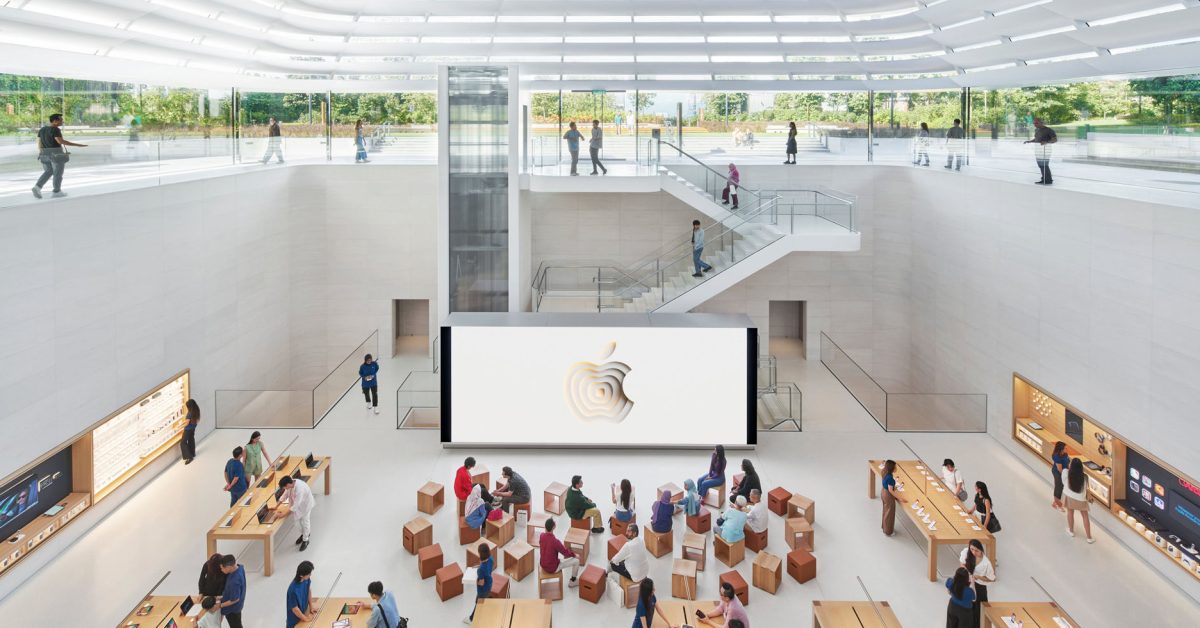


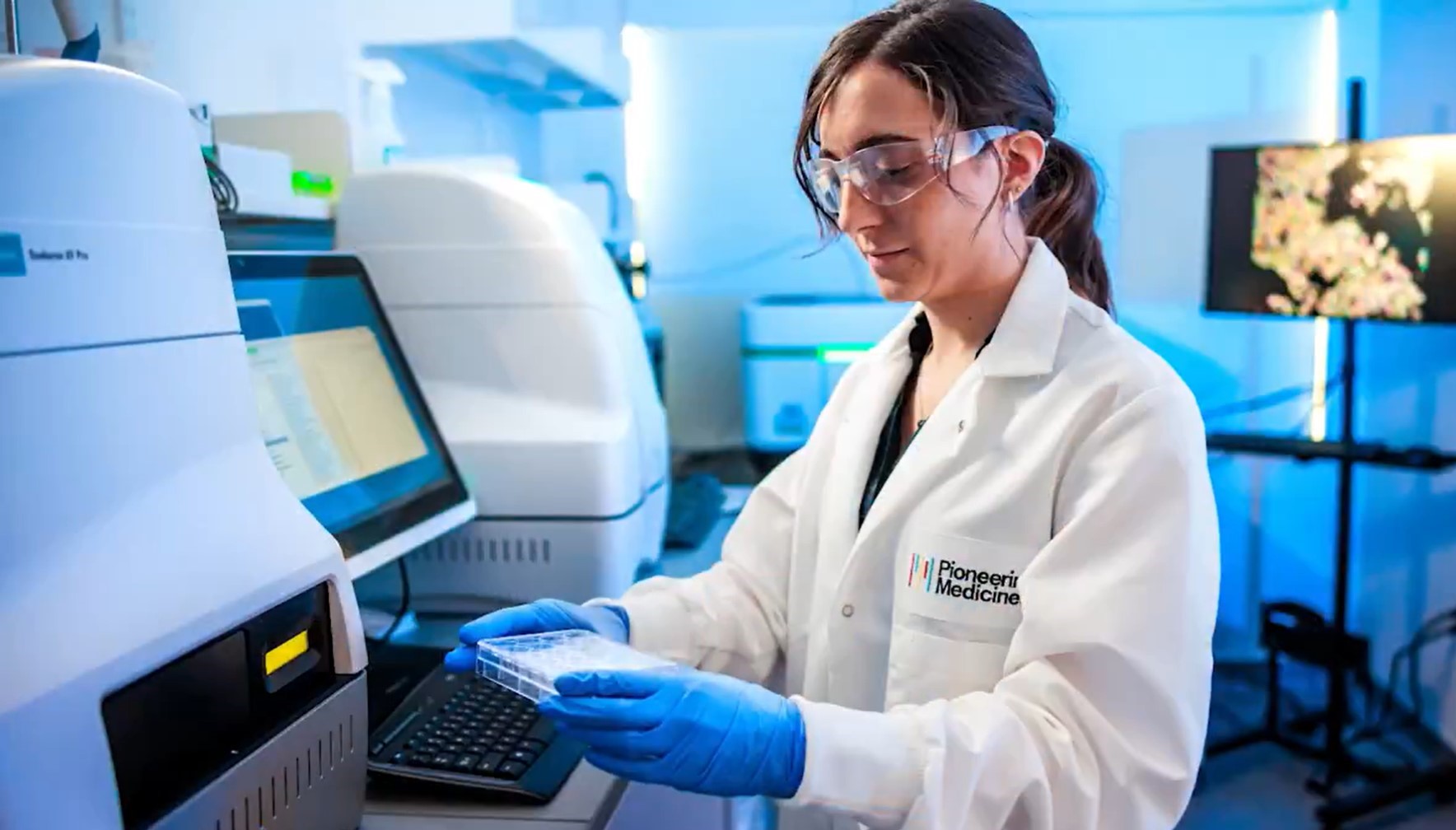
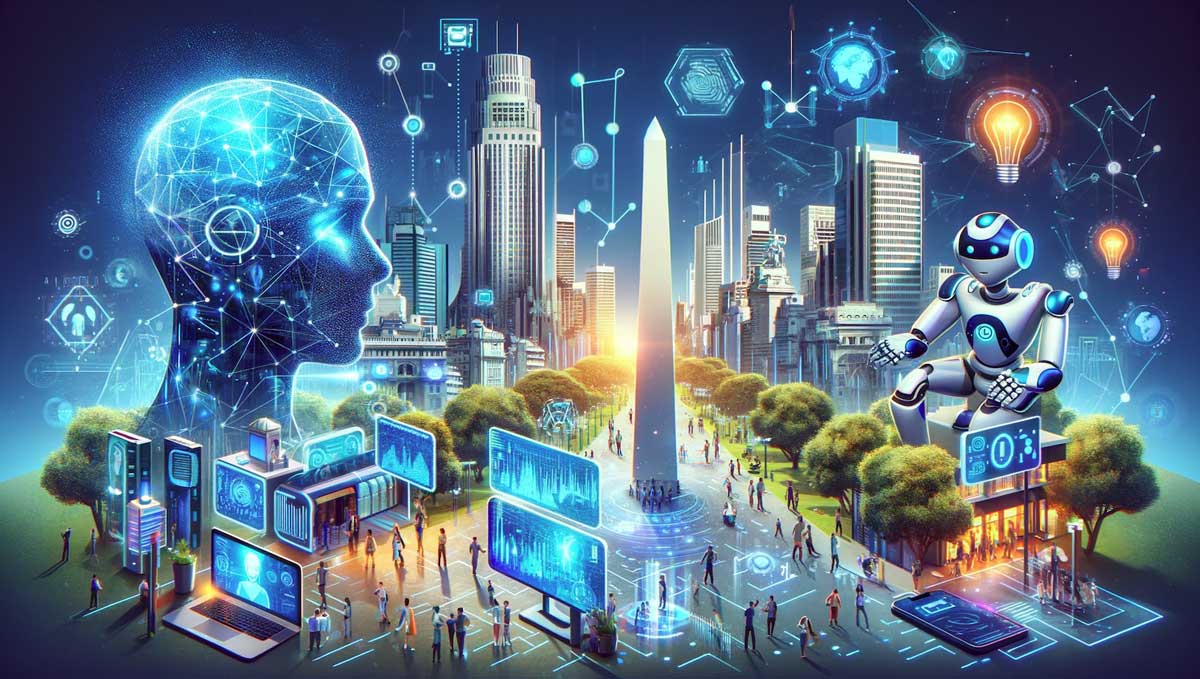

Trending
-
Startups11 meses ago
Remove.bg: La Revolución en la Edición de Imágenes que Debes Conocer
-
Tutoriales11 meses ago
Cómo Comenzar a Utilizar ChatGPT: Una Guía Completa para Principiantes
-
Startups9 meses ago
Startups de IA en EE.UU. que han recaudado más de $100M en 2024
-
Recursos11 meses ago
Cómo Empezar con Popai.pro: Tu Espacio Personal de IA – Guía Completa, Instalación, Versiones y Precios
-
Startups11 meses ago
Deepgram: Revolucionando el Reconocimiento de Voz con IA
-
Recursos11 meses ago
Perplexity aplicado al Marketing Digital y Estrategias SEO
-
Recursos11 meses ago
Suno.com: La Revolución en la Creación Musical con Inteligencia Artificial
-
Noticias9 meses ago
Dos periodistas octogenarios deman a ChatGPT por robar su trabajo