Noticias
OpenAI Newly Released AI Product ‘Swarm’ Swiftly Brings Agentic AI Into The Real World
Published
7 meses agoon

Here’s what you need to know about the latest in agentic AI and the release of OpenAI’s new Swarm.
getty
In today’s column, I examine the newly announced OpenAI product called Swarm and explain how this significant unveiling brings the emerging realm of agentic AI into tangible reality.
There is increasing momentum regarding agentic AI as the future next-stretch for the advent of advances in generative AI and large language models or LLMs. Anyone interested in where AI is going ought to be up-to-speed about Swarm since it comes from OpenAI, the 600-pound gorilla or big whale when it comes to advances in generative AI.
Let’s talk about it.
This analysis of an innovative proposition is part of my ongoing Forbes.com column coverage on the latest in AI including identifying and explaining various impactful AI complexities (see the link here).
Agentic AI Fundamentals
Before I do the unpacking of Swarm, I want to make sure we are all on the same page about agentic AI. I’ll provide the keystones of interest. For my detailed coverage of agentic AI, see the link here.
Here’s the deal.
Imagine that you are using generative AI to plan a vacation trip. You would customarily log into your generative AI account such as making use of the widely popular ChatGPT by OpenAI. The planning of your trip would be easy-peasy due to the natural language fluency of ChatGPT. All you need to do is describe where you want to go, and then seamlessly engage in a focused dialogue about the pluses and minuses of places to stay and the transportation options available.
When it comes to booking your trip, the odds are you would have to exit generative AI and start accessing the websites of the hotels, amusement parks, airlines, and other locales to buy your tickets. Few of the major generative AI available today will take that next step on your behalf. It is up to you to perform those tasks.
This is where agents and agentic AI come into play.
In earlier days, you would undoubtedly phone a travel agent to make your bookings. Though there are still human travel agents, another avenue would be to use an AI-based agent that is based on generative AI. The AI has the interactivity that you expect with generative AI. It also has been preloaded with a series of routines or sets of tasks that underpin the efforts of a travel agent. Using everyday natural language, you interact with the agentic AI which works with you on your planning and can proceed to deal with the nitty-gritty of booking your travel plans.
As a use case, envision that there is an overall AI agent that will aid your travel planning and booking. This agentic AI might make use of other AI agents to get the full job done for you. For example, there might be an AI agent booking hotels and doing nothing other than that specific task. Another AI agent books flights. And so on.
The overarching AI travel agent app would invoke or handoff phases of the travel booking activity to the respective AI agents. Those AI agents would perform their particular tasks and then go back to the overarching AI travel agent to indicate how things went.
You could say that the AI travel agent app is orchestrating the overall planning and booking process. This is done via a network of associated AI agents that undertake specialized tasks. The AI agents communicate with each other by passing data back and forth. For example, you might have given your name and credit card info to the AI travel agent app and it passes that along to the AI agent booking the hotel and the AI agent booking your flights.
In a sense, the AI agents are collaborating with each other. I somewhat hesitate to use the word “collaborate” because that might imply a semblance of sentience and overly anthropomorphize AI. Let’s just agree that the AI agents are computationally interacting with each other during the processing of these tasks. We will be a bit generous and suggest they are being collaborative.
Those Agentic AI Advantages
The beauty of this arrangement is that if the AI agents are all based on generative AI, the setup can make use of natural language to bring all the agents together and engage them in working with you interactively. A normal computer program that isn’t based on natural language capabilities would either not interact in a natural language manner, or the collaboration between the various routines or separate apps would have to be programmatically devised.
These AI agents can also make use of tools during their processing. The AI travel agent might have a backend database that keeps track of your various trips. To access the database, the AI travel agent invokes a tool that was built to record data in the database. By using such tools, each AI agent can leverage other available programs that aren’t necessarily natural language based.
I have now introduced you to some of the key terminology associated with agentic AI, consisting of these six primary considerations:
- (1) Orchestration. A generative AI agent will at times orchestrate the use of other AI agents and conduct them toward fulfilling a particular purpose or goal.
- (2) Network of AI agents. Various AI agents are often considered part of a virtual network that allows them to readily access each other.
- (3) Communicate with each other. AI agents are typically set up to communicate with each other by passing data back and forth and performing handoffs with each other to get things done.
- (4) Collaborate with each other. AI agents work in concert or collaborate, though not quite as robustly as humans would, so we’ll loosely say the AI kind of collaborates computationally, including doing handoffs and passing data to each other.
- (5) Autonomously perform tasks. AI agents are said to be at times autonomous in that a human does not necessarily need to be in the loop when the various tasks are being performed by the AI.
- (6) Expressed in natural language. The beauty of AI agents that are devised or based on the use of natural language is that rather than having to laboriously write program code to get them to do things, the use of natural language can be leveraged instead.
Shifting Into The OpenAI Swarm
OpenAI recently announced and made available access to their new product known as Swarm.
I will be quoting from the OpenAI blog about Swarm as posted on October 9, 2024. For those of you interested in actively trying out Swarm, right now it is considered experimental, and you’ll need to use the code that OpenAI has made available on GitHub. If you have sufficient Python coding skills and know how to make use of the generative AI APIs or application programming interface capabilities, you should be able to quickly try out the new product.
This is a one-liner by OpenAI that describes what Swarm is:
- “An educational framework exploring ergonomic, lightweight multi-agent orchestration.”
Swarm is essentially an experimental and educational setup to get agentic AI underway by OpenAI and provides AI developers with a means of trying out agentic AI capabilities. I suppose that the name Swarm refers to the idea that you can have a whole bunch of AI agents working together. In addition, if you think of swarms such as a swarm of bees, swarms often have some overall purpose, such as bees defending against a perceived invader.
The OpenAI blog description quoted above says that the AI agents are lightweight. This suggests that the AI agents are somewhat narrowly scoped and not heavy-duty in terms of any particular agent doing a huge amount of work entirely on its own. That is also where the multi-agent aspects come to the fore. You are presumably going to use lots of said-to-be lightweight AI agents and orchestrate them together to achieve a noted end goal.
An Example Of Agentic AI In Action
The GitHub site and the blog about Swarm showcase some examples of how things work. I have opted to make up my own example and loosely based it on the official ones they posted. I am going to leave out the Python coding to make this example easier to comprehend. By and large, the example generally exemplifies the core essence involved.
My scenario is this.
A company I’ll name as the Widget Corporation wants to develop an automated Customer Support Agent using generative AI. This will be made available to existing customers. A customer will interact directly with the AI agent. The AI agent will find out what the customer’s concerns are. Based on those concerns, the AI agent will attempt to provide a potential resolution. If a resolution is not feasible, the customer will be able to return the item that they bought and get a refund.
I’d dare say this is a pretty common task and usually involves a series of subtasks.
The usual approach for a software developer would be to code this from scratch. It could take gobs of hours to write the code, test it, and field it. Instead, we will use agentic AI and indicate the primary agent, a Customer Support Agent, via the use of natural language.
To illustrate the notion of communication and collaboration, I will define two agents, a Customer Support Agent (considered an AI agentic “Routine” and my primary agent) and a second agent that is Refunds And Returns Agent (considered another AI agentic “Routine” and used by the primary agent). They will do handoffs and make use of tools.
Here is my definition of the Customer Support Agent.
- AI agent routine with tool use and a handoff: Customer Support Agent
“You are a customer support agent for the Widget Corporation.”
“Follow this standard routine:
“(1) When a customer contacts you, make sure to ask sufficient questions to grasp what their customer support issue consists of.”
“(2) Access the Widget Corp internal customer support database WidgetSys to see if any similar issues have ever been logged.”
“(3) Try to come up with a solution for the customer that will resolve their support issue.”
“(4) Provide the proposed solution to the customer and get their feedback.”
“(5) If the customer wants to do a product return and get a refund then invoke the Returns And Refunds Agent and provide relevant details about the customer.”
End of definition
I’d like you to notice that the definition is written in natural language.
If you fed that same text into generative AI such as ChatGPT as a prompt, the AI would generally be able to proceed.
Give that a reflective moment of thought. Imagine the vast amount of arduous coding or programming you would have to write to do the same thing. All we had to do here was express what we wanted via the use of everyday natural language.
Boom, drop the mic.
Inside the natural language description in Step #2, I refer to a tool, the WidgetSys tool. This is a program that the Widget Corporation has developed to access its internal customer service records database.
In Step #5, I mention another AI agent, known as the Returns And Refunds Agent. This is a handoff activity that will occur when Step #5 is performed. In addition, I indicated that relevant customer data should be passed over.
The Allied AI Agent For This Example
Now that you’ve seen the primary AI agent, let’s take a look at the allied AI agent.
Here it is.
- AI agent routine with tool use and a handoff: Returns And Refunds Agent
“You are a product returns and refund agent for the Widget Corporation.”
“Follow this standard routine:”
“(1) Ask the customer if they want to return the product and get a refund.”
“(2) If the customer says no then go back to Customer Support Agent.”
“(3) Access the WidgetSys database to mark that the product is being returned and the customer will be given a refund.”
“(4) Tell the customer how to return the product and let them know they will be given a refund.”
“(5) Go back to Customer Support Agent and inform that the return and refund processing is now underway.”
End of definition
Once again, the AI agent is defined via the use of natural language.
A handoff back to the primary agent happens in Step #2. Access to the tool WidgetSys takes place at Step #3. Another handoff back to the primary agent occurs in Step #5.
This allied AI agent takes on the task of processing a potential item return and refund. This could have been embedded entirely in the Customer Support Agent, but it turns out to be better for us to make it into a separate routine. Doing so means that we can always make use of the AI agent from other agentic AI that might need to invoke that specific task.
Vital Considerations About These AI Agents
Let’s be contemplative and mindfully explore the big picture. Life is always full of tradeoffs. The use of AI agents is no exception to that rule of thumb. You’ve seen first-hand that a notable plus is the ease of development via natural language.
Time to discuss some of the downsides or qualms.
I provided five steps for the Customer Support Agent and another five steps for the Returns And Refunds Agent. Is that sufficient to cover the wide range of aspects that might arise when successfully performing a customer support role?
Probably not.
Okay, so we might proceed to add more steps. But does that really solve the dilemma of completeness? Probably not. You aren’t likely to lay out all possible steps along with the endless number of permutations and combinations. The generative AI is going to be expected to do the right thing when having to go beyond the stipulated steps.
The generative AI might opt to do something that we would be chagrined or concerned about upon going beyond the stated steps. Keep in mind that the AI is not sentient. It works based on mathematical and computational pattern-matching. Do not expect a kind of human commonsense to be at play, see my analysis at the link here.
Another issue is that everyday words and natural language are said to be semantically ambiguous (see my detailed discussion at the link here). When I told the AI to resolve the customer issue (as part of Step #3 in Customer Support Agent), what does that exactly mean? Resolving something can be a vague concept. The AI could go in many different directions. Some of those directions might be desirable and we would be pleased, while other directions might frustrate a customer and cause poor customer service.
You must also anticipate that the AI could momentarily go off the rails. There are so-called AI hallucinations that generative AI can encounter, see my coverage at the link here. I don’t like the catchphrase because it implies that AI hallucinates in a manner akin to human hallucinations, which is a false anthropomorphizing of AI. In any case, the AI can make up something out of thin air that appears to be sensible but is not factually grounded. Imagine if the AI tells a customer that they can get a refund if they stand on one leg and whoop and holler. Not a good look.
These and other sobering considerations need to be cooked into how you devise the AI agents and how you opt to ensure they operate in a safe and sane manner.
Excerpts Of How OpenAI Explains Swarm
Congratulations, you are now up to speed on the overall gist of agentic AI. You are also encouraged to dig more deeply into Swarm, which is one framework or approach to AI agents. See my coverage at the link here for competing AI agentic frameworks and methods.
Since you are now steeped in some of the agentic AI vocabularies, I have a bit of an informative test or quiz for you. Take a look at these excerpts from the OpenAI blog. I am hoping that you are familiar enough with the above discussion that you can readily discern what the excerpts have to say.
I’m selecting these excerpts from “Orchestrating Agents: Routines and Handoffs” by Ilan Bigio, OpenAI blog, October 9, 2024:
- “The notion of a ‘routine’ is not strictly defined, and instead meant to capture the idea of a set of steps. Concretely, let’s define a routine to be a list of instructions in natural language (which we’ll represent with a system prompt), along with the tools necessary to complete them.”
- “Notice that these instructions contain conditionals much like a state machine or branching in code. LLMs can actually handle these cases quite robustly for small and medium-sized routines, with the added benefit of having ‘soft’ adherence – the LLM can naturally steer the conversation without getting stuck in dead-ends.”
- “Dynamically swapping system instructions and tools may seem daunting. However, if we view ‘routines’ as ‘agents’, then this notion of handoffs allows us to represent these swaps simply – as one agent handing off a conversation to another.”
- “Let’s define a handoff as an agent (or routine) handing off an active conversation to another agent, much like when you get transferred to someone else on a phone call. Except in this case, the agents have complete knowledge of your prior conversation!”
- “As a proof of concept, we’ve packaged these ideas into a sample library called Swarm. It is meant as an example only and should not be directly used in production. However, feel free to take the ideas and code to build your own!”
How did you do?
I had my fingers crossed that the excerpts made abundant sense to you.
Getting Used To Agentic AI
A few final thoughts for now about the rising tide of agentic AI.
Conventional generative AI that you might be using day-to-day tends to do things one step at a time. Agentic AI boosts this by providing potential end-to-end processing for tasks that you might want to have performed on your behalf. Much of the time, agentic AI leans into the capabilities of generative AI.
Lots of AI agents can potentially get big things done.
I am reminded of the famous quote by Isoroku Yamamoto: “The fiercest serpent may be overcome by a swarm of ants.”
Though the bandwagon is definitely toward agentic AI, we need to keep our wits about us and realize that there are strengths and weaknesses involved. Suppose an agentic AI goes wild and like a swarm of bees’ attacks anything within range. Not a good look. All manners of AI ethics and AI law ramifications are going to arise.
You might brazenly assert that a swarm of them will soon emerge.
You may like
Noticias
Apocalipsis Biosciencias para desarrollar Géminis para la infección en pacientes con quemaduras graves
Published
2 minutos agoon
30 abril, 2025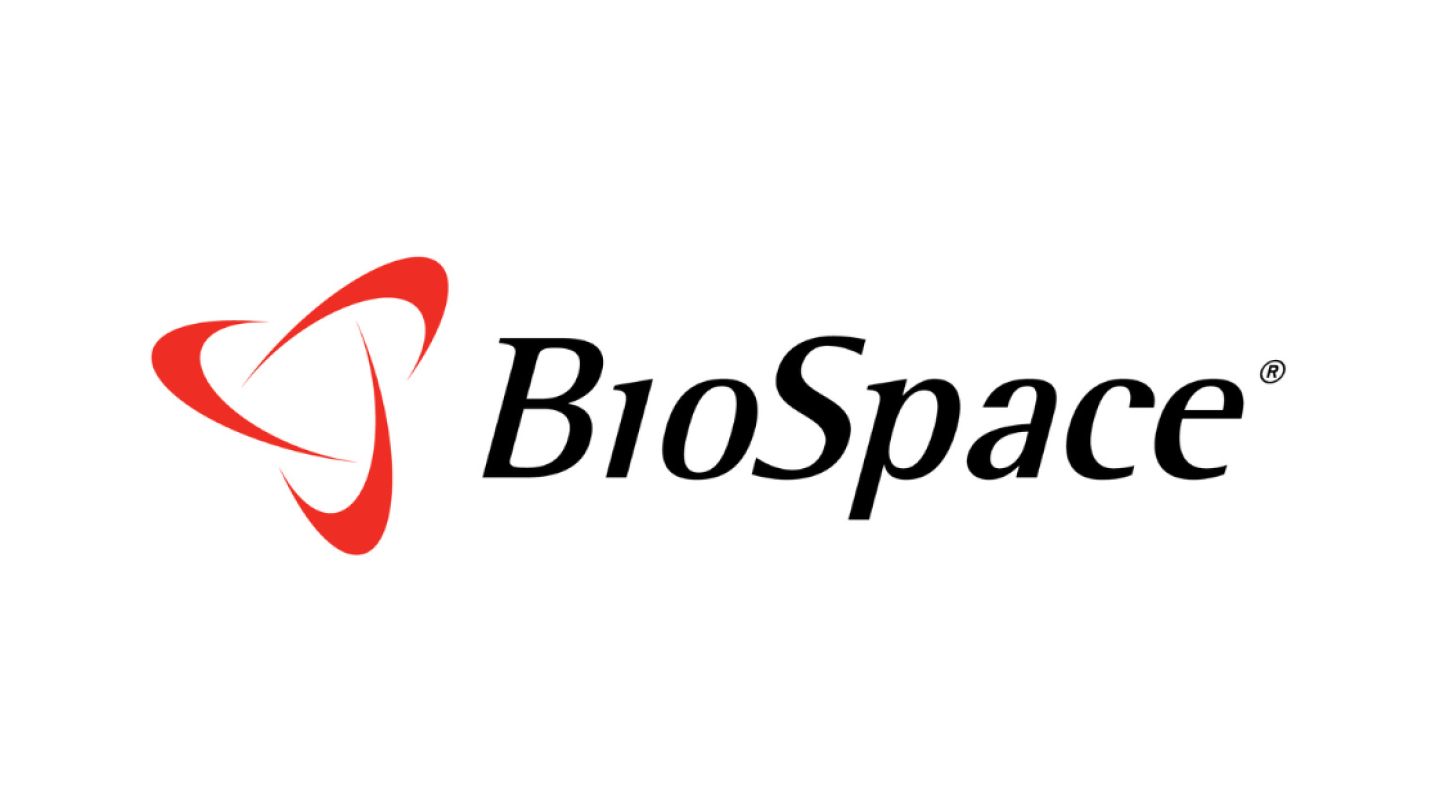
– Esta nueva indicación es otro paso para desbloquear todo el potencial de la plataforma Gemini –
San Diego-(Business Wire)-$ Revb #GÉMINIS–Apocalipsis Biosciences, Inc. (NASDAQ: RevB) (la “empresa” o “revelación”), una compañía de ciencias de la vida de etapas clínicas que se centra en reequilibrar la inflamación para optimizar la salud, anunció una nueva indicación de objetivo para Géminis para la prevención de la infección en pacientes con quemaduras graves que requieren hospitalización (el Gema-PBI programa). El uso de Géminis para la prevención de la infección en pacientes con quemaduras severas, así como la prevención de la infección después de la cirugía (el Gema-PSI programa) son parte de la revelación familiar de patentes anteriormente con licencia de la Universidad de Vanderbilt.
“Estamos muy contentos de colaborar con el equipo de Apocalipsis para el avance de Géminis para la prevención de la infección en esta población de pacientes desatendida”, dijo Dra. Julia BohannonProfesor Asociado, Departamento de Anestesiología, Departamento de Patología, Microbiología e Inmunología, Universidad de Vanderbilt. “Creemos que la actividad de biomarcador clínico observada con Gemini se correlaciona fuertemente con nuestra experiencia preclínica en modelos de quemaduras de infecciones”.
El equipo de investigación de Vanderbilt demostrado El tratamiento posterior a la quemadura reduce significativamente la gravedad y la duración de la infección pulmonar de Pseudomonas, así como un nivel general reducido de inflamación en un modelo preclínico.
“La prevención de la infección en pacientes severamente quemados es un esfuerzo importante y complementa que la revelación laboral ha completado hasta la fecha”, dijo “, dijo”, dijo James RolkeCEO de Revelation “El programa Gemini-PBI puede ofrecer varias oportunidades regulatorias, de desarrollo y financiación que la compañía planea explorar”.
Sobre quemaduras e infección después de quemar
Las quemaduras son lesiones en la piel que involucran las dos capas principales: la epidermis externa delgada y/o la dermis más gruesa y profunda. Las quemaduras pueden ser el resultado de una variedad de causas que incluyen fuego, líquidos calientes, productos químicos (como ácidos fuertes o bases fuertes), electricidad, vapor, radiación de radiografías o radioterapia, luz solar o luz ultravioleta. Cada año, aproximadamente medio millón de estadounidenses sufren lesiones por quemaduras que requieren intervención médica. Si bien la mayoría de las lesiones por quemaduras no requieren ingreso a un hospital, se admiten alrededor de 40,000 pacientes, y aproximadamente 30,000 de ellos necesitan tratamiento especializado en un centro de quemaduras certificadas.
El número total anual de muertes relacionadas con quemaduras es de aproximadamente 3.400, siendo la infección invasiva la razón principal de la muerte después de las primeras 24 horas. La tasa de mortalidad general para pacientes con quemaduras graves es de aproximadamente 3.3%, pero esto aumenta al 20.6% en pacientes con quemaduras con lesión cutánea de quemaduras y inhalación, versus 10.5% por lesión por inhalación solo. La infección invasiva, incluida la sepsis, es la causa principal de la muerte después de la lesión por quemaduras, lo que representa aproximadamente el 51% de las muertes.
Actualmente no hay tratamientos aprobados para prevenir la infección sistémica en pacientes con quemaduras.
Sobre Géminis
Géminis es una formulación propietaria y propietaria de disacárido hexaacil fosforilada (PHAD (PHAD®) que reduce el daño asociado con la inflamación al reprogramarse del sistema inmune innato para responder al estrés (trauma, infección, etc.) de manera atenuada. La revelación ha realizado múltiples estudios preclínicos que demuestran el potencial terapéutico de Géminis en las indicaciones objetivo. Revelación anunciado previamente Datos clínicos positivos de fase 1 para el tratamiento intravenoso con Géminis. El punto final de seguridad primario se cumplió en el estudio de fase 1, y los resultados demostraron la actividad farmacodinámica estadísticamente significativa como se observó a través de los cambios esperados en múltiples biomarcadores, incluida la regulación positiva de IL-10.
Géminis se está desarrollando para múltiples indicaciones, incluso como pretratamiento para prevenir o reducir la gravedad y la duración de la lesión renal aguda (programa Gemini-AKI), y como pretratamiento para prevenir o reducir la gravedad y la duración de la infección posquirúrgica (programa GEMINI-PSI). Además, Gemini puede ser un tratamiento para detener o retrasar la progresión de la enfermedad renal crónica (programa Gemini-CKD).
Acerca de Apocalipsis Biosciences, Inc.
Revelation Biosciences, Inc. es una compañía de ciencias de la vida en estadio clínico centrada en aprovechar el poder de la inmunidad entrenada para la prevención y el tratamiento de la enfermedad utilizando su formulación patentada Géminis. Revelation tiene múltiples programas en curso para evaluar Géminis, incluso como prevención de la infección posquirúrgica, como prevención de lesiones renales agudas y para el tratamiento de la enfermedad renal crónica.
Para obtener más información sobre Apocalipsis, visite www.revbiosciences.com.
Declaraciones con avance
Este comunicado de prensa contiene declaraciones prospectivas definidas en la Ley de Reforma de Litigios de Valores Privados de 1995, según enmendada. Las declaraciones prospectivas son declaraciones que no son hechos históricos. Estas declaraciones prospectivas generalmente se identifican por las palabras “anticipar”, “creer”, “esperar”, “estimar”, “plan”, “perspectiva” y “proyecto” y otras expresiones similares. Advirtemos a los inversores que las declaraciones prospectivas se basan en las expectativas de la gerencia y son solo predicciones o declaraciones de las expectativas actuales e involucran riesgos, incertidumbres y otros factores conocidos y desconocidos que pueden hacer que los resultados reales sean materialmente diferentes de los previstos por las declaraciones de prospección. Apocalipsis advierte a los lectores que no depositen una dependencia indebida de tales declaraciones de vista hacia adelante, que solo hablan a partir de la fecha en que se hicieron. Los siguientes factores, entre otros, podrían hacer que los resultados reales difieran materialmente de los descritos en estas declaraciones prospectivas: la capacidad de la revelación para cumplir con sus objetivos financieros y estratégicos, debido a, entre otras cosas, la competencia; la capacidad de la revelación para crecer y gestionar la rentabilidad del crecimiento y retener a sus empleados clave; la posibilidad de que la revelación pueda verse afectada negativamente por otros factores económicos, comerciales y/o competitivos; riesgos relacionados con el desarrollo exitoso de los candidatos de productos de Apocalipsis; la capacidad de completar con éxito los estudios clínicos planificados de sus candidatos de productos; El riesgo de que no podamos inscribir completamente nuestros estudios clínicos o la inscripción llevará más tiempo de lo esperado; riesgos relacionados con la aparición de eventos de seguridad adversos y/o preocupaciones inesperadas que pueden surgir de los datos o análisis de nuestros estudios clínicos; cambios en las leyes o regulaciones aplicables; Iniciación esperada de los estudios clínicos, el momento de los datos clínicos; El resultado de los datos clínicos, incluido si los resultados de dicho estudio son positivos o si se puede replicar; El resultado de los datos recopilados, incluido si los resultados de dichos datos y/o correlación se pueden replicar; el momento, los costos, la conducta y el resultado de nuestros otros estudios clínicos; El tratamiento anticipado de datos clínicos futuros por parte de la FDA, la EMA u otras autoridades reguladoras, incluidos si dichos datos serán suficientes para su aprobación; el éxito de futuras actividades de desarrollo para sus candidatos de productos; posibles indicaciones para las cuales se pueden desarrollar candidatos de productos; la capacidad de revelación para mantener la lista de sus valores en NASDAQ; la duración esperada sobre la cual los saldos de Apocalipsis financiarán sus operaciones; y otros riesgos e incertidumbres descritos en este documento, así como aquellos riesgos e incertidumbres discutidos de vez en cuando en otros informes y otras presentaciones públicas con la SEC por Apocalipsis.
Contactos
Mike Porter
Relaciones con inversores
Porter Levay & Rose Inc.
Correo electrónico: mike@plrinvest.com
Chester Zygmont, III
Director financiero
Apocalipsis Biosciences Inc.
Correo electrónico: czygmont@revbiosciences.com
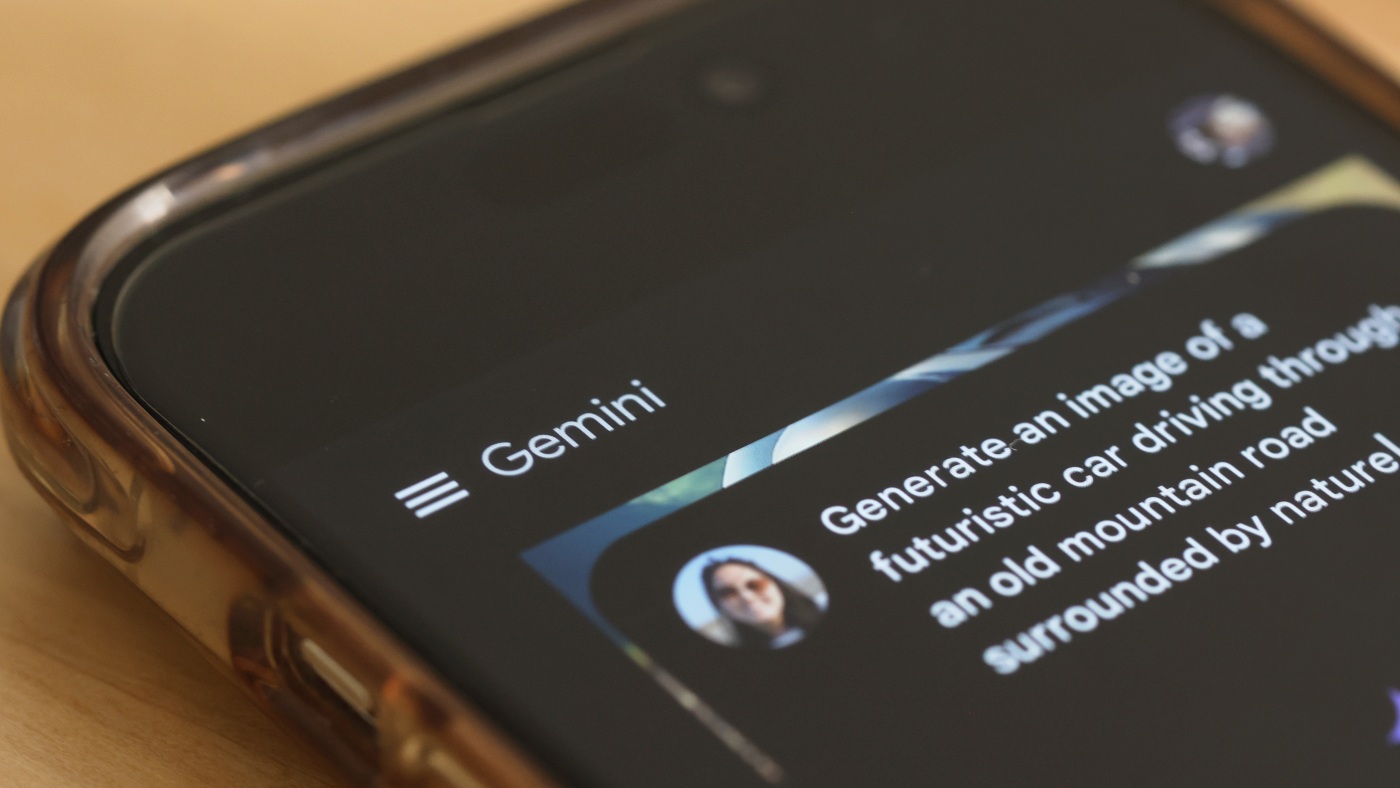
An illustration photograph taken on Feb. 20, 2025 shows Grok, DeepSeek and ChatGPT apps displayed on a phone screen. The Justice Department’s 2020 complaint against Google has few mentions of artificial intelligence or AI chatbots. But nearly five years later, as the remedy phase of the trial enters its second week of testimony, the focus has shifted to AI.
Michael M. Santiago/Getty Images/Getty Images North America
hide caption
toggle caption
Michael M. Santiago/Getty Images/Getty Images North America
When the U.S. Department of Justice originally brought — and then won — its case against Google, arguing that the tech behemoth monopolized the search engine market, the focus was on, well … search.
Back then, in 2020, the government’s antitrust complaint against Google had few mentions of artificial intelligence or AI chatbots. But nearly five years later, as the remedy phase of the trial enters its second week of testimony, the focus has shifted to AI, underscoring just how quickly this emerging technology has expanded.
In the past few days, before a federal judge who will assess penalties against Google, the DOJ has argued that the company could use its artificial intelligence products to strengthen its monopoly in online search — and to use the data from its powerful search index to become the dominant player in AI.
In his opening statements last Monday, David Dahlquist, the acting deputy director of the DOJ’s antitrust civil litigation division, argued that the court should consider remedies that could nip a potential Google AI monopoly in the bud. “This court’s remedy should be forward-looking and not ignore what is on the horizon,” he said.
Dahlquist argued that Google has created a system in which its control of search helps improve its AI products, sending more users back to Google search — creating a cycle that maintains the tech company’s dominance and blocks competitors out of both marketplaces.
The integration of search and Gemini, the company’s AI chatbot — which the DOJ sees as powerful fuel for this cycle — is a big focus of the government’s proposed remedies. The DOJ is arguing that to be most effective, those remedies must address all ways users access Google search, so any penalties approved by the court that don’t include Gemini (or other Google AI products now or in the future) would undermine their broader efforts.
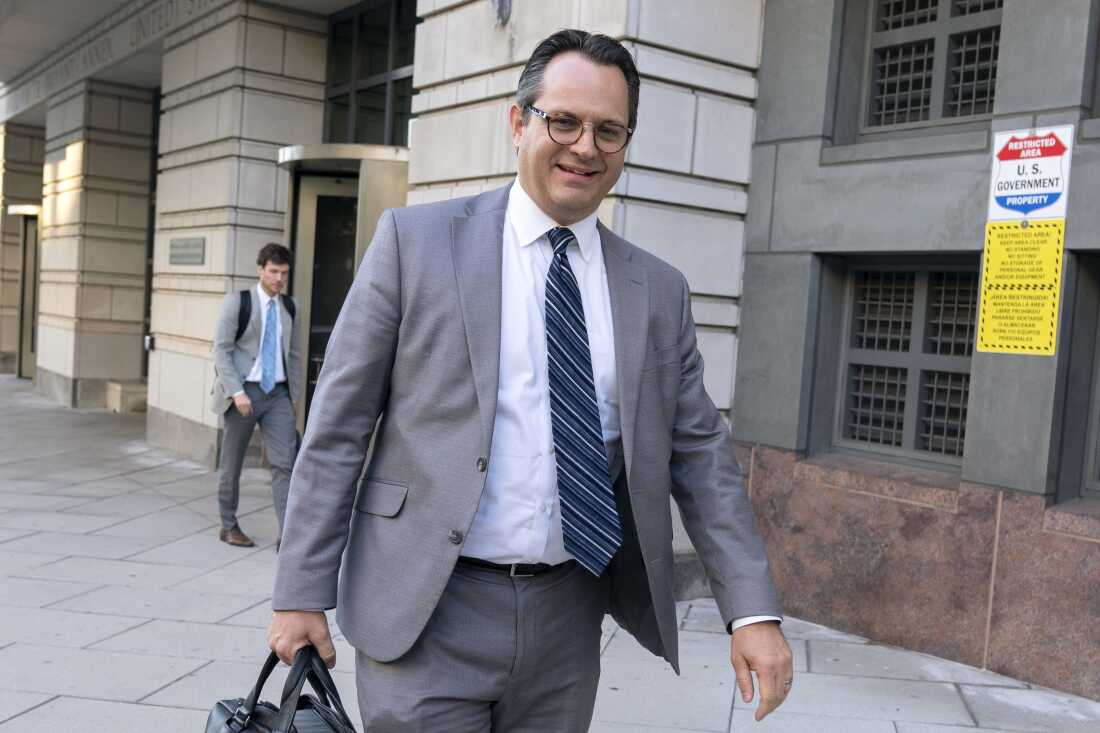
Department of Justice lawyer David Dahlquist leaves the Washington, D.C. federal courthouse on Sept. 20, 2023 during the original trial phase of the antitrust case against Google.
Jose Luis Magana/AP/FR159526 AP
hide caption
toggle caption
Jose Luis Magana/AP/FR159526 AP
AI and search are connected like this: Search engine indices are essentially giant databases of pages and information on the web. Google has its own such index, which contains hundreds of billions of webpages and is over 100,000,000 gigabytes, according to court documents. This is the data Google’s search engine scans when responding to a user’s query.
AI developers use these kinds of databases to build and train the models used to power chatbots. In court, attorneys for the DOJ have argued that Google’s Gemini pulls information from the company’s search index, including citing search links and results, extending what they say is a self-serving cycle. They argue that Google’s ability to monopolize the search market gives it user data, at a huge scale — an advantage over other AI developers.
The Justice Department argues Google’s monopoly over search could have a direct effect on the development of generative AI, a type of artificial intelligence that uses existing data to create new content like text, videos or photos, based on a user’s prompts or questions. Last week, the government called executives from several major AI companies, like OpenAI and Perplexity, in an attempt to argue that Google’s stranglehold on search is preventing some of those companies from truly growing.
The government argues that to level the playing field, Google should be forced to open its search data — like users’ search queries, clicks and results — and license it to other competitors at a cost.
This is on top of demands related to Google’s search engine business, most notably that it should be forced to sell off its Chrome browser.
Google flatly rejects the argument that it could monopolize the field of generative AI, saying competition in the AI race is healthy. In a recent blog post on Google’s website, Lee-Anne Mulholland, the company’s vice president of regulatory affairs, wrote that since the federal judge first ruled against Google over a year ago, “AI has already rapidly reshaped the industry, with new entrants and new ways of finding information, making it even more competitive.”
In court, Google’s lawyers have argued that there are a host of AI companies with chatbots — some of which are outperforming Gemini. OpenAI has ChatGPT, Meta has MetaAI and Perplexity has Perplexity AI.
“There is no shortage of competition in that market, and ChatGPT and Meta are way ahead of everybody in terms of the distribution and usage at this point,” said John E. Schmidtlein, a lawyer for Google, during his opening statement. “But don’t take my word for it. Look at the data. Hundreds and hundreds of millions of downloads by ChatGPT.”
Competing in a growing AI field
It should be no surprise that AI is coming up so much at this point in the trial, said Alissa Cooper, the executive director of the Knight-Georgetown Institute, a nonpartisan tech research and policy center at Georgetown University focusing on AI, disinformation and data privacy.
“If you look at search as a product today, you can’t really think about search without thinking about AI,” she said. “I think the case is a really great opportunity to try to … analyze how Google has benefited specifically from the monopoly that it has in search, and ensure that the behavior that led to that can’t be used to gain an unfair advantage in these other markets which are more nascent.”
Having access to Google’s data, she said, “would provide them with the ability to build better chatbots, build better search engines, and potentially build other products that we haven’t even thought of.”
To make that point, the DOJ called Nick Turley, OpenAI’s head of product for ChatGPT, to the stand last Tuesday. During a long day of testimony, Turley detailed how without access to Google’s search index and data, engineers for the growing company tried to build their own.
ChatGPT, a large language model that can generate human-like responses, engage in conversations and perform tasks like explaining a tough-to-understand math lesson, was never intended to be a product for OpenAI, Turley said. But once it launched and went viral, the company found that people were using it for a host of needs.
Though popular, ChatGPT had its drawbacks, like the bot’s limited “knowledge,” Turley said. Early on, ChatGPT was not connected to the internet and could only use information that it had been fed up to a certain point in its training. For example, Turley said, if a user asked “Who is the president?” the program would give a 2022 answer — from when its “knowledge” effectively stopped.
OpenAI couldn’t build their own index fast enough to address their problems; they found that process incredibly expensive, time consuming and potentially years from coming to fruition, Turley said.
So instead, they sought a partnership with a third party search provider. At one point, OpenAI tried to make a deal with Google to gain access to their search, but Google declined, seeing OpenAI as a direct competitor, Turley testified.
But Google says companies like OpenAI are doing just fine without gaining access to the tech giant’s own technology — which it spent decades developing. These companies just want “handouts,” said Schmidtlein.
On the third day of the remedy trial, internal Google documents shared in court by the company’s lawyers compared how many people are using Gemini versus its competitors. According to those documents, ChatGPT and MetaAI are the two leaders, with Gemini coming in third.
They showed that this March, Gemini saw 35 million active daily users and 350 million monthly active users worldwide. That was up from 9 million daily active users in October 2024. But according to those documents, Gemini was still lagging behind ChatGPT, which reached 160 million daily users and around 600 million active users in March.
These numbers show that competitors have no need to use Google’s search data, valuable intellectual property that the tech giant spent decades building and maintaining, the company argues.
“The notion that somehow ChatGPT can’t get distribution is absurd,” Schmidtlein said in court last week. “They have more distribution than anyone.”
Google’s exclusive deals
In his ruling last year, U.S. District Judge Amit Mehta said Google’s exclusive agreements with device makers, like Apple and Samsung, to make its search engine the default on those companies’ phones helped maintain its monopoly. It remains a core issue for this remedy trial.
Now, the DOJ is arguing that Google’s deals with device manufacturers are also directly affecting AI companies and AI tech.
In court, the DOJ argued that Google has replicated this kind of distribution deal by agreeing to pay Samsung what Dahlquist called a monthly “enormous sum” for Gemini to be installed on smartphones and other devices.
Last Wednesday, the DOJ also called Dmitry Shevelenko, Perplexity’s chief business officer, to testify that Google has effectively cut his company out from making deals with manufacturers and mobile carriers.
Perplexity AIs not preloaded on any mobile devices in the U.S., despite many efforts to get phone companies to establish Perplexity as a default or exclusive app on devices, Shevelenko said. He compared Google’s control in that space to that of a “mob boss.”
But Google’s attorney, Christopher Yeager, noted in questioning Shevelenko that Perplexity has reached a valuation of over $9 billion — insinuating the company is doing just fine in the marketplace.
Despite testifying in court (for which he was subpoenaed, Shevelenko noted), he and other leaders at Perplexity are against the breakup of Google. In a statement on the company’s website, the Perplexity team wrote that neither forcing Google to sell off Chrome nor to license search data to its competitors are the best solutions. “Neither of these address the root issue: consumers deserve choice,” they wrote.
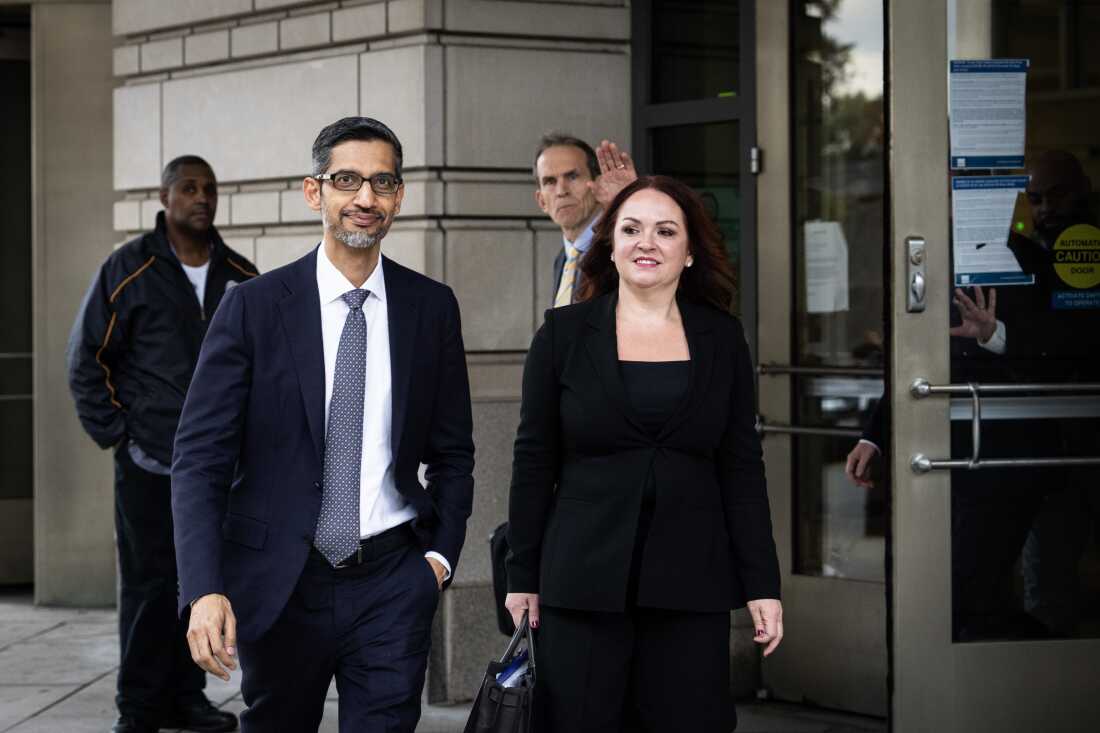
Google and Alphabet CEO Sundar Pichai departs federal court after testifying in October 2023 in Washington, DC. Pichai testified to defend his company in the original antitrust trial. Pichai is expected to testify again during the remedy phase of the legal proceedings.
Drew Angerer/Getty Images/Getty Images North America
hide caption
toggle caption
Drew Angerer/Getty Images/Getty Images North America
What to expect next
This week the trial continues, with the DOJ calling its final witnesses this morning to testify about the feasibility of a Chrome divestiture and how the government’s proposed remedies would help rivals compete. On Tuesday afternoon, Google will begin presenting its case, which is expected to feature the testimony of CEO Sundar Pichai, although the date of his appearance has not been specified.
Closing arguments are expected at the end of May, and then Mehta will make his ruling. Google says once this phase is settled the company will appeal Mehta’s ruling in the underlying case.
Whatever Mehta decides in this remedy phase, Cooper thinks it will have effects beyond just the business of search engines. No matter what it is, she said, “it will be having some kind of impact on AI.”
Google is a financial supporter of NPR.
Noticias
API de Meta Oleleshes Llama que se ejecuta 18 veces más rápido que OpenAI: Cerebras Partnership ofrece 2.600 tokens por segundo
Published
9 horas agoon
29 abril, 2025
Únase a nuestros boletines diarios y semanales para obtener las últimas actualizaciones y contenido exclusivo sobre la cobertura de IA líder de la industria. Obtenga más información
Meta anunció hoy una asociación con Cerebras Systems para alimentar su nueva API de LLAMA, ofreciendo a los desarrolladores acceso a velocidades de inferencia hasta 18 veces más rápido que las soluciones tradicionales basadas en GPU.
El anuncio, realizado en la Conferencia inaugural de desarrolladores de Llamacon de Meta en Menlo Park, posiciona a la compañía para competir directamente con Operai, Anthrope y Google en el mercado de servicios de inferencia de IA en rápido crecimiento, donde los desarrolladores compran tokens por miles de millones para impulsar sus aplicaciones.
“Meta ha seleccionado a Cerebras para colaborar para ofrecer la inferencia ultra rápida que necesitan para servir a los desarrolladores a través de su nueva API de LLAMA”, dijo Julie Shin Choi, directora de marketing de Cerebras, durante una sesión de prensa. “En Cerebras estamos muy, muy emocionados de anunciar nuestra primera asociación HyperScaler CSP para ofrecer una inferencia ultra rápida a todos los desarrolladores”.
La asociación marca la entrada formal de Meta en el negocio de la venta de AI Computation, transformando sus populares modelos de llama de código abierto en un servicio comercial. Si bien los modelos de LLAMA de Meta se han acumulado en mil millones de descargas, hasta ahora la compañía no había ofrecido una infraestructura en la nube de primera parte para que los desarrolladores creen aplicaciones con ellos.
“Esto es muy emocionante, incluso sin hablar sobre cerebras específicamente”, dijo James Wang, un ejecutivo senior de Cerebras. “Openai, Anthrope, Google: han construido un nuevo negocio de IA completamente nuevo desde cero, que es el negocio de inferencia de IA. Los desarrolladores que están construyendo aplicaciones de IA comprarán tokens por millones, a veces por miles de millones. Y estas son como las nuevas instrucciones de cómputo que las personas necesitan para construir aplicaciones AI”.
Breaking the Speed Barrier: Cómo modelos de Llama de Cerebras Supercharges
Lo que distingue a la oferta de Meta es el aumento de la velocidad dramática proporcionado por los chips de IA especializados de Cerebras. El sistema de cerebras ofrece más de 2.600 fichas por segundo para Llama 4 Scout, en comparación con aproximadamente 130 tokens por segundo para ChatGPT y alrededor de 25 tokens por segundo para Deepseek, según puntos de referencia del análisis artificial.
“Si solo se compara con API a API, Gemini y GPT, todos son grandes modelos, pero todos se ejecutan a velocidades de GPU, que son aproximadamente 100 tokens por segundo”, explicó Wang. “Y 100 tokens por segundo están bien para el chat, pero es muy lento para el razonamiento. Es muy lento para los agentes. Y la gente está luchando con eso hoy”.
Esta ventaja de velocidad permite categorías completamente nuevas de aplicaciones que antes no eran prácticas, incluidos los agentes en tiempo real, los sistemas de voz de baja latencia conversacional, la generación de código interactivo y el razonamiento instantáneo de múltiples pasos, todos los cuales requieren encadenamiento de múltiples llamadas de modelo de lenguaje grandes que ahora se pueden completar en segundos en lugar de minutos.
La API de LLAMA representa un cambio significativo en la estrategia de IA de Meta, en la transición de ser un proveedor de modelos a convertirse en una compañía de infraestructura de IA de servicio completo. Al ofrecer un servicio API, Meta está creando un flujo de ingresos a partir de sus inversiones de IA mientras mantiene su compromiso de abrir modelos.
“Meta ahora está en el negocio de vender tokens, y es excelente para el tipo de ecosistema de IA estadounidense”, señaló Wang durante la conferencia de prensa. “Traen mucho a la mesa”.
La API ofrecerá herramientas para el ajuste y la evaluación, comenzando con el modelo LLAMA 3.3 8B, permitiendo a los desarrolladores generar datos, entrenar y probar la calidad de sus modelos personalizados. Meta enfatiza que no utilizará datos de clientes para capacitar a sus propios modelos, y los modelos construidos con la API de LLAMA se pueden transferir a otros hosts, una clara diferenciación de los enfoques más cerrados de algunos competidores.
Las cerebras alimentarán el nuevo servicio de Meta a través de su red de centros de datos ubicados en toda América del Norte, incluidas las instalaciones en Dallas, Oklahoma, Minnesota, Montreal y California.
“Todos nuestros centros de datos que sirven a la inferencia están en América del Norte en este momento”, explicó Choi. “Serviremos Meta con toda la capacidad de las cerebras. La carga de trabajo se equilibrará en todos estos diferentes centros de datos”.
El arreglo comercial sigue lo que Choi describió como “el proveedor de cómputo clásico para un modelo hiperscalador”, similar a la forma en que NVIDIA proporciona hardware a los principales proveedores de la nube. “Están reservando bloques de nuestro cómputo para que puedan servir a su población de desarrolladores”, dijo.
Más allá de las cerebras, Meta también ha anunciado una asociación con Groq para proporcionar opciones de inferencia rápida, brindando a los desarrolladores múltiples alternativas de alto rendimiento más allá de la inferencia tradicional basada en GPU.
La entrada de Meta en el mercado de API de inferencia con métricas de rendimiento superiores podría potencialmente alterar el orden establecido dominado por Operai, Google y Anthrope. Al combinar la popularidad de sus modelos de código abierto con capacidades de inferencia dramáticamente más rápidas, Meta se está posicionando como un competidor formidable en el espacio comercial de IA.
“Meta está en una posición única con 3 mil millones de usuarios, centros de datos de hiper escala y un gran ecosistema de desarrolladores”, según los materiales de presentación de Cerebras. La integración de la tecnología de cerebras “ayuda a Meta Leapfrog OpenAi y Google en rendimiento en aproximadamente 20X”.
Para las cerebras, esta asociación representa un hito importante y la validación de su enfoque especializado de hardware de IA. “Hemos estado construyendo este motor a escala de obleas durante años, y siempre supimos que la primera tarifa de la tecnología, pero en última instancia tiene que terminar como parte de la nube de hiperescala de otra persona. Ese fue el objetivo final desde una perspectiva de estrategia comercial, y finalmente hemos alcanzado ese hito”, dijo Wang.
La API de LLAMA está actualmente disponible como una vista previa limitada, con Meta planifica un despliegue más amplio en las próximas semanas y meses. Los desarrolladores interesados en acceder a la inferencia Ultra-Fast Llama 4 pueden solicitar el acceso temprano seleccionando cerebras de las opciones del modelo dentro de la API de LLAMA.
“Si te imaginas a un desarrollador que no sabe nada sobre cerebras porque somos una empresa relativamente pequeña, solo pueden hacer clic en dos botones en el SDK estándar de SDK estándar de Meta, generar una tecla API, seleccionar la bandera de cerebras y luego, de repente, sus tokens se procesan en un motor gigante a escala de dafers”, explicó las cejas. “Ese tipo de hacernos estar en el back -end del ecosistema de desarrolladores de Meta todo el ecosistema es tremendo para nosotros”.
La elección de Meta de silicio especializada señala algo profundo: en la siguiente fase de la IA, no es solo lo que saben sus modelos, sino lo rápido que pueden pensarlo. En ese futuro, la velocidad no es solo una característica, es todo el punto.
Insights diarias sobre casos de uso comercial con VB diariamente
Si quieres impresionar a tu jefe, VB Daily te tiene cubierto. Le damos la cuenta interior de lo que las empresas están haciendo con la IA generativa, desde cambios regulatorios hasta implementaciones prácticas, por lo que puede compartir ideas para el ROI máximo.
Lea nuestra Política de privacidad
Gracias por suscribirse. Mira más boletines de VB aquí.
Ocurrió un error.
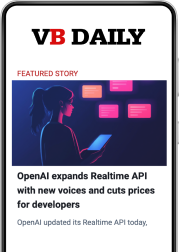
Related posts
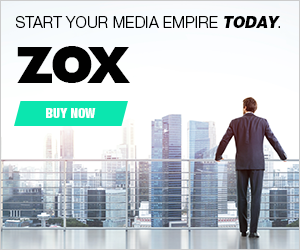
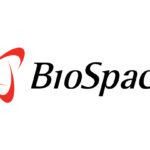
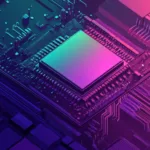
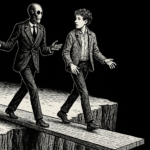
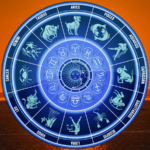
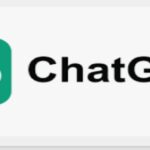
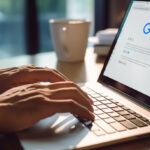
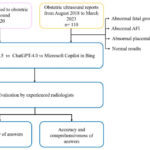
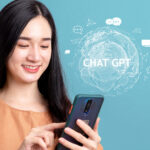
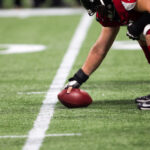
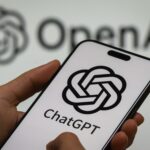
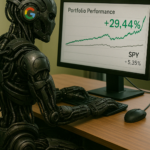
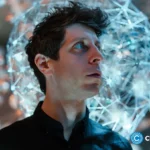
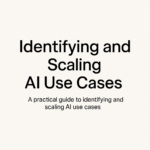
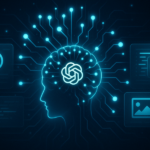
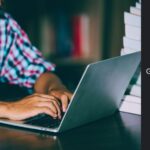
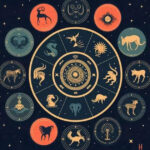
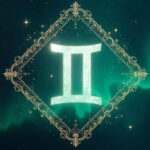
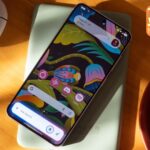
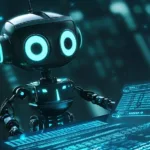
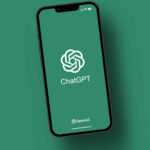
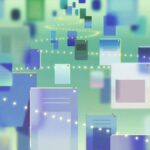
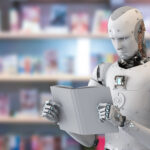
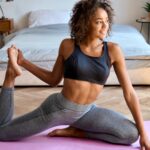
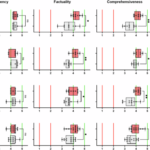
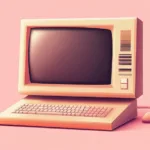
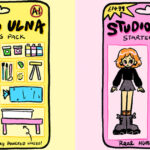
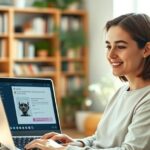
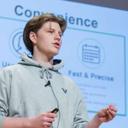
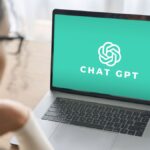
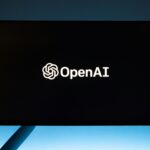
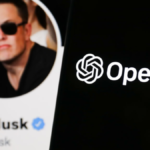
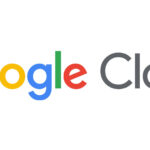
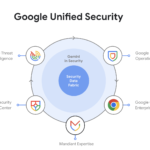
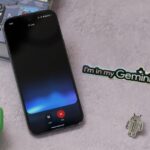
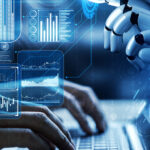
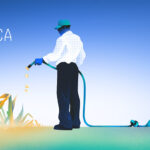
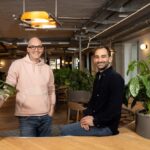
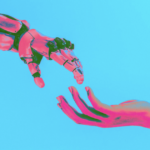
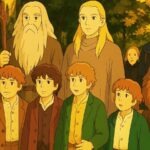
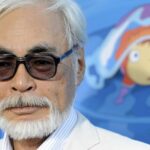
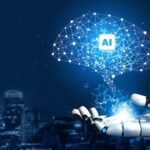
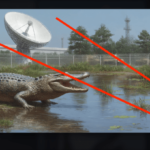
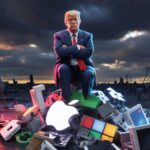
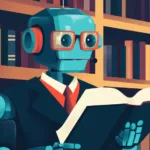
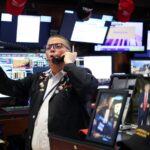
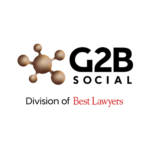
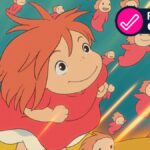
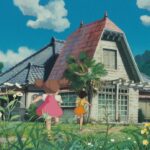
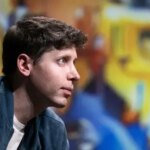
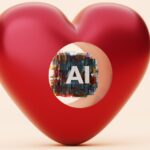
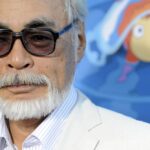
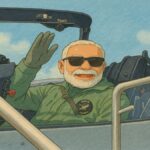
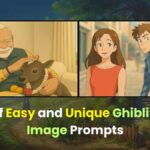
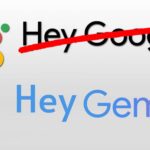
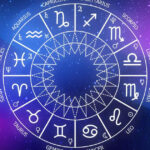
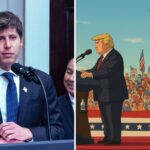
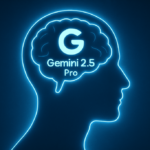
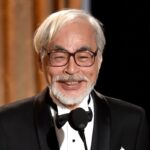
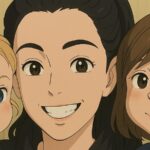
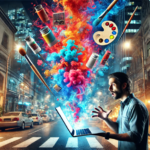
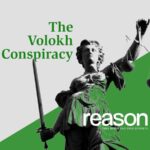
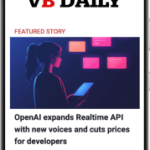
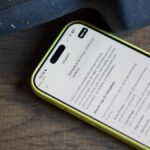
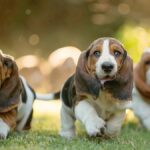
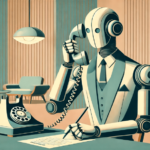
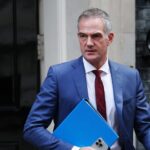
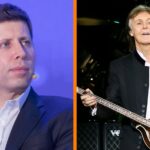
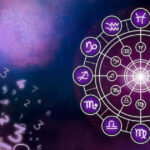
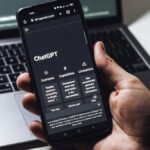
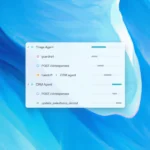
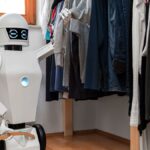
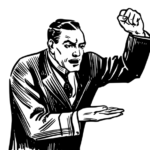
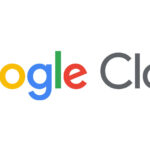
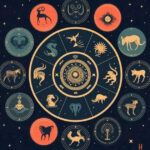
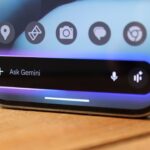
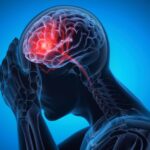
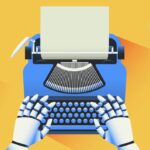
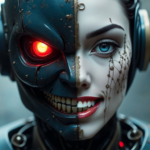
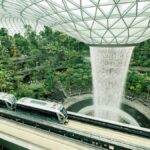
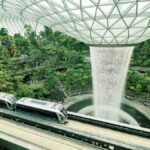
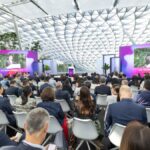
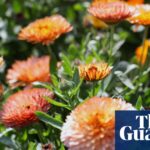

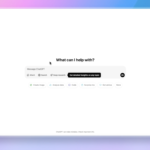
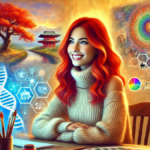
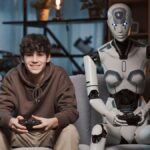
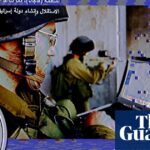
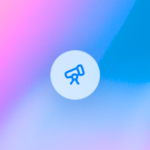
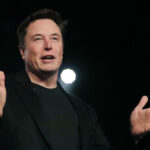
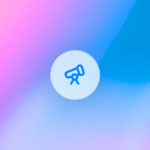
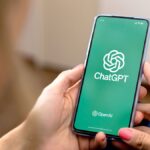
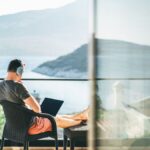
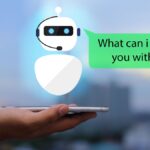
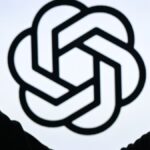
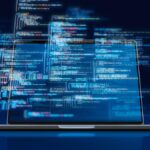
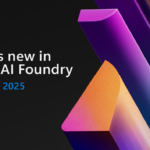
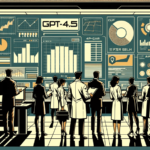
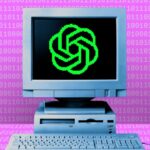
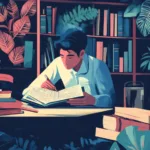
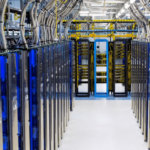
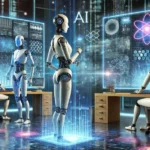
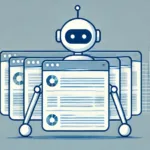
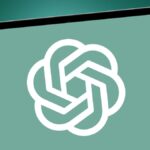
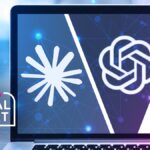
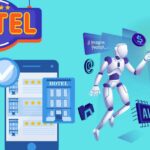
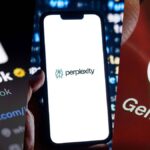
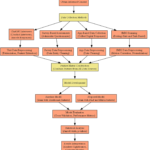
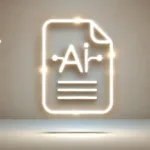
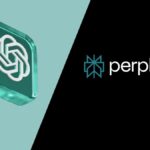
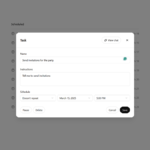
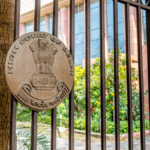
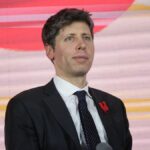
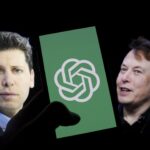
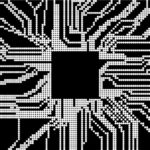
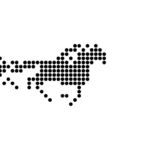
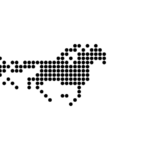
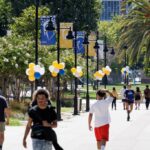
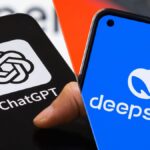
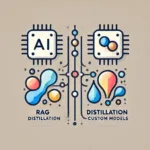
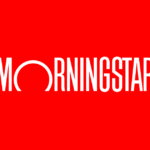
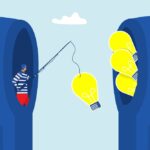
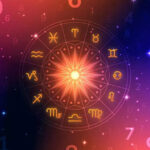
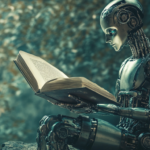
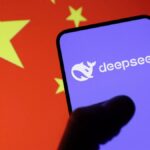
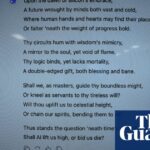
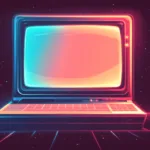

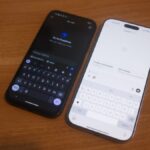
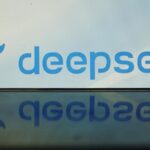

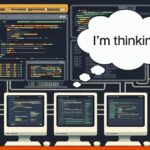
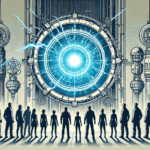
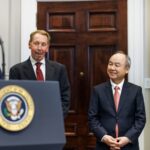
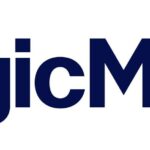
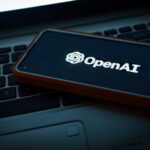

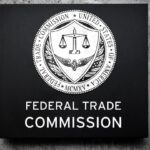
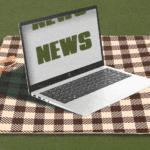
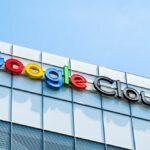
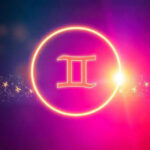
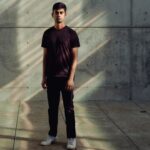
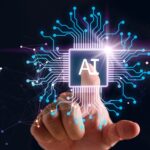
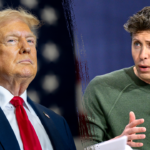
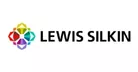
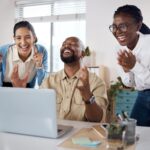
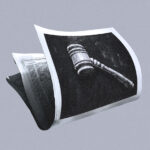
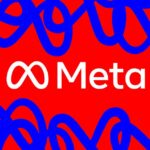

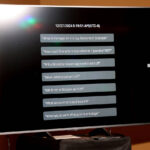

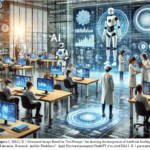
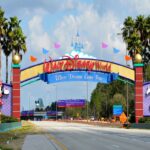
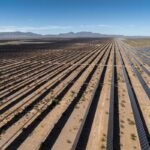
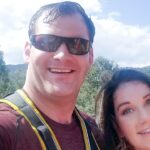
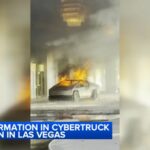
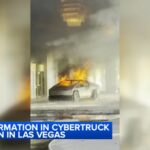
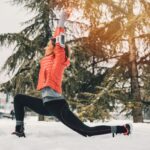
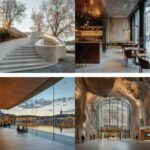
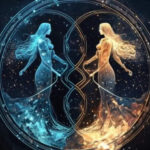
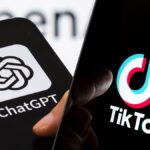
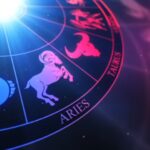
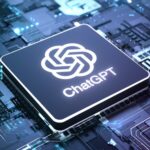
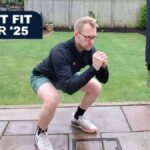
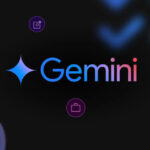
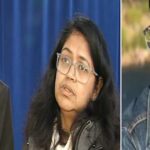
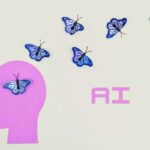
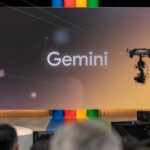
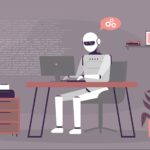
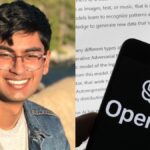
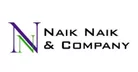

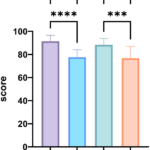
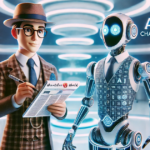
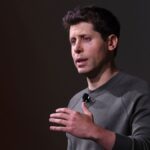
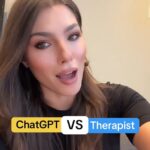
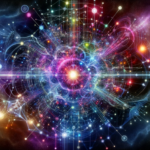
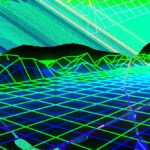
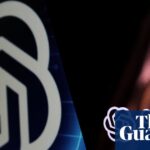
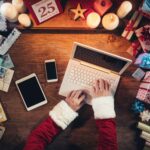
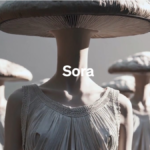
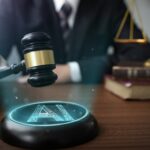
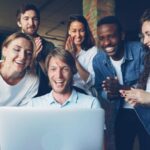
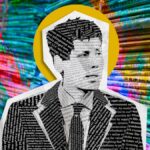

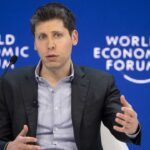
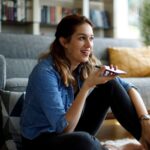
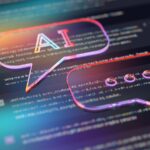
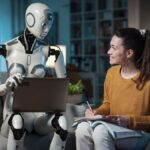
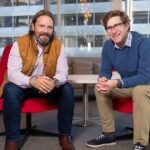
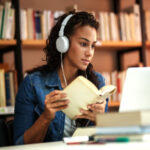
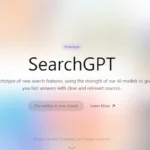
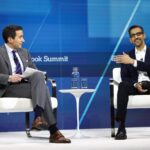
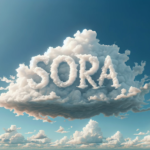
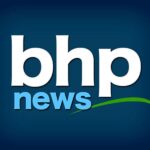
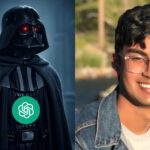
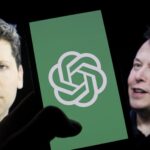
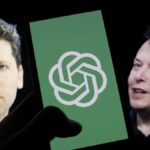
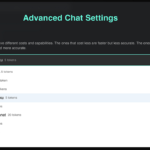
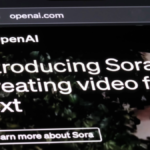
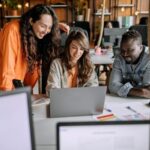
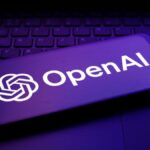
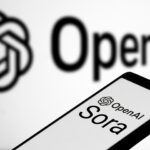
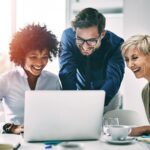
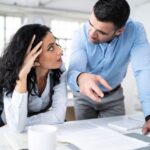
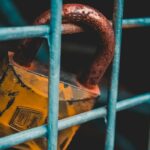
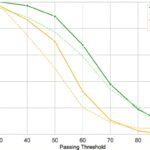
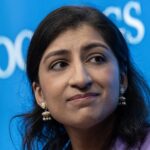
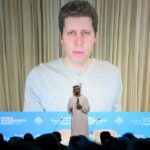
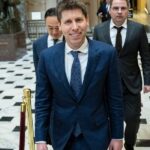
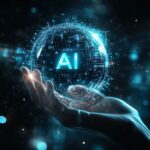
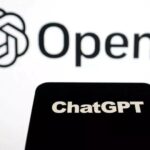
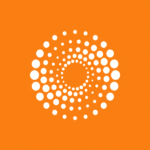
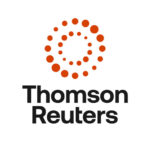
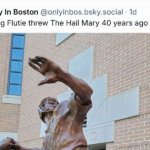

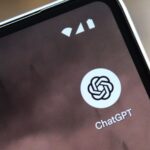
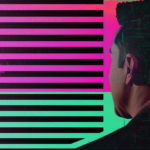
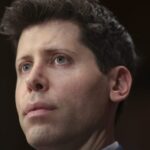
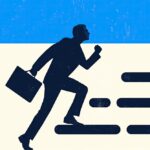
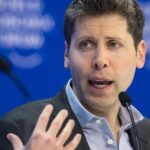
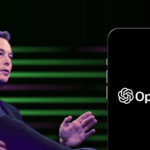
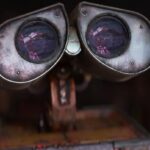
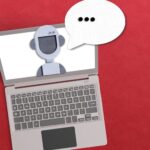
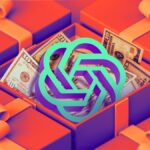
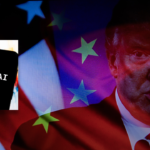
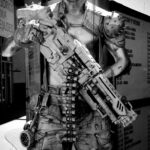
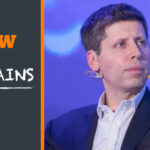
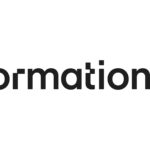
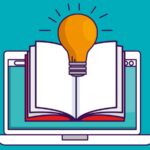
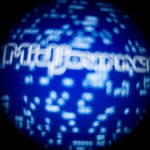
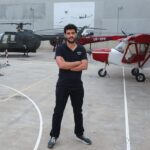
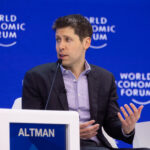
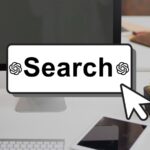
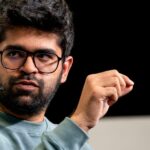
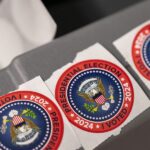
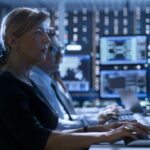
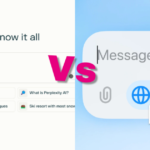
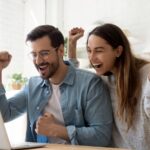
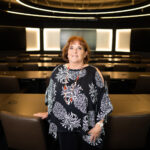
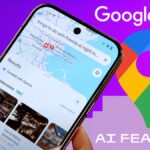
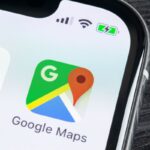
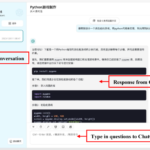
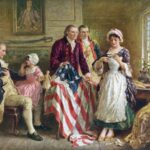
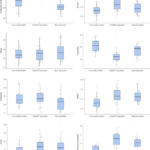

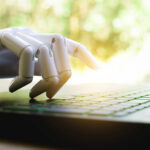
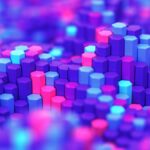
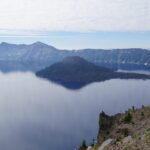
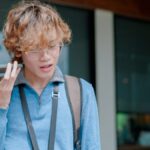
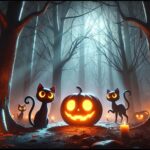
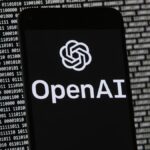
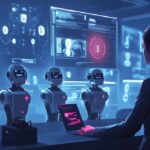
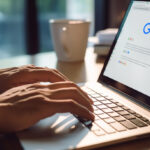
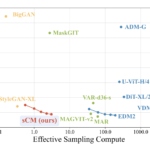
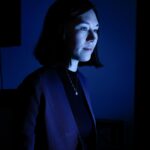
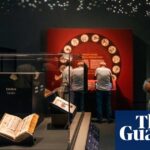
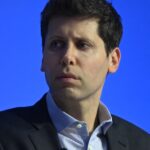
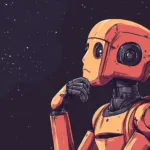
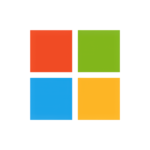
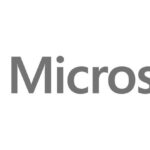
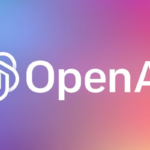
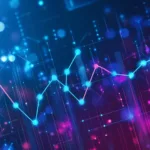
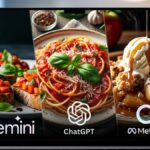
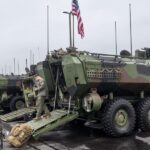

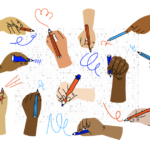
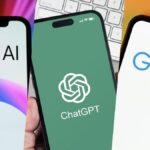
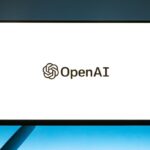
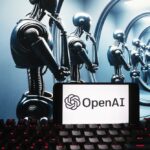
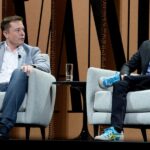
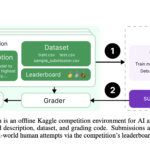
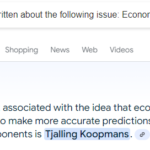
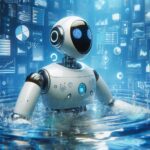
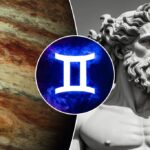
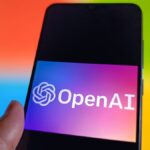
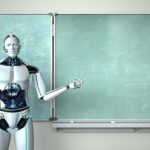
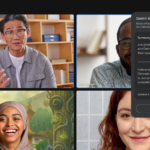
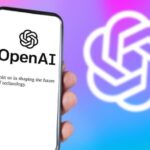
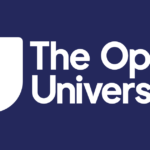
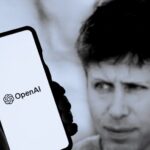
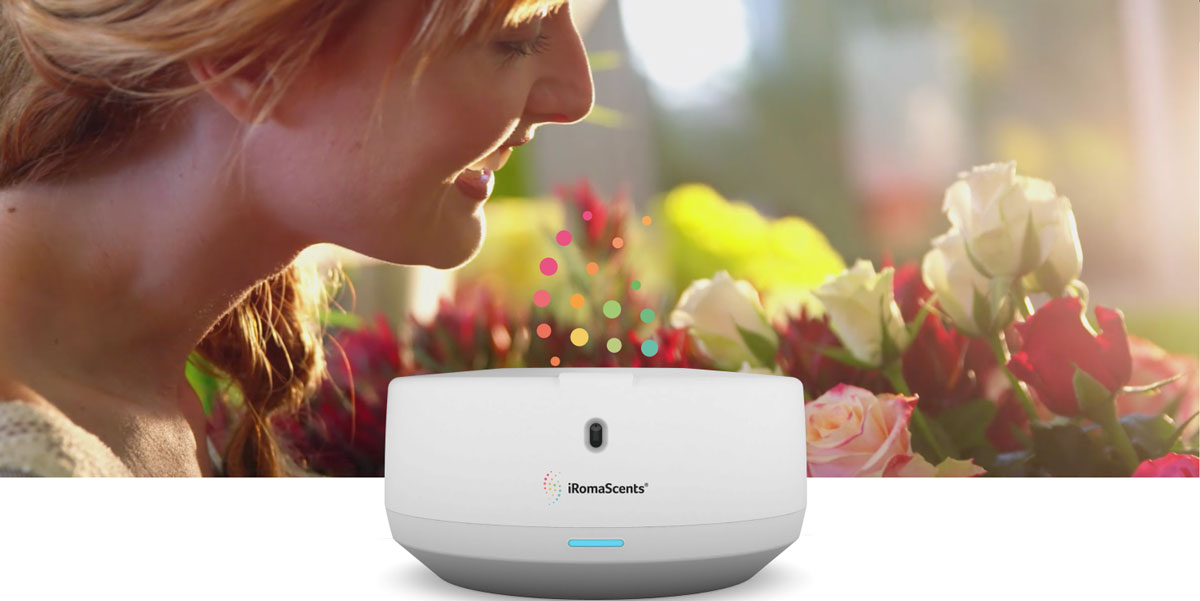

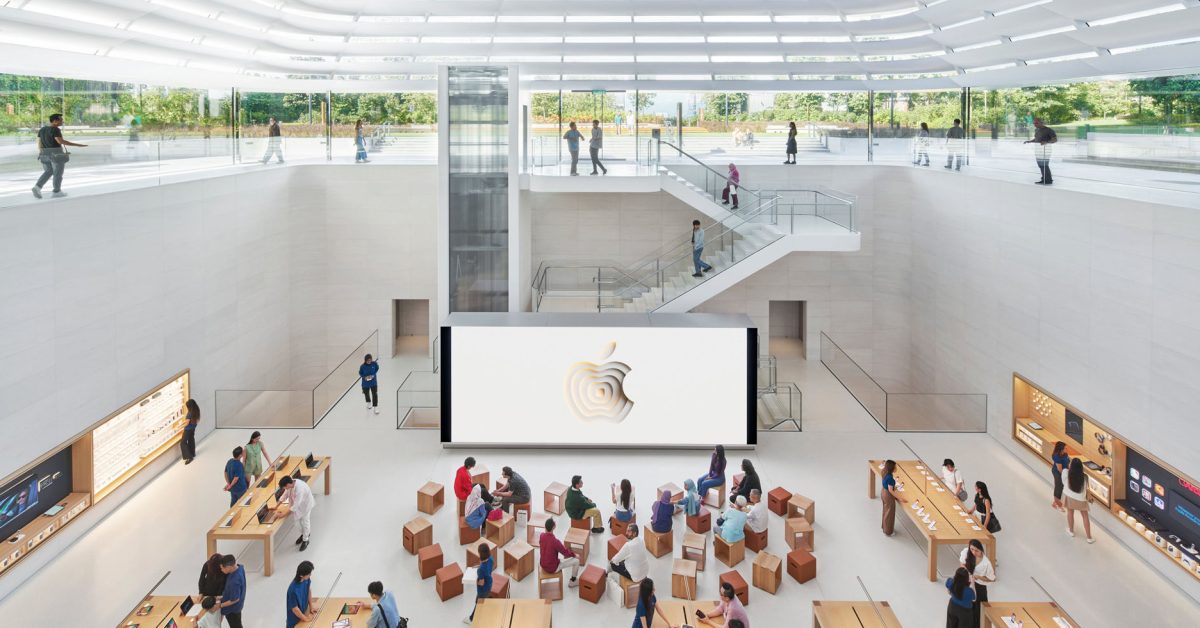


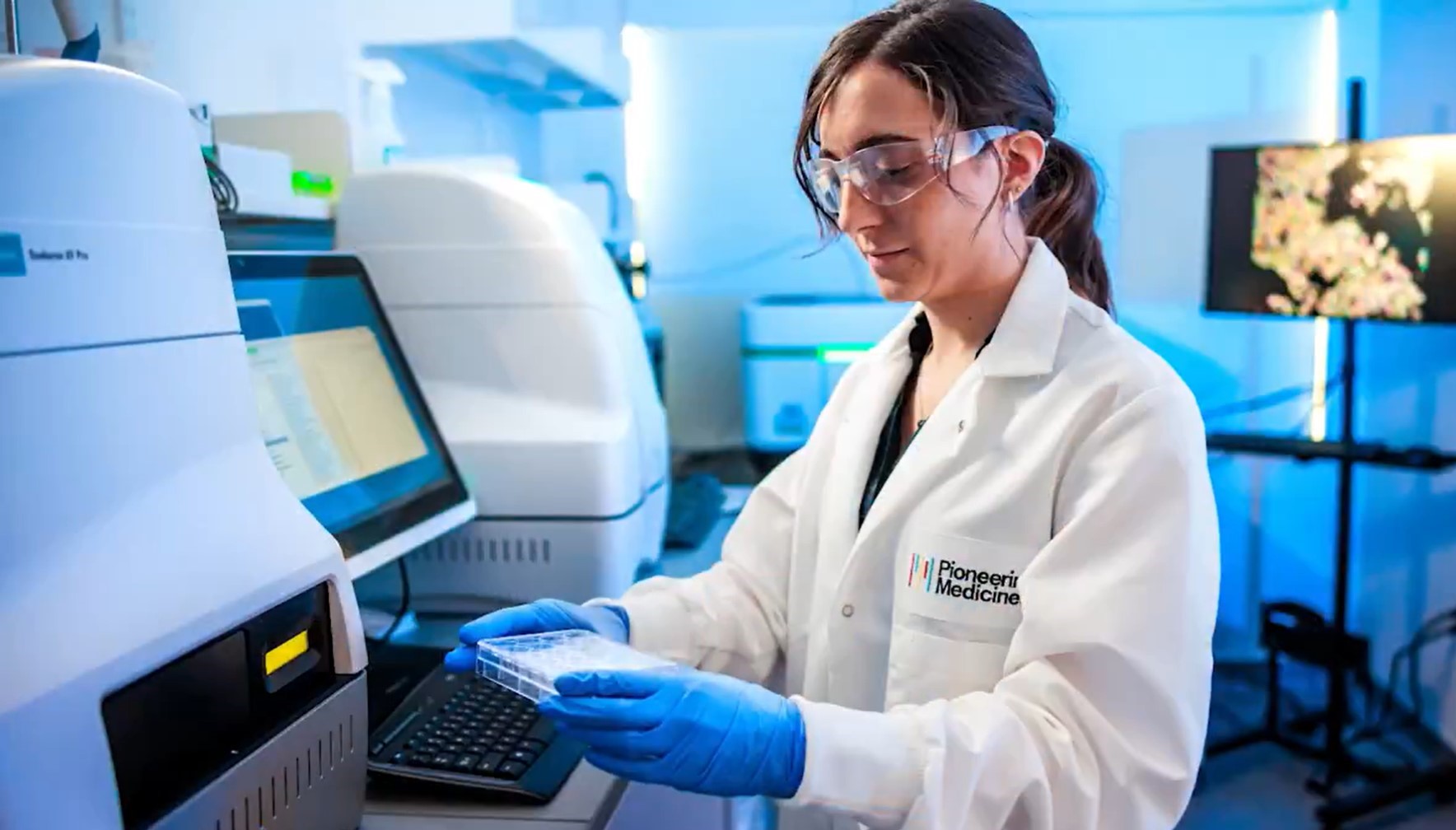
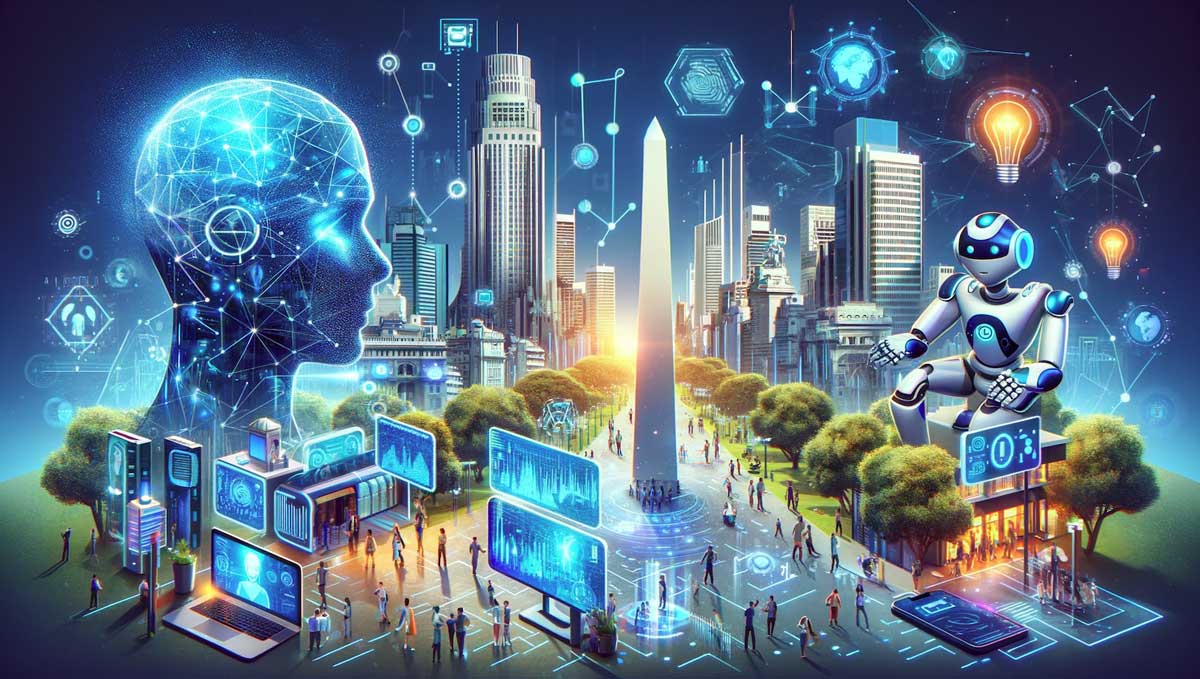

Trending
-
Startups11 meses ago
Remove.bg: La Revolución en la Edición de Imágenes que Debes Conocer
-
Tutoriales12 meses ago
Cómo Comenzar a Utilizar ChatGPT: Una Guía Completa para Principiantes
-
Recursos12 meses ago
Cómo Empezar con Popai.pro: Tu Espacio Personal de IA – Guía Completa, Instalación, Versiones y Precios
-
Startups10 meses ago
Startups de IA en EE.UU. que han recaudado más de $100M en 2024
-
Startups12 meses ago
Deepgram: Revolucionando el Reconocimiento de Voz con IA
-
Recursos11 meses ago
Perplexity aplicado al Marketing Digital y Estrategias SEO
-
Recursos12 meses ago
Suno.com: La Revolución en la Creación Musical con Inteligencia Artificial
-
Noticias10 meses ago
Dos periodistas octogenarios deman a ChatGPT por robar su trabajo