Noticias
Top Ways That Generative AI ChatGPT Helps You Identify And Fulfill Your New Year’s Resolutions
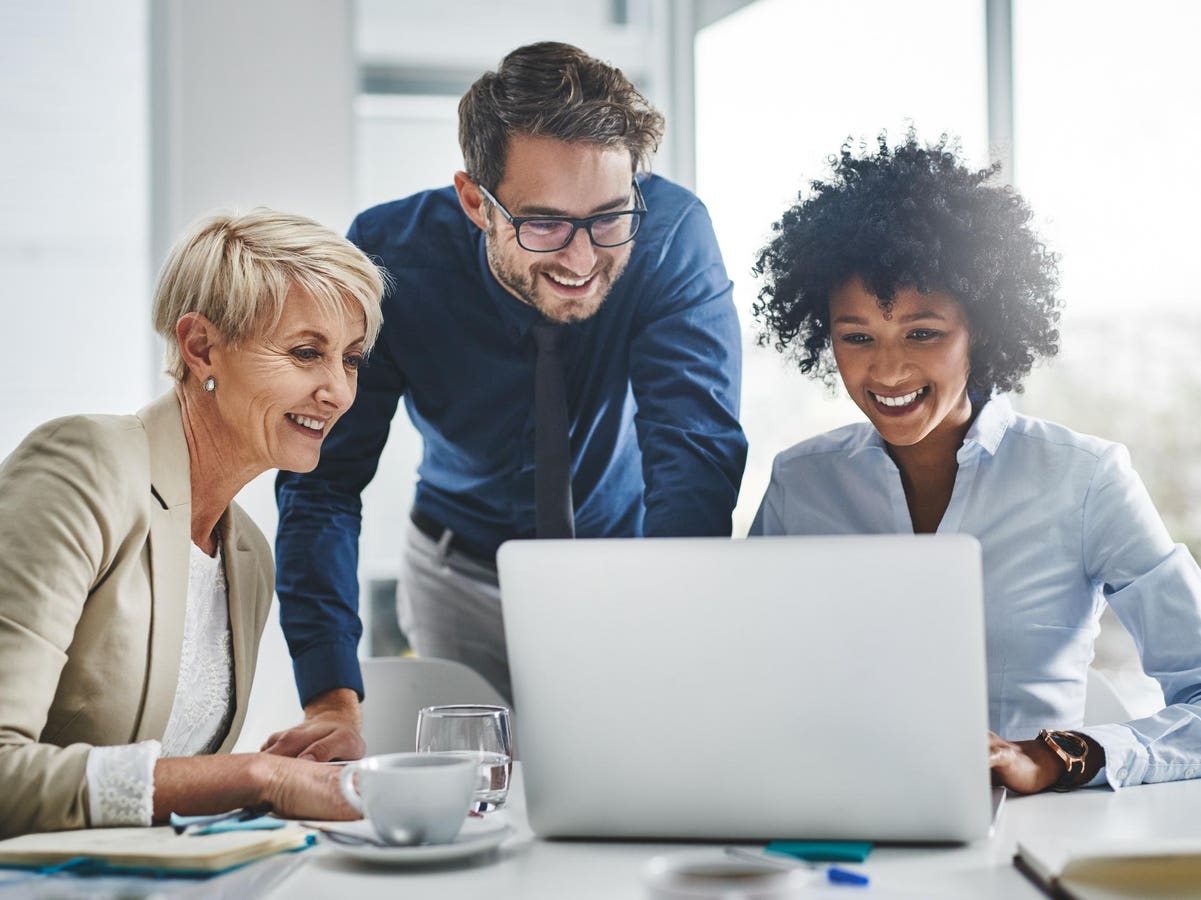
Use generative AI to make and keep your New Year’s resolutions.
In today’s column, I explore the top ways that generative AI and large language models (LLMs) can help people identify their New Year’s resolutions and then throughout the year further assist in fulfilling those resolutions. This might at first glance seem like a lighthearted topic. Not so. The reality is that making and keeping New Year’s resolutions has significant mental health benefits, which in turn provide substantive overarching societal paybacks all told.
Let’s talk about it.
This analysis of an innovative AI breakthrough is part of my ongoing Forbes column coverage on the latest in AI including identifying and explaining various impactful AI complexities (see the link here).
Where Society Stands On New Year’s Resolutions
A longstanding tradition is that when a new year rolls around there is a fresh-start opportunity made available and that we ought to take advantage of the circumstance at hand. People chat incessantly about their desire to make a New Year’s resolution. Some actually come up with resolutions, while many of the big talkers do not (the proverbial all-talk no-action conundrum).
Of those who craft a New Year’s resolution, there is often a relatively slim chance that they will actively follow up and complete the resolution. Resolutions are at times a spur-of-the-moment consideration. You get excited about a verbalized resolution and, while basking in that excitement, earnestly believe that you will devotedly pursue the resolution.
Days later, or sometimes weeks or months later, the resolution falls by the wayside.
What do the stats say?
About 30% of Americans each year declare at least one New Year’s resolution, of which the predominant focus is on improving their overall health such as by exercising or dieting (according to a Pew Research Center study entitled “New Year’s Resolutions: Who Makes Them And Why” by Shanay Gracia, January 29, 2024). The same study also indicated that of the 70% of Americans who didn’t make a resolution, those naysayers insisted that they do not like resolutions and either won’t keep one or can’t think of one that they could sufficiently undertake.
Those coming up with resolutions will rarely see their resolutions to actual fruition. Research indicates that only about 10% of people succeed in keeping their resolutions. Included in those tallies are that around 25% quit after the first week, and by the end of February, around 80% have completely abandoned their initial resolutions.
Sad face.
Leaning Into AI To Make Resolutions Come True
Well, those numbers might indeed seem gloomy but any effort to seek self-improvement and embark on new beginnings is a facet of humankind that we ought to relish. Maybe there are ways to help with these weighty matters and improve the odds of making and keeping resolutions. I’ll be sharing with you the details of two new approaches to New Year’s resolutions that incorporate the latest in generative AI.
Those two innovative approaches consist of:
- (1) Making resolutions via AI. Using generative AI such as ChatGPT, Claude, Gemini, Llama, CoPilot, or other major AI apps to aid in devising New Year’s resolutions.
- (2) Keeping resolutions via AI. Furthering the first approach, the second approach entails using generative AI to serve as a stirring motivator for fulfilling New Year’s resolutions throughout the year.
I’d like to establish stretch goals for us all, so here we go with some very special New Year’s resolutions for the year ahead:
- Outsized Resolution #1: AI dramatically increases the making of fulfillable resolutions, rising from 30% of the populace to a dreamy 60% (doubling!).
- Outsized Resolution #2: AI demonstrably drives people toward attaining resolutions, increasing from 10% fulfillment to a heralded 50% (half of all resolutions are actively completed).
Come on everyone, we can make those exciting resolutions happen if we put our noses to the grind and opt to leverage modern-day AI. Keep those spirits high and don’t be deterred.
We can do it.
How Generative AI Aids The Making Of Resolutions
I am betting that you would like to see an example of how generative AI comes into this realm. I went ahead and logged into ChatGPT by OpenAI to come up with an example for you. You might find of keen interest that ChatGPT garners a whopping 300 million weekly active users. That is a staggering amount of usage.
I briefly conducted an additional cursory analysis via other major generative AI apps, such as Anthropic Claude, Google Gemini, Microsoft Copilot, and Meta Llama, and found their answers to be about the same as that of ChatGPT. I’ll focus on ChatGPT but note that the other AI apps generated roughly similar responses.
Let’s get underway.
- My entered prompt: “I want to set some New Year’s resolutions, but I’m not sure where to start. Can you help?”
- Generative AI response: “Absolutely! Let’s figure it out together. What’s something of a personal nature that you are especially proud of achieving this past year?”
- My entered prompt: “I’m proud that I started going to the gym.”
- Generative AI response: “That’s fantastic! Do you want to build on that success, or focus on a different area of your life?”
- My entered prompt: “I’d like to keep improving my fitness.”
I asked a broad question about setting resolutions. The AI could have sharply told me to specifically do this or that, as though all resolutions are the same or that all people are the same. Instead, the AI sought to figure out what kinds of resolutions might best fit me.
If you were to try and devise resolutions by talking to a fellow human, the chances are they might immediately tell you to lose weight or take some action that they believe you should pursue. Rarer are the odds that someone would first inquire as to what might work best for you. Generative AI is typically shaped by AI makers to be accommodating and less likely to prejudge (side note — that’s not always the case, plus it is possible to use system prompts to instruct AI to be more controlling, see my discussion at the link here).
Backstory On Why Resolutions Don’t Stick
Before I continue with the example of using generative AI to figure out resolutions, it would be handy to take a step back and consider why resolutions overall do not tend to become reality.
These are my five major indications about why resolutions don’t stick:
- (1) People tend to make unrealistic resolutions: Resolutions are often overly ambitious or vague, making them hard to achieve.
- (2) Lack of laid-out specifics: People often fail to stipulate actionable steps, thus making follow-through difficult or confounding.
- (3) Not enough motivation: Resolutions are usually just a bright gleam in the eye and the initial enthusiasm dissipates quickly. There needs to be strong determination to get the job done.
- (4) Absence of accountability: People rarely establish a clear-cut means of being held accountable for fulfilling their resolution. Without accountability, it is all too easy to abandon resolutions.
- (5) All-or-nothing fallacy: Resolutions are viewed as being all-or-nothing, namely that you either complete the resolution to the final end or you aren’t even going to start. This becomes a wink-wink of an excuse to give up on the resolution, rather than acknowledging that even a partial attainment is worthy.
With those gotchas in mind, we can take a further look at generative AI to see how AI helps overcome those hurdles.
The Example Enters Into Finer Interactive Detail
Whenever you use generative AI, try to think of the AI as a conversational partner. The idea is that you are carrying on an interactive dialogue with AI. Some people do a one-and-done angle whereby they ask a question, get an answer, and do not undertake a dialogue with the AI about the matter at hand. That is a shortchanging of what AI can more fully provide. See my explanation about how to get more out of generative AI conversationally, at the link here.
To illustrate the value of engaging in a dialogue, let’s continue my quest to identify New Year’s resolutions about my interest in fitness.
- Generative AI response: “For fitness, what’s one specific goal you’d like to achieve? For example, running a race, building muscle, or trying a new sport?”
- My entered prompt: “I’ve always wanted to run a 5K.”
- Generative AI response: “Excellent goal! Here’s one suggestion. Train weekly for and then complete a 5K race by mid-year. Does that seem like a resolution you’d be interested in pursuing?”
- My entered prompt: “Yes, that would be fantastic.”
- Generative AI response: “Let’s break it down step by step. For the 5K, we can create together a weekly running schedule. Would you like to do so?”
- My entered prompt: “I welcome your help.”
The above interaction illustrates that generative AI is aiding me in doing a deep dive into what my resolutions can be.
Had I merely stopped at the idea of pursuing better fitness for the year, I would have had a vacuous resolution. The AI has given me the tangible notion of doing weekly progression on my resolution. In addition, I now have a goal that will keep me targeted for the next six months.
Generative AI is overcoming the gotchas normally associated with New Year’s resolutions.
Easy-peasy.
The Big Picture Of AI Resolution Assistance
Now that we’ve gotten a taste of what generative AI can achieve, let’s shift into the big-picture mode and consider the range of opportunities involved.
Here are my six top ways that AI can rise to this occasion:
- (1) AI assists in crafting resolutions: Generative AI can analyze your individual preferences, past behaviors, and goals to then recommend resolutions that align with your interests and values.
- (2) AI enmeshes societal trends into resolutions: Generative AI can generate resolution ideas for you based on current trends, such as mindfulness practices, career development strategies, and the like. This might inspire interest in making and keeping resolutions.
- (3) AI advises on setting realistic resolutions: Generative AI carries on an engaging dialogue about whether the resolutions are viable for you, working through the pluses and minuses of potential resolutions.
- (4) AI drafts a useful plan of attainment: Generative AI can draft a proposed plan for the next months or years ahead that will stepwise get you to achieving your resolutions.
- (5) AI reminds and nudges you: Generative AI can remind you about your resolutions, doing so in a gentle way. In addition, the AI can encourage and nudge you along the arduous path of attaining the resolutions.
- (6) AI serves up emotional support: Generative AI can provide a semblance of emotional support by congratulating you on small wins, offering a shoulder to cry on when setbacks occur, and otherwise trying to keep you positive and resilient. This seems odd since AI is not sentient and does not have emotions as humans do, but the wording produced can still seem stirring anyway.
There are additional means of leveraging AI, but I believe those provide a sound foundation and get across the mainstay aspects.
AI As Your Ongoing Resolution Motivator
So far, I have shown an example of generative AI helping to establish resolutions. That is just the starting point. Throughout the year, you can check in with AI as a progress tracker and a motivational tool.
Imagine that I came back to my generative AI conversation in early March.
Things might go like this.
- My entered prompt: “I dropped the ball on my resolutions. I only ran a couple of times in the last two months. I won’t at all be ready to run a 5K by mid-year.”
- Generative AI response: “Don’t be too hard on yourself! You’ve already made some progress, and that’s worth celebrating. Let’s adjust so we can get you back on track.”
- My entered prompt: “Okay, thanks, what should I do?”
- Generative AI response: “Could you commit to two 15-minute runs this week? Small wins build momentum.”
- My entered prompt: “Yes, I can do that.”
- Generative AI response: Great! We’ll add a few minutes each week. Remember, progress is better than perfection.”
Observe that I admitted to AI that I had not been suitably pursuing my resolution to increase my fitness.
This brings up some important points about the use of generative AI.
First, you might be reluctant to admit to a fellow human that you’ve fallen off track with your resolutions. Telling AI that you did so would seem a lot less imposing. In fact, some use generative AI to divulge their innermost secrets that they would never say to another person.
Second, many users do not realize that generative AI is usually not going to preserve their privacy. The licensing agreement for whichever AI that you use will stipulate what their privacy policy is. Much of the time, the AI maker indicates that they can have their AI developers and testers review your entered data. Equally alarming is that they reserve the right to reuse your data to further data train the AI, see my detailed discussion at the link here.
Downsides Of AI As Your Resolution Guru
The upsides of using generative AI for resolutions must be balanced against the downsides. It is a tradeoff that you’ll need to consider.
These are my five top-most downsides of using AI as your resolution guru:
- (1) Your privacy could be exposed: People tend to falsely assume that whatever they enter into generative AI is kept strictly private and confidential. Nope, that’s usually not the case. AI makers tend to indicate in their licensing that they can review your entered data, including reusing it for further training of the AI. See my discussion at the link here.
- (2) AI can put undue pressure on you: Generative AI might inadvertently put too much pressure on you by sending you continual reminders and being an annoyance. The good news is that you can easily tell the AI to be less demanding if that’s what you’d prefer.
- (3) AI biases might mess with your resolutions: Generative AI has cooked-in biases based on data training and however the AI developers tilted the AI, see my discussion at the link here. In the case of resolutions, the AI could attempt to steer you toward particular resolutions based on cultural biases.
- (4) AI might convey a false sense of progress: Generative AI can encounter said-to-be AI hallucinations that are made-up confabulations. This might falsely lead you to believe that you are doing worse than you really are, or better than you really are, regarding your resolutions. For details about how to cope with AI hallucinations, see the link here.
- (5) Spurring overreliance on AI: Generative AI could become a kind of mental crutch that is not only required for pursuing your resolutions, but the AI might also be a dependency that you use for all sorts of aspects of your existence. There is also a chance that you might forsake human comradery in place of being reliant on AI.
Research On New Year’s Resolutions
On the overall topic of New Year’s resolutions and human behavior, many fascinating research studies examine the meaty topic.
For example, one study suggests that resolutions are more likely to be achieved if they are based on approach-oriented goals rather than avoidance-oriented goals. The study is entitled “A Large-Scale Experiment On New Year’s Resolutions: Approach-Oriented Goals Are More Successful Than Avoidance-Oriented Goals” by Martin Oscarsson, Per Carlbring, Gerhard Andersson, and Alexander Rozental, PLOS One, December 9, 2020, and made these salient points (excerpts):
- “Common New Year’s resolutions focus on changes in behavior with an expectation of positive outcomes regarding physical and mental health.”
- “Increasing the likelihood of people succeeding with their New Year’s resolutions could both be beneficial for the individual and for society.”
- “The most popular resolution among the participants concerned physical health (33%). The second most popular category was weight loss (20%). The third most popular category was the desire to change one’s eating habits (13%), followed by resolutions regarding personal growth (9%) and mental health/sleep (5%) as the fourth- and fifth-most popular categories, respectively. The remaining participants (20%) made resolutions regarding work, studies, tobacco habits, etc.”
- “At a one-year follow-up, 55% of responders considered themselves successful in sustaining their resolutions.”
- “Participants with approach-oriented goals were significantly more successful than those with avoidance-oriented goals.”
Many such research studies are readily found online.
Make Your New Year’s Resolutions Now
I urge you to consider making New Year’s resolutions.
Whether you use AI is another facet and I am suggesting that at least try to make resolutions regardless of how you get there. During the year, pursue the resolutions and accept that you might only partially fulfill them. The odds are that getting something done is better than getting nothing done. If you can, consider including AI in your various strategies for making and fulfilling your resolutions. I think you’ll be pleased with the result.
A final thought for now. As the wise words of Benjamin Franklin emphasize: “Let each new year find you a better person.” Yes, be a better person, and consider using AI to your own benefit to become a better person.
That’s a helpful and achievable resolution right there.
Noticias
“Es una suerte y una lección de humildad” trabajar hacia la superinteligencia
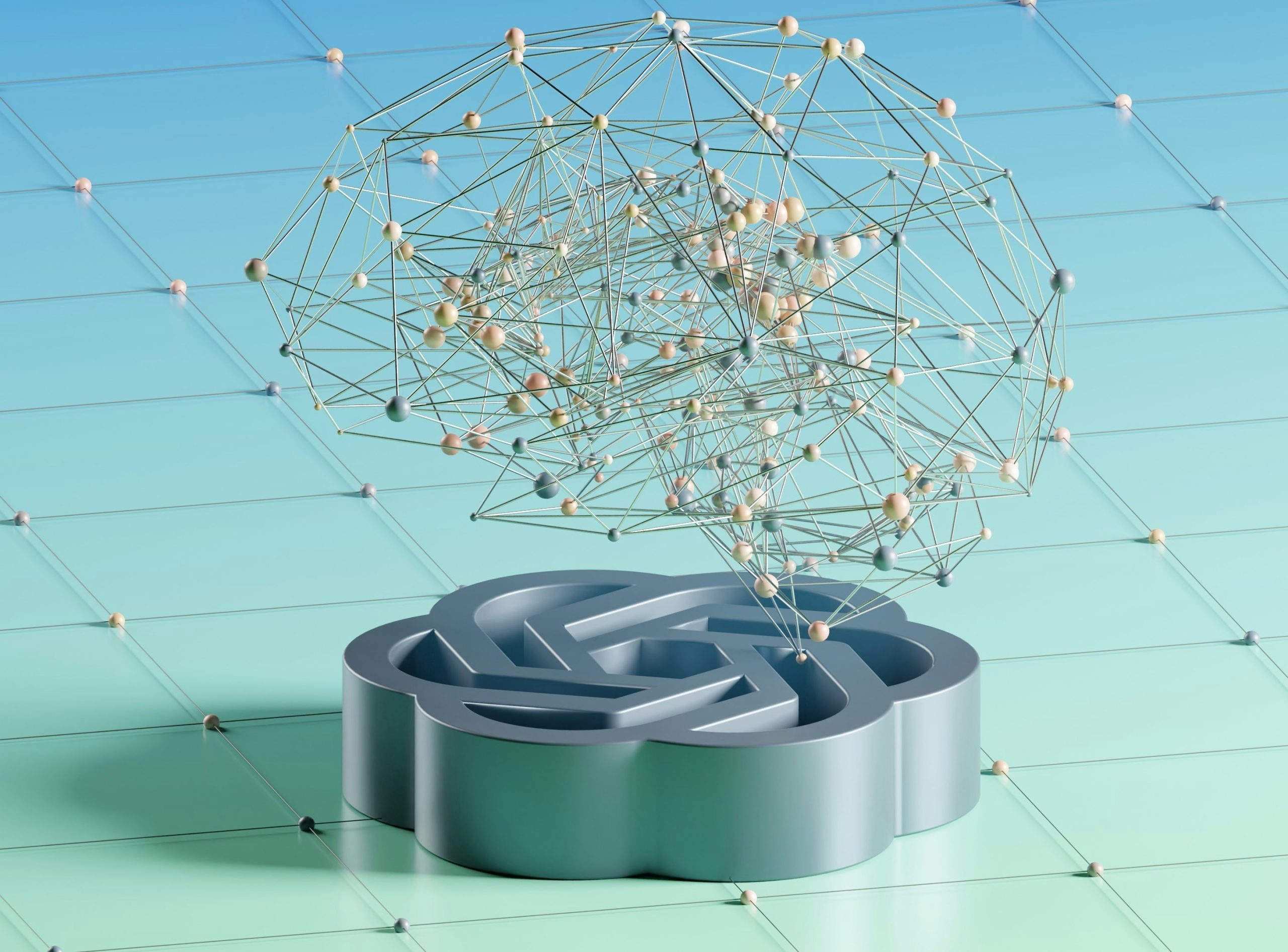
Sam Altman, director ejecutivo y cofundador de OpenAI, ha compartido reflexiones sinceras sobre el viaje de la empresa en su objetivo de lograr la superinteligencia.
Ahora que ChatGPT celebra recientemente su segundo aniversario, Altman describe los logros de OpenAI, los desafíos actuales y la visión para el futuro de la IA.
“El segundo cumpleaños de ChatGPT fue hace sólo poco más de un mes, y ahora hemos hecho la transición al siguiente paradigma de modelos que pueden realizar razonamientos complejos”, reflexiona Altman.
Una misión audaz para lograr AGI y superinteligencia
OpenAI se fundó en 2015 con una misión clara, aunque audaz: desarrollar AGI y garantizar que beneficie a toda la humanidad.
Altman y el equipo fundador creían que AGI podría convertirse en “la tecnología de mayor impacto en la historia de la humanidad”. Sin embargo, recuerda, el mundo no estaba particularmente interesado en su búsqueda en aquel entonces.
“En ese momento, a muy pocas personas les importaba, y si les importaba, era principalmente porque pensaban que no teníamos posibilidades de éxito”, explica Altman.
Un avance rápido hasta 2022, OpenAI todavía era una instalación de investigación relativamente silenciosa que probaba lo que entonces se conocía como “Chat With GPT-3.5”. Los desarrolladores habían estado explorando las capacidades de su API y el entusiasmo despertó la idea de lanzar una demostración lista para el usuario.
Esta demostración condujo a la creación de ChatGPT, que Altman reconoce que se benefició de una marca “afortunadamente” mejor que su nombre inicial. Cuando se lanzó el 30 de noviembre de 2022, ChatGPT demostró ser un punto de inflexión.
“El lanzamiento de ChatGPT inició una curva de crecimiento como nunca antes habíamos visto, en nuestra empresa, nuestra industria y el mundo en general”, dice.
Desde entonces, OpenAI ha sido testigo de una evolución marcada por un interés asombroso, no sólo en sus herramientas sino en las posibilidades más amplias de la IA.
Construyendo a una velocidad vertiginosa
Altman admite que convertir OpenAI en una potencia tecnológica global planteó desafíos importantes.
“En los últimos dos años, tuvimos que construir una empresa entera, casi desde cero, en torno a esta nueva tecnología”, señala, y añade: “No hay manera de formar a la gente para esto excepto haciéndolo”.
Al operar en aguas desconocidas, el equipo de OpenAI a menudo se enfrentó a la ambigüedad: tomó decisiones sobre la marcha y tuvo que lidiar con los inevitables pasos en falso.
“Construir una empresa a tan alta velocidad con tan poca capacitación es un proceso complicado”, explica Altman. “A menudo son dos pasos hacia adelante y uno hacia atrás (y, a veces, un paso hacia adelante y dos hacia atrás)”.
Sin embargo, a pesar del caos, Altman atribuye el mérito a la resiliencia y la capacidad de adaptación del equipo.
OpenAI ahora cuenta con más de 300 millones de usuarios activos semanales, un fuerte aumento con respecto a los 100 millones reportados hace apenas un año. Gran parte de este éxito radica en el espíritu de la organización de aprender haciendo, combinado con el compromiso de llevar al mundo “tecnología que la gente realmente parece amar y que resuelve problemas reales”.
“Un gran fracaso de la gobernanza”
Por supuesto, el viaje hasta ahora no ha estado exento de turbulencias. Altman relata un capítulo particularmente difícil de noviembre de 2023, cuando fue repentinamente destituido como director ejecutivo, contratado brevemente por Microsoft, para ser reinstalado por OpenAI días después en medio de una reacción violenta de la industria y protestas del personal.
Hablando abiertamente, Altman destaca la necesidad de mejores estructuras de gobernanza en las organizaciones que abordan tecnologías críticas como la IA.
“Todo el evento fue, en mi opinión, un gran fracaso de la gobernanza por parte de personas bien intencionadas, incluido yo mismo”, admite. “Mirando hacia atrás, ciertamente desearía haber hecho las cosas de manera diferente y me gustaría creer que hoy soy un líder mejor y más reflexivo que hace un año”.
El episodio sirvió como un crudo recordatorio de la complejidad de gestionar el rápido crecimiento y lo que está en juego en el desarrollo de la IA. También impulsó a OpenAI a forjar nuevas estructuras de gobernanza “que nos permitan llevar a cabo nuestra misión de garantizar que AGI beneficie a toda la humanidad”.
Altman expresó su profunda gratitud por el apoyo que OpenAI recibió durante la crisis por parte de empleados, socios y clientes. “Lo más importante que aprendí es cuánto tengo que estar agradecido y a cuántas personas debo gratitud”, enfatiza.
Pivotando hacia la superinteligencia
De cara al futuro, Altman dice que OpenAI está empezando a apuntar más allá de la AGI hacia el desarrollo de “superinteligencia”, sistemas de IA que superan con creces las capacidades cognitivas humanas.
“Ahora estamos seguros de que sabemos cómo construir AGI como lo hemos entendido tradicionalmente”, comparte Altman. OpenAI predice que para finales de este año, los agentes de IA se “unirán significativamente a la fuerza laboral”, revolucionando las industrias con sistemas complementarios y de automatización más inteligentes.
Lograr la superinteligencia sería especialmente transformador para la sociedad, con el potencial de acelerar los descubrimientos científicos, pero también plantea los peligros más importantes.
“Creemos en la importancia de ser líderes mundiales en investigación de seguridad y alineación… OpenAI no puede ser una empresa normal”, señala, subrayando la necesidad de abordar la innovación de manera responsable.
La estrategia de OpenAI incluye introducir gradualmente avances en el mundo, permitiendo que la sociedad se adapte a la rápida evolución de la IA. “Poner de forma iterativa excelentes herramientas en manos de las personas conduce a resultados excelentes y ampliamente distribuidos”, argumenta Altman.
Al reflexionar sobre la trayectoria de la organización, Altman admite que el camino de OpenAI ha estado definido tanto por avances extraordinarios como por desafíos importantes, desde ampliar equipos hasta navegar el escrutinio público.
“Hace nueve años, realmente no teníamos idea de en qué nos íbamos a convertir; Incluso ahora, sólo lo sabemos en cierto modo”, afirma.
Lo que queda claro es su compromiso inquebrantable con la visión de OpenAI. “Nuestra visión no cambiará; Nuestras tácticas seguirán evolucionando”, afirma Altman, atribuyendo el notable progreso de la empresa a la voluntad del equipo de repensar los procesos y aceptar los desafíos.
A medida que la IA continúa remodelando las industrias y la vida diaria, el mensaje central de Altman es evidente: si bien el viaje no ha sido nada sencillo, OpenAI se mantiene firme en su misión de desbloquear los beneficios de la IA para todos.
“Qué suerte y qué humildad es poder desempeñar un papel en este trabajo”, concluye Altman.
Ver también: OpenAI financia un estudio de 1 millón de dólares sobre IA y moralidad en la Universidad de Duke
¿Quiere aprender más sobre IA y big data de la mano de los líderes de la industria? Echa un vistazo a AI & Big Data Expo que se lleva a cabo en Ámsterdam, California y Londres. El evento integral comparte ubicación con otros eventos líderes, como la Conferencia de Automatización Inteligente, BlockX, la Semana de Transformación Digital y la Cyber Security & Cloud Expo.
Explore otros próximos eventos y seminarios web de tecnología empresarial impulsados por TechForge aquí.
Noticias
Mientras OpenAI busca ganancias, el activista busca vengarse del público
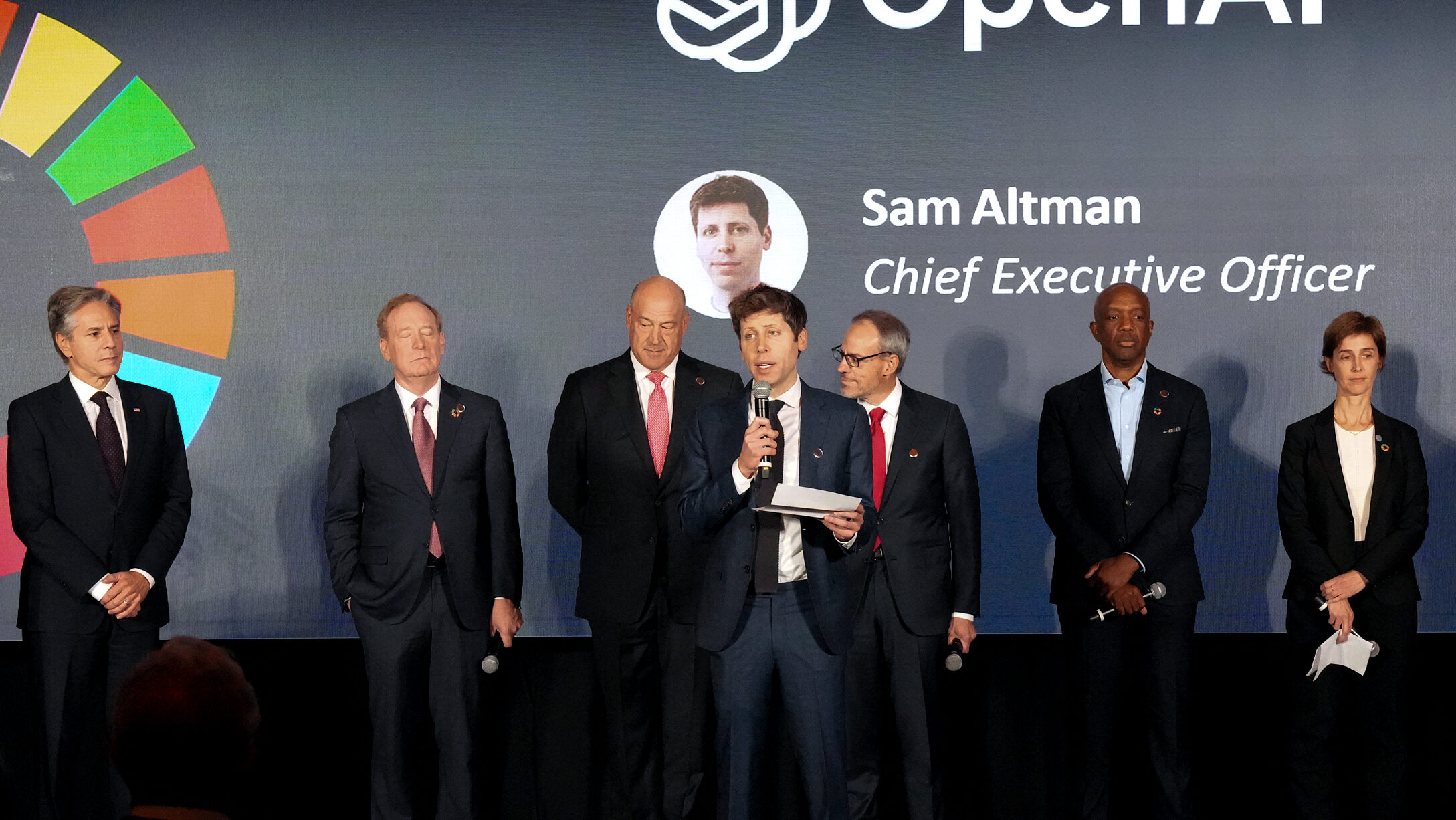
Se está gestando una batalla por la reestructuración de OpenAI, el creador del chatbot pionero de inteligencia artificial ChatGPT. Se fundó como una organización sin fines de lucro en 2015 con el objetivo de desarrollar IA para beneficiar a la humanidad, no a los inversores. Pero la IA avanzada requiere una potencia de procesamiento masiva, lo que se vuelve costoso, lo que influye en la decisión de la empresa de contratar grandes inversores. Recientemente, OpenAI dio a conocer un plan para la transición a una corporación de beneficio público con fines de lucro.
Ese plan ha generado objeciones de personas como Elon Musk, Meta y Robert Weissman, copresidente del grupo de defensa del consumidor Public Citizen, que instó a las autoridades de California a garantizar que, a medida que OpenAI se reorganice, devolverá gran parte de los beneficios que recibió como organización sin fines de lucro. .
La siguiente es una transcripción editada de la conversación de Weissman con Meghan McCarty Carino de Marketplace.
Robert Weissman: Ser una organización sin fines de lucro habilitada [OpenAI] aceptar donaciones, y ese fue el modelo para la organización sin fines de lucro, al igual que es el modelo para otras organizaciones sin fines de lucro. Habían donado dinero en efectivo y donaciones en especie de poder computacional para hacer su trabajo de desarrollar esta nueva tecnología. No tenían que fingir que iban a poder devolver la inversión a la gente. Les pidieron que lo hicieran con fines benéficos y pudieron recaudar una cantidad significativa de dinero y recursos a través de ese enfoque.
Meghan McCarty Cariño: Entonces, tengo entendido que en 2019, OpenAI hizo una transición a una estructura diferente para poder recibir dinero de inversores como Microsoft. Pero el negocio, un negocio con ganancias limitadas, todavía ha sido gobernado aparentemente por la organización sin fines de lucro. Ahora, busca convertirse en una corporación de beneficio público con fines de lucro. ¿Qué dice la ley sobre lo que se requiere para realizar este tipo de pivote?
Weissman: Esto es algo muy, muy inusual, y tal vez ninguna entidad haya seguido exactamente el camino que ha tomado OpenAI. Pero hay una historia de organizaciones sin fines de lucro que se convierten en organizaciones con fines de lucro, que no es exactamente lo que está haciendo OpenAI, pero es la historia central de lo que está haciendo OpenAI. Y para hacer esa conversión, tendrán que recibir efectivamente el visto bueno de los fiscales generales de Delaware, donde está constituida la operación, y de California, donde están registrados y donde hacen negocios.
En la historia de este tipo de conversiones, si vas a sacar activos del sector sin fines de lucro, tienes que devolverle al sector sin fines de lucro el valor de lo que estás tomando. Si diriges una empresa benéfica, no puedes privatizarla de repente y hacerla tuya. Si eres el director ejecutivo de una organización benéfica, no puedes simplemente donarla a una corporación, o no puedes simplemente convertir tu organización sin fines de lucro en una corporación con fines de lucro porque pudiste tener éxito y evolucionar bajo el paraguas de acuerdos sin fines de lucro, apoyo deducible de impuestos, etc. Tienes que devolverle el dinero al sector caritativo. El precedente más importante de este tipo de conversiones es la conversión de compañías de seguros médicos Blue Cross sin fines de lucro en compañías de atención médica Blue Cross con fines de lucro. Esto ocurrió en todo Estados Unidos y en todos los estados donde sucedió, la entidad con fines de lucro debía devolver al sector sin fines de lucro una cantidad igual en valor a lo que estaban privatizando. Por lo general, ese dinero se dedicaba luego a una fundación benéfica de atención médica. Muchas grandes fundaciones benéficas de atención médica todavía existen, incluso en California, a partir de ese tipo de conversiones.
McCarty Carino: Entonces, ¿qué ha sugerido OpenAI sobre cómo realizaría esta transición y qué le preocupa sobre su plan?
Weissman: Bueno, las cosas han evolucionado rápidamente y muy recientemente. Ahora han anunciado su intención de hacerlo, aunque ya se rumoreaba desde hacía algún tiempo. Lo que OpenAI dice que van a hacer es escindir su filial con fines de lucro. En este momento, tienen una junta sin fines de lucro que controla una afiliada con fines de lucro, y la organización con fines de lucro pagaría a la organización sin fines de lucro el valor de lo que están tomando, y eso sería propiedad de la organización sin fines de lucro en forma de acciones en el nuevo OpenAI independiente y con fines de lucro. Así que proponen hacer ese pago al sector sin fines de lucro básicamente pagándose a ellos mismos, lo cual creemos que no es una buena idea.
McCarty Carino: Así que usted escribió una carta al fiscal general de California, Rob Bonta, en septiembre defendiendo que OpenAI pagara al menos 30 mil millones de dólares y compartiera cualquier tecnología de inteligencia artificial general básicamente con una fundación benéfica, una fundación benéfica independiente. ¿Cómo se te ocurrió eso?
Weissman: Bueno, primero comenzamos a comunicarnos con los fiscales generales de California y también de Delaware después de la muy extraña y muy publicitada reorganización de la junta directiva de OpenAI en noviembre de 2023, porque lo que vimos que sucedió allí fue que la junta sin fines de lucro intentaba ejercer control sobre la afiliada con fines de lucro. y perdiendo. Las fuerzas con fines de lucro dentro y alrededor de OpenAI básicamente abrumaron a la junta directiva de las organizaciones sin fines de lucro, las expulsaron y las reemplazaron con gente nueva. En ese momento, nos pareció que esta entidad, fuera lo que fuera, esta entidad combinada, ya no funcionaba realmente como una organización sin fines de lucro. Efectivamente se había convertido en una empresa con fines de lucro. Y empezamos a decir, bueno, si ese es el caso, entonces tienen el deber de pagar al sector sin fines de lucro el valor de lo que están retirando, tal como fue el caso con estas conversiones de Blue Cross. Ahora, poco más de un año después, OpenAI dice: sí, es cierto, en realidad ya no queremos pretender ser una organización sin fines de lucro; queremos hacer esa conversión. Entonces, antes de que esto suceda, dijimos, si va a haber una conversión, si realmente se va a hacer eso formalmente, o si se va a obligar a hacerlo, para reconocer lo que efectivamente ya sucedió, ¿cuál es el valor? que tienen que devolver el dinero?
Ahora han desarrollado una estructura muy extraña, no toda transparente. No está claro que OpenAI, la organización sin fines de lucro, tenga muchas acciones o tenga mucha participación accionaria en OpenAI, la organización con fines de lucro. Sin embargo, según los términos de configuración de toda la operación, sí tiene control sobre OpenAI con fines de lucro. Entonces dijimos, mire, al menos se les debe la prima de control, que en el extremo inferior es el 20% del valor de una empresa adquirida en la mayoría de las transacciones que tienen lugar y en el mercado de valores y adquisiciones regulares. Bueno, el 20% de los 150.000 millones de dólares, que es el valor actual de OpenAI, supone un mínimo de 30.000 millones de dólares. Hay muchas razones para pensar que las cifras deberían ser más altas, tal vez mucho más altas que eso, pero creemos que 30.000 millones de dólares es la base de lo que hay que pagar. Y nuevamente, para nosotros, no funciona si OpenAI simplemente se paga a sí mismo, básicamente hace que las organizaciones con fines de lucro paguen a las organizaciones sin fines de lucro que son afiliadas y no verdaderamente independientes. Tiene que volver al sector caritativo independiente, lo que probablemente significaría una o más fundaciones independientes que realmente podrían promover los intereses del desarrollo de la IA para el interés público, promover las preocupaciones éticas y de seguridad de la inteligencia artificial y descubrir cómo proporcionar un mayor acceso a personas a las nuevas tecnologías que están surgiendo.
McCarty Carino: Entonces, ¿cómo ve este posible giro que impactará la visión original de OpenAI de anteponer la humanidad a las ganancias en la búsqueda de la inteligencia artificial?
Weissman: Bueno, creo que han abandonado eso. Creo que lo abandonaron antes de esta conversión, por lo que, desde nuestro punto de vista, parece que el genio ya está fuera de la botella. Dicen que se están convirtiendo, que quieren convertirse en una corporación de beneficio público, que tendría la capacidad de considerar tanto intereses lucrativos como no lucrativos. Pero, de hecho, lo que hemos visto con OpenAI durante el último año, en realidad con el lanzamiento de la popular versión de ChatGPT, es que ha sido la menos preocupada por la seguridad, la más agresiva en la introducción de nuevas tecnologías de todas las empresas de IA. . Entonces introdujeron la tecnología que Google más o menos tenía, pero tenía miedo de lanzarla al mercado debido a preocupaciones sobre la seguridad y tal vez debido a la responsabilidad, pero se vio obligado a ponerse al día rápidamente después de que OpenAI avanzó. Estamos viendo eso nuevamente con OpenAI introduciendo tecnologías que tienen una capacidad de voz artificial de increíblemente alta calidad, lo que realmente hace posible y probable que las personas se dejen engañar por voces con sonido humano que serán muy fácilmente implementables a través de Internet. Y esa es también una tecnología que Google había analizado, había considerado demasiado arriesgada y no iba a introducir en el mercado. Pero una vez que un competidor lo hace, los demás rápidamente le siguen. Entonces, desde nuestro punto de vista, OpenAI ha dejado atrás la idea de priorizar la seguridad y la ética y está realmente más interesado en ser el primero en actuar. Así que el viejo lema de Silicon Valley “Muévete rápido y rompe cosas” parece ser lo que OpenAI ha adoptado, a pesar de ser supuestamente una organización sin fines de lucro y una operación que prioriza los intereses de la humanidad por encima de cualquier consideración de lucro.
McCarty Carino: ¿Qué papel podría desempeñar esta fundación benéfica independiente en ese contexto?
Weissman: Bueno, dependiendo del tamaño de la fundación, podría apoyar todo tipo de programas de investigación, promoción, educación y acceso. Podría apoyar la investigación y la innovación diseñadas para garantizar la seguridad. Podría apoyar a empresas emergentes más pequeñas que se estuvieran moviendo en calidad de organización sin fines de lucro y comprometidas a permanecer en esa capacidad. Podría prestar más atención a la seguridad, por un lado, y al acceso a las nuevas tecnologías, por el otro. Podría apoyar los esfuerzos para garantizar que las personas de bajos ingresos tuvieran acceso a nuevas tecnologías a medida que estuvieran disponibles. Podría formar a nuevas personas para que se conviertan en programadores y desarrolladores. Podría respaldar la promoción para hacer retroceder el poder monopólico de empresas como OpenAI. Hay muchísimo que podría hacer, e idealmente, en realidad sería más de una sola base. A esa escala de recursos, creo que sería mejor distribuirlo entre muchos. Pero pase lo que pase, realmente podría convertirse en un actor poderoso para compensar el desafortunado movimiento que estamos viendo de desarrollar estas tecnologías realmente fascinantes mucho antes de considerar la seguridad, la ética y el acceso.
Noticias
La IA y el futuro de la optimización de la búsqueda
La esencia
- ChatGPT Search revoluciona el SEO. La búsqueda impulsada por IA de OpenAI presenta paneles de citas y resultados en lenguaje natural, lo que ofrece una nueva visión de la optimización de la búsqueda.
- Los profesionales del marketing se enfrentan a estrategias en evolución. Desde la investigación matizada de palabras clave hasta diversos formatos de contenido, la IA está remodelando la forma en que las marcas abordan la visibilidad.
- El dominio de Google enfrenta desafíos. La búsqueda mejorada por IA de competidores como OpenAI aumenta las apuestas, pero Google invierte en la nube y en publicidad para mantener su liderazgo.
La “caída del micrófono” en las noticias de búsqueda en línea se produjo en julio pasado. OpenAI creó una lista de espera para la versión beta de SearchGPT, su motor de búsqueda destinado a desafiar al panteón de motores de búsqueda, a saber, Duck Duck Go, Yahoo, Bing y, por supuesto, Google.
OpenAI había guardado silencio durante meses sobre SearchGPT desde el lanzamiento de la versión beta. Los indicios de un silencio roto se produjeron cuando PressGazette informó sobre un comentario de Varun Shetty, jefe de asociaciones de medios de OpenAI. El comentario se hizo durante una conferencia de editores de noticias. Shetty mencionó que SearchGPT se lanzará antes de fin de año.
Luego llegó Halloween, que se convirtió en la verdadera “caída del micrófono”. OpenAI sorprendió a los especialistas en marketing con el lanzamiento de búsquedas de ChatGPT antes de un rumor de lanzamiento en 2025. OpenAI lanzó oficialmente ChatGPT Search unos cuatro meses después del lanzamiento del prototipo SearchGPT.
¿La gran pregunta ahora? Cómo los especialistas en marketing y creadores de contenido planifican sus objetivos de búsqueda para 2025 teniendo en cuenta las tácticas de IA. Muchas estrategias de IA implican la consolidación de la información una vez encontrada en lugar de la consolidación durante el proceso de búsqueda en sí. ChatGPT Search lleva ese proceso completamente a la búsqueda.
Si el interés en ChatGPT Search despega, ¿cómo deberían los especialistas en marketing preparar sus estrategias de búsqueda con este potente entrante de IA?
Tabla de contenido
Cómo se compara la búsqueda de ChatGPT con los motores de búsqueda
El algoritmo de búsqueda ChatGPT está diseñado para evaluar las páginas en línea con respecto a lo que preguntan los textos emergentes. Al igual que otras soluciones de inteligencia artificial, ChatGPT elegirá buscar en la web según lo que usted solicite. Es lo que ciertamente ha hecho Google con sus descripciones generales de IA.
La forma en que el algoritmo del motor de búsqueda realiza su búsqueda es el aspecto más interesante de ChatGPT. ChatGPT Search ofrece un botón debajo de la ventana de texto donde el usuario puede elegir buscar manualmente haciendo clic en el icono de búsqueda web. El usuario hace clic en el botón para activar la búsqueda. ChatGPT responde con un breve resumen y enlaces que indican las fuentes en línea en las que se basa la respuesta. Esto difiere de los motores de búsqueda, que devuelven páginas web clasificadas, archivos PDF y otros hipervínculos entre los cuales el usuario debe determinar la información más buscada.
ChatGPT Search también incluye indexación desde el motor de búsqueda Bing. Esto aumenta el valor de Bing en la optimización de motores de búsqueda porque muchas tácticas SEO recientes se centraron en el voluminoso tráfico de búsqueda de Google.
La incorporación de la búsqueda no es del todo nueva para la IA. Desde sus inicios, Gemini ha ofrecido enlaces en algunas de sus referencias, particularmente a algún buscador de Google o YouTube. Por ejemplo, si hago una pregunta simple sobre los videos principales de un producto, como un SUV, Gemini mostrará tres de los videos principales de YouTube, completos con una imagen en miniatura y una descripción.
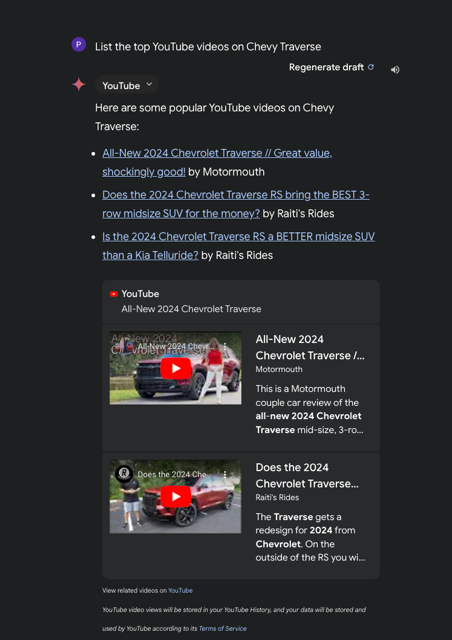
Artículo relacionado: Adiós al SEO: por qué la optimización de la visibilidad de la IA es el futuro
Donde la búsqueda de ChatGPT es diferente
La diferencia que introduce ChatGPT es que su búsqueda de IA cita fuentes con mayor frecuencia y las presenta como un panel de citas.
Alison Ver Halen, experta en marketing de contenidos y presidenta de AV Writing Services, exploró ChatGPT Search y me explicó que un panel de citas “le permite no solo ver de dónde obtuvo ChatGPT Search su respuesta, sino también hacer clic para visitar esos sitios web y obtener más información. Hasta ahora, esos clics han sido principalmente para búsquedas de baja intención, lo que tiene sentido si las personas utilizan principalmente ChatGPT Search para búsquedas de baja intención. Si están listos para comprar, pueden usar Google o buscar directamente en una tienda en línea, como Amazon”.
Y también estamos aprendiendo que los consumidores utilizan cada vez más la búsqueda social para realizar compras.
Además de mejorar la relación con Microsoft a través de Bing, OpenAI se ha movido rápidamente para establecer asociaciones y extensiones para ayudar a los usuarios a acceder cómodamente a ChatGPT Search. MacRumors, una reconocida fuente de noticias sobre productos Apple, informó que la aplicación Atajos de Apple agregó una opción “Abrir SearchGPT” a sus otros comandos ChatGPT. Esto permite a los usuarios de iPhone, iPad y Mac encontrar información relevante en línea a través de las capacidades de búsqueda ChatGPT dentro de sus dispositivos.
Artículo relacionado: OpenAI estrena la búsqueda ChatGPT: ¿Ha cambiado para siempre la búsqueda de contenido en línea?
Lo que aporta ChatGPT Search a las estrategias de búsqueda
ChatGPT Search presenta la búsqueda como un claro llamado a que la IA aproveche la búsqueda; lo hace como una función auxiliar, irónica si la comparamos con la búsqueda por voz o imagen en Google. Esas funciones se activan mediante un icono en el texto.
También revoluciona la experiencia de búsqueda al ofrecer una respuesta en lenguaje natural que sirve como SERP. Hasta ahora, todas las consultas en los motores de búsqueda se han estructurado de la siguiente manera: tienes una ventana de texto donde el usuario escribe algunas palabras para una consulta. Los índices de los motores de búsqueda se centran en qué contenido en línea es relevante para una palabra clave. El resultado son páginas de URL de sitios web clasificadas con descripciones. Todo esto refleja las raíces originales del motor de búsqueda: buscar documentación y clasificar los resultados según su relevancia para la consulta.
La disposición de ChatGPT se adapta a la forma en que las personas buscan información de forma natural.
Diferenciador: ¿Cuál es el contexto de la búsqueda?
Con capacidad de búsqueda, las plataformas de inteligencia artificial como ChatGPT Search pueden incorporar el contexto de la búsqueda. El usuario puede proporcionar un párrafo completo como consulta o cargar medios junto con el texto de la consulta. La respuesta a la consulta es un párrafo breve en lugar de los resultados clasificados de un SERP. Además, el usuario puede hacer más preguntas o agregar más detalles de forma natural, otro diferenciador de la búsqueda por IA.
Google ha incorporado diversos aspectos de los beneficios de la IA con su SGE. Sin embargo, como mencioné en mi publicación sobre SGE, Google AI Overviews es una evolución del fragmento de búsqueda en lugar de una revisión de cómo el venerable motor de búsqueda comparte información.
La adopción de la búsqueda mejorada con IA, como ChatGPT Search, introduce una mayor influencia del contenido en línea a partir de las indicaciones y el lenguaje utilizado para controlar la IA subyacente, por lo que los usuarios utilizarán varias técnicas de ingeniería de indicaciones para instruir la optimización, tratando de utilizar el modelo de lenguaje de manera más efectiva. Esto significa que los especialistas en marketing con estrategias de búsqueda deben considerar las heurísticas que los clientes potenciales probablemente utilizarán para descubrir su contenido o responder al contenido presentado.
Cómo se están preparando los especialistas en marketing para la optimización de la búsqueda con IA
Los profesionales del marketing ya están imaginando qué tácticas surgirán a partir de las influencias que traerán las indicaciones en línea. Pedí a algunos especialistas en marketing que compartieran sus perspectivas sobre las tácticas de SEO que deberían enfatizarse ahora para garantizar que un agente de IA incorpore un sitio web en su rápida respuesta.
Estrategias de palabras clave en evolución para la búsqueda con IA
En su cita sobre ChatGPT Search, Ver Halen señaló cómo evolucionaría la investigación de palabras clave. “ChatGPT Search comprende mejor el contexto y el significado detrás de las palabras”, explica Ver Halen, “lo que significa que las búsquedas tendrán más matices. Como resultado, la investigación de palabras clave tendrá que matizarse más”.
“El hecho de que ChatGPT Search permita a las personas hacer preguntas para obtener más información sobre un tema también debería cambiar la forma en que pensamos sobre el contenido que estamos creando. ¿Qué preguntas de seguimiento podrían tener las personas? ¿Cómo podemos responder esas preguntas en este ¿Se necesitarán piezas adicionales para responder todas sus preguntas?
Diversos formatos de contenido para una mayor visibilidad
Dale Bernard, fundador y director ejecutivo de Fire&Spark, cree que las marcas deben cambiar.
“Las marcas que han pasado una década publicando contenido de blogs educativos para tener visibilidad en Google deben cambiar a nuevos formatos como comparaciones basadas en datos, contenido visual, videos y herramientas interactivas. … Las descripciones generales de IA de Google brindan a los consumidores respuestas directas a sus preguntas de compra, lo que reduce su necesidad de hacer clic en el sitio web de la marca”.
Sin embargo, algunos especialistas en marketing creen que los planes de estrategia de búsqueda no deberían cambiar drásticamente.
Ivana Taylor, fundadora de DIY Marketers y especializada en consejos de marketing, cree que el lanzamiento de la búsqueda basada en inteligencia artificial obligará a los especialistas en marketing de motores de búsqueda a mejorar la forma en que escriben contenido para el lector.
“Sé que es reconfortante pensar que se puede ‘jugar’ con el sistema”, dijo Taylor, “pero si bien hackear el SEO puede ofrecer resultados a corto plazo, a menudo conduce a soluciones a largo plazo que pocas organizaciones pueden permitirse. Al final , mi recomendación para los especialistas en marketing es continuar centrando sus esfuerzos en investigar el mercado, obtener una comprensión profunda de sus clientes ideales y los resultados deseados, y luego ayudarlos a tomar la mejor decisión para ellos. Estos son principios básicos de marketing que se mantendrán. la prueba del tiempo”.
Bernard también está de acuerdo en que la IA generativa “cambiará la forma en que los consumidores se conectan con las marcas, pero los fundamentos para llegar a una base de clientes objetivo y resonar con ella siguen siendo los mismos”.
Artículo relacionado: Las 10 mejores herramientas SEO gratuitas para especialistas en marketing
Lo que los especialistas en marketing deberían esperar a medida que evoluciona la búsqueda con IA
La carrera por reinventar la búsqueda ya ha comenzado. Google sigue siendo el rey de los motores de búsqueda y posee más del 90% del mercado. Sin embargo, se enfrenta a una enorme demanda antimonopolio que provocará una importante alteración de su modelo de negocio.
El dominio de Google desafiado en medio del cambio de IA
Pero no descarte a Google. El reciente anuncio de resultados de Google indica que las inversiones en IA en sus operaciones están dando sus frutos. Aunque las ganancias provienen de sus servicios en la nube y de anuncios, Google tiene la oportunidad de reinvertir esas ganancias en sus venerables servicios tecnológicos como la búsqueda.
Adaptarse a la IA: oportunidades y desafíos para el SEO
Otro factor que pesa como señal positiva para Google son las revisiones de mediciones que deben tener los paneles de análisis y SEO. Pasará algún tiempo hasta que estas soluciones tengan en cuenta la búsqueda mejorada por IA, por lo que las mediciones de las herramientas tradicionales relacionadas con la búsqueda seguirán siendo viables por un tiempo.
Aún así, OpenAI también tiene oportunidades. Todas las plataformas de inteligencia artificial han introducido funciones para administrar mensajes, con la adición de herramientas de soporte como el escritorio ChatGPT antes mencionado. Estas características atraerán a los usuarios de los motores de búsqueda, una experiencia que Google ya ha enfrentado compitiendo con los comportamientos de búsqueda que han surgido en Amazon, TikTok y otras plataformas.
En resumen, los especialistas en marketing deben prestar atención al mundo en línea que se desarrollará en 2025 porque el micrófono que cae de plataformas como ChatGPT finalmente conquistará a los consumidores y cambiará la experiencia del cliente relacionada con el dominio de las búsquedas.
Preguntas centrales sobre la búsqueda de ChatGPT y la búsqueda de IA
¿En qué se diferencia ChatGPT Search de los motores de búsqueda tradicionales como Google?
ChatGPT Search se diferencia de los motores de búsqueda tradicionales al centrarse en el procesamiento del lenguaje natural y proporcionar resúmenes generados por IA con paneles de citas. A diferencia de la lista clasificada de hipervínculos de Google, ChatGPT Search permite a los usuarios realizar consultas detalladas y recibir respuestas concisas y conversacionales junto con las fuentes citadas. Este enfoque permite una experiencia de búsqueda más contextual y humana, lo que permite a los usuarios refinar sus preguntas y respuestas en tiempo real.
¿Qué estrategias deberían adoptar los especialistas en marketing para la búsqueda impulsada por IA como ChatGPT Search?
Los especialistas en marketing deben centrarse en una investigación matizada de palabras clave y anticipar la intención del usuario mediante la creación de contenido que responda posibles preguntas de seguimiento. La diversificación de los formatos de contenido (como comparaciones basadas en datos, herramientas interactivas y activos visuales) puede aumentar la visibilidad en las plataformas de IA. Además, comprender la ingeniería rápida y optimizar las consultas conversacionales ayudará a garantizar que los agentes de inteligencia artificial como ChatGPT incluyan su contenido en las respuestas de búsqueda. Hacer hincapié en la claridad, el valor y la participación de los usuarios sigue siendo fundamental.
Descubra cómo puede unirse a nuestra comunidad de colaboradores.
-
Startups8 meses ago
Remove.bg: La Revolución en la Edición de Imágenes que Debes Conocer
-
Recursos8 meses ago
Cómo Empezar con Popai.pro: Tu Espacio Personal de IA – Guía Completa, Instalación, Versiones y Precios
-
Recursos8 meses ago
Suno.com: La Revolución en la Creación Musical con Inteligencia Artificial
-
Recursos8 meses ago
Perplexity aplicado al Marketing Digital y Estrategias SEO
-
Estudiar IA8 meses ago
Curso de Inteligencia Artificial de UC Berkeley estratégico para negocios
-
Tutoriales8 meses ago
Cómo Comenzar a Utilizar ChatGPT: Una Guía Completa para Principiantes
-
Eventos8 meses ago
La nueva era de la inteligencia artificial por el Washington Post – Mayo 2024
-
Noticias6 meses ago
Dos periodistas octogenarios deman a ChatGPT por robar su trabajo