Noticias
Centros de datos, reglas de IA, límites de chips y política de conversaciones de OpenAI
Published
3 meses agoon
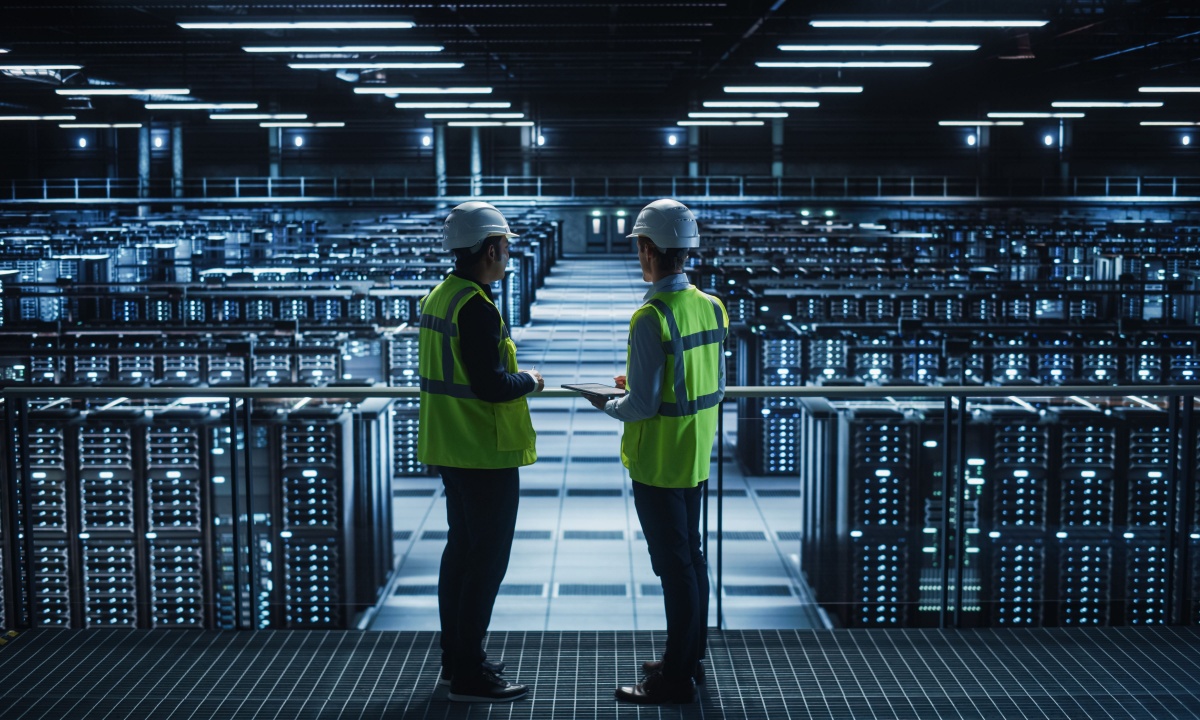
Cuando queda una semana en el cargo, el presidente Biden firmado una orden ejecutiva que reserva terrenos federales para la construcción de centros de datos de inteligencia artificial (IA), y el costo total corre a cargo de los desarrolladores de modelos básicos (o de frontera) de IA como el GPT-4o de OpenAI. Los desarrolladores de modelos de IA también deben asegúrese de que haya una fuente de energía limpia para estos centros de datos, ya que las cargas de trabajo intensas de IA consumen mucha energía.
La última orden sigue a la de Biden de octubre de 2023 orden ejecutiva Establecer barreras protectoras para potentes modelos de IA de frontera o básicos. Incluye garantizar que el gobierno evalúe los sistemas de inteligencia artificial antes de implementarlos en áreas como la ciberseguridad y otros riesgos de seguridad nacional.
Biden también prometido desarrollar mecanismos de etiquetado y procedencia del contenido, para que los consumidores puedan saber qué contenido está generado por IA. Trump emitió la primera orden ejecutiva sobre IA en 2020, pidiendo su uso en el gobierno federal. Diferentes estados (California, Texas y otros) también tienen sus propias reglas de IA.
Regulaciones del Reino Unido y la UE
Las regulaciones de IA difieren en los EE. UU. de las del Reino Unido y Europa. La Ley de IA de la UE es mucho más amplia legislación que evalúa las aplicaciones de IA en función de tres niveles de riesgo: riesgo inaceptable (similar a la puntuación de individuos administrada por el gobierno según su posición social), alto riesgo (herramientas de IA para escanear currículums que rangos solicitantes de empleo) y que no es prohibido o considerado de alto riesgo.
El primer ministro británico, Keir Starmer anunciado el lunes (13 de enero) se presentó un plan de acción para convertir a Gran Bretaña en un líder en IA, incluida la ampliación de la capacidad de su centro de datos para cargas de trabajo de IA. Starmer dijo que se avecinan regulaciones formales de IA. Su predecesor Rishi Sunak desvelado un marco regulatorio de IA para que lo sigan los reguladores existentes.
Controles de exportación de chips de IA
Biden también expandido en su chip AI 2022 y 2023 controles de exportación destinado a evitar que China y otras naciones adversarias tengan en sus manos hardware de IA. Las nuevas regulaciones de esta semana dividen al mundo en grupos de ricos y pobres: 18 aliados y socios no tendrán ninguna restricción en absolutomientras que los compradores de pedidos de chips más pequeños de hasta 1.700 GPU avanzadas en potencia computacional también obtienen luz verde. Por lo general, se trata de universidades y organizaciones de investigación.
Sin embargo, más de 120 otros países Según se informa, se enfrentan a nuevas reglas a la hora de establecer instalaciones informáticas de IA. Las entidades confiables incluyen aquellas con sede en países que son aliados cercanos de Estados Unidos y que no tienen su sede en un “país de preocupación”. Aquellos que no tengan su sede en países aliados aún pueden comprar hasta 50.000 GPU avanzadas por país. Biden también estableció reglas que mantendrían secreto los pesos de un modelo de IA de entidades que no son de confianza, entre otros controles de seguridad.
Nvidia critica los límites de los chips
Se espera que las reglas afecten a Nvidia, cuyos chips GPU han sido el silicio elegido para el entrenamiento y la inferencia de modelos de IA. La empresa tiene un cuota de mercado fijado en más del 80%.
Nvidia se posicionó para una revolución de la IA en 2006; Sus GPU se desarrollaron inicialmente para manejar juegos y otras aplicaciones con uso intensivo de gráficos. El cofundador y director ejecutivo, Jensen Huang, apostó el futuro de la empresa al giro hacia la IA, a pesar de que los avances en la IA se han estancado. en el pasado los llamados “inviernos de IA”.
Ned Finkle, vicepresidente de asuntos gubernamentales de Nvidia, condenó las nuevas reglas de Biden. Escribió en un blog que el avance de la IA a nivel mundial está “ahora en peligro”. Dijo que la política “equivocada” de Biden “amenaza con descarrilar la innovación y el crecimiento económico en todo el mundo”.
Finkle calificó las reglas ampliadas de control de exportaciones de Biden como un “marañón regulatorio de más de 200 páginas, redactado en secreto y sin una revisión legislativa adecuada”. Tal acción regulatoria “amenaza con malgastar La ventaja tecnológica que Estados Unidos ha logrado con tanto esfuerzo”, añadió.
Finkle elogió a la primera administración Trump por fomentar un entorno de innovación en inteligencia artificial y dijo que “esperaba con ansias” volver a sus políticas mientras el expresidente se prepara para prestar juramento.
La Asociación de la Industria de Semiconductores intervino con su propio declaración. “Estamos profundamente decepcionados de que un cambio de política de esta magnitud e impacto se esté implementando rápidamente días antes de una transición presidencial. y sin ningún aporte significativo de la industria”.
El plan de OpenAI para EE. UU.
Cuando el director ejecutivo de OpenAI, Sam Altman, señaló sus planes de asistir a la toma de posesión del presidente Trump, su startup de inteligencia artificial lanzó de manera preventiva un plan para mantener a Estados Unidos a la vanguardia del desarrollo de la inteligencia artificial.
“Creemos que Estados Unidos debe actuar ahora para maximizar IA posibilidades y minimizando sus daños. La IA es una tecnología demasiado poderosa para ser dirigida y moldeada por autócratas, pero ese es el riesgo creciente que enfrentamosmientras la oportunidad económica que presenta la IA es demasiado convincente para perderla”, según OpenAI “IA en Estados Unidos” plan económico.
La visión de OpenAI depende de las siguientes creencias:
- Chips, datos, energía. y El talento es clave para ganar la carrera de la IA. Estados Unidos debe ser un mercado atractivo para las inversiones en IA, con 175 mil millones de dólares en fondos globales esperando ser desplegados. De lo contrario, estos se destinarán a proyectos respaldados por China.
- Un mercado libre que promueva la competencia libre y justa para impulsar la innovación. Esto incluye libertad para que los desarrolladores y usuarios trabajen con herramientas de IA mientras siguen estándares claros y de sentido común para mantener la IA segura para todos. El gobierno no debe utilizar estas herramientas para acumular poder y controlar a los ciudadanos.
- Para garantizar la seguridad del modelo fronterizo, el gobierno federal debe desarrollar mejores prácticas para prevenir contra mal uso; limitar la exportación de modelos fronterizos a naciones adversarias; desarrollar alternativas al mosaico de regulaciones estatales e internacionales, como una coalición internacional liderada por Estados Unidos.
- tEl gobierno federal debería compartir su experiencia sobre cómo proteger la propiedad intelectual de los desarrolladores de IA contra amenazas y ayudar a las empresas a acceder a infraestructuras seguras, como grupos informáticos clasificados. evaluar riesgos y salvaguardas del modelo, crean una vía voluntaria para que las empresas que desarrollan grandes modelos lingüísticos trabajen con el gobierno para definir evaluaciones de modelos, probar modelos e intercambiar información para respaldar las salvaguardas de las empresas.
- Si bien el gobierno federal debería liderar los asuntos de IA relacionados con la seguridad nacional, los estados pueden actuar para maximizar los beneficios de la IA para su propio ecosistema de IA. Pueden mejorar la experimentación con IA apoyando a nuevas empresas y empresas de IA más pequeñas. a encontrar Maneras de resolver los problemas cotidianos.
You may like
Noticias
¿Qué significa Sun Sextile Júpiter para su signo del zodiaco?
Published
42 minutos agoon
6 abril, 2025
¡Prepárate para montar la ola de suerte y expansión!
El 6 de abril, mientras transmite el signo audaz y ardiente de Aries, el Sol se reunirá con Júpiter en Géminis en un afortunado sextil, creando una oportunidad emocionante para el crecimiento, la prosperidad y los nuevos y audaces comienzos.
Si ha estado ansiando algo nuevo y emocionante, este tránsito podría ser la luz verde que ha estado esperando. Es el momento perfecto para arriesgarse, expandir sus horizontes y celebrar hitos.
El Sol en Aries tiene que ver con la acción, el coraje y el liderazgo. Como el primer signo en el zodiaco, Aries encarna una chispa de iniciación, al igual que su gobernante planetario, Marte. Entonces, con el sol viajando a través de este intrépido signo de fuego, es hora de avanzar y adoptar desafíos con confianza y coraje.
Júpiter, por otro lado, es el planeta de abundancia, optimismo y expansión. En el signo cerebral de Géminis (curiosidad, comunicación y adaptabilidad, Júpiter abre un mundo de posibilidades. Amplifica la necesidad de exploración intelectual, nuevas ideas y conexiones sociales.
Este tránsito nos invita a ampliar nuestras perspectivas, pensar fuera de la caja y sumergirnos en nuevas aventuras que expanden nuestras vidas personales y profesionales.
El sextil del sol a Júpiter el 6 de abril es una poderosa combinación de pasión ardiente y expansión intelectual. Es un momento en que las oportunidades de crecimiento y exploración se sienten abundantes, y el cosmos recompensa a aquellos que están dispuestos a correr riesgos y adoptar el cambio.
Ya sea que esté comenzando un nuevo proyecto, tomando una gran decisión o sentirse inspirado para hacer algo nuevo, este tránsito ofrece el potencial de prosperidad y abundancia.
Siga leyendo para lo que esto significa para su signo del zodiaco.
Aries (del 20 de marzo al 19 de abril)
¡Eres la estrella del espectáculo, Aries! Además de que es su temporada de regreso solar, con el sol encendido por su primera casa, está uniendo fuerzas con Lucky Júpiter … y bueno, ¡eres imparable! Este es el momento perfecto para lanzar un proyecto personal o renovar su imagen. Considere actualizar su marca o presencia en las redes sociales para que coincida con su energía. Este tránsito se trata de ti, poseerlo y brillar.
Tauro (del 19 de abril del 20 de mayo)
Este es un momento para la reflexión y el crecimiento espiritual, Tauro. Aries gobierna su introspectiva casa 12 de patrones subconscientes y la iluminación de Júpiter en su segunda casa de finanzas y valores, lo que le brinda la claridad de liberarse de los viejos patrones mentales que ya no le sirven. Tal vez es hora de dejar de lado esos sistemas de creencias limitantes en torno al dinero o su autoestima. Confíe en que las nuevas y prósperas oportunidades esperan una vez que lo haga.
Géminis (del 20 de mayo al 20 de junio)
¡Es tu día de suerte, Géminis! A medida que el Sol energiza a su 11ª Casa de Asuntos Comunitarios, Júpiter aporta expansión y oportunidad a su letrero (¡y en la puerta de entrada!), Lo que lo convierte en un excelente momento para conectarse con personas influyentes y aquellos que comparten objetivos y sueños similares. Una oportunidad de establecer contactos podría llegar en su camino, o podría unirse inesperadamente a un grupo que se alinee perfectamente con sus valores.
Cáncer (del 20 de junio al 22 de julio)
Alcance las estrellas: su carrera está bajo el centro de atención, el cáncer. A medida que el Sol energiza y revitaliza su décima casa de autoridad pública, Lucky Júpiter lo hace más receptivo y sintonizado con su crecimiento personal y profesional. Esto no solo ofrece ideas espirituales, sino que también te empuja a hacer movimientos audaces en tu vida profesional. El éxito está en el horizonte.
Leo (22 de julio al 22 de agosto)
¡La aventura te espera, Leo! El sol está gobernado por el sol, y mientras enciende su novena casa de expansión filosófica, unirá fuerzas con Lucky Júpiter en su 11ª Casa de Asociaciones, Asuntos Comunitarios y visiones futuras. Ya sea que se trate de un viaje de último minuto, una clase en la que se está inscribiendo o un pasatiempo nuevo que está explorando, su mente y su corazón están abiertos a nuevas experiencias. Carpe Diem.
Virgo (22 de agosto al 22 de septiembre)
Este es un gran problema: confía en que la transformación que está experimentando es para su más alto bien, Virgo. Con el sol sacudiendo su octava casa de intimidad y recursos compartidos, se reunirá con Lucky Júpiter en su décima casa de carrera y reputación pública. Una ganancia inesperada financiera o un profundo avance emocional podría estar en camino, ayudándole a entrar en su poder personal. Estar abierto a lo inesperado.
Libra (del 22 de septiembre al 22 de octubre)
Sus asociaciones y acuerdos contractuales están bajo el foco de este tránsito, Libra. A medida que el sol energiza su sector de relaciones, se reunirá con Audacy Júpiter en un sextil armonioso. Ya sea amor, negocios o amistades, una nueva conexión podría sentirse destinada. Es un buen momento para trabajar con otros en empresas conjuntas o colaboraciones que contribuyen a su crecimiento personal y profesional.
Scorpio (22 de octubre a Nov. 21)
Sus hábitos de salud pueden mejorar drásticamente bajo esta sinergia empoderadora, Scorpio. Si bien el trabajo y los asuntos de salud están a la vanguardia, el sextil de Sun a Júpiter, activando su sexta casa de bienestar y octava casa de empresas conjuntas, podría inspirarlo con la confianza y la energía que necesita para asumir nuevos desafíos. Tal vez es hora de sacudir su rutina o asumir un nuevo objetivo de salud. Una nueva oportunidad de trabajo o reconocimiento podría llegar a su manera, haciendo que sus esfuerzos se sientan más gratificantes.
Sagitario (22 de noviembre al déco de 21)
Sus jugos creativos fluyen, y eso es un eufemismo, Sagitario. Después de todo, no es todos los días que su gobernante planetario de la suerte, Júpiter, une fuerzas con el sol en su quinta casa de amor, pasión y autoexpresión. Aplastar por alguien especial? Ya sea que esté trabajando en un proyecto de pasión, atrapando sentimientos románticos o entrando en el centro de atención con un esfuerzo creativo, su aura está radiante y está listo para brillar. El amor también podría ser espontáneo y emocionante.
Capricornio (del 21 de diciembre al 19 de enero)
El hogar es donde está tu corazón, entonces, ¿por qué no darle el amor que merece, Capricornio? Con el sol que abarca su cuarta casa doméstica del hogar y la familia, mientras que en el flujo de energía armonioso con Júpiter en su sexta casa de mejora, logística y responsabilidad, puede sentirse llamado a mejorar su espacio vital o crear más espacio para sus pertenencias. Tal vez es hora de redecorar, mudarse a un nuevo espacio o incluso fortalecer los lazos familiares.
Acuario (19 de enero de 18 años)
Usa tus palabras sabiamente: son más poderosos de lo que te das cuenta, Acuario. Con el sol energizando su curiosa tercera casa de comunicación, sus pensamientos e intercambios serán clave durante este tiempo. Aún así, a medida que el Sol se armoniza con Júpiter en su quinta casa de fertilidad y expresión creativa, eres igualmente inspirador y seguro en tu enfoque. Es posible que tenga una conversación esclarecedora que lleva su relación al siguiente nivel o provoca una nueva idea para un proyecto.
Piscis (del 18 de febrero al 20 de marzo)
¡El dinero fluye y la abundancia está llamando, Piscis! A medida que el sol energiza su segunda casa de comodidad, finanzas y valores que busca la estabilidad, se reunirá con Júpiter en su cuarta casa del hogar, la familia y los lazos emocionales. ¿Listo para hacer esa inversión en su espacio vital? Otros podían sentirse llamados para gastar un poco de efectivo extra en una excursión familiar. Confía en tu intuición: la prosperidad podría venir de manera sorprendente.
Noticias
¿Cuál fue el misterioso asistente de Pixie del Pixel 9?
Published
2 horas agoon
6 abril, 2025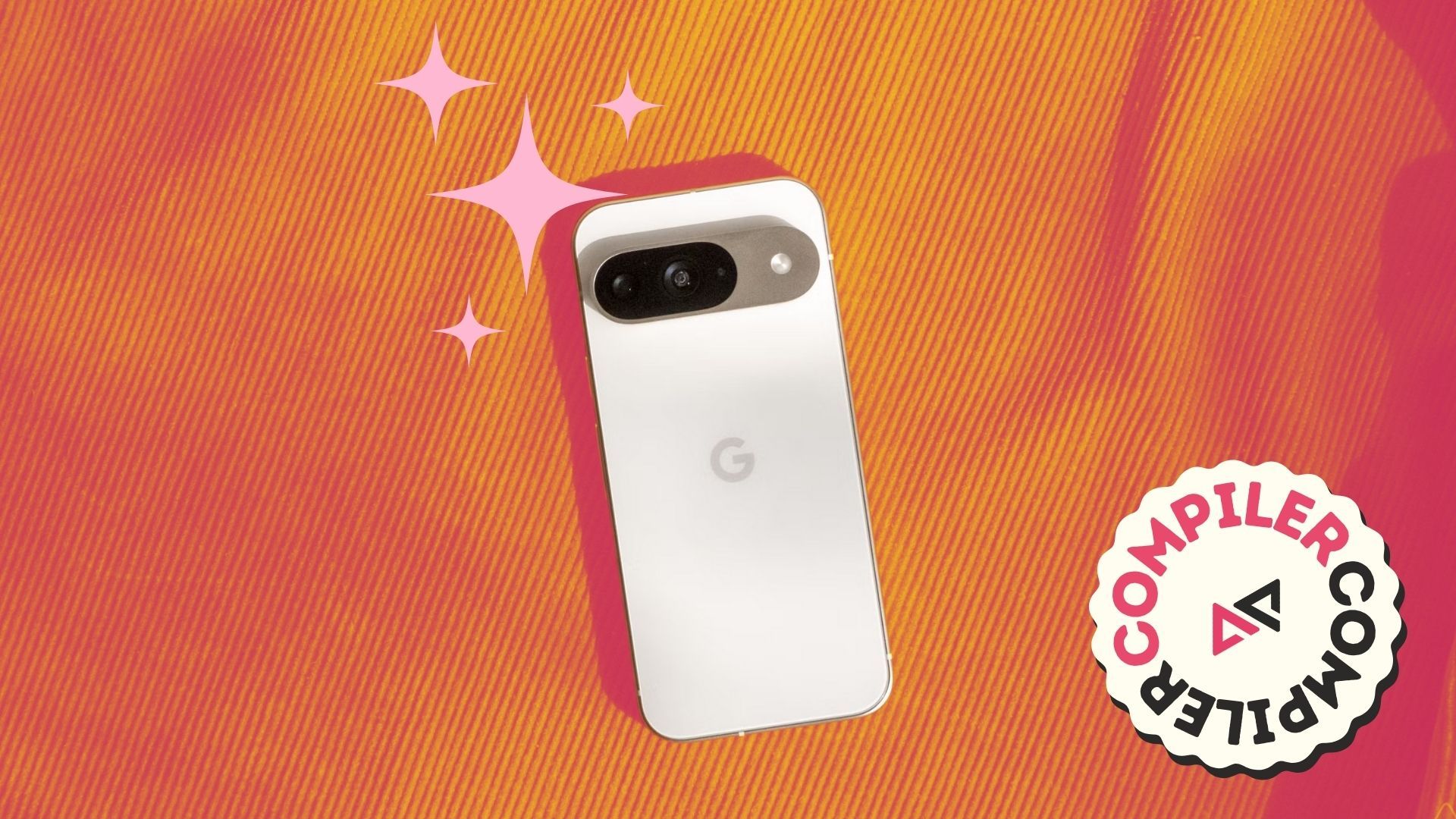
A finales de 2023, escuchamos que Google estaba trabajando en un nuevo asistente digital que evidentemente se planeó debutar en el Pixel 9 en 2024. Evidentemente llamado Pixie, el asistente habría manejado tareas de IA en el dispositivo usando un modelo de Gemini Nano. Pero la IA nunca se materializó. Entonces, ¿qué pasó con Pixie, y lo volveremos a ver?
Bienvenido al compiladorsu resumen semanal de Goings-On. Paso mis días mientras el editor de Google leyendo y escribiendo sobre lo que Google está haciendo a través de Android, Pixel, Gemini y más, y hablo de todo aquí en esta columna. Esto es lo que ha estado en mi mente esta semana.
¿Qué era Pixie?
Según los informes de la información, Pixie estaba destinado a ser un asistente de IA en el dispositivo exclusivo de los teléfonos de Google Pixel, concebido antes de que el chatbot Gemini llegara a la escena (todavía era Google Bard en aquel entonces). Usando un modelo local de Géminis Nano, la IA habría extraído datos de las aplicaciones de Google en su teléfono para ofrecer asistencia más personalizada. La información dijo en 2023 que Pixie podría haber evolucionado “a una versión mucho más personalizada del Asistente de Google”.
Aparentemente, Pixie estaba planeado para asumir las tareas del asistente del Asistente de Google en los teléfonos de Google. Obviamente, eso nunca sucedió, aunque Google ha anunciado oficialmente sus planes para eliminar gradualmente al Asistente Legacy a favor de Gemini en el futuro cercano.
Relacionado
Géminis está reemplazando oficialmente al Asistente de Google, esto es lo que eso significa para ti
Google Assistant se está jubilando este año
¿Qué pasó con Pixie?
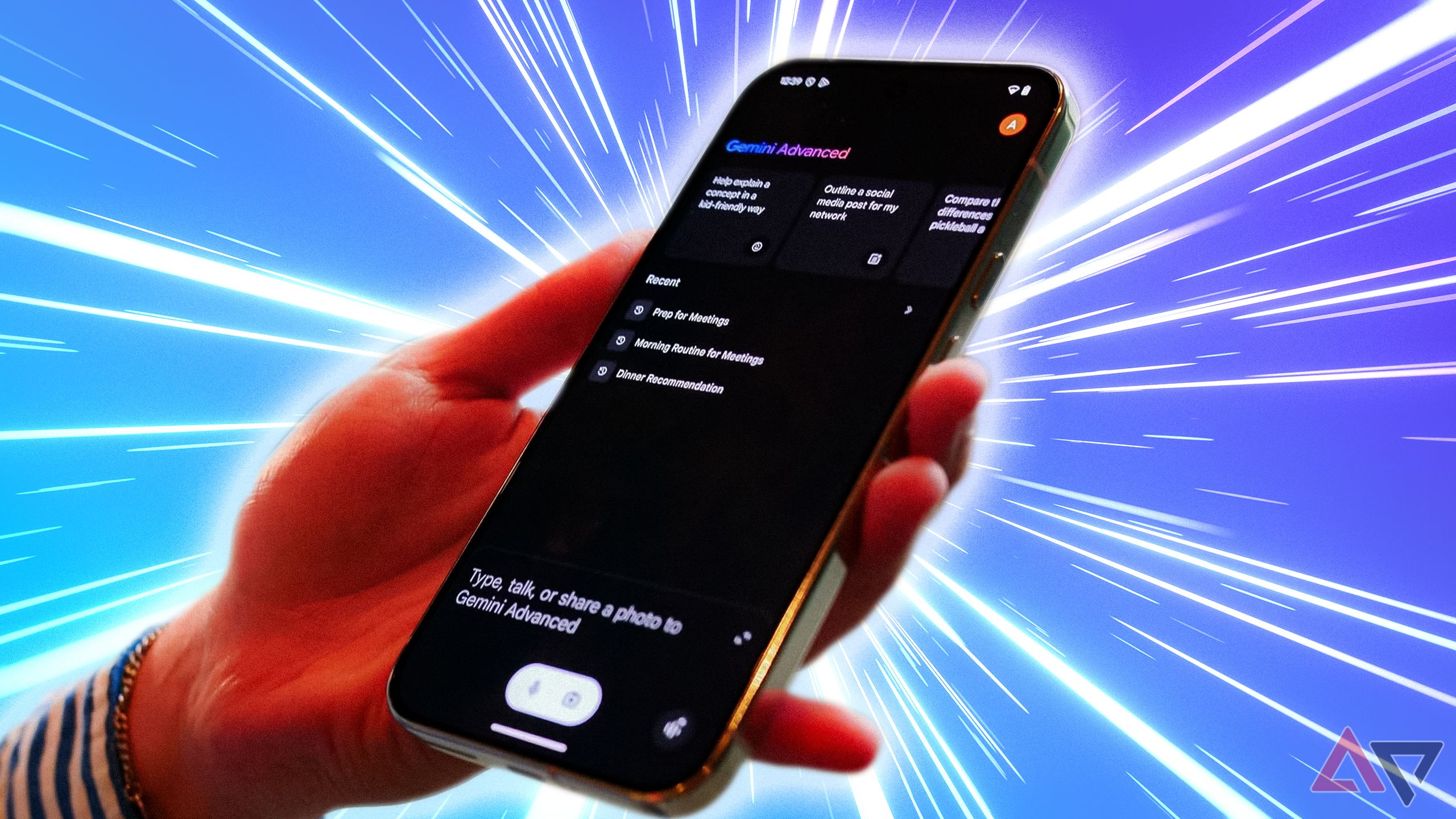
La serie Pixel 9 aterrizó el año pasado sin mencionar el asistente de Pixie que se rumoreaba el año anterior. El informe posterior de la información (llamado a nuestra atención por 9to5Google) arroja algo de luz sobre lo que pudo haberle sucedido a Pixie.
Según los informes, el CEO de Google, Sundar Pichai, ordenó personalmente un cambio en la estrategia con Pixie para evitar la competencia con el asistente de IA prioritario de Google, Gemini. La marca Pixie parece estar bien y verdaderamente retirada, pero algunas características de Pixie terminaron llegando al Pixel 9. Capturas de pantalla de Pixel, una característica de IA local impulsada por el modelo Gemini Nano XS, evidentemente comenzó su vida como funcionalidad de Pixie. 9to5Google también ha informado que la extensión de servicios públicos de Gemini que permite a la IA controlar directamente la configuración del dispositivo, la reproducción de medios, las alarmas y más, al mismo tiempo, se planeó ser parte de la experiencia de Pixie.
La información ha informado que estas características aparentemente salieron de Pixie no hacen una experiencia tan similar a lo que Pixie habría sido. Sin embargo, es posible que veamos más de lo que Pixie podría haber sido en el futuro.
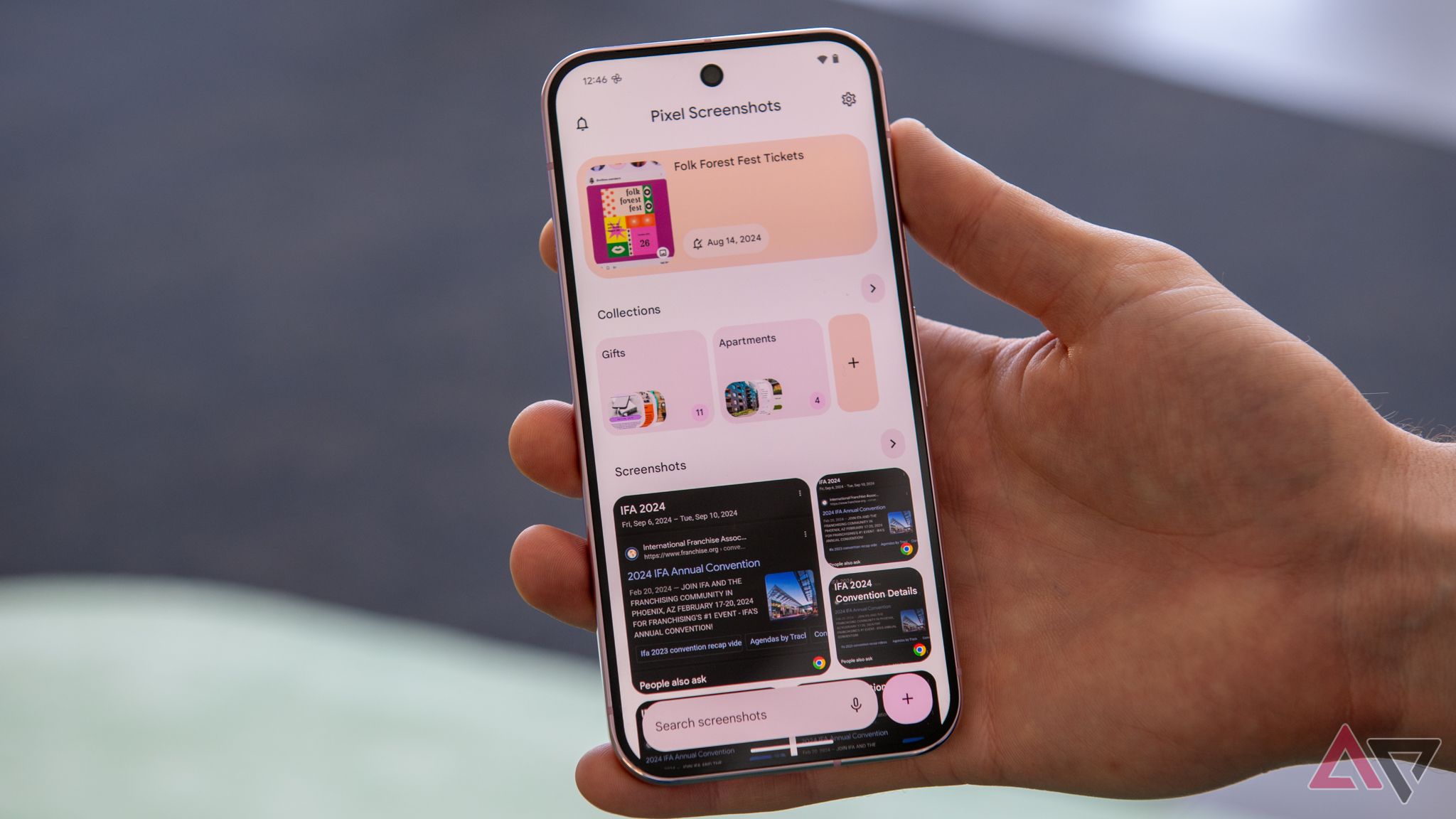
Relacionado
La pequeña aplicación de capturas de pantalla de Pixel de Google finalmente me convenció de que AI podría ser un gran problema
Las capturas de pantalla de Pixel pueden ser la forma más clara de Google de demostrar que la IA es útil
¿Podría Pixie regresar?
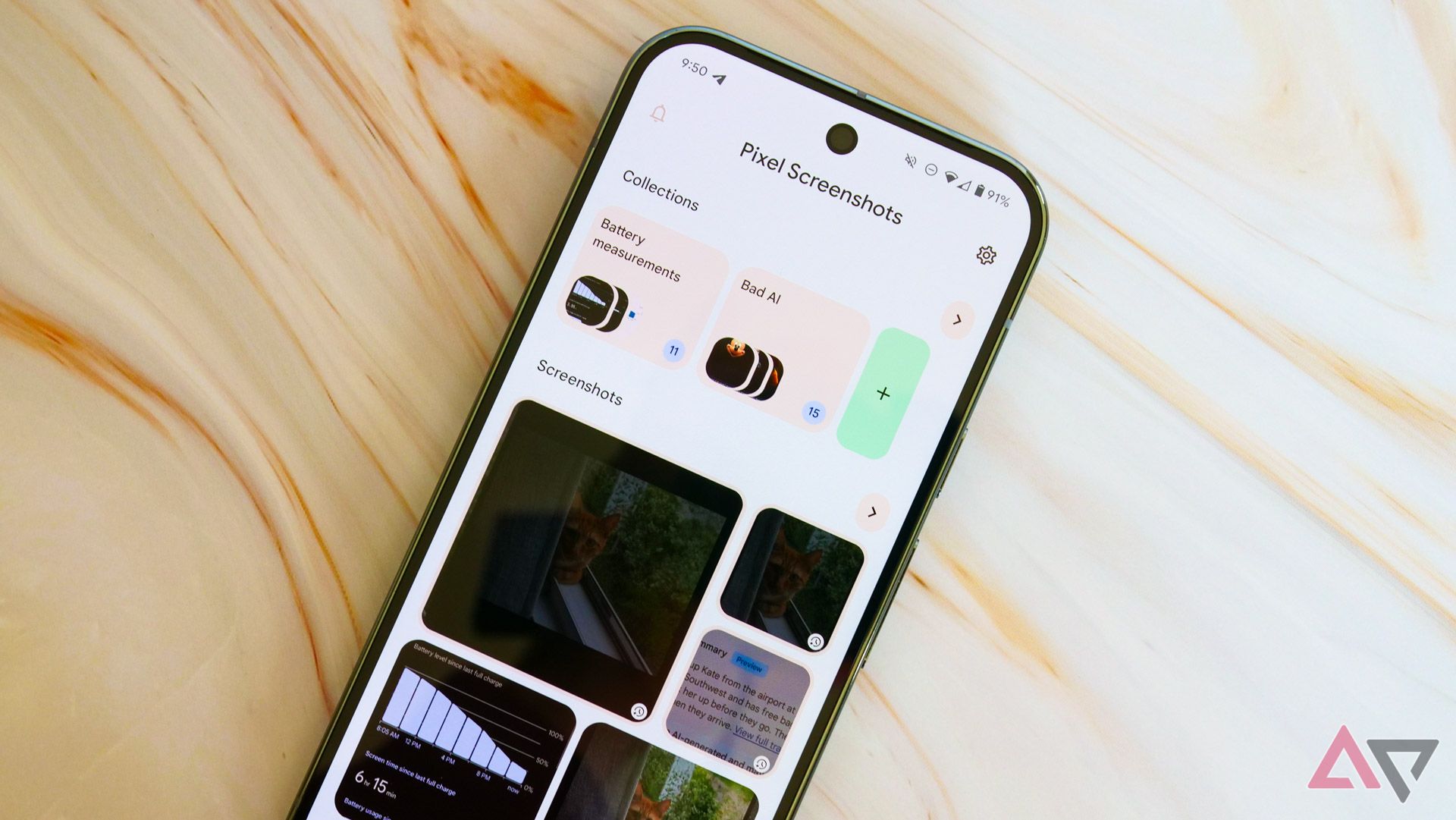
Con Gemini tan claramente una prioridad para Google, parece poco probable que veamos el lanzamiento de un asistente de inteligencia artificial exclusivo de píxeles que se superpone con el conjunto de funciones de Gemini; de hecho, con Gemini listo para reemplazar el Asistente de Google en la mayoría de los dispositivos en el futuro cercano, Google parece que va en la dirección opuesta. Pero un informe de marzo de Android Authority que hace referencia a “Una fuente dentro de Google” dice que más funcionalidad de Pixie llegará a la serie Pixel 10 en forma de una nueva aplicación llamada Pixel Sense.
Parece que Pixel Sense no competirá directamente con Gemini, sino que intentará proporcionar sugerencias “predictivas” basadas en el contexto de sus aplicaciones de Google conectadas, incluidos Calendar, Chrome, Gmail, Keep, Maps y más. Pixel Sense aparentemente también podrá organizar sus capturas de pantalla en un archivo de búsqueda como lo hace la aplicación de capturas de pantalla Pixel actual, insinuando que puede reemplazar las capturas de pantalla por completo.
Pixel Sense funcionará completamente en el dispositivo; Android Authority cita una fuente diciendo que “sus datos permanecen privados, visibles solo para usted, ni siquiera Google puede verlo”.
Los informes de AA no pintan una imagen completa de cómo funcionará realmente la aplicación Pixel Sense, pero como se describe, parece que podría funcionar de manera similar a la de Google Now, o la función S25 similar de Samsung, ahora breve. Esas características también tienen como objetivo proporcionar información según lo necesite, informado por el contexto de sus cuentas conectadas. Con el acceso a lo que parece esencialmente toda la información almacenada en su cuenta de Google y alimentada por Gemini Nano, Pixel Sense podría hacer un mejor trabajo para ofrecer actualizaciones útiles a medida que las necesita.
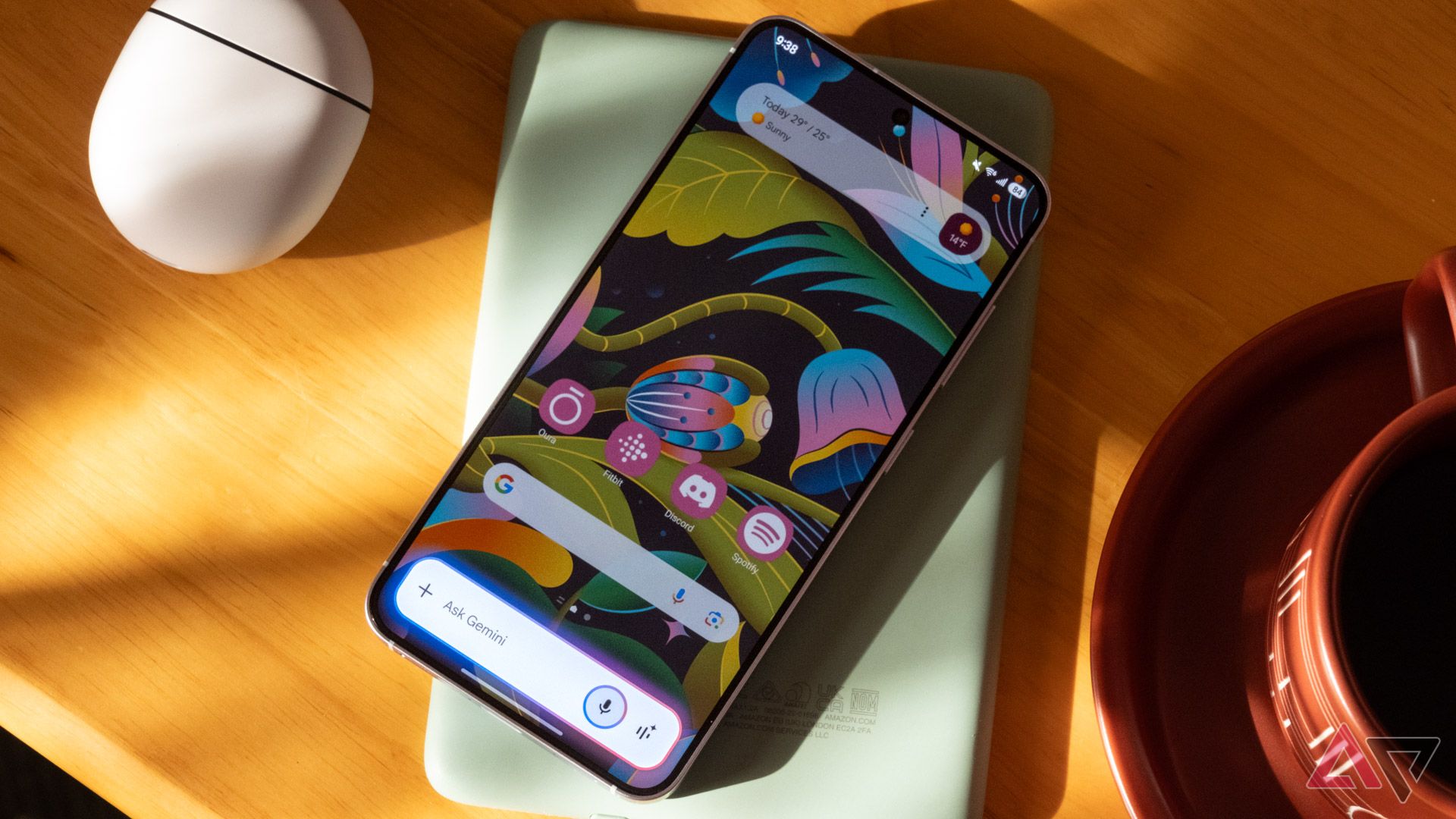
Relacionado
Revisión: El Samsung Galaxy S25 es un teléfono pequeño sin grandes ideas
Todavía bastante agradable, aunque
Géminis es tu asistente de IA de Android para lo previsible
El asistente de Pixie probablemente nunca verá la luz del día en su forma inicialmente planificada; Google está poniendo todos sus huevos de asistente en la canasta de Géminis. Pero parece que obtendremos más de la funcionalidad de Pixie en la serie Pixel 10 en forma de la nueva aplicación Pixel Sense. En cuanto a qué es exactamente el sentido de Pixel, tendremos que esperar y ver. Es posible que escuchemos más en Google I/O, que comienza el próximo mes.
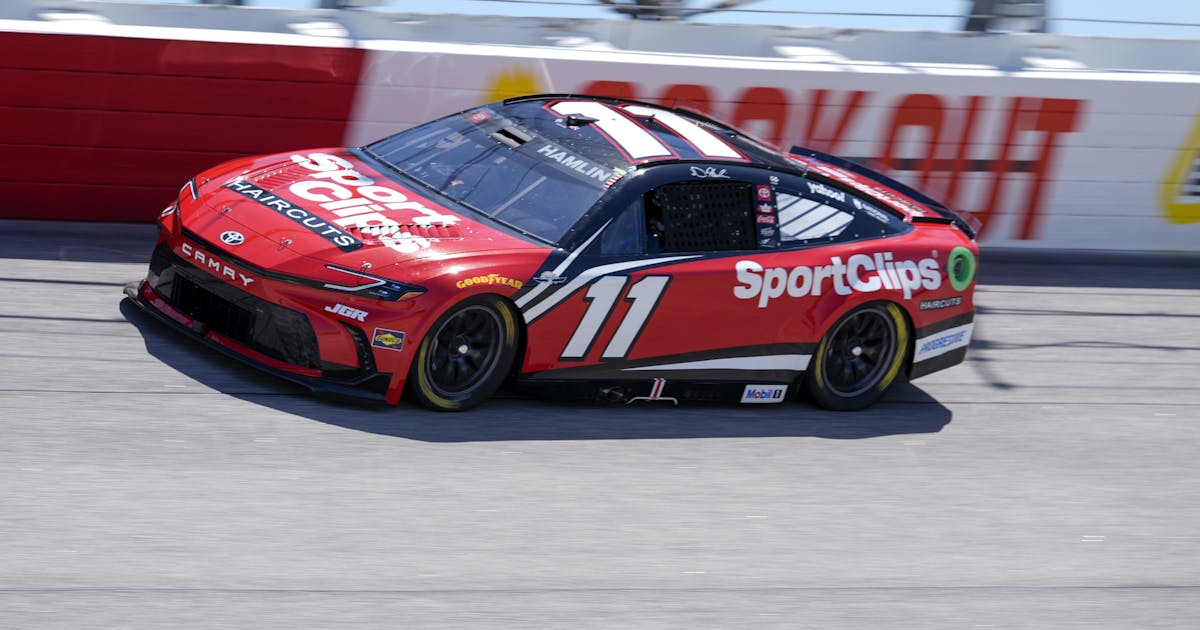
After correctly predicting Denny Hamlin to win his first race of the NASCAR Cup Series season, we’re turning to AI to help us predict the winner and full finishing order for the Goodyear 400 at Darlington Raceway today at 3 p.m. ET (FS1).
We asked ChatGPT for its NASCAR at Darlington predictions based on historical data, betting odds, and statistical trends – including its pick to win, best prop bet, and favorite long shot, as well as the results for every driver for today’s 38-car field.
Along with our 2025 Goodyear 400 predictions at Darlington, here are our AI-powered NASCAR best bets and full AI-simulated finishing order:
NASCAR AI picks & predictions for Goodyear 400 at Darlington
We’ve previously used ChatGPT to predict its March Madness bracket, Super Bowl picks, and even its Canada vs. USA predictions, and we’re once again turning to OpenAI’s popular chatbot to predict the winner of today’s Goodyear 400 at Darlington.
We trained ChatGPT’s latest and most advanced AI model to study the latest NASCAR odds, betting history, and relevant trends before predicting this weekend’s winner:
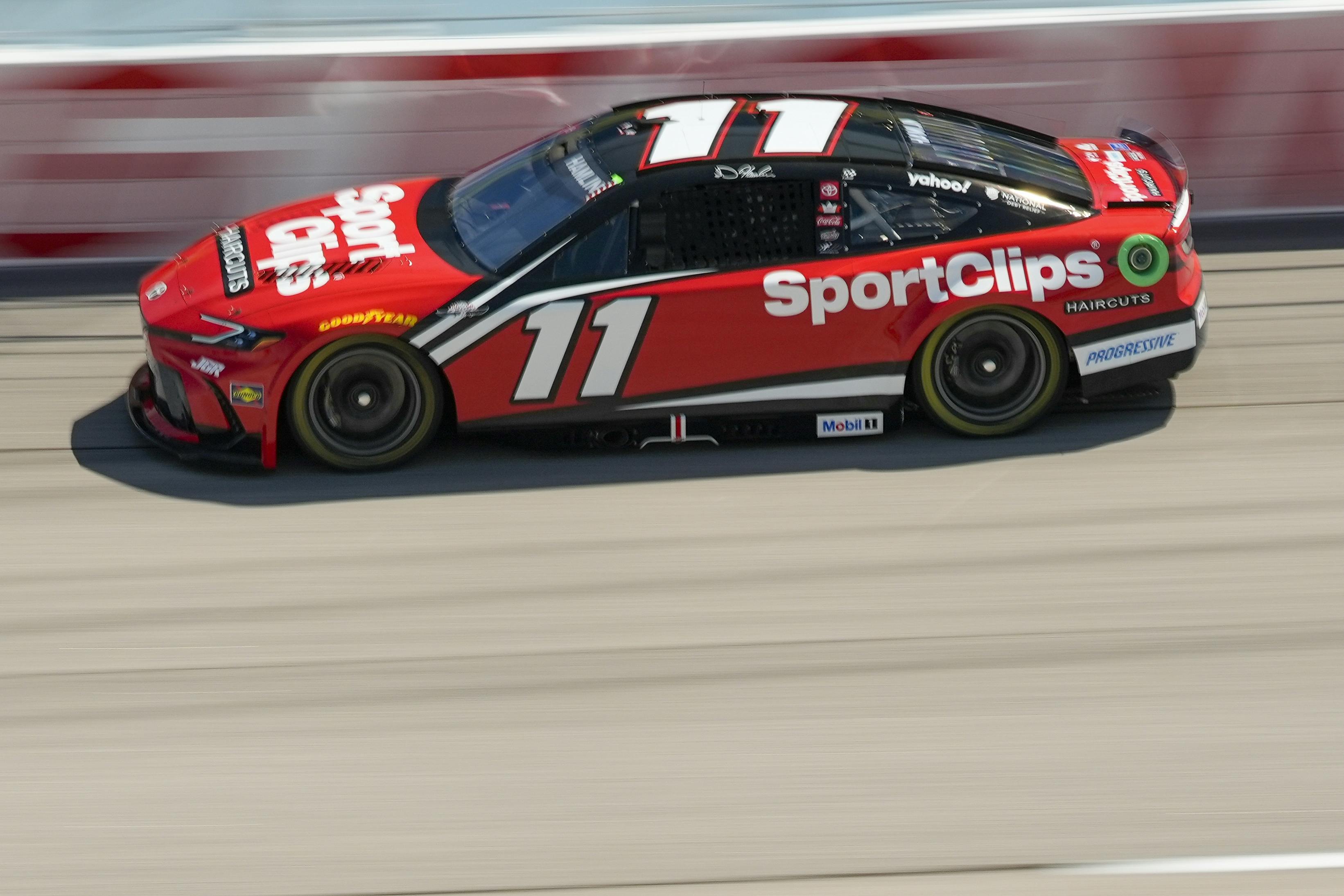
ChatGPT’s pick to win Goodyear 400 at Darlington
ChatGPT predicts Denny Hamlin will win the Goodyear 400 at Darlington. His best odds are +800 via BetMGM, which would turn a winning $10 bet into an $80 profit with an implied win probability of 11.11%.
Here’s why the AI model is predicting Hamlin will win today’s race:
Short-track savvy: Hamlin’s long history on short, punishing tracks gives him the experience needed to navigate Darlington’s notorious “Track Too Tough to Tame,” avoiding the mishaps and attrition that often plague less experienced drivers.
Team excellence & strategy: Joe Gibbs Racing consistently fields strong cars at Darlington, and Hamlin’s ability to manage cautions, fuel strategy, and late-race restarts is a big advantage on this challenging circuit.
Proven consistency: Despite the inherent chaos of Darlington, Hamlin’s track record as a veteran who can execute under pressure makes him a standout in a field where even the favorites have slim win probabilities.
AI confidence level:
(15% win probability)
Up To $1,500 First Bet
Bonus bets will expire in 7 days. One new customer offer only. Additional terms and conditions apply.
Each product featured on our website has been meticulously researched and selected by our team of experts. If you sign up through our links, we may get a commission. 21+ and present in AZ. Gambling Problem? Call 1-800-NEXT-STEP or text NEXTSTEP to 53342.
ChatGPT’s best prop bet for Goodyear 400 at Darlington
ChatGPT predicts Chase Briscoe will finish in the top three as its best NASCAR prop bet for Darlington. Briscoe’s best odds to score a top-3 finish are +550 via bet365, which would turn a winning $10 bet into a $55 profit with an implied win probability of 15.38%.
Here’s why the AI model is predicting Briscoe to finish in the top three today:
Strong starting position & package: Briscoe qualified 4th with Joe Gibbs Racing – a team known for building competitive cars at Darlington – and his starting position puts him right in the mix.
Experience on tough tracks: JGR drivers have a good track record at Darlington, and Briscoe’s aggressive yet measured driving style makes him well-suited to navigate Darlington’s unique challenges.
Ability to capitalize on caution-fueled restarts: Darlington is notorious for its unpredictable cautions and restarts. If the leaders are caught up in strategy or on-track incidents, Briscoe’s aptitude for seizing opportunities could see him climbing into the top three.
AI confidence level:
(25% chance of top-3 finish)
Bet $5 and Get $150 in Bonus Bets Win or Lose*
*Deposit required. Bonus Bets wager excluded from returns. T&Cs, time limits and exclusions apply. Gambling problem? Call 1-800-NEXT-STEP
Each product featured on our website has been meticulously researched and selected by our team of experts. If you sign up through our links, we may get a commission. 21+ and present in AZ. Gambling Problem? Call 1-800-NEXT-STEP or text NEXTSTEP to 53342.
ChatGPT’s best long shot to win Goodyear 400 at Darlington
ChatGPT predicts Alex Bowman to win the Goodyear 400 as its best long shot at Darlington. His best odds to win are +5000 via Caesars, which would turn a winning $10 bet into a $500 profit with an implied win probability of 1.96%.
Here’s why the AI model is predicting Bowman as its best long shot today:
Undervalued starting position vs. team quality: Although Bowman qualified 33rd, his Hendrick Motorsports car is typically one of the best on the circuit. A deep starting position can mask his true race pace if his team brings a strong setup for Darlington.
Opportunity in Darlington’s chaos: Darlington’s reputation for attrition, unpredictable cautions, and strategic gambles means that drivers starting deep can climb the order dramatically if they avoid early incidents.
Proven ability to overperform: Bowman has shown in past races that he can make up significant ground when conditions favor his driving style. His experience and skill could allow him to exploit a chaotic race and deliver a surprise win.
AI confidence level:
(4% win probability)
Bet $1, Get Ten 100% Profit Boosts
New users only. Must use promo code. Min. $10 deposit. Max. bonus is ten 100% Profit Boost tokens. Profit Boost tokens expire in 14 days. Void where prohibited. Full terms.
Each product featured on our website has been meticulously researched and selected by our team of experts. If you sign up through our links, we may get a commission. 21+ and present in AZ. Gambling Problem? Call 1-800-NEXT-STEP or text NEXTSTEP to 53342.
Goodyear 400 AI projections for full finishing order
Here is our predicted Goodyear 400 finishing order and results for every driver based on AI projections. While ChatGPT is a large language model and not specifically designed for predicting sporting events, it can spot trends or insights that NASCAR bettors might overlook.
ChatGPT’s full NASCAR finishing order predictions at Darlington
1. Denny Hamlin (No. 11, Joe Gibbs Racing Toyota) – Using his veteran short‐track savvy and ability to manage fuel and cautions, Hamlin pulls away to take the win.
2. William Byron (No. 24, Hendrick Motorsports Chevrolet) – Byron’s strong car and clean air from starting first help him finish near the front.
3. Chase Briscoe (No. 19, Joe Gibbs Racing Toyota) – A well-handled race by Briscoe capitalizing on strategic restarts secures him a podium finish.
4. Bubba Wallace (No. 23, 23XI Racing Toyota) – Wallace’s aggressive style suits Darlington’s unpredictable nature, keeping him in the top four.
5. Kyle Busch (No. 8, Richard Childress Racing Chevrolet) – Busch’s experience and ability to avoid trouble make him a steady presence in the top five.
6. Joey Logano (No. 22, Team Penske Ford) – Logano overcomes a deeper starting position with a series of strong restarts to climb into the top six.
7. Chase Elliott (No. 9, Hendrick Motorsports Chevrolet) – Despite a mid-pack start, Elliott’s racecraft and Hendrick’s setup allow him to finish strongly.
8. Tyler Reddick (No. 45, 23XI Racing Toyota) – Reddick’s speed and determination help him navigate the short-track mayhem for a top-10 finish.
9. Austin Cindric (No. 2, Team Penske Ford) – Cindric’s consistency and a smart pit strategy keep him in contention among the leaders.
10. Christopher Bell (No. 20, Joe Gibbs Racing Toyota) – Bell capitalizes on clean air and a well-timed move to round out the top 10.
11. Ryan Blaney (No. 12, Team Penske Ford) – Blaney stays in the mix and finishes solidly in the upper group.
12. Kyle Larson (No. 5, Hendrick Motorsports Chevrolet) – Larson’s talent sees him fighting through traffic for a top-12 finish.
13. Todd Gilliland (No. 34, Front Row Motorsports Ford) – In the midst of the short-track chaos, Gilliland manages to keep a respectable position.
14. Ryan Preece (No. 60, RFK Racing Ford) – Preece, starting near the front, is jostled around early and slips to 14th.
15. Michael McDowell (No. 71, Spire Motorsports Chevrolet) – McDowell’s early speed is tempered by the attrition typical of Darlington, landing him mid-pack.
16. Ty Gibbs (No. 54, Joe Gibbs Racing Toyota) – The young gun shows promise but finishes behind the veterans as the race unfolds.
17. Carson Hocevar (No. 77, Spire Motorsports Chevrolet) – Hocevar’s package keeps him in contention, but he ultimately settles in the lower mid-field.
18. Chris Buescher (No. 17, RFK Racing Ford) – Buescher’s RFK setup allows him to cruise steadily, finishing just outside the top 15.
19. Justin Haley (No. 7, Spire Motorsports Chevrolet) – Haley makes a late charge but is held back by traffic, finishing in the upper mid-field.
20. Ross Chastain (No. 1, Trackhouse Racing Chevrolet) – Chastain’s bold moves see him climb significantly – but contact and cautions slow his progress, putting him 20th.
21. Austin Dillon (No. 3, Richard Childress Racing Chevrolet) – Dillon’s car struggles with the track’s relentless demands, dropping him into the lower mid-field.
22. Josh Berry (No. 21, Wood Brothers Racing Ford) – Berry’s knack for close-quarters racing keeps him around the 20th–22nd range.
23. Brad Keselowski (No. 6, RFK Racing Ford) – Keselowski battles through on-track incidents to finish in the low 20s.
24. Zane Smith (No. 38, Front Row Motorsports Ford) – Smith’s less competitive package and a few missteps push him slightly back.
25. A.J. Allmendinger (No. 16, Kaulig Racing Chevrolet) – Allmendinger’s aggressive style yields mixed results, and he ends up mid-pack.
26. Noah Gragson (No. 4, Front Row Motorsports Ford) – Gragson is caught in the frequent Darlington cautions, finishing in the mid-field.
27. John Hunter Nemechek (No. 42, Legacy Motor Club Toyota) – Nemechek’s strategic driving helps him inch forward, but he remains in the lower mid-field.
28. Ricky Stenhouse Jr. (No. 47, Hyak Motorsports Chevrolet) – Stenhouse Jr. is involved in a couple of incidents, dropping him further back.
29. Ty Dillon (No. 10, Kaulig Racing Chevrolet) – With a modest package, Ty Dillon finishes in the latter part of the field.
30. Daniel Suarez (No. 99, Trackhouse Racing Chevrolet) – Suarez’s volatile style sees him struggle with consistency, landing him near the back.
31. Cole Custer (No. 41, Haas Factory Team Ford) – Custer’s car isn’t well-suited for Darlington, resulting in a lower-field finish.
32. Riley Herbst (No. 35, 23XI Racing Toyota) – Inexperience and a lack of track finesse see Herbst fade in the latter half.
33. Alex Bowman (No. 48, Hendrick Motorsports Chevrolet) – Bowman’s pace drops off amid the chaos, and he falls toward the back.
34. Erik Jones (No. 43, Legacy Motor Club Toyota) – Jones is hampered by on-track contact and ends up further down the order.
35. Cody Ware (No. 51, Rick Ware Racing Ford) – With one of the least competitive packages, Ware is forced into a deep back finish.
36. Shane van Gisbergen (No. 88, Trackhouse Racing Chevrolet) – The international star struggles to adapt to Darlington’s brutal demands and falls out of contention.
37. Austin Hill (No. 33, Richard Childress Racing Chevrolet) – Hill’s inexperience on this demanding track sends him near the rear.
38. J.J. Yeley (No. 44, NY Racing Team Chevrolet) – Yeley’s limited Cup experience sees him finish in the final stretch.
NASCAR best bets for Goodyear 400 at Darlington
Bet | Driver | Odds | Implied win probability |
---|---|---|---|
Denny Hamlin | +800 | 11.11% | |
Chase Briscoe (top-3) | +550 | 15.38% | |
Alex Bowman | +5000 | 1.96% |
How to watch the 2025 Goodyear 400 at Darlington
Race date: Sunday, April 6
Start time: 3 p.m. ET
Track: Darlington Raceway (Darlington, S.C.)
TV: FS1 | Streaming: Fox Sports App
Best NASCAR betting sites for Goodyear 400 at Darlington
Looking to bet on the Goodyear 400 at Darlington Raceway? Here are our top-rated NASCAR best sports betting sites as determined by our expert team at Sportsbook Review, along with our best sportsbook promos ahead of today’s race at 3 p.m. ET.
(21+. Gambling Problem? Call 1-800-GAMBLER)
* Bonuses not applicable in Ontario.
Not intended for use in MA.
Each betting site featured on SBR has been meticulously researched and selected by our team of experts. If you sign up through our links, we may get a commission.
Related posts
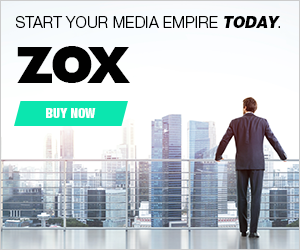
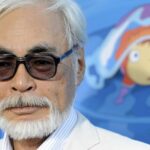
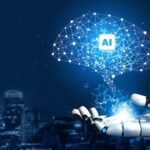
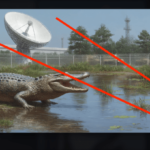
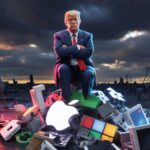
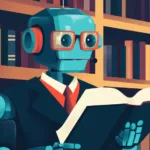
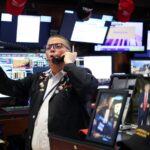
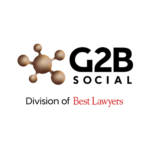
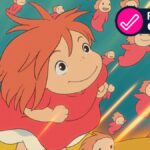
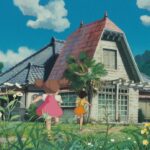
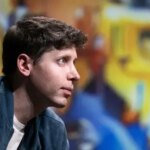
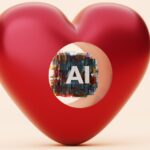
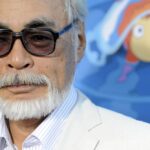
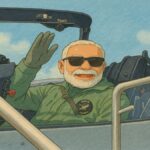
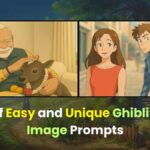
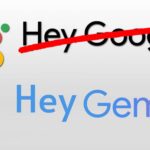
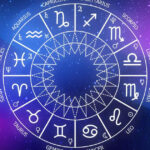
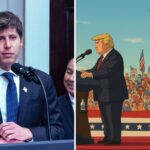
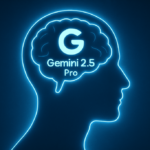
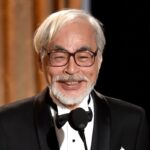
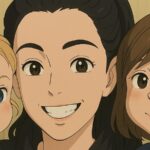
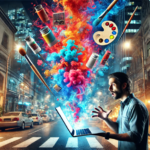
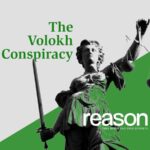
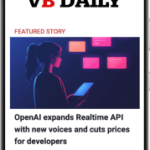
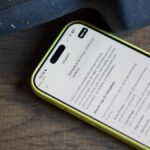
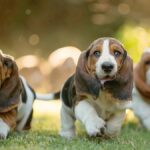
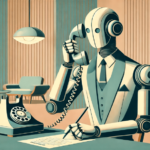
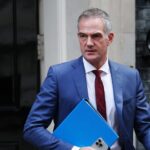
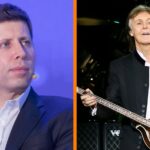
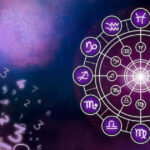
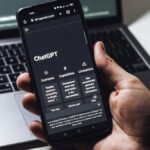
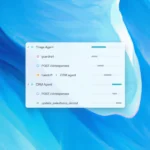
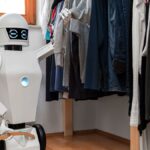
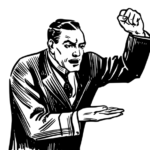
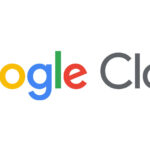
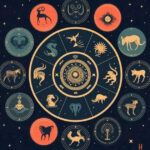
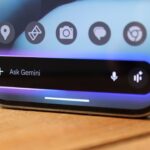
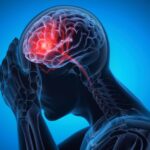
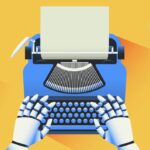
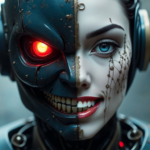
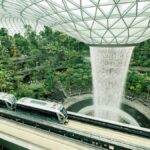
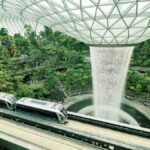
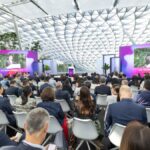
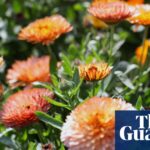

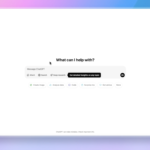
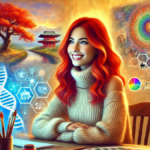
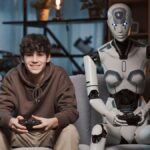
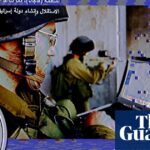
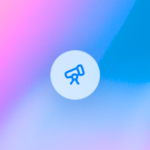
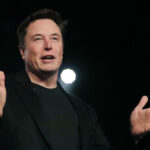
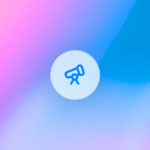
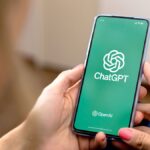
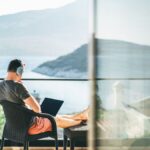
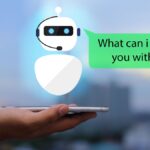
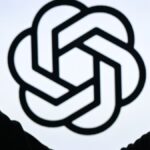
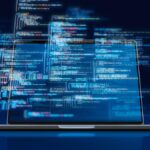
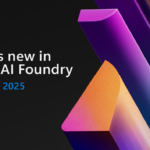
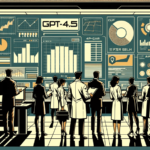
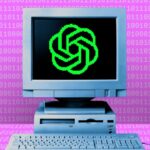
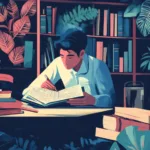
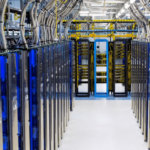
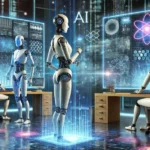
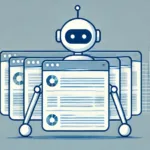
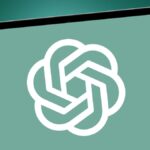
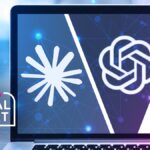
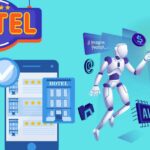
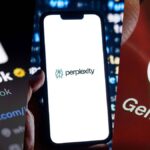
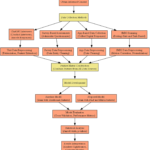
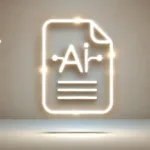
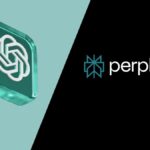
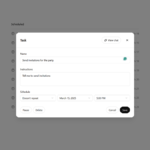
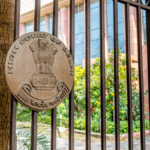
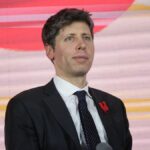
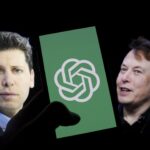
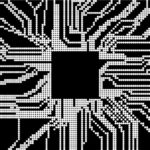
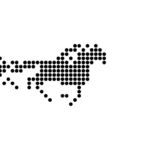
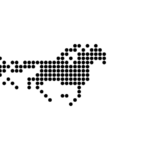
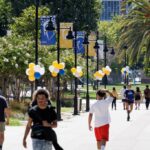
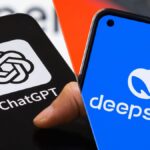
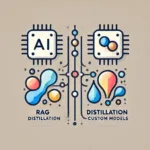
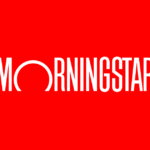
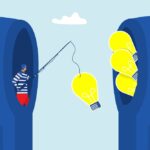
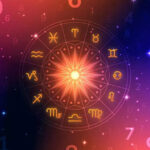
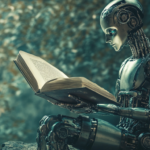
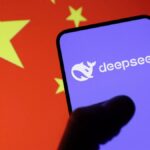
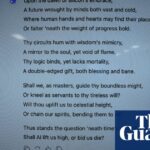
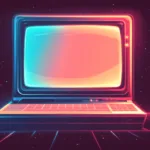

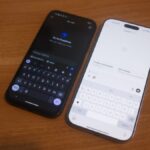
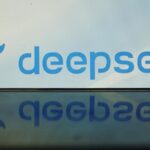

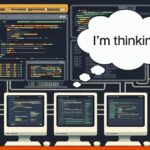
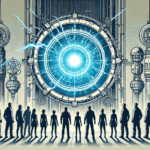
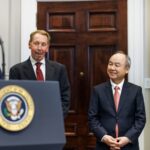
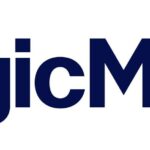
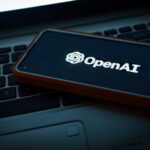

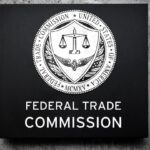
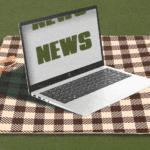
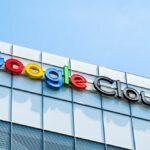
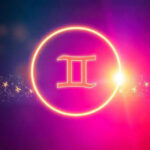
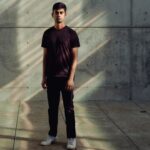
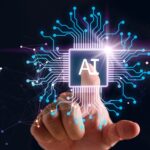
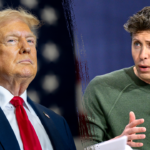
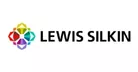
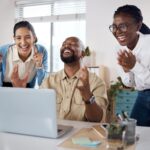
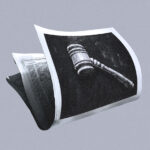
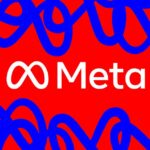

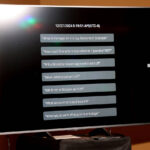

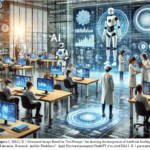
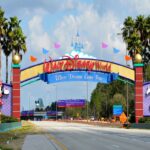
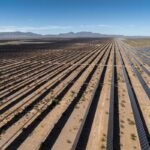
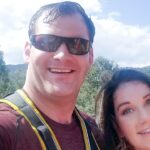
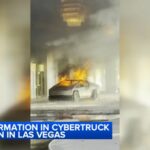
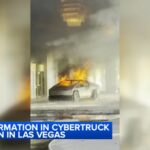
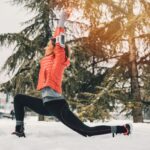
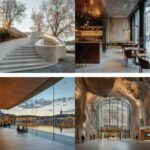
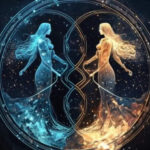
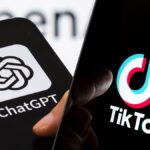
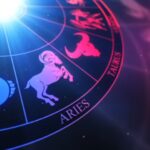
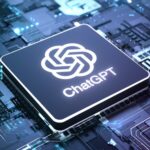
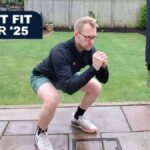
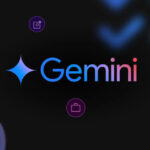
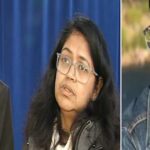
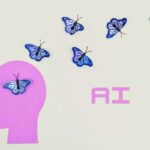
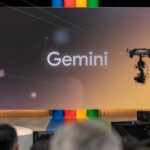
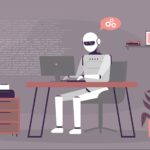
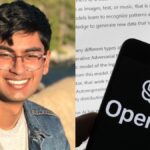
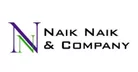

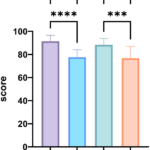
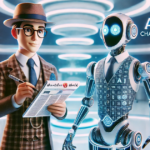
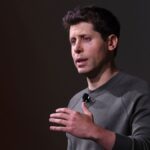
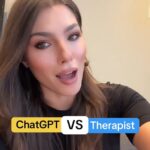
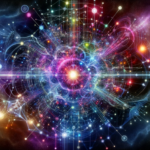
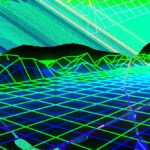
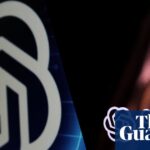
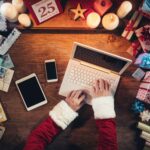
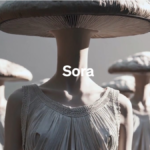
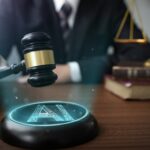
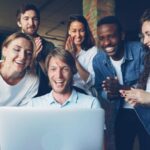
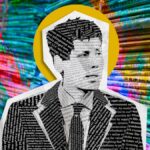

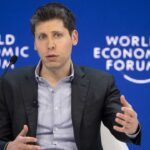
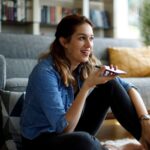
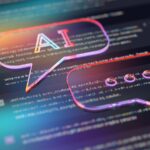
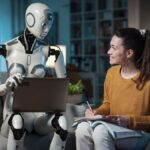
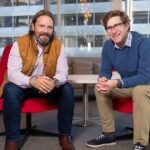
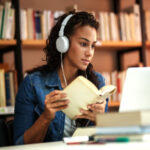
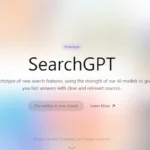
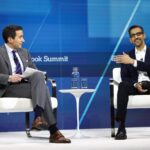
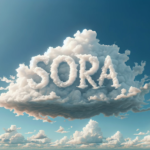
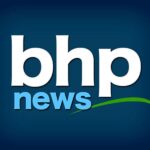
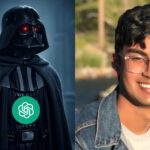
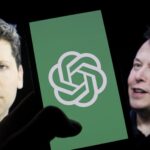
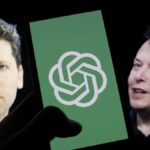
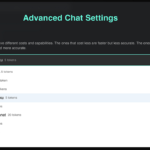
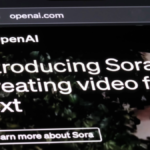
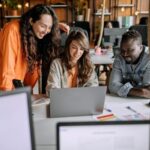
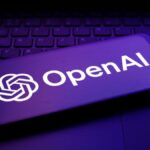
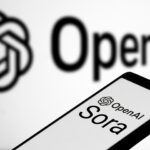
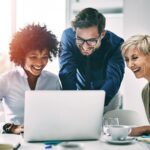
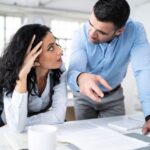
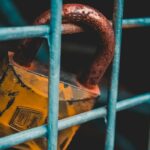
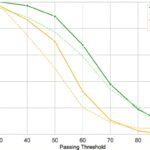
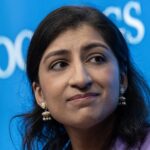
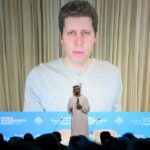
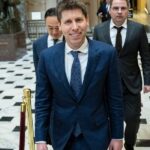
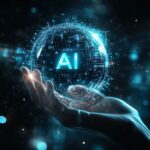
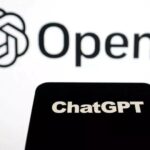
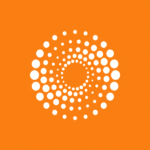
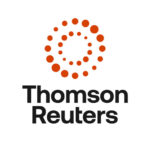
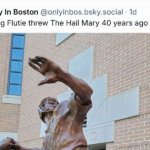

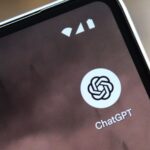
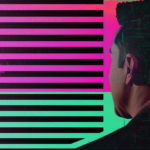
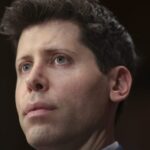
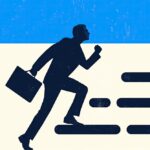
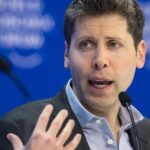
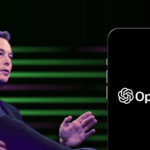
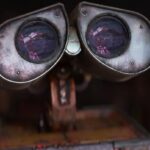
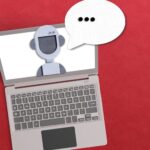
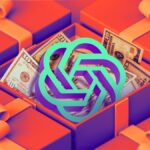
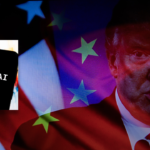
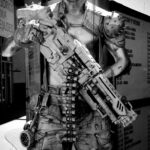
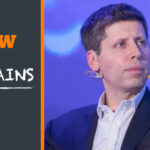
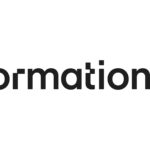
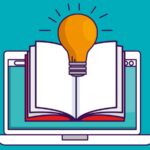
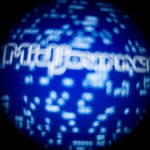
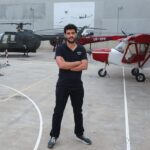
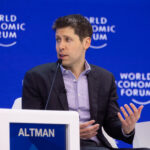
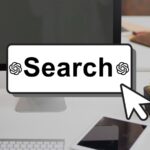
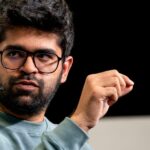
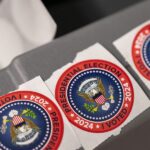
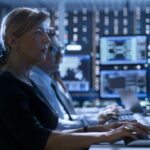
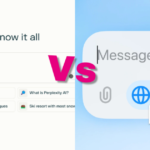
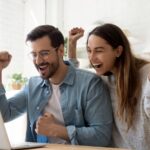
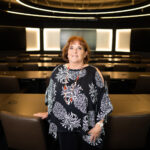
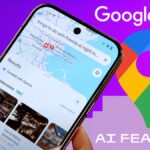
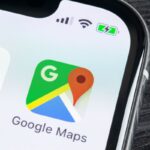
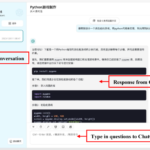
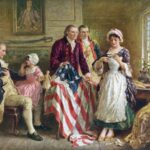
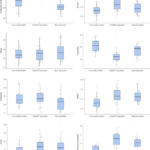

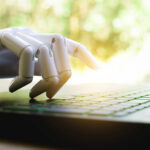
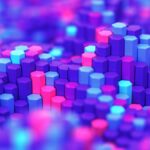
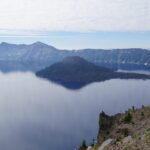
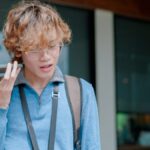
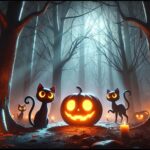
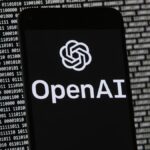
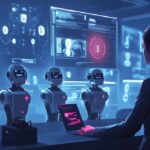
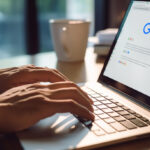
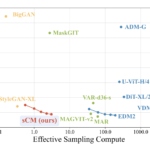
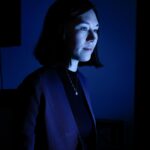
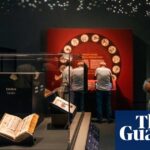
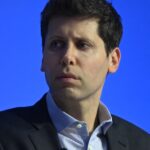
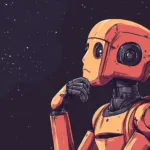
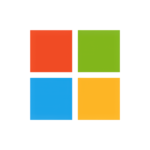
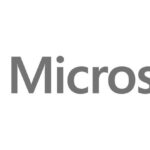
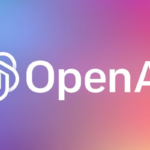
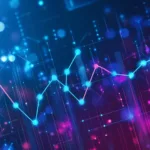
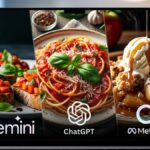
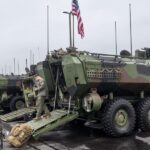

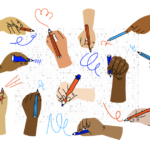
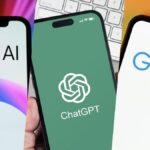
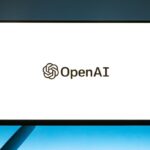
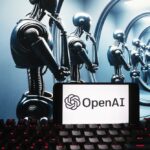
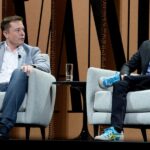
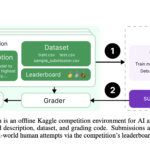
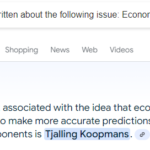
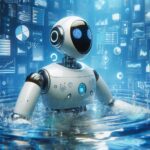
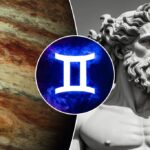
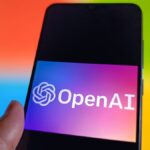
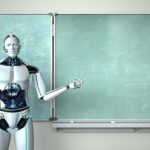
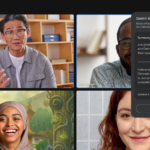
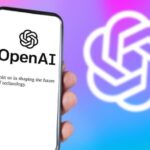
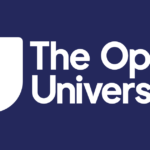
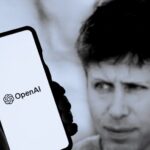
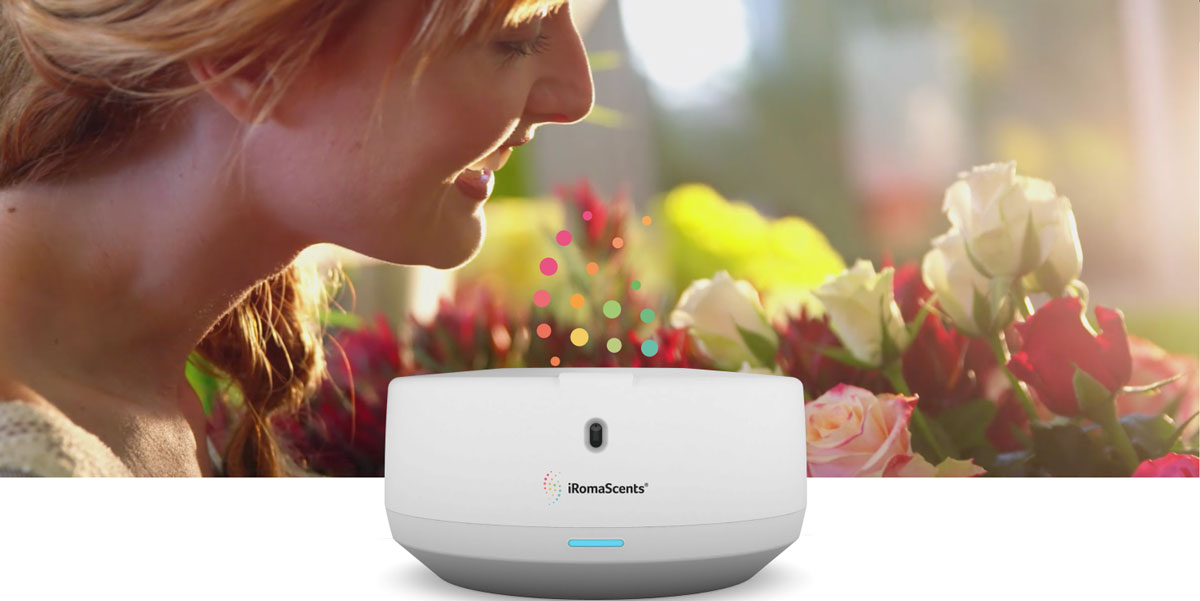

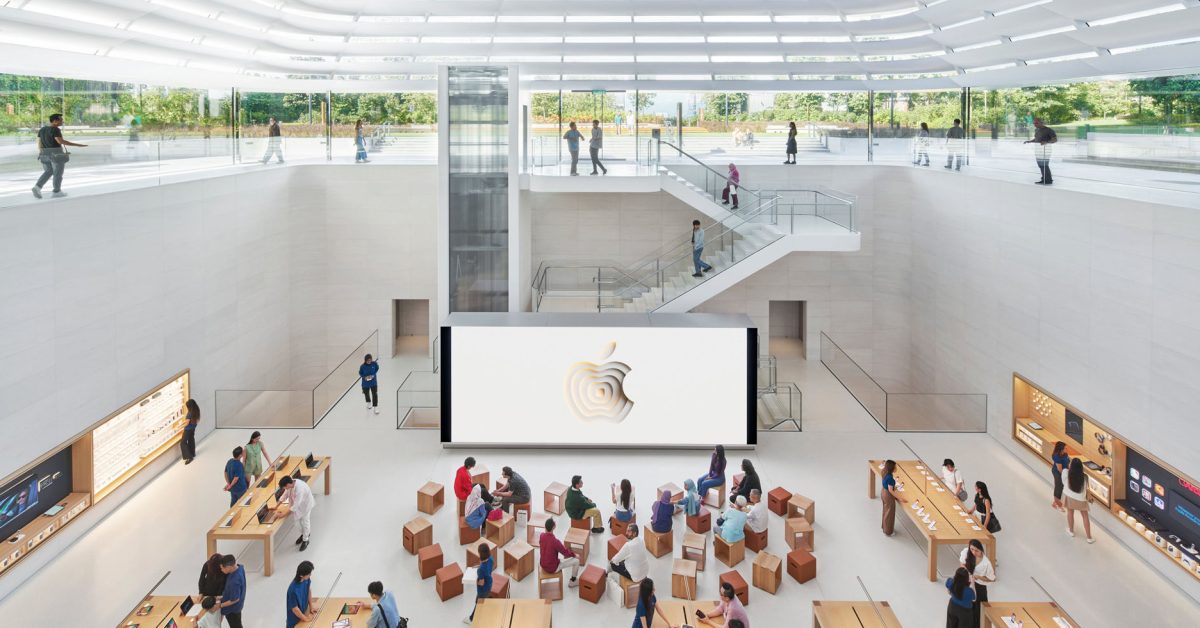


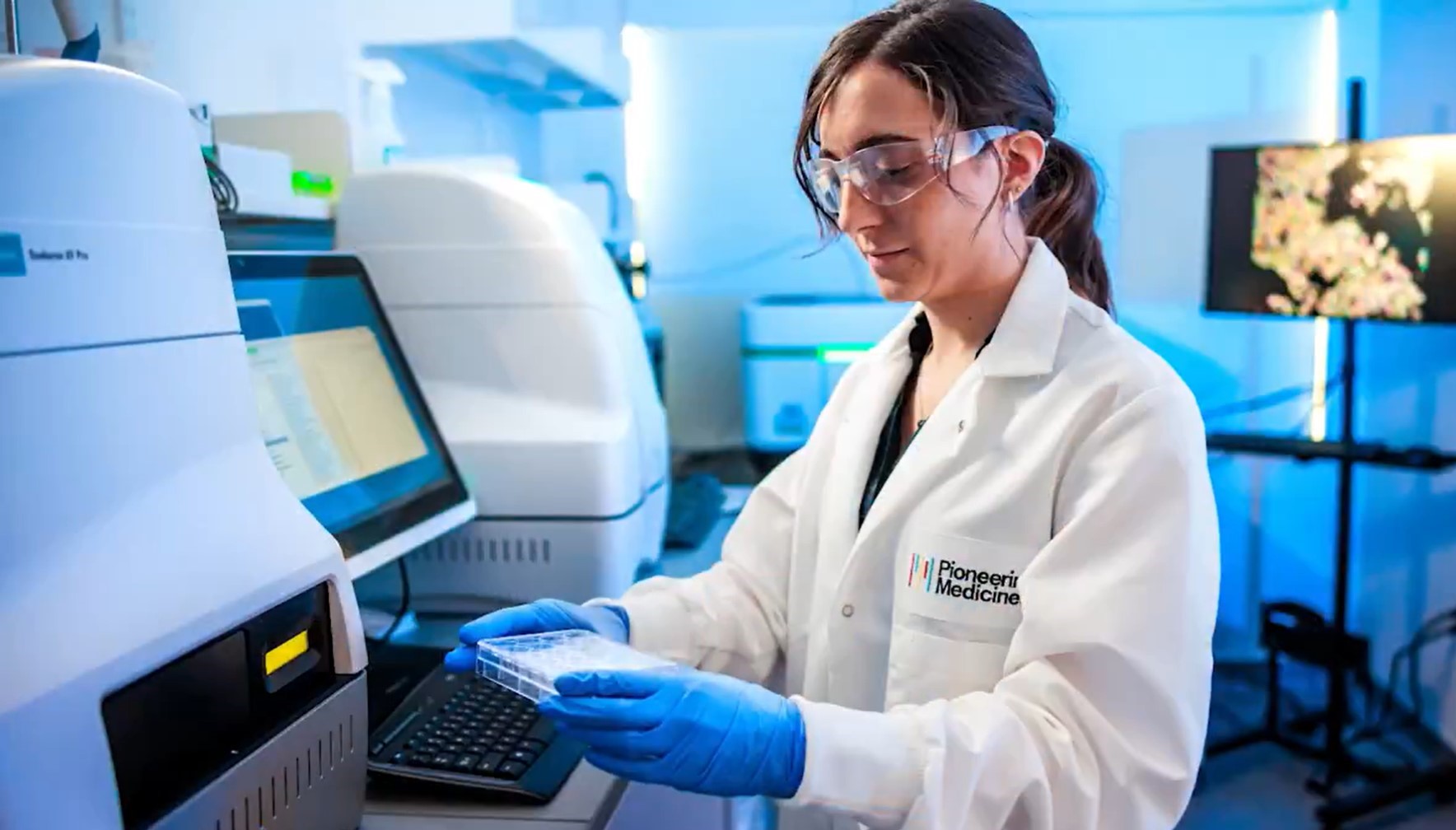
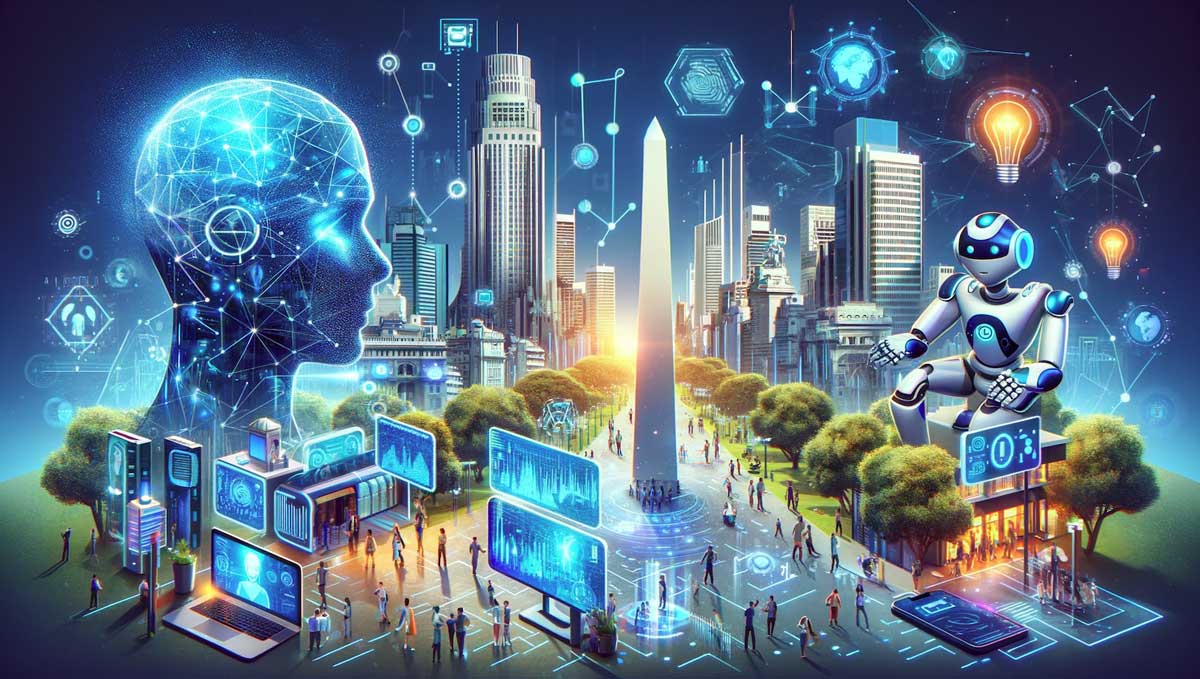

Trending
-
Startups11 meses ago
Remove.bg: La Revolución en la Edición de Imágenes que Debes Conocer
-
Tutoriales11 meses ago
Cómo Comenzar a Utilizar ChatGPT: Una Guía Completa para Principiantes
-
Startups9 meses ago
Startups de IA en EE.UU. que han recaudado más de $100M en 2024
-
Recursos11 meses ago
Cómo Empezar con Popai.pro: Tu Espacio Personal de IA – Guía Completa, Instalación, Versiones y Precios
-
Startups11 meses ago
Deepgram: Revolucionando el Reconocimiento de Voz con IA
-
Recursos11 meses ago
Suno.com: La Revolución en la Creación Musical con Inteligencia Artificial
-
Recursos11 meses ago
Perplexity aplicado al Marketing Digital y Estrategias SEO
-
Noticias9 meses ago
Dos periodistas octogenarios deman a ChatGPT por robar su trabajo