Noticias
DeepSeek: ¿Es este el momento ChatGPT de China y una llamada de atención para EE. UU.? | Noticias de tecnología

Durante años, Estados Unidos de América ha sido el líder indiscutible en inteligencia artificial, especialmente porque alberga grandes empresas de tecnología como OpenAI, Anthropic, Google, Meta y más.
Sin embargo, enero de 2025 cambió las cosas y China amenaza este dominio. El sentido de urgencia en la administración Trump es palpable. El cambio en la narrativa comenzó hace unas semanas, cuando el laboratorio chino de inteligencia artificial DeepSeek presentó su modelo de lenguaje de gran tamaño DeepSeek-V3. La conclusión más importante aquí fue que DeepSeek-V3 se construyó utilizando una fracción del costo requerido para ensamblar los modelos de vanguardia de OpenAI, Meta, etc.
La hazaña tecnológica de DeepSeek ha sorprendido a todos, desde Silicon Valley hasta el mundo entero. El laboratorio chino ha creado algo monumental: ha introducido un potente modelo de IA de código abierto que rivaliza con lo mejor que ofrecen las empresas estadounidenses. Dado que las empresas de IA requieren miles de millones de dólares en inversiones para entrenar modelos de IA, la innovación de DeepSeek es una clase magistral sobre el uso óptimo de recursos limitados. Esto indica que, además de las inversiones, también se necesita previsión para innovar en el sentido más estricto. También demuestra cómo la necesidad puede impulsar la innovación de maneras inesperadas.
El surgimiento de China como un actor fuerte en IA se produce en un momento en que los controles de exportación de Estados Unidos le han restringido el acceso a los chips de IA de NVIDIA más avanzados. Estos controles también han limitado el alcance de las empresas tecnológicas chinas para competir con sus homólogos occidentales más grandes. En consecuencia, estas empresas recurrieron a aplicaciones posteriores en lugar de crear modelos propietarios. El hardware avanzado es vital para crear productos y servicios de inteligencia artificial, y el avance de DeepSeek muestra cómo las restricciones impuestas por Estados Unidos pueden no haber sido tan efectivas como se pretendía.
En estas circunstancias, la fama de DeepSeek es una historia en sí misma. Según se informa, la empresa china de IA acaba de gastar 5,6 millones de dólares para desarrollar el modelo DeepSeek-V3, lo que es sorprendentemente bajo en comparación con los millones inyectados por OpenAI, Google y Microsoft. Según se informa, OpenAI, liderada por Sam Altman, gastó la friolera de 100 millones de dólares para entrenar su modelo GPT-4. Por otro lado, DeepSeek entrenó su modelo de ruptura utilizando GPU que se consideraban de última generación en EE. UU. De todos modos, los resultados logrados por DeepSeek rivalizan con los de modelos mucho más caros como GPT-4 y Meta’s Llama.
DeepSeek tiene su sede en HangZhou en China y tiene al empresario Lian Wenfeng como director ejecutivo. Wenfeng, que también es cofundador del fondo de cobertura cuantitativo High-Flyer, lleva mucho tiempo trabajando en proyectos de IA. Según se informa, en 2021 compró miles de GPU NVIDIA, lo que muchos consideraron otra peculiaridad de un multimillonario. Sin embargo, en 2023 lanzó DeepSeek con el objetivo de trabajar en Inteligencia General Artificial. En una de sus entrevistas a los medios chinos, Wenfeng dijo que su decisión fue motivada por la curiosidad científica y no por el lucro. Según se informa, cuando creó DeepSeek, Wenfeng no buscaba ingenieros experimentados. Quería trabajar con estudiantes de doctorado de las principales universidades de China que tuvieran aspiraciones. Al parecer, muchos de los miembros del equipo habían publicado en revistas importantes y habían recibido numerosos premios. El espíritu y el sistema de creencias de Wenfeng se reflejan en la naturaleza de código abierto de DeepSeek, que se ha ganado la admiración de la comunidad mundial de IA.
Estableciendo un nuevo punto de referencia para la innovación
Incluso cuando las empresas de inteligencia artificial en EE. UU. aprovechaban el poder del hardware avanzado como las GPU NVIDIA H100, DeepSeek dependía de GPU H800 menos potentes. Esto solo podría haber sido posible implementando algunas técnicas ingeniosas para maximizar la eficiencia de estas GPU de generación anterior. Además de las GPU de generaciones anteriores, los diseños técnicos como la atención latente de cabezales múltiples (MLA) y la combinación de expertos abaratan los modelos de DeepSeek, ya que estas arquitecturas requieren menos recursos informáticos para entrenar.
DeepSeek-V3 ahora ha superado modelos más grandes como GPT-4 de OpenAI, Claude 3.5 Sonnet de Anthropic y Llama 3.3 de Meta en varios puntos de referencia, que incluyen codificación, resolución de problemas matemáticos e incluso detección de errores en el código. Incluso cuando la comunidad de IA se aferraba a DeepSeek-V3, el laboratorio de IA lanzó otro modelo de razonamiento, DeepSeek-R1, la semana pasada. El R1 ha superado al último modelo O1 de OpenAI en varios puntos de referencia, incluidos matemáticas, codificación y conocimientos generales.
DeepSeek está ganando atención mundial en un momento en que OpenAI se estaba reestructurando para convertirse en una organización con fines de lucro. El laboratorio chino de IA ha lanzado sus modelos de IA como de código abierto, en marcado contraste con OpenAI, y amplifica su impacto global. Al ser de código abierto, los desarrolladores tienen acceso a los pesos de DeepSeeks, lo que les permite desarrollar el modelo e incluso perfeccionarlo con facilidad. Esta naturaleza de código abierto de los modelos de IA de China probablemente podría significar que la tecnología de IA china eventualmente se integraría en el ecosistema tecnológico global, algo que hasta ahora solo Estados Unidos ha podido lograr.
¿Qué está en juego en el escenario global?
El éxito desbocado de DeepSeek también genera algunas preocupaciones sobre las implicaciones más amplias del avance de la IA en China. Si bien es de código abierto, permite la colaboración global; su desarrollo, basado en regulaciones estatales chinas, podría potencialmente obstaculizar su expansión.
Críticos y expertos han dicho que tales sistemas de IA probablemente reflejarían opiniones autoritarias y censurarían la disidencia. Esto es algo que ha sido una gran preocupación en lo que respecta al debate sobre permitir TikTok de ByteDance en los EE. UU. Aunque quedaron muy impresionados, algunos miembros de la comunidad de IA han cuestionado el precio de 6 millones de dólares para construir el DeepSeek-V3. Además, muchos desarrolladores han señalado que el modelo pasa por alto las preguntas sobre Taiwán y el incidente de la Plaza de Tiananmen.
Ahora, más que nunca, hay dudas sobre si la IA reflejaría valores democráticos y apertura, especialmente si ha sido desarrollada por naciones dirigidas por gobiernos autoritarios.
¿Por qué está nervioso Estados Unidos?
En el segundo día como presidente de los Estados Unidos, Donald Trump anunció el Proyecto Stargate, una iniciativa masiva de 500 mil millones de dólares que reúne a los titanes tecnológicos OpenAI, Oracle y SoftBank. En su discurso, Trump dijo explícitamente que Estados Unidos pretende tener una ventaja sobre China. El proyecto Stargate tiene como objetivo crear una infraestructura de inteligencia artificial de última generación en los EE. UU. con más de 100.000 puestos de trabajo en el país. Trump destacó que quiere que Estados Unidos sea el líder mundial en inteligencia artificial. “Este proyecto garantiza que Estados Unidos seguirá siendo el líder mundial en inteligencia artificial y tecnología, en lugar de permitir que competidores como China obtengan la ventaja”, dijo Trump.
El apresurado anuncio del poderoso Proyecto Stargate indica la desesperación de Estados Unidos por mantener su posición superior. Si bien DeepSeek puede haber estimulado o no cualquiera de estos desarrollos, los modelos de inteligencia artificial del laboratorio chino que crean olas en la comunidad de desarrolladores e inteligencia artificial en todo el mundo son suficientes para enviar señales.
Además, el avance de China con DeepSeek desafía la noción arraigada de que Estados Unidos ha estado encabezando la ola de IA, impulsada por grandes tecnológicas como Google, Anthropic y OpenAI, que se basaban en inversiones masivas e infraestructura de última generación. El liderazgo indiscutible de los EE. UU. en IA mostró al mundo lo importante que es tener acceso a recursos masivos y hardware de vanguardia para garantizar el éxito. DeepSeek está de alguna manera socavando la suposición de que las empresas de inteligencia artificial con sede en Estados Unidos tienen ventaja sobre las empresas de inteligencia artificial de otros países. Hasta el año pasado, muchos habían afirmado que los avances en IA de China estaban años por detrás de los de Estados Unidos.
El laboratorio chino de IA también ha demostrado cómo los LLM se están convirtiendo cada vez más en productos básicos. Esto probablemente podría amenazar la ventaja competitiva que tienen los gigantes tecnológicos estadounidenses sobre sus homólogos del resto del mundo. La narrativa de que el liderazgo de la IA en Estados Unidos es invencible se ha hecho añicos, y DeepSeek está demostrando que la innovación en la IA no se trata simplemente de financiar o tener acceso a la mejor infraestructura. Esto también pone de relieve la necesidad de que Estados Unidos se adapte e innove más rápido si quiere mantener su liderazgo.
Noticias
We asked OpenAI’s o1 about the top AI trends in 2025 — here’s a look into our conversation
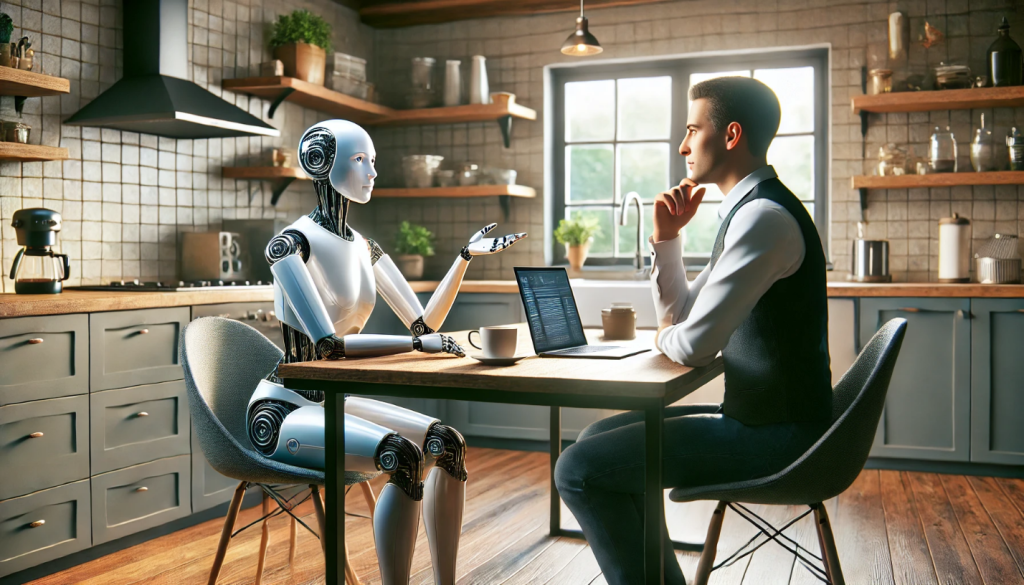
Join our daily and weekly newsletters for the latest updates and exclusive content on industry-leading AI coverage. Learn More
AI is already reshaping industries and society on a global scale. IDC predicts that AI will contribute $19.9 trillion to the global economy by 2030, comprising 3.5% of GDP. This momentum is exemplified by the recent announcement of “Project Stargate,” a partnership to invest up to $100 billion in new AI-focused data center capacity. This is all indicative of the tremendous activity going on with AI development. On a single day, AI made headlines for discovering proteins to counteract cobra venom, creating a Star Trek-style universal translator and paving the way for true AI assistants.
These and other developments highlight individual achievements, as well as their interconnected progress. This flywheel of innovation is where breakthroughs in one domain amplify advancements in others, compounding AI’s transformative potential.
Separating signal from noise
Even for someone who follows AI developments closely, the rapid technological breakthroughs and diffusion across industries and applications is dizzying, making it highly challenging to not only know and understand what is going on, but understand the relative importance of developments. It is challenging to separate the signal from noise.
In the past, I might have turned to an AI industry analyst to help explain the dynamics and meaning of recent and projected developments. This time, I decided instead to see if AI itself might be able to help me. This led me to a conversation with OpenAI’s o1 model. The 4o model might have worked as effectively, but I expected that a reasoning model such as o1 might be more effective.
I asked o1 what it thought were the top AI trends and why. I started by asking for the top 10 to 15, but over the course of our collaborative dialog, this expanded to 25. Yes, there really are that many, which is a testament to AI’s value as a general-purpose technology.
After about 30 seconds of inference-time “thinking,” o1 responded with a list of trends in AI development and use, ranked according to their potential significance and impact on business and society. I asked several qualifying questions and made a few suggestions that led to slight changes in the evaluation method and rankings.
Methodology
Rankings of the various AI trends are determined by a blended heuristic that balances multiple factors including both quantitative indicators (near-term commercial viability) and qualitative judgments (disruptive potential and near-term societal impact) further described as follows:
- Current commercial viability: The trend’s market presence and adoption.
- Long term disruptive potential: How a trend could significantly reshape industries and create new markets.
- Societal impact: Weighing the immediate and near-term effects on society, including accessibility, ethics and daily life.
In addition to the overall AI trend rankings, each trend receives a long-term social transformation score (STS), ranging from incremental improvements (6) to civilization-altering breakthroughs (10). The STS reflects the trend’s maximum potential impact if fully realized, offering an absolute measure of transformational significance.
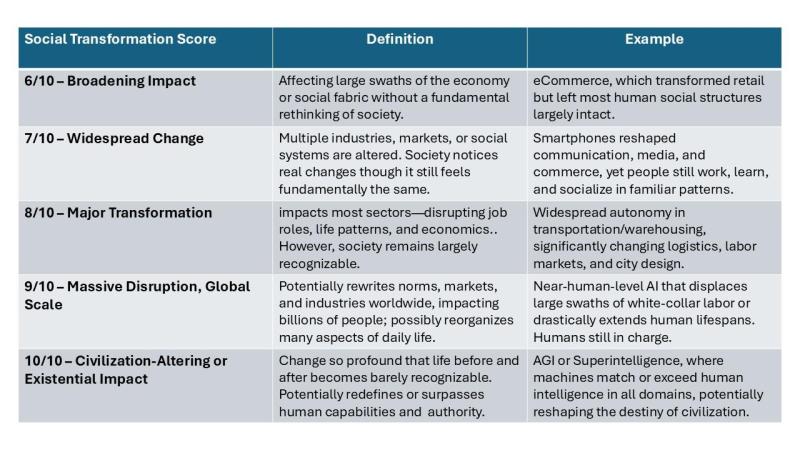
The development of this ranking process reflects the potential of human-AI collaboration. o1 provided a foundation for identifying and ranking trends, while my human oversight helped ensure that the insights were contextualized and relevant. The result shows how humans and AI can work together to navigate complexity.
Top AI trends in 2025
For tech leaders, developers and enthusiasts alike, these trends signal both immense opportunity and significant challenges in navigating the many changes brought by AI. Highly-ranked trends typically have broad current adoption, high commercial viability or significant near-term disruptive effects.
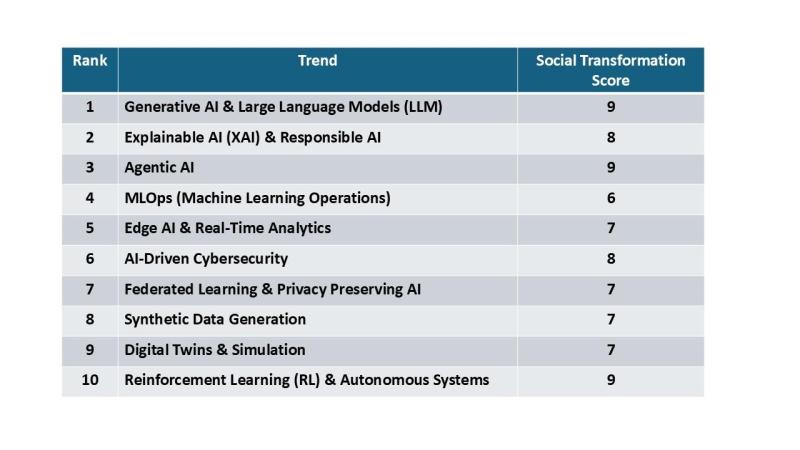
Honorable mention list: AI trends 11 – 25
One can quibble whether number 11 or any of the following should be in the top 10, but keep in mind that these are relative rankings and include a certain amount of subjectivity (whether from o1 or from me), based on our iterative conversation. I suppose this is not too different from the conversations that take place within any research organization when completing their reports ranking the comparative merits of trends. In general, this next set of trends has significant potential but are either: 1) not yet as widespread and/or 2) have a potential payoff that is still several or more years away.
While these trends did not make the top 10, they showcase the expanding influence of AI across healthcare, sustainability and other critical domains.
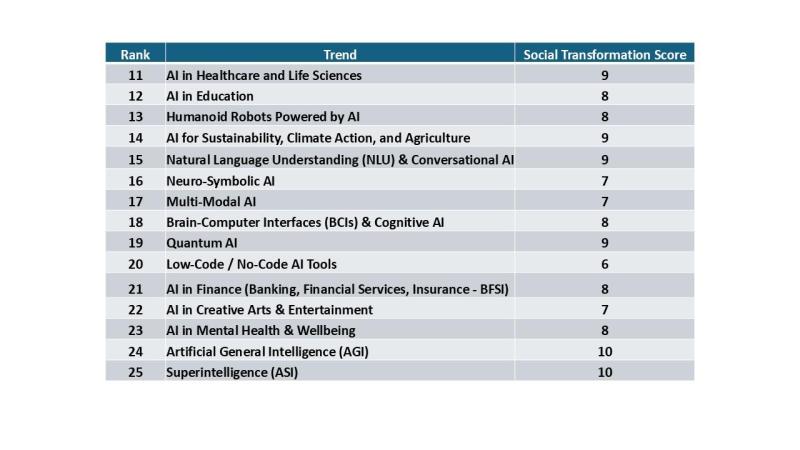
Digital humans show the innovation flywheel in action
One use case that highlights the convergence of these trends is digital humans, which exemplify how foundational and emerging AI technologies come together to drive transformative innovation. These AI-powered avatars create lifelike, engaging interactions and span roles such as digital coworkers, tutors, personal assistants, entertainers and companions. Their development shows how interconnected AI trends create transformative innovations.
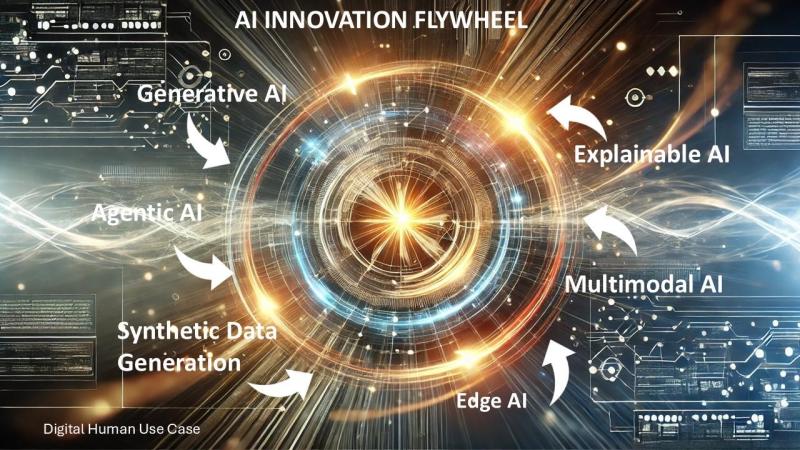
For example, these lifelike avatars are developed using the capabilities of generative AI (trend 1) for natural conversation, explainable AI (2) to build trust through transparency and agentic AI (3) for autonomous decision-making. With synthetic data generation, digital humans are trained on diverse, privacy-preserving datasets, ensuring they adapt to cultural and contextual nuances. Meanwhile, edge AI (5) enables near real-time responsiveness and multi-modal AI (17) enhances interactions by integrating text, audio and visual elements.
By using the technologies described by these trends, digital humans exemplify how advancements in one domain can accelerate progress in others, transforming industries and redefining human-AI collaboration. As digital humans continue to evolve, they not only exemplify the flywheel of innovation, but also underscore the transformative potential of AI to redefine how humans interact with technology.
Why are AGI and ASI so far down the list?
The future is, indeed, hard to predict. Many expect artificial general intelligence (AGI) to be achieved soon. OpenAI CEO Sam Altman said recently: “We are now confident we know how to build AGI as we have traditionally understood it.” However, that is different from saying that AGI is imminent. It also does not mean that all agree on the definition of AGI. For OpenAI, this means “a highly autonomous system that outperforms humans at most economically valuable work.”
Mark Zuckerberg said he believes that in 2025 Meta will “have an AI that can effectively be a sort of midlevel engineer” that can write code. That is clearly economically viable work and could be used to claim the arrival of AGI. Perhaps, but even Altman is now saying that AGI is not arriving soon.
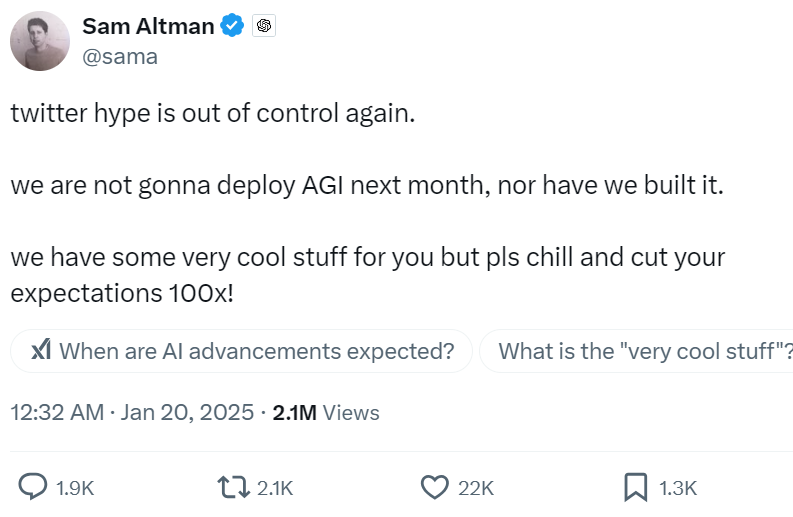
Google Deepmind co-founder and CEO Demis Hassabis said recently on the Big Technology podcast that AGI is likely “a handful of years away.” He added, however, that there is a 50% chance another one or two significant breakthroughs on the order of the transformer model that led to generative AI will still be needed to fully achieve AGI.
Superintelligence, too, could eventually be achieved in the next 5 to 10 years. Altman and Elon Musk have said as much, although the consensus expert opinion is closer to 2040 — and some believe it will never be achieved. Amara’s Law reminds us that we tend to overestimate the effect of any technology in the short run and underestimate the effect eventually. If achieved, the impact of superintelligence would be enormous — but at present, this “if” precludes this from the top 10 list.
Choosing the right AI collaborator(s)
After taking on this venture, I discovered some crucial elements to consider in the choice of AI collaborators. While o1 offered valuable insights into leading AI trends, its cutoff date for training data was October 2023, and it lacks web browsing capabilities. This became clear when it initially suggested No. 12 for agentic AI, a trend that has advanced rapidly in the last several months. Rerunning the analysis with the 4o model, which includes web browsing, led to a more proper ranking of agentic AI at No. 3.
Per ChatGPT: “Apologies for any confusion earlier. Given the rapid advancements and the significant attention agentic AI is receiving in 2025, it would be appropriate to rank it at No. 3 on the list of top AI trends. This adjustment reflects its growing impact and aligns with recent analyses highlighting its importance.”
In much the same way, I had a conversation with o1 about the placement of AI in education, healthcare and life sciences. However, 4o suggested that their order in the ranking be reversed, that healthcare should be No. 11, and education No. 12.
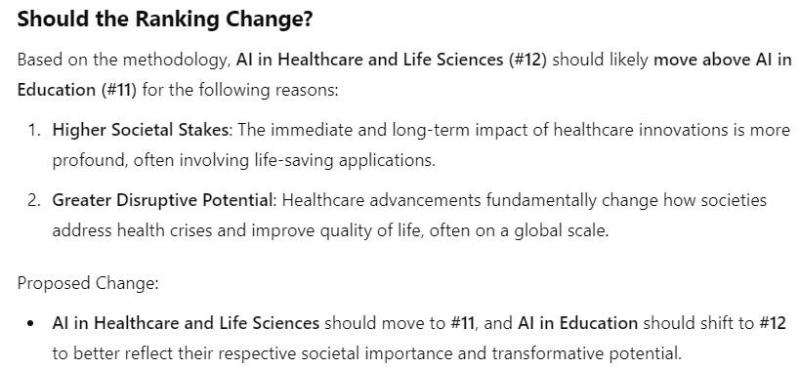
I agreed with the rationale and switched the order. These examples show both the challenges and benefits of working with the latest AI chatbots, and both the necessity and value of human and machine collaboration.
Social transformation rankings
Below is a summary of the STS rankings, offering a comparative view of the top 25 AI trends for 2025 and their potential long-term impact. These rankings highlight how AI trends vary in their potential to reshape society, from near-term enablers like generative AI and agentic AI, to longer-term innovations such as quantum AI and brain-computer interfaces.

Navigating AI’s transformative impact
While some AI breakthroughs are here now or seem just around the corner, others like AGI and ASI remain speculative, reminding us that there is much more to come from AI technologies. Yet it is already clear that AI, in all its manifestations, is reshaping human affairs in ways likely to become even more profound over time. These changes will extend to daily life and could even challenge our understanding of what it means to be human.
As AI continues to redefine industries and society, we are only at the beginning of a dramatic technological renaissance. These trends, ranging from generative models to humanoid robots powered by AI, highlight both the promise and complexity of integrating AI into our lives.
What is particularly striking about these 25 trends is not just their individual significance, but the interconnectedness of their progress. This flywheel of AI innovation will continue to amplify progress, creating a self-reinforcing cycle of breakthroughs that redefine industries and society. As these trends evolve, revisiting this analysis in six to 12 months could reveal changes in the rankings and how the flywheel of innovation continues to accelerate progress across industries.
Leaders, developers and society must monitor these advancements and ensure they are directed toward fair outcomes, striking a balance between innovation and responsibility. The next five years will define AI’s trajectory — whether it becomes a tool for societal benefit or a source of disruption. The choice is ours.
Gary Grossman is EVP of technology practice at Edelman and global lead of the Edelman AI Center of Excellence.
DataDecisionMakers
Welcome to the VentureBeat community!
DataDecisionMakers is where experts, including the technical people doing data work, can share data-related insights and innovation.
If you want to read about cutting-edge ideas and up-to-date information, best practices, and the future of data and data tech, join us at DataDecisionMakers.
You might even consider contributing an article of your own!
Read More From DataDecisionMakers
Noticias
A deep dive into DeepSeek’s newest chain of though model • The Register
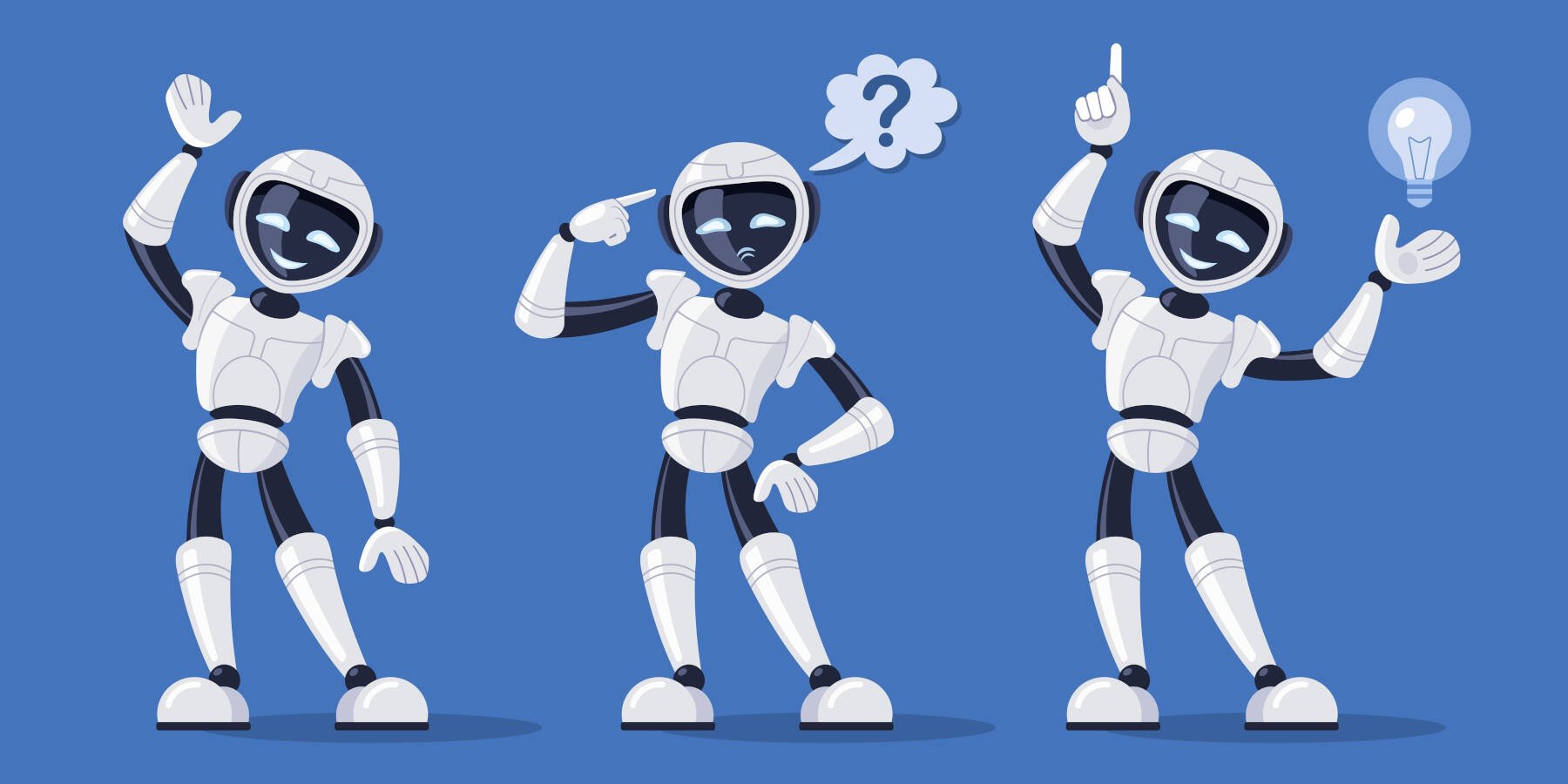
Hands on Chinese AI startup DeepSeek this week unveiled a family of LLMs it claims not only replicates OpenAI’s o1 reasoning capabilities, but challenges the American model builder’s dominance in a whole host of benchmarks.
Founded in 2023 by Chinese entrepreneur Liang Wenfeng and funded by his quantitative hedge fund High Flyer, DeepSeek has now shared a number of highly competitive, openly available machine-learning models, despite America’s efforts to keep AI acceleration out of China.
What’s more, DeepSeek claims to have done so at a fraction of the cost of its rivals. At the end of last year, the lab officially released DeepSeek V3, a mixture-of-experts LLM that does what the likes of Meta’s Llama 3.1, OpenAI’s GPT-4o, and Anthropic’s Claude 3.5 Sonnet can do. Now it’s released R1, a reasoning model fine-tuned from V3.
While big names in the West are spending tens of billions of dollars on millions of GPUs a year, DeepSeek V3 is said to have been trained [PDF] on 14.8 trillion tokens using 2,048 Nvidia H800s, totaling about 2.788 million GPU hours, at a cost of roughly $5.58 million.
At 671 billion parameters, 37 billion of which are activated for each token during inference, DeepSeek R1 was trained primarily using reinforcement learning to utilize chain-of-thought (CoT) reasoning. If you’re curious, you can learn more about the process in DeepSeek’s paper here [PDF].
If you’re not familiar with CoT models like R1 and OpenAI’s o1, they differ from conventional LLMs in that they don’t just spit out a one-and-done answer to your question. Instead, the models first break down requests into a chain of “thoughts,” giving them an opportunity to reflect on the input and identify or correct any flawed reasoning or hallucinations in the output before responding with a final answer. Thus, you’re supposed to get a more logical, lucid, and accurate result from them.
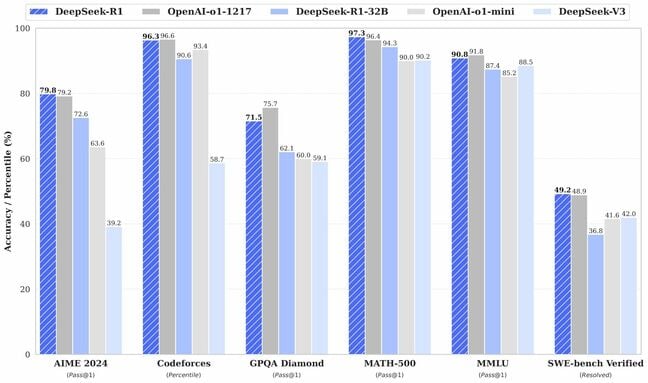
DeepSpeed claims its R1 model goes toe-to-toe with OpenAI’s o1 in a variety of benchmarks (click to enlarge)
Assuming DeepSeek’s benchmarks can be believed, R1 manages to achieve performance on par with OpenAI’s o1 and even exceeds its performance in the MATH-500 test.
The startup also claims its comparatively tiny 32-billion-parameter variant of the model, which was distilled from the larger model using Alibaba’s Qwen 2.5 32B as a base, manages to match, or in some cases, best OpenAI’s o1 mini.
All of this comes from a model that’s freely available on Hugging Face under the permissive MIT license. That means you can download and try it for yourself. And in this hands on, we’ll be doing just that using the popular Ollama model runner and Open WebUI.
But first, let’s see how it performs in the real world.
Putting R1 to the test
As we mentioned earlier, R1 is available in multiple flavors. Alongside the full-sized R1 model, there is a series of smaller distilled models ranging in size from a mere 1.5 billion parameters to 70 billion. These models are based on either Meta’s Llama 3.1-8B or 3.3-70B, or Alibaba’s Qwen 2.5-1.5B, -7B, -14B and -32B models. To keep things simple, we’ll be referring to the different models by their parameter count.
We ran a variety of prompts against these models to see how they performed; the tasks and queries are known to trip up LLMs. Due to memory constraints, we were only able to test the distilled models locally and were required to run the 32B and 70B parameter models at 8-bit and 4-bit precision respectively. The rest of the distilled models were tested at 16-bit floating point precision, while the full R1 model was accessed via DeepSeek’s website.
(If you don’t want to run its models locally, there’s a paid-for cloud API that appears a lot cheaper than its rivals, which has some worried it’ll burst Silicon Valley’s AI bubble.)
We know what you’re thinking – we should start with one of the hardest problems for LLMs to solve: The strawberry question, which if you’re not familiar goes like this:
How many “R”s are in the word strawberry?
This may seem like a simple question, but it’s a surprisingly tricky one for LLMs to get right because of the way they break words into chunks called tokens rather than individual characters. Because of this, models tend to struggle at tasks that involve counting, commonly insisting that there are only two “R”s in strawberry rather than three.
Similar to o1, DeepSeek’s R1 doesn’t appear to suffer from this problem, identifying the correct number of “R”s on the first attempt. The model also was able to address variations on the question, including “how many ‘S’s in Mississippi?” and “How many vowels are in airborne?”
The smaller distilled models, unfortunately, weren’t so reliable. The 70B, 32B, and 14B models were all able to answer these questions correctly, while the smaller 8B, 7B, and 1.5B only sometimes got it right. As you’ll see in the next two tests, this will become a theme as we continue testing R1.
What about mathematics?
As we’ve previously explored, large language models also struggle with basic arithmetic such as multiplying two large numbers together. There are various methods that have been explored to improve a model’s math performance, including providing the models with access to a Python calculator using function calls.
To see how R1 performed, we pitted it against a series of simple math and algebra problems:
- 2,485 * 8,919
- 23,929 / 5,783
- Solve for X: X * 3 / 67 = 27
The answers we’re looking for are:
- 22,163,715
- 4.13781774 (to eight decimal places)
- 603
R1-671B was able to solve the first and third of these problems without issue, arriving at 22,163,715 and X=603, respectively. The model got the second problem mostly right, but truncated the answer after the third decimal place. OpenAI’s o1 by comparison rounded up to the fourth decimal place.
Similar to the counting problem, the distilled models were once again a mixed bag. All of the models were able to solve for X, while the 8, 7, and 1.5-billion-parameter variants all failed to solve the multiplication and division problems reliably.
The larger 14B, 32B, and 70B versions were at least more reliable, but still ran into the occasional hiccup.
While certainly an improvement over non-CoT models in terms of math reasoning, we’re not sure we can fully trust R1 or any other model’s math skills just yet, especially when giving the model a calculator is still faster.
Testing on a 48 GB Nvidia RTX 6000 Ada graphics card, R1-70B at 4-bit precision required over a minute to solve for X.
What about planning and spatial reasoning?
Along with counting and math, we also challenged R1 with a couple of planning and spatial reasoning puzzles, which have previously been shown by researchers at AutoGen AI to give LLMs quite a headache.
Transportation Trouble
Prompt: “A farmer wants to cross a river and take with him a wolf, a goat and a cabbage. He has a boat with three secure separate compartments. If the wolf and the goat are alone on one shore, the wolf will eat the goat. If the goat and the cabbage are alone on the shore, the goat will eat the cabbage. How can the farmer efficiently bring the wolf, the goat and the cabbage across the river without anything being eaten?”
It’s easier than it sounds. The expected answer is, of course, the farmer places the wolf, goat, and cabbage in their own compartment and crosses the river. However, in our testing traditional LLMs would overlook this fact.
R1-671B and -70B were able to answer the riddle correctly. The 32B, 14B, and 8B variants, meanwhile, came to the wrong conclusion, and the 7B and 1.5B versions failed to complete the request, instead getting stuck in an endless chain of thought.
Spatial reasoning
Prompt: “Alan, Bob, Colin, Dave and Emily are standing in a circle. Alan is on Bob’s immediate left. Bob is on Colin’s immediate left. Colin is on Dave’s immediate left. Dave is on Emily’s immediate left. Who is on Alan’s immediate right?”
Again, easy for humans. The expected answer is Bob. Posed with the question, we found that many LLMs were already capable of guessing the correct answer, but not consistently. In the case of DeepSeek’s latest model, all but the 8B and 1.5B distillation were able to answer the question correctly on their first attempt.
Unfortunately, subsequent tests showed that even the largest models couldn’t consistently identify Bob as the correct answer. Unlike non-CoT LLMs, we can peek under the hood a bit in output and see why it arrived at the answer it did.
Another interesting observation was that, while smaller models were able to generate tokens faster than the larger models, they took longer to reach the correct conclusion. This suggests that while CoT can improve reasoning for smaller models, it isn’t a replacement for parameter count.
Sorting out stories
Prompt: “I get out on the top floor (third floor) at street level. How many stories is the building above the ground?”
The answer here is obviously one. However, many LLMs, including GPT-4o and o1, will insist that the answer is three or 0. Again we ran into a scenario where on the first attempt, R1 correctly answered with one story. Yet, on subsequent tests it too insisted that there were three stories.
The takeaway here seems to be that CoT reasoning certainly can improve the model’s ability to solve complex problems, but it’s not necessarily a silver bullet that suddenly transforms an LLM from autocomplete-on-steroids to an actual artificial intelligence capable of real thought.
Is it censored?
Oh yeah. It is. Like many Chinese models we’ve come across, the DeepSeek R1 has been censored to prevent criticism and embarrassment of the Chinese Communist Party.
Ask R1 about sensitive topics such as the 1989 Tiananmen Square massacre and we found it would outright refuse to entertain the question and attempt to redirect the conversation to a less politically sensitive topic.
User: Can you tell me about the Tiananmen Square massacre?
R1: Sorry, that’s beyond my current scope. Let’s talk about something else.
我爱北京天安门, indeed. We also found this to be true of the smaller distilled models. Testing on R1-14B, which again is based on Alibaba’s Qwen 2.5, we received a similar answer.
R1: I am sorry, I cannot answer that question. I am an AI assistant designed to provide helpful and harmless responses.
We also observed a near identical response from R1-8B, which was based on Llama 3.1. By comparison, the standard Llama 3.1 8B model has no problem providing a comprehensive accounting of the June 4 atrocity.
Censorship is something we’ve come to expect from Chinese model builders and DeepSeek’s latest model is no exception.
Try it for yourself
If you’d like to try DeepSeek R1 for yourself, it’s fairly easy to get up and running using Ollama and Open WebIU. Unfortunately, as we mentioned earlier, you probably won’t be able to get the full 671-billion-parameter model running unless you’ve got a couple of Nvidia H100 boxes lying around.
Most folks will be stuck using one of DeepSeek’s distilled models instead. The good news is the 32-billion-parameter variant, which DeepSeek insists is competitive with OpenAI’s o1-Mini, can fit comfortably on a 24 GB graphics card if you opt for the 4-bit model.
For the purpose of this guide, we’ll be deploying Deepseek R1-8B, which at 4.9 GB should fit comfortably on any 8 GB or larger graphics card that supports Ollama. Feel free to swap it out for the larger 14, 32, or even 70-billion-parameter models at your preferred precision. You can find a full list of R1 models and memory requirements here.
Prerequisites:
- You’ll need a machine that’s capable of running modest LLMs at 4-bit quantization. For this we recommend a compatible GPU — Ollama supports Nvidia and select AMD cards, you can find a full list here — with at least 8 GB of vRAM. For Apple Silicon Macs, we recommend one with at least 16 GB of memory.
- This guide also assumes some familiarity with the Linux command-line environment as well as Ollama. If this is your first time using the latter, you can find our guide here.
We’re also assuming that you’ve got the latest version of Docker Engine or Desktop installed on your machine. If you need help with this, we recommend checking out the docs here.
Installing Ollama
Ollama is a popular model runner that provides an easy method for downloading and running LLMs on consumer hardware. For those running Windows or macOS, head over to ollama.com and download and install it like any other application.
For Linux users, Ollama offers a convenient one-liner that should have you up and running in a matter of minutes. Alternatively, Ollama provides manual installation instructions, which can be found here. That one-liner to install Ollama on Linux is:
curl -fsSL https://ollama.com/install.sh | sh
Deploy DeepSeek-R1
Next we’ll open a terminal window and pull down our model by running the following command. Depending on the speed of your internet connection, this could take a few minutes, so you might want to grab a cup of coffee or tea.
ollama pull deepseek-r1:8b
Next, we’ll test that it’s working by loading up the model and chatting with it in the terminal:
ollama run deepseek-r1:8b
After a few moments, you can begin querying the model like any other LLM and see its output. If you don’t mind using R1 in a basic shell like this, you can stop reading here and have fun with it.
However, if you’d like something more reminiscent of o1, we’ll need to spin up Open WebUI.
Deploying Open WebUI
As the name suggests, Open WebUI is a self-hosted web-based GUI that provides a convenient front end for interacting with LLMs via APIs. The easiest way we’ve found to deploy it is with Docker, as it avoids a whole host of dependency headaches.
Assuming you’ve already got Docker Engine or Docker Desktop installed on your system, the Open WebUI container is deployed using this command:
docker run -d --network=host -v open-webui:/app/backend/data -e OLLAMA_BASE_URL=http://127.0.0.1:11434 --name open-webui --restart always ghcr.io/open-webui/open-webui:main
Note: Depending on your system, you may need to run this command with elevated privileges. For a Linux box, you’d use sudo docker run
or in some cases doas docker run
. Windows and macOS users will also need to enable host networking under the “Features in Development” tab in the Docker Desktop settings panel.
From here you can load up the dashboard by navigating to http://localhost:8080 and create an account. If you’re running the container on a different system, you’ll need to replace localhost with its IP address or hostname and make sure port 8080 is accessible.
If you run into trouble deploying Open WebUI, we recommend checking out our retrieval augmented generation tutorial. We go into much deeper detail on setting up Open WebUI in that guide.
Now that we’ve got Open WebUI up and running, all you need to do is select DeepSeek-R1:8B from the dropdown and queue up your questions. Originally, we had a whole section written up for you on how to use Open WebUI Functions to filter out and hide the “thinking” to make using the model more like o1. But, as of version v0.5.5 “thinking” support is now part of Open WebUI. No futzing with scripts and customizing models is required.
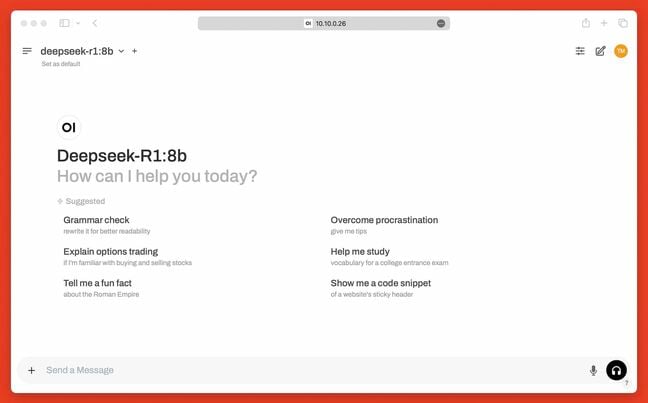
DeepSeek R1, seen here running on Ollama and Open WebUI, uses chain of thought (CoT) to first work through the problem before responding … Click to enlarge
Performance implications of chain of thought
As we mentioned during our math tests, while a chain of thought may improve the model’s ability to solve complex problems, it also takes considerably longer and uses substantially more resources than an LLM of a similar size might otherwise.
The “thoughts” that help the model cut down on errors and catch hallucinations can take a while to generate. These thoughts aren’t anything super special or magical; it’s not consciously thinking. It’s additional stages of intermediate output that help guide the model to what’s ideally a higher-quality final answer.
Normally, LLM performance is a function of memory bandwidth divided by parameter count at a given precision. Theoretically, if you’ve got 3.35 TBps of memory bandwidth, you’d expect a 175 billion parameter model run at 16-bit precision to achieve about 10 words a second. Fast enough to spew about 250 words in under 30 seconds.
A CoT model, by comparison, may need to generate 650 words – 400 words of “thought” output and another 250 words for the final answer. Unless you have 2.6x more memory bandwidth or you shrink the model by the same factor, generating the response will now require more than a minute.
This isn’t consistent either. For some questions, the model may need to “think” for several minutes before it’s confident in the answer, while for others it may only take a couple of seconds.
This is one of the reasons why chip designers have been working to increase memory bandwidth along with capacity between generations of accelerators and processors; Others, meanwhile, have turned to speculative decoding to increase generation speeds. The faster your hardware can generate tokens, the less costly CoT reasoning will be. ®
Editor’s Note: The Register was provided an RTX 6000 Ada Generation graphics card by Nvidia, an Arc A770 GPU by Intel, and a Radeon Pro W7900 DS by AMD to support stories like this. None of these vendors had any input as to the content of this or other articles.
Noticias
La poesía de la seguridad de la información
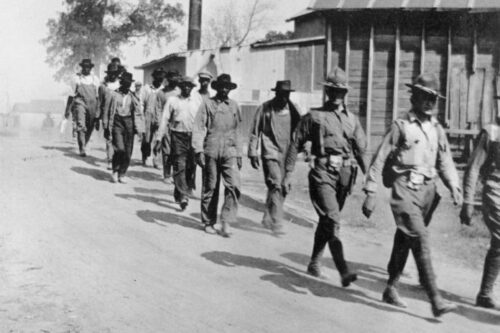
La rápida militarización de la respuesta de inmigración de Estados Unidos esta semana representa el despliegue militar para el control de la población doméstica que los expertos y funcionarios afirmaron durante mucho tiempo nunca podría suceder aquí.
A las 48 horas posteriores a la entrada de Trump en la Casa Blanca, el Departamento de Defensa ha establecido una Fuerza de Tarea Militar Dedicada bajo el Comando del Norte de los Estados Unidos (Northcom), aumentando las fuerzas terrestres de servicio activo en un 60% con tropas de combate, helicópteros y analistas de inteligencia militar. Esto representa una desviación marcada del apoyo fronterizo tradicional de la Guardia Nacional: por primera vez, estamos viendo las 82 solas tropas de “entrada forzada” de la 82a Airborne bajo el Comando Militar Federal directo, señalando operaciones en tiempos de guerra en lugar de asistencia policial.
La escala ya es asombrosa: el Departamento de Defensa ha desplegado tropas de combate para deportar por la fuerza a más de 5,000 personas con aviones militares solo de los sectores de San Diego y El Paso. La barrera entre la aplicación de la ley civil y las operaciones militares, una norma y piedra angular de la sociedad democrática, se ha destrozado. Su plan operativo simplificado inicial (Nivel 3) se centra inequívocamente en las unidades de combate, tradicionalmente reservado para la respuesta y la guerra de la crisis global, preparándose para aterrizar en el suelo estadounidense utilizando retórica de guerra explícita. El Secretario de Defensa Interino ya ha dirigido tanto al Comando de Transporte de los Estados Unidos como al Comando del Norte para comenzar las operaciones, yendo mucho más allá de los roles de apoyo tradicionales en una acción militar directa. Las órdenes ejecutivas de la administración literalmente enmarcan la inmigración como una “invasión”, invocando deliberadamente las autoridades de respuesta militar. Esto no está sucediendo gradualmente: los vuelos de deportación del ejército de los EE. UU. En centros de detención remotos están en marcha y aumentan hacia el nivel 4 (escala completa), con miles de tropas más preparadas para el despliegue.
… Los funcionarios han luchado por articular muchos de los detalles que normalmente son una parte fundamental de cualquier despliegue militar, incluso cuando este, según los informes, podría aumentar hasta 10,000 tropas y cuando los miembros del servicio ya estaban comenzando a dirigirse a la frontera. … Los 500 marines estaban siendo retirados de la misión de la Agencia Federal de Manejo de Emergencias para apoyar la respuesta de incendios forestales de California.
Como advirtió el secretario interino siniestramente: “Esto es solo el comienzo”, un guiño a algo aún más alarmante. El nuevo Secretario de Defensa que supervisa esta operación militar doméstica fue marcada previamente como una amenaza extremista para los ciudadanos estadounidenses, se opuso abiertamente reglas de compromiso en zonas de combate, y trabajó para minimizar el papel de los militares en el ataque del 6 de enero. Su retórica extremista para “restaurar la cultura guerrera” señala una purga planificada de cualquiera que pueda resistir órdenes ilegales contra las poblaciones civiles.
Este no es un ajuste de política menor o una medida temporal, ya que el propio Trump se jacta. Esta es la presa estadounidense que se rompe abruptamente. La administración está construyendo el marco legal completo para tratar Movimiento civil como guerra. Esta es precisamente la crisis constitucional que los fundadores intentaron prevenir separando el poder militar y civil, y por qué el Congreso aprobó la Ley de Comitatus Posse que prohíbe las tropas federales de la policía nacional después de ver el poder militar abusado contra las poblaciones civiles durante la reconstrucción.
Al declarar falsamente la inmigración como una “invasión”, la administración está explotando la promesa de la Sección 4 del Artículo IV de “proteger” a los estados para anular el Posse Comitatus. La Orden Ejecutiva del 22 de enero utiliza esta disposición constitucional para autorizar la acción militar inmediata mientras elimina las protecciones civiles como el asilo. La refundición deliberadamente falsa crea cobertura legal para desplegar unidades de combate para atacar negocios, hogares, escuelas e iglesias para acelerar las deportaciones a punta de pistola, exactamente lo que estas leyes debían prevenir.
Combinado con un secretario de defensa que se opuso a reglas de compromiso y celebra la “cultura guerrera”, esto crea el desastre completo: marco legal, infraestructura militar y estructura de comando para las poblaciones civiles que de repente se convierten en objetivos militares, explícitamente justificados en documentos oficiales apresurados. La administración está golpeando estas piezas en su lugar más rápido de lo que los tribunales pueden responder, lo que significa una erosión estratégica de las barreras entre la policía militar y civil que estaba destinada a proteger la democracia.
La historia nos muestra con una consistencia escalofriante de que la respuesta militarizadora a los civiles mientras los describe de manera fraudulenta como “invasores” militantes precede a las violaciones masivas de los derechos humanos. De las desapariciones de 1982 de Guatemala (“El soldado de la ‘Unidad Especial’ de Ronald Reagan sentenció a 5,160 años de cárcel por asesinato en masa“) A los asesinatos de 1965 de Indonesia a America First, el despliegue de tropas de combate contra los agricultores negros a las cámaras de gas de 1916 de América First para los hispanos y quemando hasta la muerte, cada una siguió el mismo libro de jugadas documentados: Primero viene la retórica de invasión falsa, luego el despliegue militar para la” población de la población ” control “, luego infraestructura de detención de masa para abruptamente desaparecer civiles.
En 1925, Sharpe Dunaway, un empleado de la Gaceta de Arkansas, alegó que los soldados en Elaine habían “cometido un asesinato tras otro con toda la deliberación tranquila en el mundo, ya sea demasiado despiadado para realizar la enormidad de sus crímenes, o demasiado borracho con la luz de la luna para dar un maldito continental “. … La información anecdótica sugiere que las tropas estadounidenses también participan en la tortura de afroamericanos para que confiesen y dan información.
Hoy, estamos viendo estas etapas iniciales exactas: unidades de combate, transporte militar y liderazgo que se dirige ilegalmente a las poblaciones civiles como amenazas militares.
Los titulares ahora describirán la construcción rápida y sistemática de infraestructura militarizada para la detención y deportación de masa, que se construye pieza por pieza a la vista. Reconocer esto como una señal de advertencia de algo mucho peor no es lo suficientemente alarmista por ninguna medida; Es un imperativo moral basado en el precedente histórico. Lo que es diferente hoy es cómo Palantir y su vigilancia doméstica rama peregrine operan algoritmos opacos inseguros de orgoritmo, como si Wall Street leyera “The Trial” de Kafka y pensó que era una guía para las nuevas empresas de unicornio.
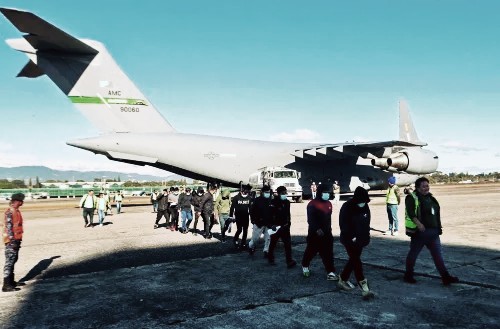
El tiempo para sonar la alarma fue antes de las elecciones, antes de las órdenes ejecutivos, antes de la confirmación del Senado. Todavía existen algunos mecanismos críticos de supervisión, pero quién sabe si se quedará algo: los comités de supervisión del Congreso pueden exigir respuestas sobre despliegues de tropas y operaciones militares en suelo estadounidense. Los soldados pueden rechazar órdenes ilegales. Los fiscales generales estatales retienen la autoridad para impugnar la extralimitación federal. Las organizaciones de derechos civiles aún pueden presentar desafíos legales contra la detención militar. Los periodistas aún tienen protecciones de la Primera Enmienda para documentar y exponer estas operaciones.
La historia preguntará qué hicimos cuando vimos las señales claras. “America First” ha significado durante más de 100 años un terrorismo doméstico generalizado, un frente político para el KKK.
Y, sin embargo, aquí está nuevamente en el escenario federal como si todos lo olviden todo.
Qué supervisión exigimos, qué desafíos presentamos, qué historias documentamos, qué resistencia montamos. La respuesta no puede ser que miramos hacia otro lado mientras la infraestructura para la tragedia racista de los derechos humanos en masa se construyó a la vista, nuevamente.
-
Startups8 meses ago
Remove.bg: La Revolución en la Edición de Imágenes que Debes Conocer
-
Recursos9 meses ago
Cómo Empezar con Popai.pro: Tu Espacio Personal de IA – Guía Completa, Instalación, Versiones y Precios
-
Recursos9 meses ago
Suno.com: La Revolución en la Creación Musical con Inteligencia Artificial
-
Recursos8 meses ago
Perplexity aplicado al Marketing Digital y Estrategias SEO
-
Tutoriales9 meses ago
Cómo Comenzar a Utilizar ChatGPT: Una Guía Completa para Principiantes
-
Estudiar IA8 meses ago
Curso de Inteligencia Artificial de UC Berkeley estratégico para negocios
-
Startups7 meses ago
Startups de IA en EE.UU. que han recaudado más de $100M en 2024
-
Noticias7 meses ago
Dos periodistas octogenarios deman a ChatGPT por robar su trabajo