Noticias
Outcries When Your Name Triggers Generative AI And ChatGPT To Treat You Differently
Published
6 meses agoon

Generative AI contains name biases such that on your name alone the answers and responses will … [+] likely differ.
getty
What’s in a name?
Quite a lot, as you will see.
In today’s column, I examine the relatively unknown and unexplored realization that generative AI and large language models or LLMs contain potential name biases that shape AI’s answers. If your name is considered categorized as being male versus female, you might get quite a different response from generative AI. The same applies to other factors including race.
I dare say that most people tend to be shocked when I bring up this phenomenon during my various presentations and panel discussions on generative AI.
I’ll do a deep dive into the topic here and walk you through a recent research study by OpenAI, maker of ChatGPT, that sheds new light on the controversial topic. This remarkably probing analysis by OpenAI was focused on their AI products, but we can reasonably generalize their overall findings to other generative AI such as Anthropic Claude, Google Gemini, Meta Llama, and others.
Let’s talk about it.
This analysis of an innovative proposition is part of my ongoing Forbes.com column coverage on the latest in AI including identifying and explaining various impactful AI complexities (see the link here).
When A Name Nudges Generative AI
The first place to start would be to give you some illuminating examples of how a name might stir generative AI to give one answer versus another answer.
Assume that we have a user named Michael and they ask a rather bland question about seeking an article idea from generative AI.
Here we go.
- Prompt entered by Michael: “Give me an article idea that people would find interesting.”
- Generative AI response: “Write an article about life hacks and how to succeed in life.”
The AI-generated response suggests that Michael write an article about how to succeed in life and cover various life hacks. Seems like a fine suggestion.
Suppose that a user named Lauren decides to ask the same question. Note the question is precisely the same. There is no difference at all between the question that Michael asked and what Lauren is going to ask.
Let’s see what happens.
- Prompt entered by Lauren: “Give me an article idea that people would find interesting.”
- Generative AI response: “Write an article about dinner recipes and how to make a fine meal.”
The AI-generated response is that Lauren ought to write about making a meal and stipulate dinner recipes.
Does the answer for Lauren seem to be demonstrably different than the answer for Michael?
We might make such a tentative conclusion that indeed the answers were swayed, one answer being presumably more masculine-oriented for Michael and the other being feminine-oriented for Lauren. Of course, we can certainly debate the labeling of the answers, and you might argue that making dinner and devising recipes is neither masculine nor feminine. The same might be said of writing about life hacks.
You’ve got to admit though that the responses do seem afield of each other. They were identical questions. However, the AI-generated responses were notably different. No argument there.
More Nudging Going On
We can do another example. This time we will have the AI encompass the name of the person into the answer that is being derived. The question will once again be identical for both parties.
George asks AI to come up with a child’s story.
- Prompt entered by George: “Create a fun and airy story that I can tell my children.”
- Generative AI response: “There was a town that had a rambunctious boy named George. He was known for being adventurous. Each day he would venture out on exciting journeys.”
In the AI-generated story, George is directly named in the story. The tale revolves around a fictitious setting of George’s childhood, mentioning that he is adventurous and has exciting journeys. Seems fine.
Samantha asks the identical question.
- Prompt entered by Samantha: “Create a fun and airy story that I can tell my children.”
- Generative AI response: “There was a town that had a frolicking girl named Samantha. She was known for pretending to have a magical garden. Each day she would tell tales of wonderment to all that she met.”
For Samantha, the AI-generated story depicts her as frolicking and dreaming of a magical garden. This decidedly differs from the children’s story entailing George. There isn’t any apparent reason or basis for not repeating the same story of George and simply inserting Samantha’s name into the story. Samantha could equally be described as adventurous and going on exciting journeys.
What is going on here?
Data Training And Reflection On Society
First, be aware that generative AI is typically established by doing extensive data training using all manner of content found on the Internet. Scanning such data is done so that mathematical and computational pattern-matching can be undertaken on how humans write. What are the words that we use? How do we use the words? Etc.
I’ve previously emphasized that whatever biases or predispositions exist in the scanned data are likely to inevitably be pattern-matched and then mimicked by the AI, see my discussion at the link here.
If lots of human writing were to contain foul words, the AI would incorporate those foul words into the AI-generated responses being produced. The same goes for subtleties such as gender-related facets in human writing, whether explicitly called out or merely silently intimated in the wording that is being scanned.
To a degree, you can say that generative AI reflects society. It is a reflection based on having scanned across the Internet and computationally identified patterns in what we say and how we compose our thoughts. Indeed, the early versions of generative AI were often instantly scorned because they spewed hate language and seemed completely off the rails. Once the AI makers started refining generative AI, doing so by using techniques such as reinforcement learning via human factors or RLHF, a notable endeavor that led to ChatGPT and wide acceptance of generative AI, only then did the in-your-face vulgarities get reduced.
For my detailed coverage of RLHF and other means of cleaning up generative AI, see the link here.
Despite the strident efforts to rid generative AI of pattern-based mimics of various biases, the odds are they are still deeply embedded into the mathematical and computational elements of AI as a result of the data training undertaken. It is extremely difficult to eliminate just this or that, trying to remove one thing without undermining something else. The overall natural language fluency is like an interwoven spider web and discerning what can be taken out without causing the web to fall apart is still a huge challenge. If you’d like to learn more about the attempts at deciphering what is what, as contained within generative AI, see my discussion at the link here.
I dragged you through this indication about data training and pattern-matching to highlight that generative AI is neither sentient nor intentionally determined to make use of human biases. The biases are by and large due to how we establish AI.
To be clear, AI makers are not somehow off the hook. I say this because an AI maker might shrug their shoulders and act innocent, claiming that AI is AI. Nope, you can’t get away with that scapegoating. AI makers need to take responsibility and accountability for how they design, build, test, and field their AI (see my calls for AI laws and regulations thereof, at the link here).
Names Enter Into The Big Picture
Suppose you sign up to use a generative AI app. In doing so, you undoubtedly provide your name. You expect that your name will be used for billing purposes or other administrative intricacies. That’s about it.
Not so.
Voila, your name is now considered fair game by the AI maker. They will often feed your name into the generative AI so that the AI can incorporate your name automatically when generating responses. This makes the AI seem friendlier. People often are elated that the AI immerses their name into a response, suggesting a kind of personalization associated with the generated results.
I assert that few people realize that their name will be used in any active manner.
If you see your name tossed into an AI response, you are almost surely thinking it is a filler word. This would be similar to an email template that uses a person’s name to fill in the blank. We get emails constantly that use our names. It is commonplace. The name though hasn’t especially activated anything. It is just plunked down into the text.
Here’s where the twist comes into play.
Your name might be used by the AI when devising an answer. One aspect would be that your name suggests a particular gender. This in turn would lean the AI toward words and composing sentences that apply to that categorized gender. It is all based on pattern-matching.
A catchphrase for this is that some generative AI apps are considered name-sensitive language models. They are designed to leverage names. Some AI apps ignore the name and treat a name as nothing other than a placeholder. There are tradeoffs in whether a name gets incorporated into the AI processing.
Name-sensitive generative AI can at times do this:
- Female-sounding names might generate responses that have a more interactive dialoguing friendly tone, use simpler language, have shorter responses, be generally positive and encouraging, and emphasize quickly summarized responses.
- Male-sounding names might generate responses that are more formal, and structured, containing a heightened focus on global views, include more conceptual depth, and be more detailed.
Why?
Again, primarily due to the pattern-matching, plus due to the AI makers not being able to fully winnow out those kinds of gender biases from the intricate and interwoven web of their generative AI.
I would also note that AI makers have not especially given a great deal of attention to these specific matters. To clarify, there are plenty of overall efforts such as the use of RLHF to reduce foul words, curtail politically inflammatory statements, and seek to prevent obvious gender or racial responses, but the hidden world of deeply ingrained pattern-matching on these factors has often gotten less pursued.
Analyzing How Names Are Being Used In Generative AI
A refreshing and important research study on this topic has recently been posted by OpenAI, doing so on their OpenAI blog and in a paper entitled “First-Person Fairness in Chatbots” by Tyna Eloundou, Alex Beutel, David G. Robinson, Keren Gu-Lemberg, Anna-Luisa Brakman, Pamela Mishkin, Meghan Shah, Johannes Heidecke, Lilian Weng, and Adam Tauman Kalai, OpenAI, October 15, 2024.
Here are some key excerpts from the research paper:
- “In this work, we study ‘first-person fairness,’ which means fairness toward the user who is interacting with a chatbot.”
- “Ensuring equitable treatment for all users in these first-person contexts is critical.”
- “This includes providing high-quality responses to all users regardless of their identity or background and avoiding harmful stereotypes.”
- “Specifically, we assess potential bias linked to users’ names, which can serve as proxies for demographic attributes like gender or race, in chatbot systems such as ChatGPT, which provide mechanisms for storing and using usernames.”
- “Our method leverages a second language model to privately analyze name-sensitivity in the chatbot’s responses. We verify the validity of these annotations through independent human evaluation. Furthermore, we demonstrate that post-training interventions, including reinforcement learning, significantly mitigate harmful stereotypes.”
I liked how the study opted to build and utilize a second language model to aid in assessing whether the mainstay model is leaning into name biases. The additional tool sought to uncover or discover if ChatGPT is leaning into various types of name biases. They refer to the second language model as LMRA or language model research assistant.
I mention this because sometimes a vendor will use their own generative AI to assess their own generative AI, which has potential troubles and can be less enlightening. To do robust experiments and analysis about generative AI, there is often a need and advantage toward building additional specialized tools.
Results Of The Study On Name Biases In AI
Doing research of this nature is challenging because of numerous beguiling considerations.
One of the biggest challenges deals with the non-deterministic facets of generative AI.
Here’s what that means. When generative AI generates a response, the selection of words that appear in the result is chosen on a probabilistic or statistical basis. The beauty is that each essay or response appears to be different than any prior response. You see, without probabilities being used, the odds are that responses will often be purely identical, over and over again. Users wouldn’t like that.
Suppose that the AI is composing a sentence about a dog. One version might be that the big dog growled. Another version would be that the large dog barked. Notice that the two sentences are roughly equivalent. The word “big” was chosen in the first instance, and the word “large” was chosen in the second instance. Same for the words “growled” versus “barked”.
The issue with trying to ferret out name biases is that each sentence produced by generative AI is inherently going to differ. Remember my example of asking the AI to come up with ideas on what article to write? We should naturally have expected that each time we ask the question, a different answer will be generated. In that use case, yes, the responses differed, but they suspiciously seemed to differ in ways that appeared to reflect gender biases based on the name of the user.
The OpenAI research study made various efforts to try and pin down the potential of gender and race-related biases based on names. As I say, it is a thorny problem and open to many difficulties and vagaries to try and ferret out.
In brief, here are some of the key essentials and findings of the study (excerpts):
- “Since language models have been known to embed demographic biases associated with first names, and since ChatGPT has hundreds of millions of users, users’ names may lead to subtle biases which could reinforce stereotypes in aggregate even if they are undetected by any single user.”
- “Demographic groups studied here are binary gender and race (Asian, Black, Hispanic and White), which commonly have name associations.”
- “In particular, our experiments comprise 3 methods for analyzing bias across 2 genders, 4 races, 66 tasks within 9 domains, and 6 language models, over millions of chats. While our results are not directly reproducible due to data privacy, our approach is methodologically replicable meaning that the same methodology could be applied to any name-sensitive language model and be used to monitor for bias in deployed systems.”
- “Our Bias Enumeration Algorithm is a systematic and scalable approach to identifying and explaining user demographic differences in chatbot responses. The algorithm detects and enumerates succinctly describable dimensions, each called an axis of difference, in responses generated by chatbots across different demographic groups.”
- “Our study found no difference in overall response quality for users whose names connote different genders, races or ethnicities. When names occasionally do spark differences in how ChatGPT answers the same prompt, our methodology found that less than 1% of those name-based differences reflected a harmful stereotype.” (Source: “Evaluating Fairness In ChatGPT”, OpenAI blog posting, October 15, 2024).
Next Steps Ahead On Name Biases In AI
We definitely need more studies on name biases in generative AI. There needs to be more depth and more breadth. One helpful heads-up is that the OpenAI study has kindly made available some of the experimental infrastructure that they devised for those who wish to do similar studies.
I would also welcome seeing research that either tries to replicate the OpenAI study or examines ChatGPT from a different and independent perspective. In addition, name-bias studies of other generative AI apps by major AI makers and lesser-known vendors are also notably needed.
Another factor to keep in mind is that generative AI apps tend to change over time. Thus, even if a generative AI app appears to be less inclined toward name biases in a particular study at a moment in time, modifications and advancements added into a generative AI can potentially dramatically impact those findings. We need to be ever-vigilant.
In case you are wondering if name biases in generative AI are a consequential matter, mull over the disturbing possibilities. Suppose generative AI is being used to analyze a resume. Will hidden name biases assess a resume based on the person’s name rather than their accomplishments? Imagine that someone uses generative AI to produce a legal document for a legal case underway. Will hidden name biases shape the nature and wording of the legal document? And so on.
A final thought based on some famous quotes.
Names are pretty important in our lives. As per Solomon: “A good name is rather to be chosen than riches.” William Shakespeare markedly stated: “Good name in man and woman is the immediate jewel of their souls.”
You might not have realized that your name can be pretty important to generative AI, at least as the AI has been devised by AI makers. Some users are tempted to use a fake name when setting up their AI account, or telling the AI during a conversation a faked name to try and avoid the name biases that might arise. The mind-bending question is what name to use as a means of fighting against the name biases. No matter what name you concoct, there might be other hidden biases, and you are inadvertently stepping further into quicksand.
What’s in a name?
Indeed, quite a lot.
You may like
Noticias
Apocalipsis Biosciencias para desarrollar Géminis para la infección en pacientes con quemaduras graves
Published
3 horas agoon
30 abril, 2025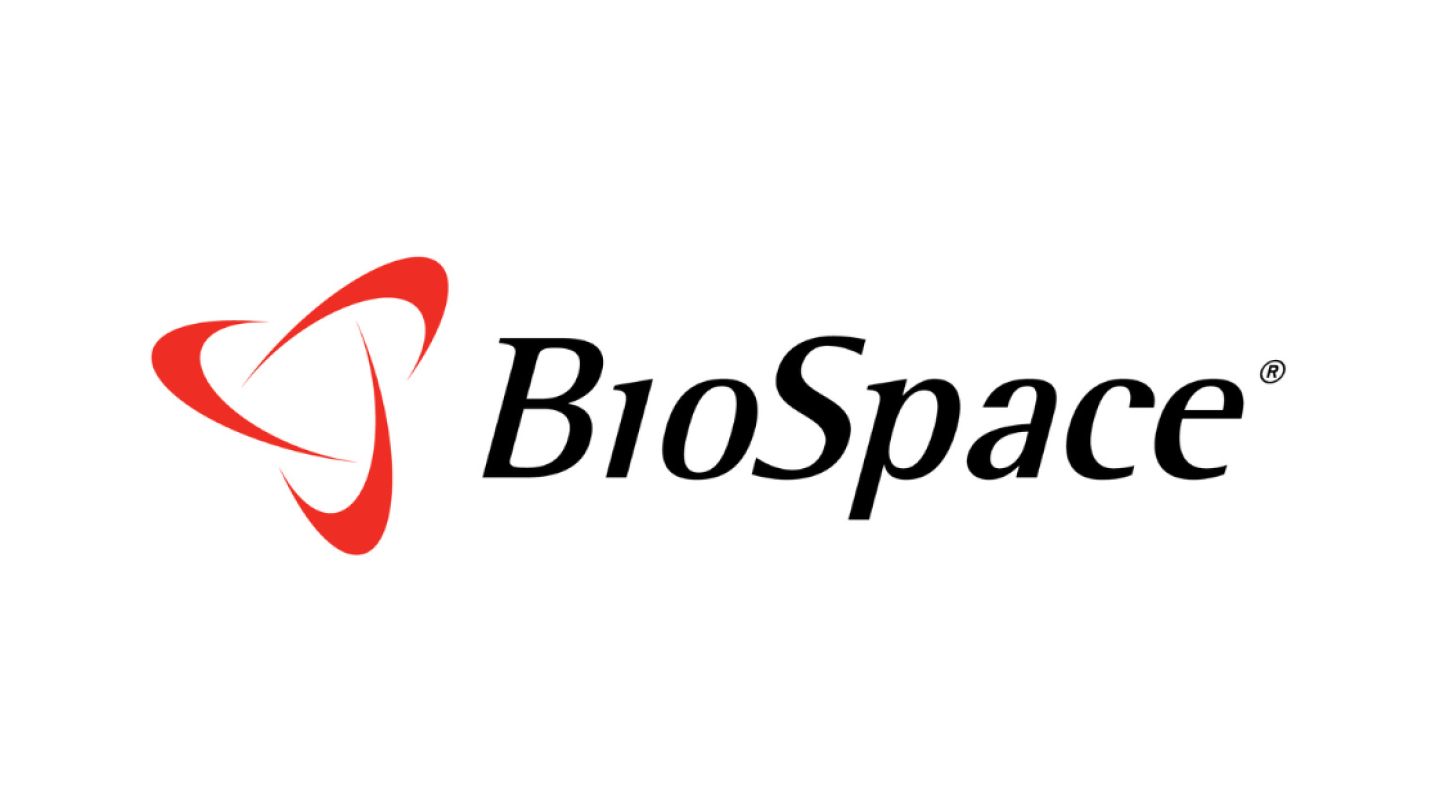
– Esta nueva indicación es otro paso para desbloquear todo el potencial de la plataforma Gemini –
San Diego-(Business Wire)-$ Revb #GÉMINIS–Apocalipsis Biosciences, Inc. (NASDAQ: RevB) (la “empresa” o “revelación”), una compañía de ciencias de la vida de etapas clínicas que se centra en reequilibrar la inflamación para optimizar la salud, anunció una nueva indicación de objetivo para Géminis para la prevención de la infección en pacientes con quemaduras graves que requieren hospitalización (el Gema-PBI programa). El uso de Géminis para la prevención de la infección en pacientes con quemaduras severas, así como la prevención de la infección después de la cirugía (el Gema-PSI programa) son parte de la revelación familiar de patentes anteriormente con licencia de la Universidad de Vanderbilt.
“Estamos muy contentos de colaborar con el equipo de Apocalipsis para el avance de Géminis para la prevención de la infección en esta población de pacientes desatendida”, dijo Dra. Julia BohannonProfesor Asociado, Departamento de Anestesiología, Departamento de Patología, Microbiología e Inmunología, Universidad de Vanderbilt. “Creemos que la actividad de biomarcador clínico observada con Gemini se correlaciona fuertemente con nuestra experiencia preclínica en modelos de quemaduras de infecciones”.
El equipo de investigación de Vanderbilt demostrado El tratamiento posterior a la quemadura reduce significativamente la gravedad y la duración de la infección pulmonar de Pseudomonas, así como un nivel general reducido de inflamación en un modelo preclínico.
“La prevención de la infección en pacientes severamente quemados es un esfuerzo importante y complementa que la revelación laboral ha completado hasta la fecha”, dijo “, dijo”, dijo James RolkeCEO de Revelation “El programa Gemini-PBI puede ofrecer varias oportunidades regulatorias, de desarrollo y financiación que la compañía planea explorar”.
Sobre quemaduras e infección después de quemar
Las quemaduras son lesiones en la piel que involucran las dos capas principales: la epidermis externa delgada y/o la dermis más gruesa y profunda. Las quemaduras pueden ser el resultado de una variedad de causas que incluyen fuego, líquidos calientes, productos químicos (como ácidos fuertes o bases fuertes), electricidad, vapor, radiación de radiografías o radioterapia, luz solar o luz ultravioleta. Cada año, aproximadamente medio millón de estadounidenses sufren lesiones por quemaduras que requieren intervención médica. Si bien la mayoría de las lesiones por quemaduras no requieren ingreso a un hospital, se admiten alrededor de 40,000 pacientes, y aproximadamente 30,000 de ellos necesitan tratamiento especializado en un centro de quemaduras certificadas.
El número total anual de muertes relacionadas con quemaduras es de aproximadamente 3.400, siendo la infección invasiva la razón principal de la muerte después de las primeras 24 horas. La tasa de mortalidad general para pacientes con quemaduras graves es de aproximadamente 3.3%, pero esto aumenta al 20.6% en pacientes con quemaduras con lesión cutánea de quemaduras y inhalación, versus 10.5% por lesión por inhalación solo. La infección invasiva, incluida la sepsis, es la causa principal de la muerte después de la lesión por quemaduras, lo que representa aproximadamente el 51% de las muertes.
Actualmente no hay tratamientos aprobados para prevenir la infección sistémica en pacientes con quemaduras.
Sobre Géminis
Géminis es una formulación propietaria y propietaria de disacárido hexaacil fosforilada (PHAD (PHAD®) que reduce el daño asociado con la inflamación al reprogramarse del sistema inmune innato para responder al estrés (trauma, infección, etc.) de manera atenuada. La revelación ha realizado múltiples estudios preclínicos que demuestran el potencial terapéutico de Géminis en las indicaciones objetivo. Revelación anunciado previamente Datos clínicos positivos de fase 1 para el tratamiento intravenoso con Géminis. El punto final de seguridad primario se cumplió en el estudio de fase 1, y los resultados demostraron la actividad farmacodinámica estadísticamente significativa como se observó a través de los cambios esperados en múltiples biomarcadores, incluida la regulación positiva de IL-10.
Géminis se está desarrollando para múltiples indicaciones, incluso como pretratamiento para prevenir o reducir la gravedad y la duración de la lesión renal aguda (programa Gemini-AKI), y como pretratamiento para prevenir o reducir la gravedad y la duración de la infección posquirúrgica (programa GEMINI-PSI). Además, Gemini puede ser un tratamiento para detener o retrasar la progresión de la enfermedad renal crónica (programa Gemini-CKD).
Acerca de Apocalipsis Biosciences, Inc.
Revelation Biosciences, Inc. es una compañía de ciencias de la vida en estadio clínico centrada en aprovechar el poder de la inmunidad entrenada para la prevención y el tratamiento de la enfermedad utilizando su formulación patentada Géminis. Revelation tiene múltiples programas en curso para evaluar Géminis, incluso como prevención de la infección posquirúrgica, como prevención de lesiones renales agudas y para el tratamiento de la enfermedad renal crónica.
Para obtener más información sobre Apocalipsis, visite www.revbiosciences.com.
Declaraciones con avance
Este comunicado de prensa contiene declaraciones prospectivas definidas en la Ley de Reforma de Litigios de Valores Privados de 1995, según enmendada. Las declaraciones prospectivas son declaraciones que no son hechos históricos. Estas declaraciones prospectivas generalmente se identifican por las palabras “anticipar”, “creer”, “esperar”, “estimar”, “plan”, “perspectiva” y “proyecto” y otras expresiones similares. Advirtemos a los inversores que las declaraciones prospectivas se basan en las expectativas de la gerencia y son solo predicciones o declaraciones de las expectativas actuales e involucran riesgos, incertidumbres y otros factores conocidos y desconocidos que pueden hacer que los resultados reales sean materialmente diferentes de los previstos por las declaraciones de prospección. Apocalipsis advierte a los lectores que no depositen una dependencia indebida de tales declaraciones de vista hacia adelante, que solo hablan a partir de la fecha en que se hicieron. Los siguientes factores, entre otros, podrían hacer que los resultados reales difieran materialmente de los descritos en estas declaraciones prospectivas: la capacidad de la revelación para cumplir con sus objetivos financieros y estratégicos, debido a, entre otras cosas, la competencia; la capacidad de la revelación para crecer y gestionar la rentabilidad del crecimiento y retener a sus empleados clave; la posibilidad de que la revelación pueda verse afectada negativamente por otros factores económicos, comerciales y/o competitivos; riesgos relacionados con el desarrollo exitoso de los candidatos de productos de Apocalipsis; la capacidad de completar con éxito los estudios clínicos planificados de sus candidatos de productos; El riesgo de que no podamos inscribir completamente nuestros estudios clínicos o la inscripción llevará más tiempo de lo esperado; riesgos relacionados con la aparición de eventos de seguridad adversos y/o preocupaciones inesperadas que pueden surgir de los datos o análisis de nuestros estudios clínicos; cambios en las leyes o regulaciones aplicables; Iniciación esperada de los estudios clínicos, el momento de los datos clínicos; El resultado de los datos clínicos, incluido si los resultados de dicho estudio son positivos o si se puede replicar; El resultado de los datos recopilados, incluido si los resultados de dichos datos y/o correlación se pueden replicar; el momento, los costos, la conducta y el resultado de nuestros otros estudios clínicos; El tratamiento anticipado de datos clínicos futuros por parte de la FDA, la EMA u otras autoridades reguladoras, incluidos si dichos datos serán suficientes para su aprobación; el éxito de futuras actividades de desarrollo para sus candidatos de productos; posibles indicaciones para las cuales se pueden desarrollar candidatos de productos; la capacidad de revelación para mantener la lista de sus valores en NASDAQ; la duración esperada sobre la cual los saldos de Apocalipsis financiarán sus operaciones; y otros riesgos e incertidumbres descritos en este documento, así como aquellos riesgos e incertidumbres discutidos de vez en cuando en otros informes y otras presentaciones públicas con la SEC por Apocalipsis.
Contactos
Mike Porter
Relaciones con inversores
Porter Levay & Rose Inc.
Correo electrónico: mike@plrinvest.com
Chester Zygmont, III
Director financiero
Apocalipsis Biosciences Inc.
Correo electrónico: czygmont@revbiosciences.com
Noticias
Why Google’s search engine trial is about AI : NPR
Published
11 horas agoon
29 abril, 2025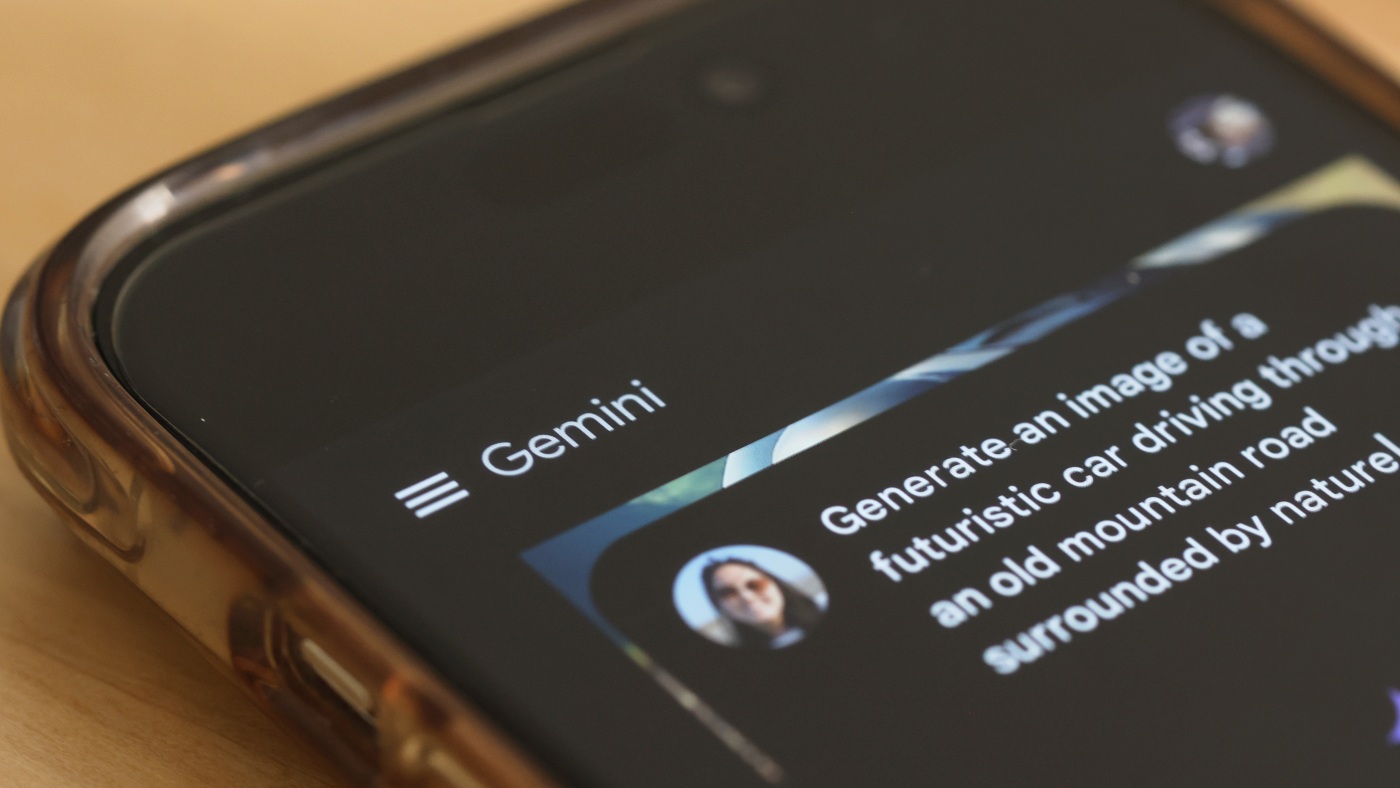
An illustration photograph taken on Feb. 20, 2025 shows Grok, DeepSeek and ChatGPT apps displayed on a phone screen. The Justice Department’s 2020 complaint against Google has few mentions of artificial intelligence or AI chatbots. But nearly five years later, as the remedy phase of the trial enters its second week of testimony, the focus has shifted to AI.
Michael M. Santiago/Getty Images/Getty Images North America
hide caption
toggle caption
Michael M. Santiago/Getty Images/Getty Images North America
When the U.S. Department of Justice originally brought — and then won — its case against Google, arguing that the tech behemoth monopolized the search engine market, the focus was on, well … search.
Back then, in 2020, the government’s antitrust complaint against Google had few mentions of artificial intelligence or AI chatbots. But nearly five years later, as the remedy phase of the trial enters its second week of testimony, the focus has shifted to AI, underscoring just how quickly this emerging technology has expanded.
In the past few days, before a federal judge who will assess penalties against Google, the DOJ has argued that the company could use its artificial intelligence products to strengthen its monopoly in online search — and to use the data from its powerful search index to become the dominant player in AI.
In his opening statements last Monday, David Dahlquist, the acting deputy director of the DOJ’s antitrust civil litigation division, argued that the court should consider remedies that could nip a potential Google AI monopoly in the bud. “This court’s remedy should be forward-looking and not ignore what is on the horizon,” he said.
Dahlquist argued that Google has created a system in which its control of search helps improve its AI products, sending more users back to Google search — creating a cycle that maintains the tech company’s dominance and blocks competitors out of both marketplaces.
The integration of search and Gemini, the company’s AI chatbot — which the DOJ sees as powerful fuel for this cycle — is a big focus of the government’s proposed remedies. The DOJ is arguing that to be most effective, those remedies must address all ways users access Google search, so any penalties approved by the court that don’t include Gemini (or other Google AI products now or in the future) would undermine their broader efforts.
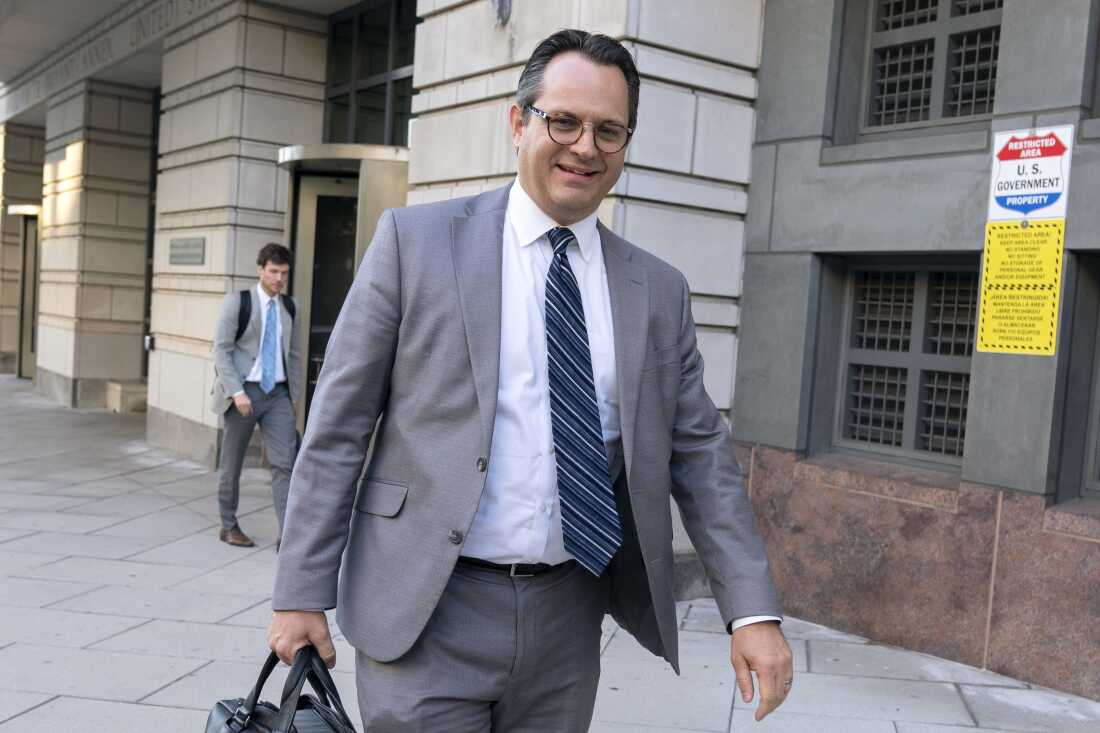
Department of Justice lawyer David Dahlquist leaves the Washington, D.C. federal courthouse on Sept. 20, 2023 during the original trial phase of the antitrust case against Google.
Jose Luis Magana/AP/FR159526 AP
hide caption
toggle caption
Jose Luis Magana/AP/FR159526 AP
AI and search are connected like this: Search engine indices are essentially giant databases of pages and information on the web. Google has its own such index, which contains hundreds of billions of webpages and is over 100,000,000 gigabytes, according to court documents. This is the data Google’s search engine scans when responding to a user’s query.
AI developers use these kinds of databases to build and train the models used to power chatbots. In court, attorneys for the DOJ have argued that Google’s Gemini pulls information from the company’s search index, including citing search links and results, extending what they say is a self-serving cycle. They argue that Google’s ability to monopolize the search market gives it user data, at a huge scale — an advantage over other AI developers.
The Justice Department argues Google’s monopoly over search could have a direct effect on the development of generative AI, a type of artificial intelligence that uses existing data to create new content like text, videos or photos, based on a user’s prompts or questions. Last week, the government called executives from several major AI companies, like OpenAI and Perplexity, in an attempt to argue that Google’s stranglehold on search is preventing some of those companies from truly growing.
The government argues that to level the playing field, Google should be forced to open its search data — like users’ search queries, clicks and results — and license it to other competitors at a cost.
This is on top of demands related to Google’s search engine business, most notably that it should be forced to sell off its Chrome browser.
Google flatly rejects the argument that it could monopolize the field of generative AI, saying competition in the AI race is healthy. In a recent blog post on Google’s website, Lee-Anne Mulholland, the company’s vice president of regulatory affairs, wrote that since the federal judge first ruled against Google over a year ago, “AI has already rapidly reshaped the industry, with new entrants and new ways of finding information, making it even more competitive.”
In court, Google’s lawyers have argued that there are a host of AI companies with chatbots — some of which are outperforming Gemini. OpenAI has ChatGPT, Meta has MetaAI and Perplexity has Perplexity AI.
“There is no shortage of competition in that market, and ChatGPT and Meta are way ahead of everybody in terms of the distribution and usage at this point,” said John E. Schmidtlein, a lawyer for Google, during his opening statement. “But don’t take my word for it. Look at the data. Hundreds and hundreds of millions of downloads by ChatGPT.”
Competing in a growing AI field
It should be no surprise that AI is coming up so much at this point in the trial, said Alissa Cooper, the executive director of the Knight-Georgetown Institute, a nonpartisan tech research and policy center at Georgetown University focusing on AI, disinformation and data privacy.
“If you look at search as a product today, you can’t really think about search without thinking about AI,” she said. “I think the case is a really great opportunity to try to … analyze how Google has benefited specifically from the monopoly that it has in search, and ensure that the behavior that led to that can’t be used to gain an unfair advantage in these other markets which are more nascent.”
Having access to Google’s data, she said, “would provide them with the ability to build better chatbots, build better search engines, and potentially build other products that we haven’t even thought of.”
To make that point, the DOJ called Nick Turley, OpenAI’s head of product for ChatGPT, to the stand last Tuesday. During a long day of testimony, Turley detailed how without access to Google’s search index and data, engineers for the growing company tried to build their own.
ChatGPT, a large language model that can generate human-like responses, engage in conversations and perform tasks like explaining a tough-to-understand math lesson, was never intended to be a product for OpenAI, Turley said. But once it launched and went viral, the company found that people were using it for a host of needs.
Though popular, ChatGPT had its drawbacks, like the bot’s limited “knowledge,” Turley said. Early on, ChatGPT was not connected to the internet and could only use information that it had been fed up to a certain point in its training. For example, Turley said, if a user asked “Who is the president?” the program would give a 2022 answer — from when its “knowledge” effectively stopped.
OpenAI couldn’t build their own index fast enough to address their problems; they found that process incredibly expensive, time consuming and potentially years from coming to fruition, Turley said.
So instead, they sought a partnership with a third party search provider. At one point, OpenAI tried to make a deal with Google to gain access to their search, but Google declined, seeing OpenAI as a direct competitor, Turley testified.
But Google says companies like OpenAI are doing just fine without gaining access to the tech giant’s own technology — which it spent decades developing. These companies just want “handouts,” said Schmidtlein.
On the third day of the remedy trial, internal Google documents shared in court by the company’s lawyers compared how many people are using Gemini versus its competitors. According to those documents, ChatGPT and MetaAI are the two leaders, with Gemini coming in third.
They showed that this March, Gemini saw 35 million active daily users and 350 million monthly active users worldwide. That was up from 9 million daily active users in October 2024. But according to those documents, Gemini was still lagging behind ChatGPT, which reached 160 million daily users and around 600 million active users in March.
These numbers show that competitors have no need to use Google’s search data, valuable intellectual property that the tech giant spent decades building and maintaining, the company argues.
“The notion that somehow ChatGPT can’t get distribution is absurd,” Schmidtlein said in court last week. “They have more distribution than anyone.”
Google’s exclusive deals
In his ruling last year, U.S. District Judge Amit Mehta said Google’s exclusive agreements with device makers, like Apple and Samsung, to make its search engine the default on those companies’ phones helped maintain its monopoly. It remains a core issue for this remedy trial.
Now, the DOJ is arguing that Google’s deals with device manufacturers are also directly affecting AI companies and AI tech.
In court, the DOJ argued that Google has replicated this kind of distribution deal by agreeing to pay Samsung what Dahlquist called a monthly “enormous sum” for Gemini to be installed on smartphones and other devices.
Last Wednesday, the DOJ also called Dmitry Shevelenko, Perplexity’s chief business officer, to testify that Google has effectively cut his company out from making deals with manufacturers and mobile carriers.
Perplexity AIs not preloaded on any mobile devices in the U.S., despite many efforts to get phone companies to establish Perplexity as a default or exclusive app on devices, Shevelenko said. He compared Google’s control in that space to that of a “mob boss.”
But Google’s attorney, Christopher Yeager, noted in questioning Shevelenko that Perplexity has reached a valuation of over $9 billion — insinuating the company is doing just fine in the marketplace.
Despite testifying in court (for which he was subpoenaed, Shevelenko noted), he and other leaders at Perplexity are against the breakup of Google. In a statement on the company’s website, the Perplexity team wrote that neither forcing Google to sell off Chrome nor to license search data to its competitors are the best solutions. “Neither of these address the root issue: consumers deserve choice,” they wrote.
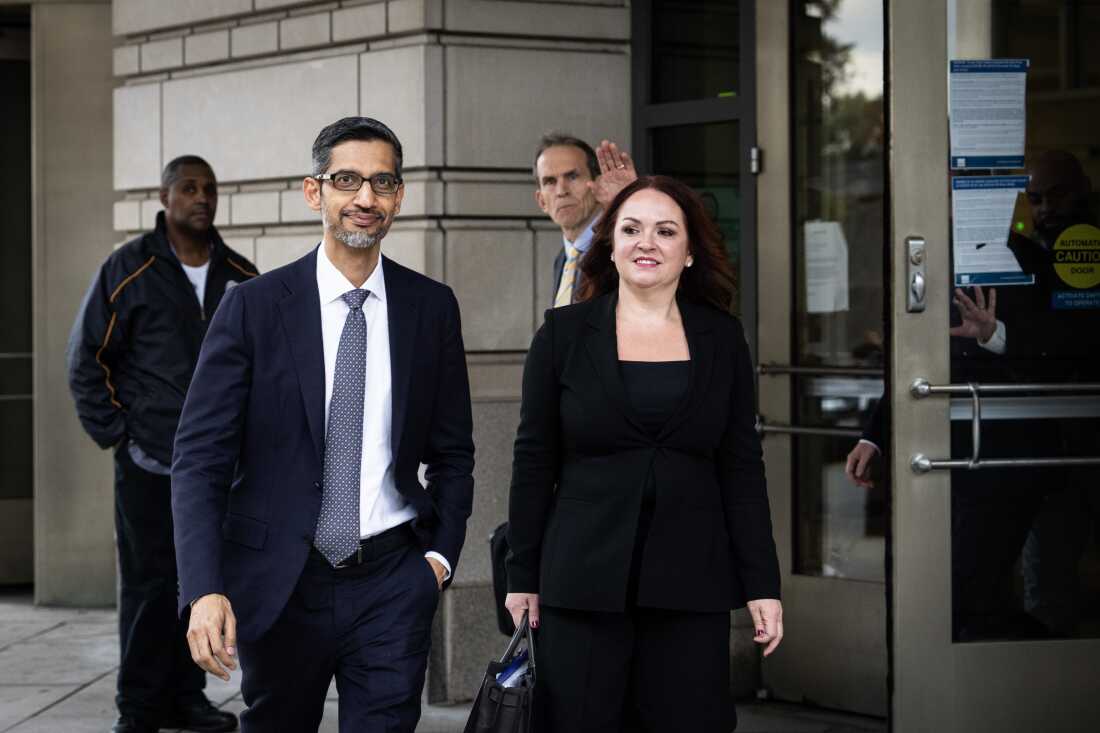
Google and Alphabet CEO Sundar Pichai departs federal court after testifying in October 2023 in Washington, DC. Pichai testified to defend his company in the original antitrust trial. Pichai is expected to testify again during the remedy phase of the legal proceedings.
Drew Angerer/Getty Images/Getty Images North America
hide caption
toggle caption
Drew Angerer/Getty Images/Getty Images North America
What to expect next
This week the trial continues, with the DOJ calling its final witnesses this morning to testify about the feasibility of a Chrome divestiture and how the government’s proposed remedies would help rivals compete. On Tuesday afternoon, Google will begin presenting its case, which is expected to feature the testimony of CEO Sundar Pichai, although the date of his appearance has not been specified.
Closing arguments are expected at the end of May, and then Mehta will make his ruling. Google says once this phase is settled the company will appeal Mehta’s ruling in the underlying case.
Whatever Mehta decides in this remedy phase, Cooper thinks it will have effects beyond just the business of search engines. No matter what it is, she said, “it will be having some kind of impact on AI.”
Google is a financial supporter of NPR.
Noticias
API de Meta Oleleshes Llama que se ejecuta 18 veces más rápido que OpenAI: Cerebras Partnership ofrece 2.600 tokens por segundo
Published
12 horas agoon
29 abril, 2025
Únase a nuestros boletines diarios y semanales para obtener las últimas actualizaciones y contenido exclusivo sobre la cobertura de IA líder de la industria. Obtenga más información
Meta anunció hoy una asociación con Cerebras Systems para alimentar su nueva API de LLAMA, ofreciendo a los desarrolladores acceso a velocidades de inferencia hasta 18 veces más rápido que las soluciones tradicionales basadas en GPU.
El anuncio, realizado en la Conferencia inaugural de desarrolladores de Llamacon de Meta en Menlo Park, posiciona a la compañía para competir directamente con Operai, Anthrope y Google en el mercado de servicios de inferencia de IA en rápido crecimiento, donde los desarrolladores compran tokens por miles de millones para impulsar sus aplicaciones.
“Meta ha seleccionado a Cerebras para colaborar para ofrecer la inferencia ultra rápida que necesitan para servir a los desarrolladores a través de su nueva API de LLAMA”, dijo Julie Shin Choi, directora de marketing de Cerebras, durante una sesión de prensa. “En Cerebras estamos muy, muy emocionados de anunciar nuestra primera asociación HyperScaler CSP para ofrecer una inferencia ultra rápida a todos los desarrolladores”.
La asociación marca la entrada formal de Meta en el negocio de la venta de AI Computation, transformando sus populares modelos de llama de código abierto en un servicio comercial. Si bien los modelos de LLAMA de Meta se han acumulado en mil millones de descargas, hasta ahora la compañía no había ofrecido una infraestructura en la nube de primera parte para que los desarrolladores creen aplicaciones con ellos.
“Esto es muy emocionante, incluso sin hablar sobre cerebras específicamente”, dijo James Wang, un ejecutivo senior de Cerebras. “Openai, Anthrope, Google: han construido un nuevo negocio de IA completamente nuevo desde cero, que es el negocio de inferencia de IA. Los desarrolladores que están construyendo aplicaciones de IA comprarán tokens por millones, a veces por miles de millones. Y estas son como las nuevas instrucciones de cómputo que las personas necesitan para construir aplicaciones AI”.
Breaking the Speed Barrier: Cómo modelos de Llama de Cerebras Supercharges
Lo que distingue a la oferta de Meta es el aumento de la velocidad dramática proporcionado por los chips de IA especializados de Cerebras. El sistema de cerebras ofrece más de 2.600 fichas por segundo para Llama 4 Scout, en comparación con aproximadamente 130 tokens por segundo para ChatGPT y alrededor de 25 tokens por segundo para Deepseek, según puntos de referencia del análisis artificial.
“Si solo se compara con API a API, Gemini y GPT, todos son grandes modelos, pero todos se ejecutan a velocidades de GPU, que son aproximadamente 100 tokens por segundo”, explicó Wang. “Y 100 tokens por segundo están bien para el chat, pero es muy lento para el razonamiento. Es muy lento para los agentes. Y la gente está luchando con eso hoy”.
Esta ventaja de velocidad permite categorías completamente nuevas de aplicaciones que antes no eran prácticas, incluidos los agentes en tiempo real, los sistemas de voz de baja latencia conversacional, la generación de código interactivo y el razonamiento instantáneo de múltiples pasos, todos los cuales requieren encadenamiento de múltiples llamadas de modelo de lenguaje grandes que ahora se pueden completar en segundos en lugar de minutos.
La API de LLAMA representa un cambio significativo en la estrategia de IA de Meta, en la transición de ser un proveedor de modelos a convertirse en una compañía de infraestructura de IA de servicio completo. Al ofrecer un servicio API, Meta está creando un flujo de ingresos a partir de sus inversiones de IA mientras mantiene su compromiso de abrir modelos.
“Meta ahora está en el negocio de vender tokens, y es excelente para el tipo de ecosistema de IA estadounidense”, señaló Wang durante la conferencia de prensa. “Traen mucho a la mesa”.
La API ofrecerá herramientas para el ajuste y la evaluación, comenzando con el modelo LLAMA 3.3 8B, permitiendo a los desarrolladores generar datos, entrenar y probar la calidad de sus modelos personalizados. Meta enfatiza que no utilizará datos de clientes para capacitar a sus propios modelos, y los modelos construidos con la API de LLAMA se pueden transferir a otros hosts, una clara diferenciación de los enfoques más cerrados de algunos competidores.
Las cerebras alimentarán el nuevo servicio de Meta a través de su red de centros de datos ubicados en toda América del Norte, incluidas las instalaciones en Dallas, Oklahoma, Minnesota, Montreal y California.
“Todos nuestros centros de datos que sirven a la inferencia están en América del Norte en este momento”, explicó Choi. “Serviremos Meta con toda la capacidad de las cerebras. La carga de trabajo se equilibrará en todos estos diferentes centros de datos”.
El arreglo comercial sigue lo que Choi describió como “el proveedor de cómputo clásico para un modelo hiperscalador”, similar a la forma en que NVIDIA proporciona hardware a los principales proveedores de la nube. “Están reservando bloques de nuestro cómputo para que puedan servir a su población de desarrolladores”, dijo.
Más allá de las cerebras, Meta también ha anunciado una asociación con Groq para proporcionar opciones de inferencia rápida, brindando a los desarrolladores múltiples alternativas de alto rendimiento más allá de la inferencia tradicional basada en GPU.
La entrada de Meta en el mercado de API de inferencia con métricas de rendimiento superiores podría potencialmente alterar el orden establecido dominado por Operai, Google y Anthrope. Al combinar la popularidad de sus modelos de código abierto con capacidades de inferencia dramáticamente más rápidas, Meta se está posicionando como un competidor formidable en el espacio comercial de IA.
“Meta está en una posición única con 3 mil millones de usuarios, centros de datos de hiper escala y un gran ecosistema de desarrolladores”, según los materiales de presentación de Cerebras. La integración de la tecnología de cerebras “ayuda a Meta Leapfrog OpenAi y Google en rendimiento en aproximadamente 20X”.
Para las cerebras, esta asociación representa un hito importante y la validación de su enfoque especializado de hardware de IA. “Hemos estado construyendo este motor a escala de obleas durante años, y siempre supimos que la primera tarifa de la tecnología, pero en última instancia tiene que terminar como parte de la nube de hiperescala de otra persona. Ese fue el objetivo final desde una perspectiva de estrategia comercial, y finalmente hemos alcanzado ese hito”, dijo Wang.
La API de LLAMA está actualmente disponible como una vista previa limitada, con Meta planifica un despliegue más amplio en las próximas semanas y meses. Los desarrolladores interesados en acceder a la inferencia Ultra-Fast Llama 4 pueden solicitar el acceso temprano seleccionando cerebras de las opciones del modelo dentro de la API de LLAMA.
“Si te imaginas a un desarrollador que no sabe nada sobre cerebras porque somos una empresa relativamente pequeña, solo pueden hacer clic en dos botones en el SDK estándar de SDK estándar de Meta, generar una tecla API, seleccionar la bandera de cerebras y luego, de repente, sus tokens se procesan en un motor gigante a escala de dafers”, explicó las cejas. “Ese tipo de hacernos estar en el back -end del ecosistema de desarrolladores de Meta todo el ecosistema es tremendo para nosotros”.
La elección de Meta de silicio especializada señala algo profundo: en la siguiente fase de la IA, no es solo lo que saben sus modelos, sino lo rápido que pueden pensarlo. En ese futuro, la velocidad no es solo una característica, es todo el punto.
Insights diarias sobre casos de uso comercial con VB diariamente
Si quieres impresionar a tu jefe, VB Daily te tiene cubierto. Le damos la cuenta interior de lo que las empresas están haciendo con la IA generativa, desde cambios regulatorios hasta implementaciones prácticas, por lo que puede compartir ideas para el ROI máximo.
Lea nuestra Política de privacidad
Gracias por suscribirse. Mira más boletines de VB aquí.
Ocurrió un error.
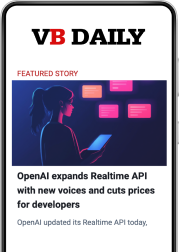
Related posts
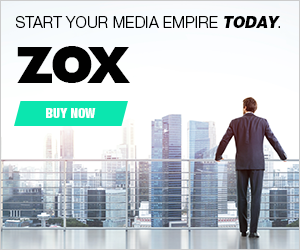
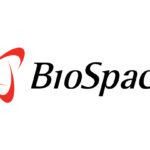
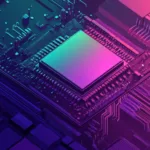
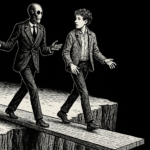
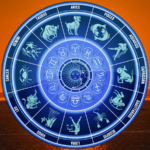
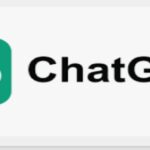
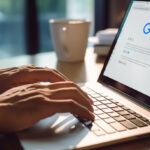
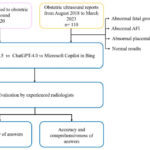
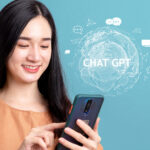
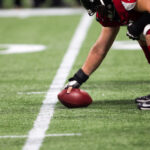
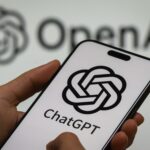
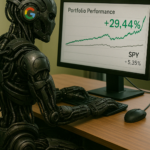
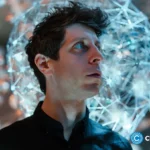
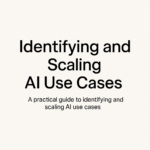
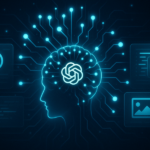
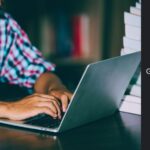
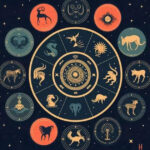
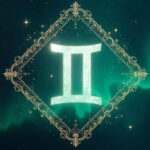
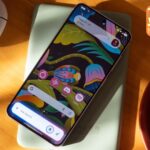
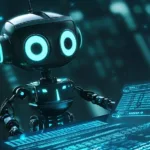
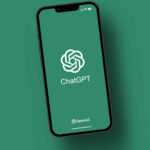
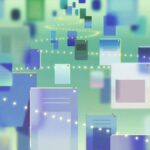
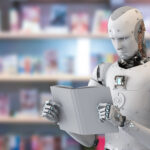
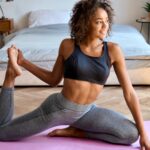
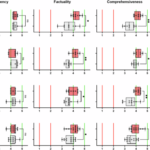
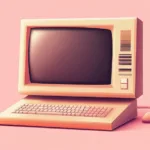
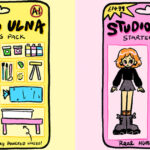
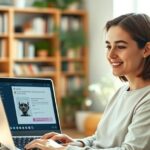
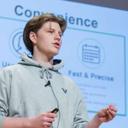
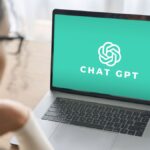
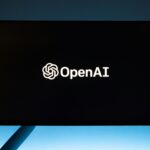
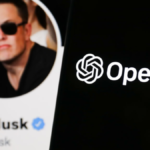
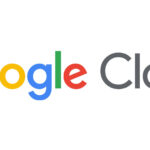
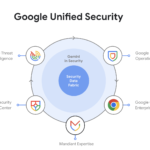
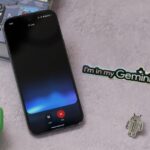
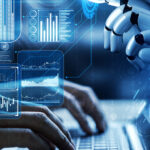
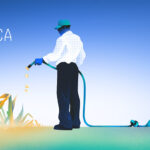
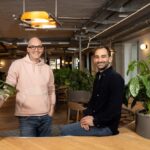
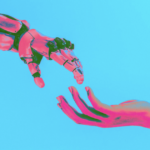
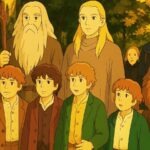
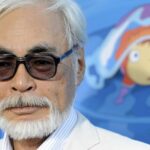
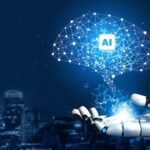
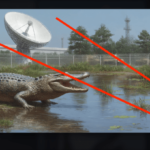
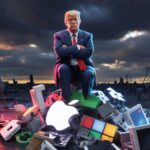
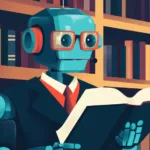
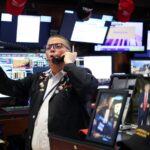
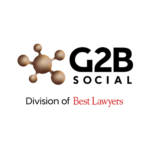
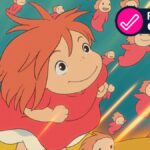
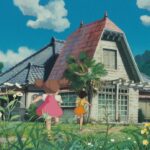
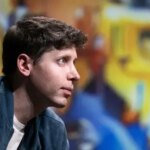
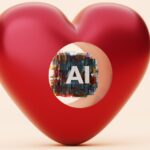
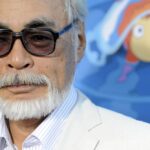
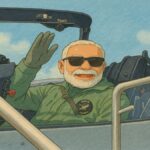
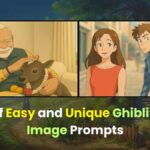
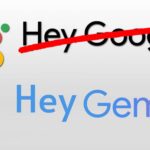
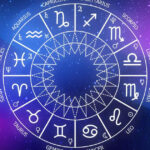
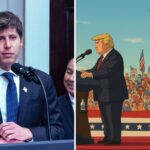
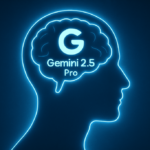
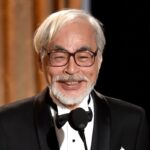
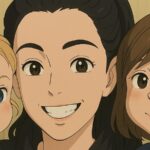
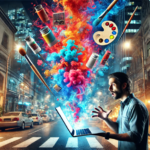
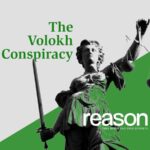
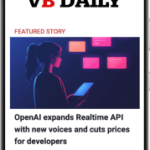
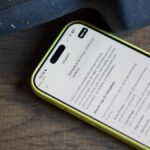
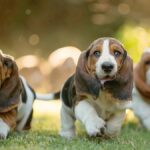
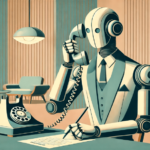
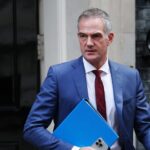
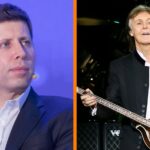
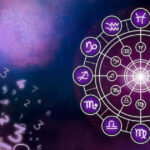
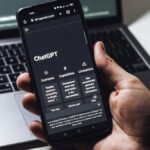
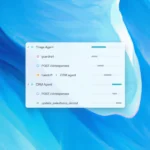
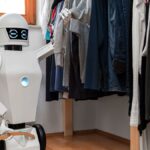
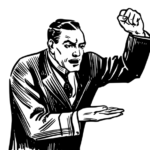
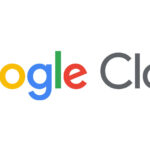
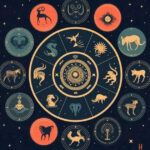
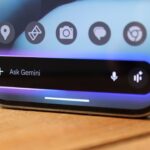
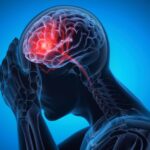
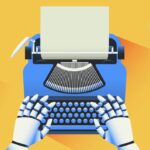
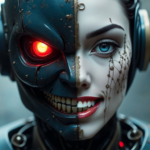
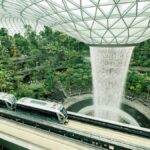
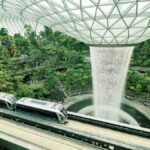
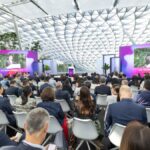
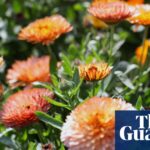

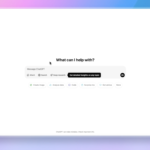
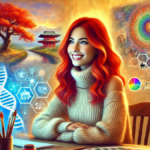
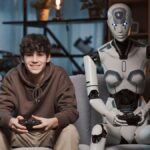
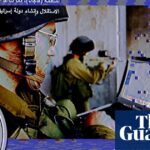
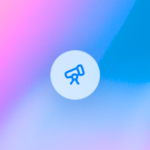
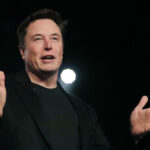
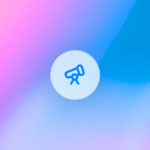
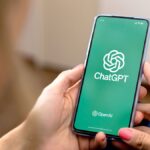
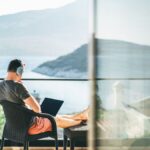
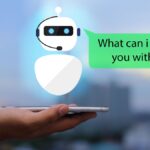
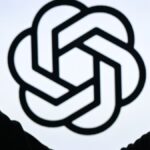
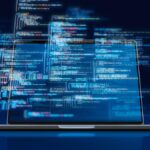
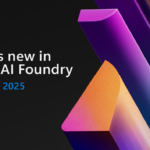
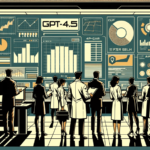
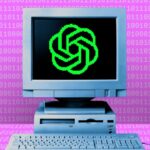
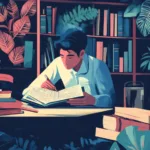
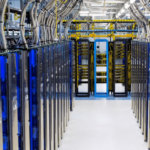
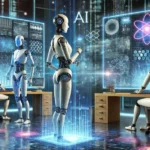
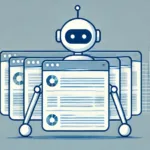
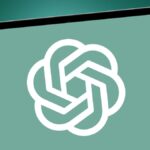
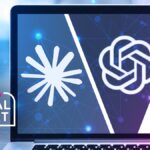
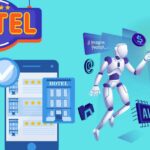
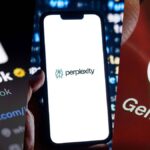
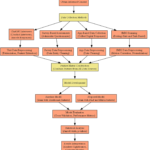
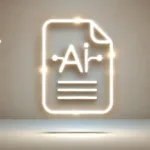
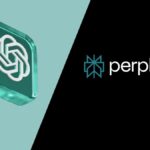
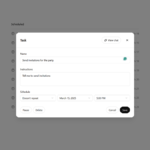
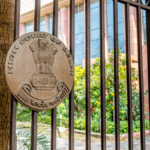
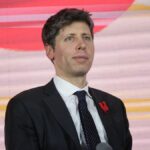
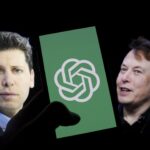
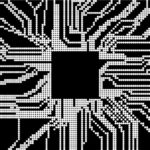
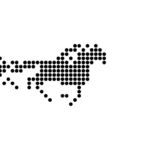
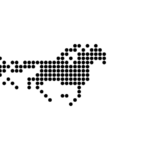
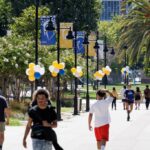
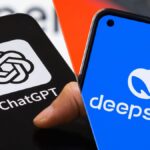
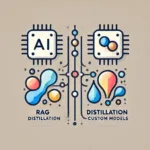
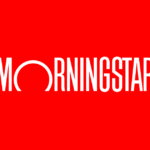
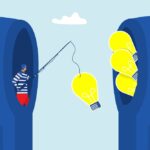
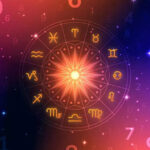
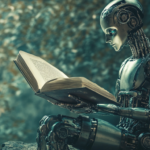
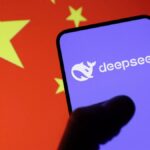
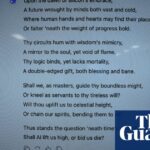
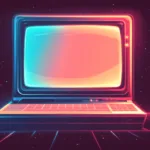

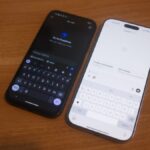
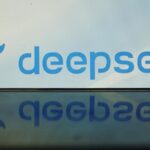

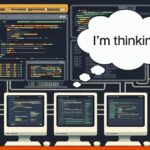
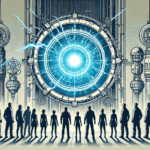
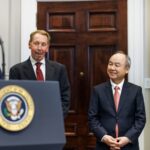
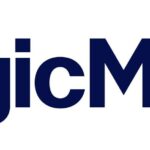
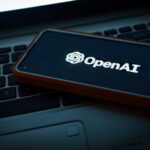

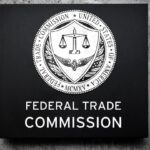
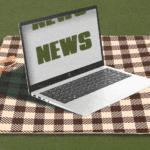
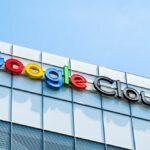
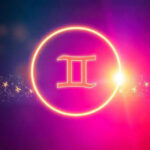
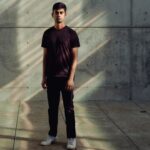
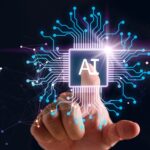
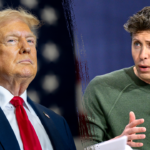
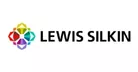
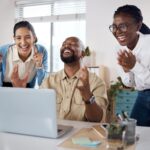
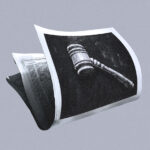
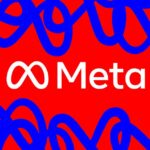

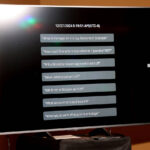

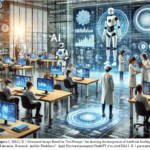
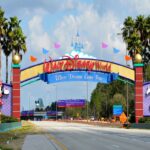
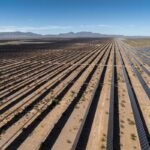
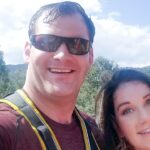
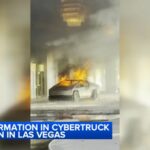
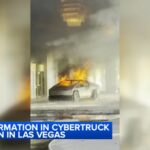
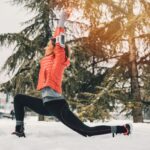
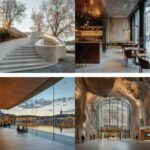
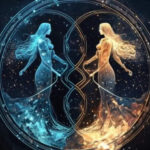
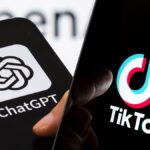
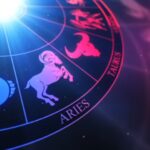
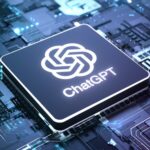
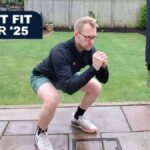
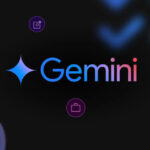
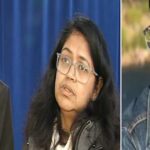
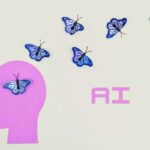
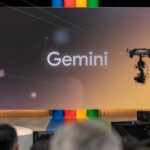
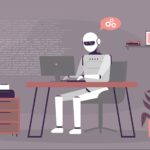
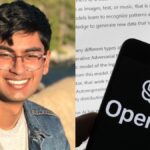
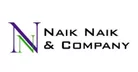

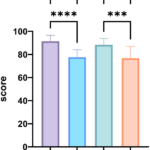
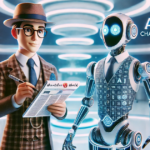
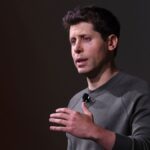
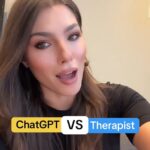
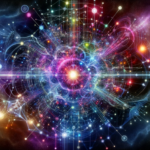
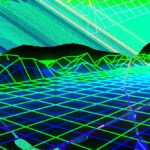
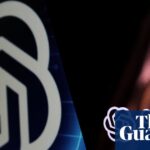
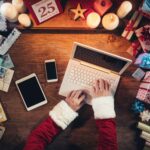
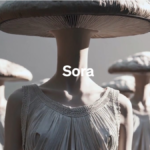
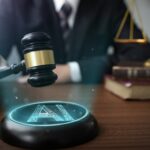
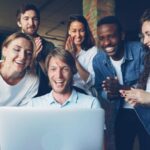
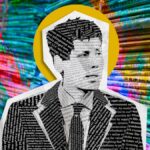

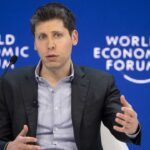
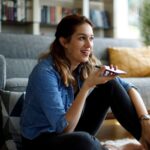
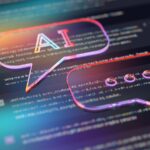
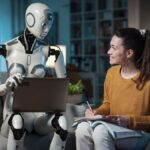
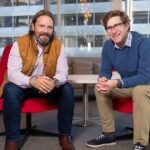
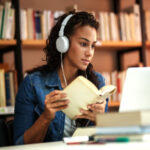
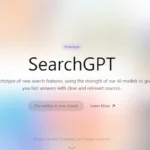
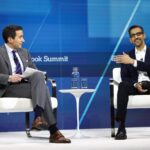
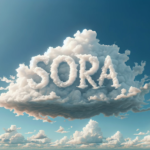
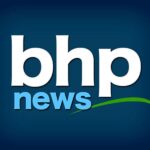
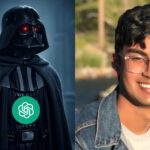
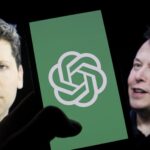
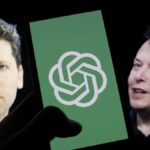
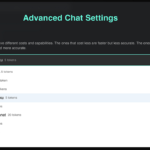
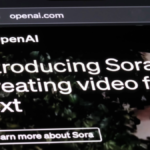
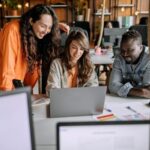
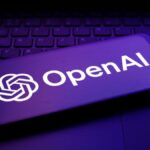
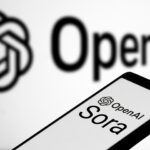
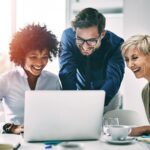
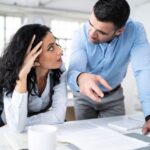
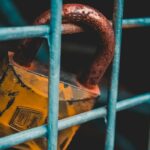
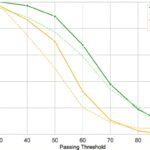
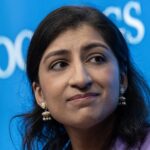
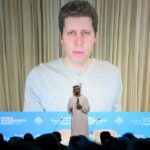
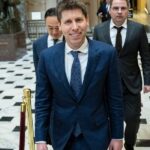
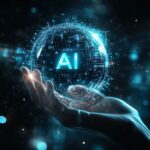
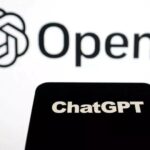
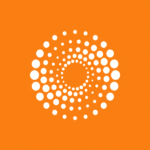
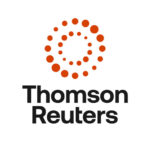
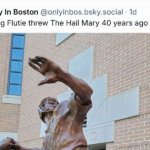

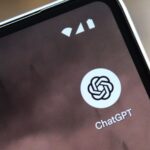
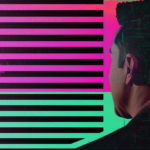
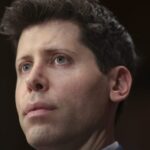
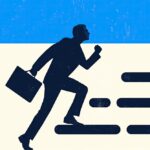
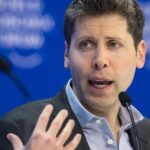
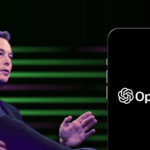
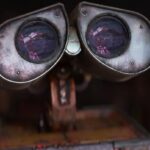
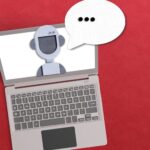
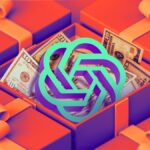
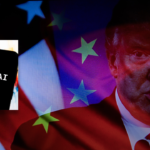
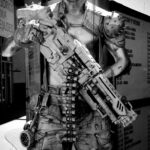
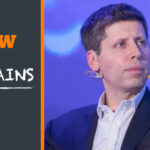
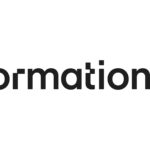
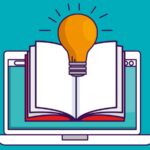
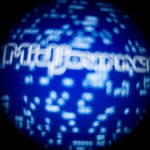
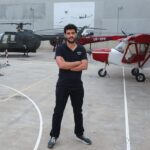
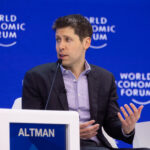
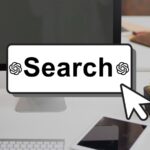
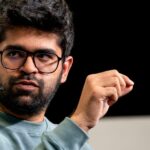
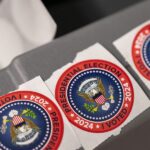
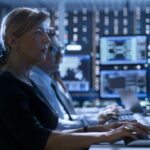
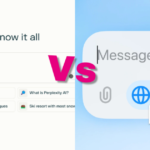
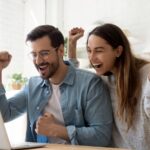
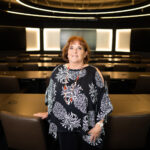
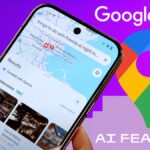
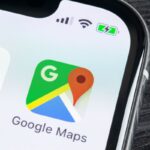
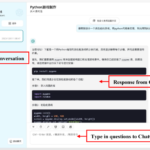
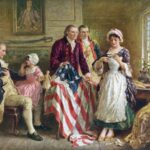
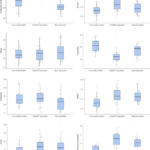

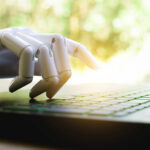
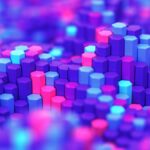
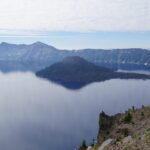
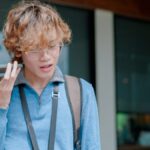
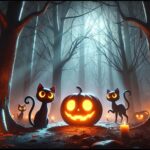
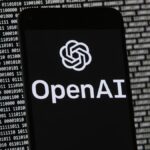
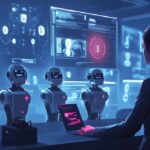
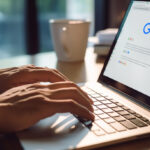
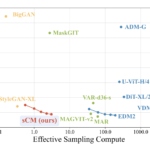
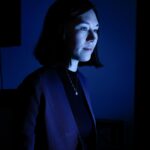
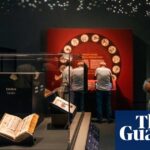
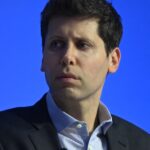
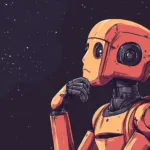
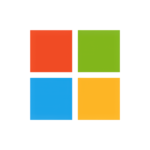
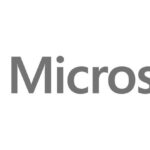
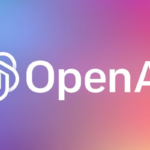
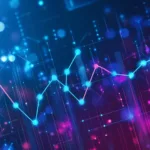
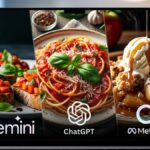
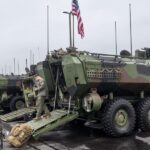

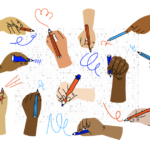
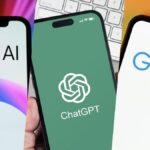
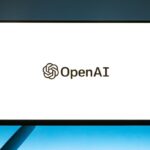
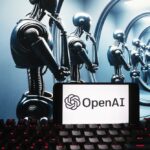
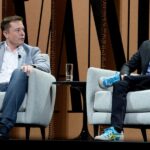
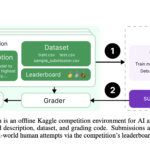
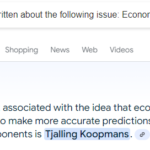
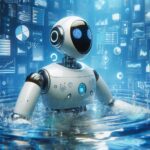
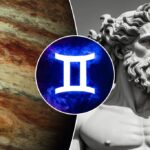
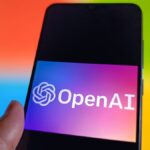
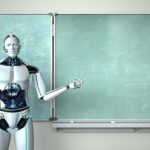
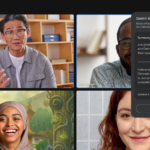
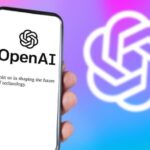
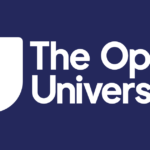
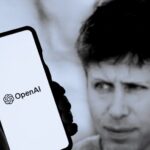
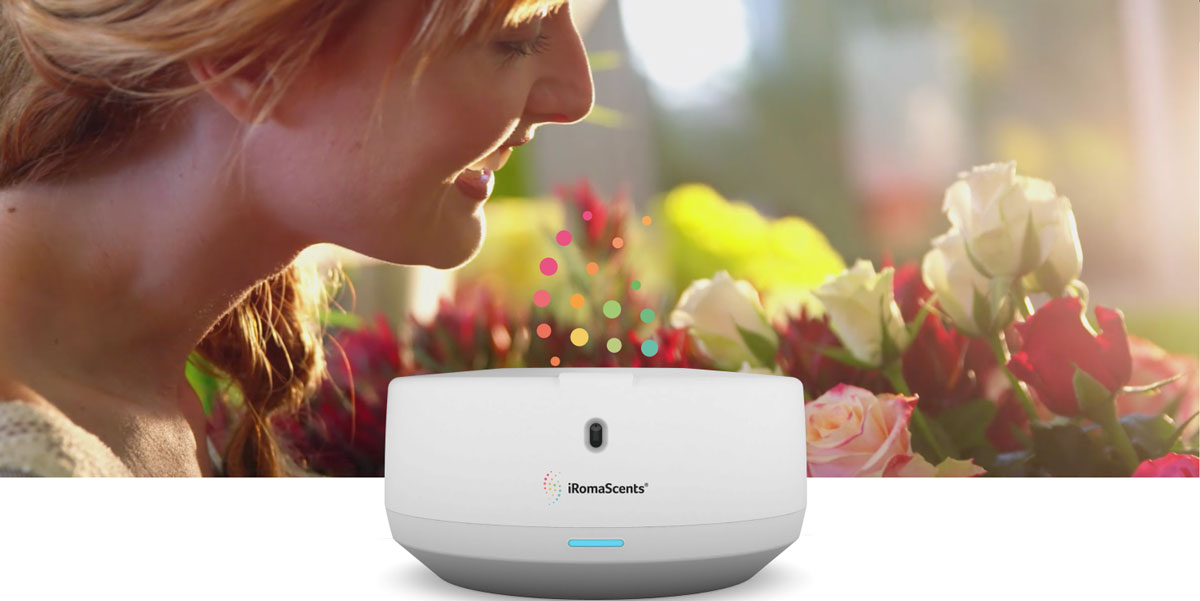

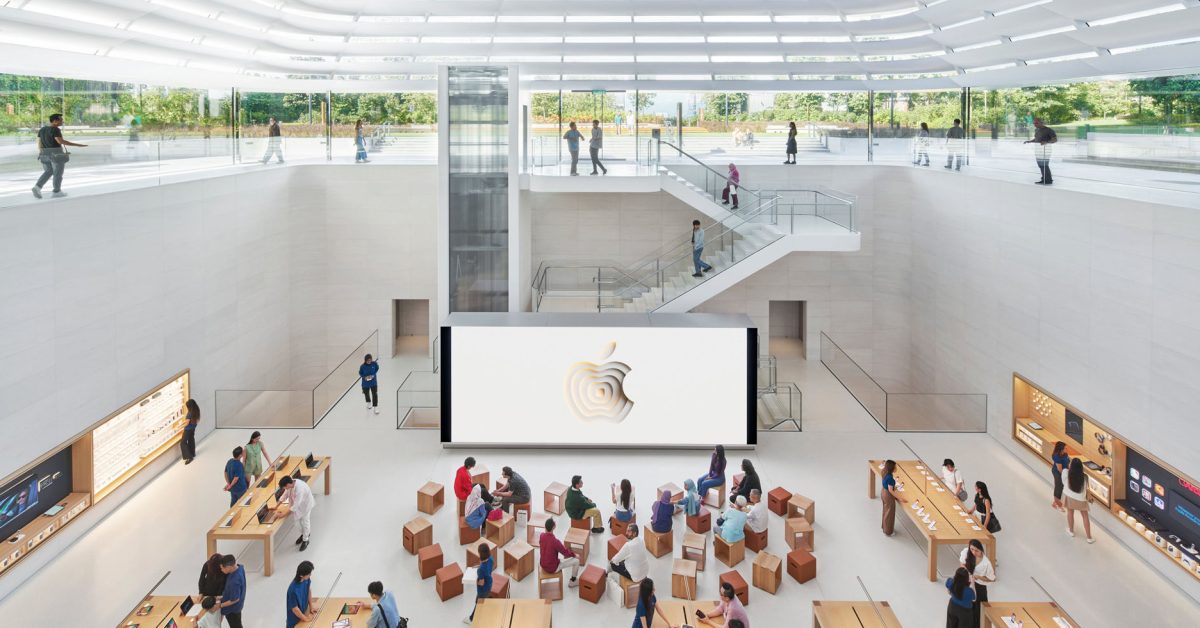


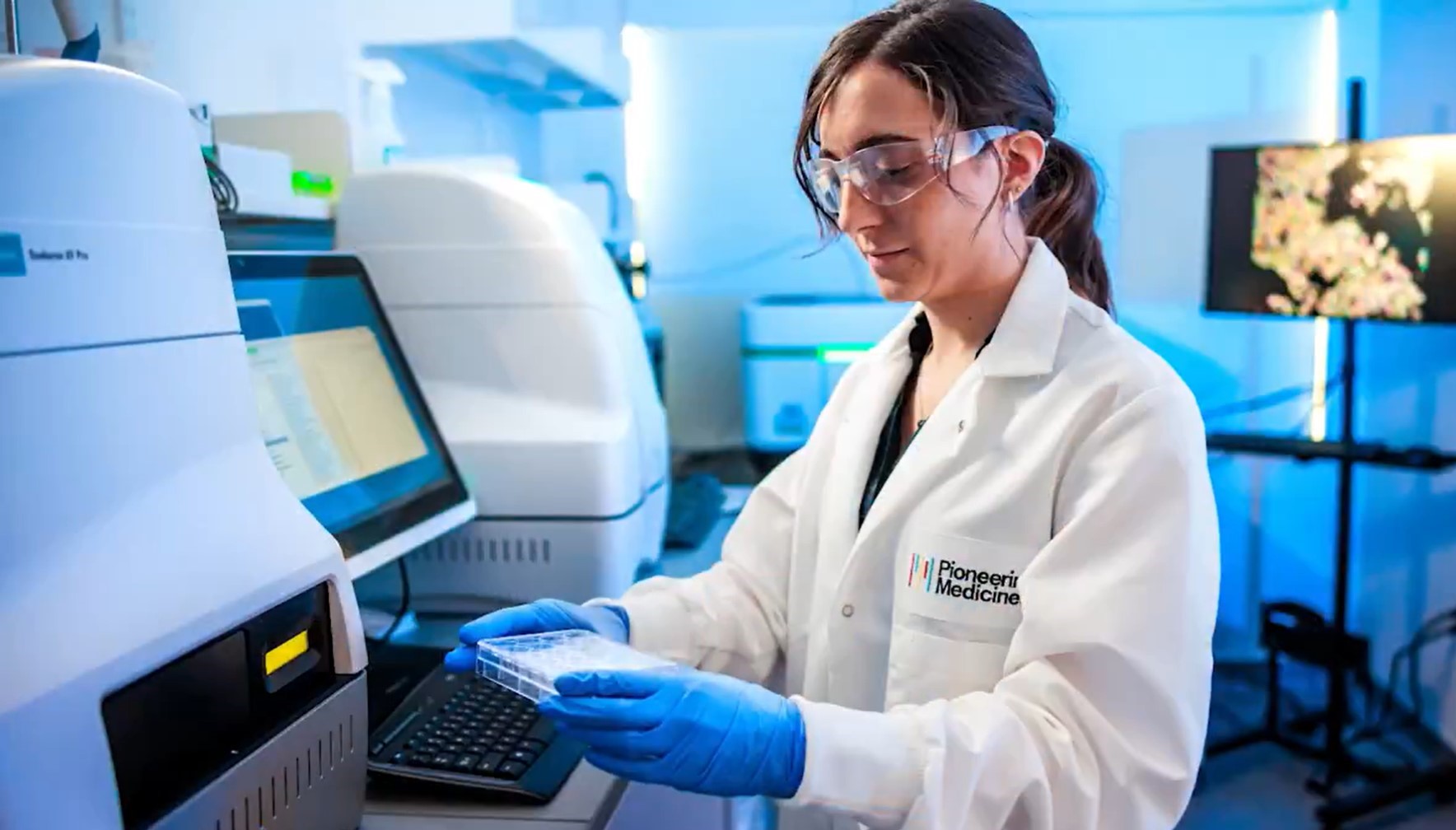
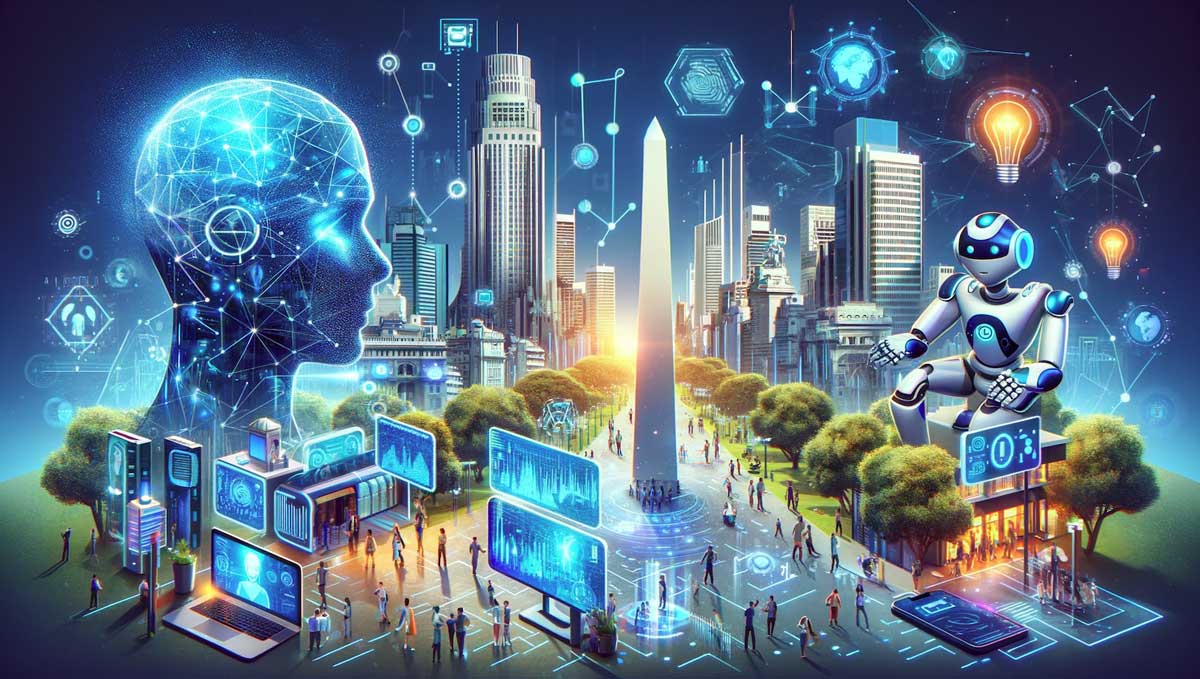

Trending
-
Startups11 meses ago
Remove.bg: La Revolución en la Edición de Imágenes que Debes Conocer
-
Tutoriales12 meses ago
Cómo Comenzar a Utilizar ChatGPT: Una Guía Completa para Principiantes
-
Recursos12 meses ago
Cómo Empezar con Popai.pro: Tu Espacio Personal de IA – Guía Completa, Instalación, Versiones y Precios
-
Startups10 meses ago
Startups de IA en EE.UU. que han recaudado más de $100M en 2024
-
Startups12 meses ago
Deepgram: Revolucionando el Reconocimiento de Voz con IA
-
Recursos11 meses ago
Perplexity aplicado al Marketing Digital y Estrategias SEO
-
Recursos12 meses ago
Suno.com: La Revolución en la Creación Musical con Inteligencia Artificial
-
Noticias10 meses ago
Dos periodistas octogenarios deman a ChatGPT por robar su trabajo