Noticias
OpenAI Researcher Dan Roberts on What Physics Can Teach Us About AI
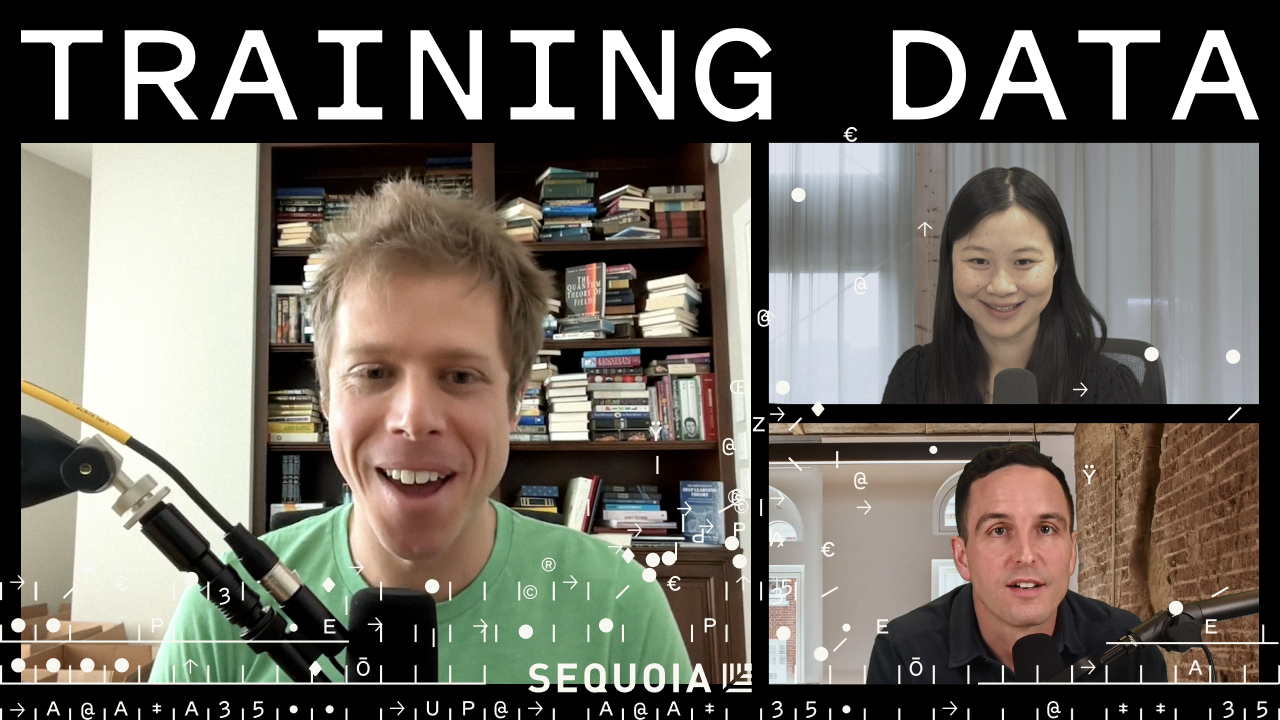
Contents
Dan Roberts: In the 40s, the physicists went to the Manhattan Project. Even if they were doing other things, that was, that was the place to be. And so now AI is the same thing and, you know, basically OpenAI is that place. So maybe, maybe we don’t need a public sector organized Manhattan Project, but it, you know, it can be OpenAI.
Sonya Huang: Joining us for this episode is Dan Roberts, a former Sequoia AI Fellow who recently joined OpenAI as a researcher. This episode was recorded on Dan’s second-to-last day at Sequoia, before he knew that he would go on to become a core contributor to o1, also known as Strawberry.
Dan is a quantum physicist who did undergraduate research on invisibility cloaks before getting a PhD at MIT and doing his postdoc at the legendary Princeton IAS. Dan has researched and written extensively about the intersection of physics and AI.
There are two main things we hope to learn from Dan in this episode. First, what can physics teach us about AI? What are the physical limits on an intelligence explosion and the limits of scaling laws, and how can we ever hope to understand neural nets? And second, what can AI teach us about physics and math and how the world works?
Thank you so much for joining us today, Dan.
The path to physics
Dan Roberts: Thanks. Delighted to be here on my, probably, second-to-last day at Sequoia. Depending on when this airs and how you’re going to talk about it.
Pat Grady: You will always be part of the Sequoia family, Dan.
Dan Roberts: Thanks, I appreciate it.
Sonya Huang: Maybe just to get started tell us a little bit about “Who is Dan?” You have a fascinating backstory. I think you worked on invisibility cloaks back in college. Like, what led you to become a theoretical physicist in the first place?
Dan Roberts: Yeah, I think–and this is, you know, my stock answer at this point, but I think it’s true–I was just an annoying three year old who never grew up. I asked “why” all the time. Curious. How does everything work? I have a 19-month at home right now, and I can see the way he followed the washing machine repairman around and had to look inside the washing machine. So like, I think I just kept that going. And when you’re more quantitatively oriented than not, rather than going to philosophy I think you sort of veer into physics. And that was sort of what interested me. How does the world work? What is all this other stuff that’s out there?
The question that you didn’t ask, but maybe I’ll just answer it ahead of time–the sort of inward facing stuff felt less quantitative and more in the realm of the humanities. Like, so “What is all this stuff?” That’s pretty physics-y. “What am I? Who am I? What does it mean to be me?” That felt not very science-y at all. But with AI it sort of seems like we can think about both “What does it mean to be intelligent,” and also “What is all this other stuff?” in some of the same frameworks. And so that’s been very exciting for me.
Sonya Huang: So we should be trying to recruit your 19-month-old right now is what you’re saying?
Dan Roberts: Oh yeah. Absolutely. He grew out of one of his Sequoia onesies, but he fits into a Sequoia toddler t-shirt now. And so he definitely is ready to be a future founder.
Sonya Huang: I guess, at what point did you know that you wanted to think about AI? At what point did that switch start to flip?
Dan Roberts: Yeah. So, I think like many people, when I discovered computers, I wanted to understand how they worked and how to program them. In undergrad, I took an AI class and it was very much good old fashioned AI. A lot of the ideas in that class actually are coming back to be relevant, but at the time it was, it seemed not very practical. It was a lot of, you know, “If this happens, do that.” And there was also some game-playing there. That was sort of interesting. It was very algorithmic, but it didn’t seem related to what it means to be intelligent.
What does it mean to be intelligent?
Pat Grady: Can we just pick up on that real quick? Like, what do you think it means to be intelligent?
Dan Roberts: That’s a great question.
Pat Grady: I can see wheels turning.
Dan Roberts: Yeah. Well, this is one of those questions where I don’t have a stock answer, but I think it’s important to not say nonsense. One of the things that’s exciting to me about AI is the ability to have systems that do what humans do, and to be able to understand them from “What are the lines of Python that cause that system to do something?” to, you know, to trace that through and understand what are the outputs, and you know, how the system can like, see and classify what it means. You know, “What is a cat? What is not a cat?” Or can write poetry and, you know, it’s just a few lines of code. Whereas, if you’re trying to study humans and ask, “What does it mean for humans to be intelligent?” you have to go from biology, through neuroscience, through psychology, up to other higher level versions of ways of approaching this question.
And so, I think maybe a nice answer for intelligence, at least the things that are interesting to me, is “The things that humans do.” And now, if you pull back the answer that I just said a second ago, that’s how I connect it to AI. AI is taking pieces of what humans do, and we’re understanding, at least an example that’s kind of simple and easy to study, that we might use to understand better what what it is that humans do.
The path to AI
Sonya Huang: So Dan, you mentioned when you were, you know, studying AI in college, there was a lot of what sounds like kind of hard-coded logic and brute force type approaches. Was there a moment that kind of clicked for you, of like, “Oh, okay. This is different”? Was there a key result or a moment where it was like, “Okay, we’re going bigger places than kind of the ‘if this, then that’ logic of the past”?
Dan Roberts: Yeah, actually it didn’t click. I sort of wrote it off. And it would be great if there was a separation between like ten years between then and the next thing that I’m going to say, but actually the writing-off maybe lasted a year or two, because then I went to the UK for the first part of grad school. I spent a very long time in grad school and I discovered machine learning and a more statistical approach to artificial intelligence, where you have a bunch of examples of large amounts of data. Or at the time, maybe we wouldn’t have said large amounts of data, but we would have said, you have data examples of a task that you want to perform. And there’s different ways that machine learning works, but you write down a very flexible algorithm that can adapt to these examples and start to perform in a way similar to the examples that you have.
And that approach borrows a lot from physics. It also, at the time, I started… So graduated college in 2009. Discovered machine learning in 2010, 2011. 2012 is the big year for deep learning. And so, you know, there’s not a big separation here between write-off and rediscovery. But I think–and machine learning clearly existed in 2009, it just wasn’t related to the class that I took–but this approach made a lot of sense to me. And it started to have, you know, I got lucky, and then it started to have real progress and seemed to fit in a framework that I understood scientifically. And I got very excited about it.
Sonya Huang: Why do you think there are so many people who come from a similar background or similar path to you? Like, a lot of ex-physicists working on AI. Like, is that a coincidence? Is that herd behavior, or do you think there’s something that makes physicists particularly well suited to understanding AI?
Dan Roberts: I think the answer is yes to all the ways you…
Sonya Huang: All the above.
Dan Roberts: You know, physicists infiltrate lots of different subjects, and we get parodied about the way that we go about trying to use our hammers to tackle these things that may or may not be nails. Throughout history, there are a lot of times that physicists have contributed to things that look like machine learning. I think in the near term, the path that physicists used to take when they didn’t remain in academia often was going into quantitative finance, then data science. And I think machine learning, and its realization in industry, was exciting because, again, it’s something that feels a lot like actual physics and is working towards a problem that’s very interesting and exciting for a lot of people.
You know, you’re doing this podcast because you’re excited about AI. Everyone’s excited about AI. And in many ways it’s a research problem that feels a lot like the physics that people were doing. But I think the methods of physics actually are different from the methods of traditional computer science and very well suited for studying large scale machine learning that we use to work on AI.
Traditionally physics involves a lot of interplay between theory and experiment. You come up with some sort of model that you have some sort of theoretical intuition about. Then you go and do a bunch of experiments and you validate that model or not. And then there’s this tight feedback loop between collecting data, coming up with theories, coming up with toy models. Understanding moves forward by that. And, you know, we get these really nice explanatory theories. And I think the way that big deep learning systems work, you have this tight feedback loop where you can do a number of experiments. The sort of math that we use is very well suited to the math that a lot of physicists are familiar with. And so I think it’s very natural for a lot of physicists to work on this. And those tools, a number of them differ from the traditional, at least theoretical, computer science and theoretical machine learning tools for studying the theoretical side of machine learning, and maybe also differentiation between just being an awesome engineer and also being a scientist. And there’s tools from doing science that are helpful in studying these systems.
Pat Grady: Dan, you wrote this, what I thought was a beautiful article, Black Holes and the Intelligence Explosion, and in there you talk about this concept of, sort of the microscopic point of view, and then the system level point of view and how physics really equips people to think about the system level point of view, and that has a sort of complimentary benefit to understanding these systems. Can you just take a minute and kind of explain sort of microscopic versus system level, and how the physics influence helps to understand the system level?
Dan Roberts: Sure. So, let me start with an analogy that I think is, like, very, you know, goes even further than an analogy. But going back, what year is it? Maybe like 200 years or so around the time of the Industrial Revolution, there was steam engines and steam power and a lot of technology that resulted from this and ultimately powered industrialization. And in the beginning, there was a lot of engineering of these steam engines, you know, and there was this high level theory of how this work called thermodynamics, where, you know, and I imagine everyone’s seen this in high school, perhaps where, you know, there’s the Ideal Gas Law that tells you that there’s some relationship between pressure and volume and temperature. And, you know, these are very macro level things. Like you can buy a thermometer. You can also measure the volume of your room and you can buy a barometer as well. Maybe people don’t or look up on the weather report. But, you know, these are like measurements that we use and we talk about. But then underlying this, and it took us a bit later to validate this and understand it, there’s the notion of atoms and molecules, the air molecules bouncing around. And somehow we now understand that those air molecules give rise to things like temperature and pressure and volume. I guess it is easier to understand that the gases, the molecules, are confined to a room.
But there’s a precise way in which you can start with a statistical understanding of those molecules and derive thermodynamics, like, derive the Ideal Gas Law from it. And you can go further than that to derive that it’s ideal because it’s wrong. It’s just a toy model. But you can, there are corrections to it, and you can sort of understand, you know, from the microscopic perspective, which is the molecules, which we don’t really interact with. You know, we don’t see them, we don’t interact with them on a day-to-day basis, but their statistical aggregate properties give rise to, sort of, this behavior that we do see at the macro scale.
And part of, to get to your question, you know, I think there’s a similar thing going on with deep learning systems. And I wrote a book with Sho Yaida and Boris Hanin on how to apply these sorts of ideas to deep learning, and, at least in an initial framework that allows you to start doing this in an initial way. And to answer your question, the sort of micro perspective is you have neurons and weights and biases, and we can talk about in detail how that works, but when people think of the architecture, there’s some very specific, some people say circuits, there’s specific ways in which these things, you know, there’s an input signal which might be an image or text, and then there’s many parameters. And, you know, it’s very simple to write down. It’s not that many lines of code even taking into account the machine learning libraries, but it’s, you know, it’s like a very simple set of equations. But there’s a lot of weights. There’s a lot of numbers that you have to know in order to get it to do something. And that’s sort of the micro… that’s like the molecules perspective.
And then there’s like the macro perspective, which is, well, what did it do? Did it produce a poem? Did it solve a math problem? How does, how do we go from that, those weights and biases to, to that macro perspective? And so for statistical physics to thermodynamics, we understand that completely. And you can imagine trying to do the same sort of thing, literally applying the same sorts of methods to understand how does the underlying micro statistical behavior of these models lead to the sort of macro or, as you said, system level perspective?
Sonya Huang: Dan, maybe speaking of scaling laws, and I think you were at our event, AI Ascent, Andre Karpathy mentioned that current AI systems are like 5 or 6 orders of magnitude off in efficiency compared to biological neural nets. How do you think about that? Do you think scaling laws get us there? Just a combination of scaling laws plus hardware getting more efficient or, like, do you think that there’s kind of big step function leaps that need to come in research?
Dan Roberts: There’s maybe two things that could be meant here. One is that the way humans seem to work at a similar scale to AI systems is much more efficient. You know, we don’t need to see trillions of tokens before we speak. You know, my toddler is already starting to speak in sentences. And he’s been exposed to far less tokens than a typical large language model. And so there’s some sort of disconnect between human efficiency at learning and what large language models do. Of course, they’re very different systems. They’re designed, you know, the way in which they learn is right now very different. And so in some sense that’s to be expected. So, there’s this gap here that you could imagine bridging.
There’s another thing that I think is not what you meant, but I think is sort of the thing to answer about with respect to scaling laws, which is, and I talked about this a bit in the article, but lots of people seem to talk about this, which is “What is the final GPT?” You know, there’s GPT-4 right now, and it could be other companies as well, but since I’m going to join OpenAI let me represent my new company, right? So is it going to be six? Is it going to be seven? At some point, assuming we have to scale things up, there are things that are going to break. Whether they’re economic, we’re going to run out of, you know, we’re going to try to train a model that’s larger than the world’s GDP or GWP, however, the D works for the world. Or we’re going to run out of, you know, we’re not going to be able to produce enough GPUs. Or we’re not going to be able to put, you know, it’s going to cover the surface of the Earth. You know, a lot of these things are going to break down at some point. And so probably the economic one happens first. So how many, you know, how many more iterations do we get before we run out of actually being able to scale practically? And where does that get us? And then, I think, to tie those two perspectives together, there’s scaling on its own. And of course, it’s impossible to disentangle this because people are making things more efficient.
But you know, there’s like, you could imagine there’s the, take literally what GPT-2 was, which was the initial big model, and keep scaling it up. Is that going to get us to some, you know, super different, exciting, economically powerful, or however you want to define what the end state of AI research and AI startups and AI in industry is? Or do we need lots of new exciting ideas? And, and again, of course, you can’t really disentangle these, but I think the general scaling hypothesis is that it’s just the scaling and it’s not the ideas that matter. Whereas, the “How do we get to be efficient like humans?” I think requires, like, non-trivial ideas. And to answer your question, the reason I’m excited about joining OpenAI is that I think there is high leverage to be had in the ideas, you know, in going beyond scaling, and that we will need that in order to get to the next steps. I have no idea what I’ll be working on, but when this airs I guess I will know what I’m working on, but you know that that’s what’s really exciting to me.
The pendulum between scale and ideas
Pat Grady: Dan, is there almost like a pendulum that swings back and forth between scale and ideas in terms of how people apply their efforts in the world of AI? Like, transformers came out. Great idea. Since then, we’ve largely been in this race to scale. It feels like things are starting to asymptote for a bunch of practical reasons that you mentioned. Is the pendulum swinging back toward ideas as the currency? You know, it’s less now about who can, you know, have the biggest GPU cluster and more about finding new architectural breakthroughs, whether that’s, you know, reasoning or something else?
Dan Roberts: Yeah. That’s a really great question. I think there’s this article by Richard Sutton called The Bitter Lesson, not the bitter pill, and it basically gives the argument that ideas aren’t important, that scale is what you need, and that all the ideas are always trumped by scaling, by scaling things up. It says a bunch of things, but maybe that’s a high level takeaway. And, you know, there’s a sense of this where there are a lot of interesting ideas that came out in the 80s and 90s that people didn’t really have scale to explore. And then, I remember when, after AlphaGo and DeepMind was writing a lot of papers, people were rediscovering those papers and re-implementing them in deep learning systems. But this was sort of still before people realized, “No, the thing that you need to do is scale up.” And even now, with transformers, people are exploring other architectures or even simpler architectures that we knew before that seem to be able to, you know, there’s a notion, you know, maybe scaling laws don’t come from as long as the architecture isn’t sick in some way.
They come from sort of the underlying data process and having large amounts of data rather than from having a special idea. I think the real answer is that there’s a balance between the two. That scale is hugely important, and maybe it was just not understood how important. And we also didn’t have the resources to to scale things up at various times, you know, the things that have to go into producing these GPU clusters that are producing these models are, you know, you guys know this as well, like there’s a lot of parts along the supply chain, or along the product chain, whatever you actually call it, in order to make those things happen and to deploy them. And even, you know, the way GPUs were originally, they’ve now co-evolved to be well suited for these models. And the reason, in some sense, you can think of transformers was a good idea was because it was designed to be well-suited to train on the systems that we had at the time. And so sure, these other architectures could do it at an ideal scientific level, but at a practical level, it was important to to get something that was that that was able to to reach that scale.
So I think, you know, if you brought in ideas to be that sort of thing that’s married with scale in some way, then I still think ultimately, like, you know, someone came up with the idea of deep learning. That was an important idea. You know, there’s Pitts and McCulloch came up with the original idea for the neuron. Rosenblatt came up with the original perceptron. And there’s like a lot of people, from going back like 80 or so years, of people just making important discoveries that were ideas that contribute. So, I think it’s both. But it’s easy to see how, you know, if you’re bottlenecked, people think about ideas. And then if you unlock a new capacity of scale somehow, then you just see a huge set of results. And it seems like scale is super important. And I really think it’s more of a synergy between the two.
The Manhattan Project of AI
Sonya Huang: Maybe on the topic of the race to scale, Dan, you mentioned kind of just the economic constraints and realities, which I guess are more, like, practically a ceiling in the private sector. You also mentioned the Manhattan Project earlier in terms of things that physicists have been involved with. Like, do you think we need a Manhattan Project style thing for AI? Like at the nation state or at the international level?
Dan Roberts: Well, one thing I can say is that part of the process that led me to OpenAI is I was talking with your partner, Shaun Maguire, who brought me to Sequoia in the first place, and trying to figure out is there a startup that makes sense for me to work on that has the right mix of, sort of, scientific questions, research questions, and also as a business? And I think it was Shaun that said–and I don’t mean the analogy in terms of the impact of, you know, in terms of like negative impact of what you might think of the Manhattan Project, but just in terms of the scale and the organization–he said, “You know, in the 40s, the physicists’ physicist went to the Manhattan Project. Even if they were doing other things, that was the place to be.” And so now AI is the same thing, and basically said OpenAI is that place. So maybe, maybe we don’t need a public sector organized Manhattan Project, but it, you know, it can be OpenAI.
Sonya Huang: OpenAI as the Manhattan Project. I love that.
Dan Roberts: Well, maybe that’s not a direct quote that we want to be taken out of context, but I think in terms of…
Sonya Huang: The metaphorical Manhattan Project.
Dan Roberts:Yeah. In terms of scale and ambition. I mean, I think a lot of physicists would love to work at OpenAI for a lot of the same reasons that they probably were excited to… Well, okay, there’s a number of different reasons. Maybe we just have to leave it as a nuanced thing rather than making broad claims.
AI’s black box
Sonya Huang: Can we talk a little bit about this… Like, can we ever understand AI, especially as we go to these deep neural nets, or do you think it’s a hopeless black box, so to speak?
Dan Roberts: Yeah, I think within the… This is my answer to the “What are you a contrarian about?” Although maybe, you know, on the internet everyone takes every side of every position, so it’s hard to say you have a contrarian position. But I think within AI communities, you know, I think my contrarian position is that we can really understand these systems. And in, you know, physics systems are extremely complicated, and we have made a huge amount of progress in understanding them. I think these systems sit in the same framework. And, you know, another principle that Sho and I talk about in our book, and that’s a principle of physics, is that there’s this often extreme simplicity at very large scales, basically due to the statistical averaging, or more technically, the central limit theorem. Things can simplify–and I’m not saying this is what happens exactly in large language models, Of course not–but I do think that we can apply sort of the methods that we have and also, you know, maybe hopefully have AI that can help us do this in the future. And by AI, I mean tools. Not like individual intelligences just going running on their own and solving these problems. But I guess I feel at the extreme end that this is not going to be an art, that the science will will catch up and that it will will be able to make extreme leaps in really understanding how these systems work and behave.
What can AI teach us about physics?
Pat Grady: So, Dan, we’ve talked a bunch about what physics can teach us about AI. Can we talk a bit about what AI can teach us about physics? Are you optimistic about domains like physics and math and how these emerging models can, you know, probe further into those domains?
Dan Roberts: Yes, I’m definitely optimistic. I guess my perspective is that math will be easier than physics, which maybe betrays the fact that I’m a physicist, not a mathematician. And I’ll say–I can give explain why I think that in a second–but, you know, I still have a lot of friends that work in physics and they, you know, there’s like a growing sense and maybe even approaching a dread, that maybe this is actually the answer to “Why do physicists work on AI?” Because, you know, if what you care about is the answer to your physics question and you want to make it happen as soon as possible, what is the highest leverage thing you can do? Maybe it’s not work on the physics question you care about, but it’s work on AI to make the, you know, because you think that the AI might end up solving those questions very rapidly anyway. And I don’t know the extent that anyone really takes it seriously, but I think within the theoretical physics community that I come from, that this is sort of a thing that somewhat gets thrown around and discussed.
I think maybe to give a more object-level answer, I think what’s exciting about math–and maybe when you have Noam Brown on, if you have him on, he’ll talk about this, but this is something that that he’s talked about for a while before he joined OpenAI–I think the that we have, you know, we made a lot of progress in terms of solving games by doing more than just looking up what is the strategy that we should use to play the games, but also being able to simulate forward and, you know, the way that if I’m in a very hard position in a particular game, rather than just playing with intuition, I might sit and think about what I should do. Yeah, sometimes this goes under the name inference-time compute rather than raining-time compute or pre-training. And you know, there’s a sense in which what it means to do reasoning is very related to this ability to sit and think. So we know how to do it for games because there’s a very clear winning and loss signal. So you can simulate ahead and sort of figure out what it means to do good or not. And I think math, in some parts of math–again, I’m not a mathematician and well, you know, I’m always scared about talking about math publicly and saying something wrong that will upset mathematicians–but it seems like certain types of math problems are not as constrained as games, but are still constrained enough where there’s a notion of like finding a proof, right? You know, there’s definite problems in terms of search, in terms of how do you figure out what is the next, you know, move in the proof, but the fact that we might call it a move suggests that there’s things in math that feel a lot like games. And so we might think the fact that we can do well at games maybe means that we can do well at certain types of mathematical discovery.
Can AI help solve physics’ biggest problems?
Pat Grady: Well, I was going to say since you mentioned Noam, he likes to use the example with test-time compute of whether it could help to prove the Riemann hypothesis. Is there a similar problem or hypothesis in the world of physics that you are optimistic AI can help to solve sometime in our lifetimes?
Dan Roberts: Yeah. So, I mean, there’s a Millennium Problem relating to physics–and if I try to remember exactly what it is I’m sure I will butcher it and then no one will believe that I’m actually a physicist–but it’s related to the, you know, it’s a mathematical physics question related to the Yang-Mills mass gap. But I think, what I wanted to say is that I think some of the flavor of what physicists care about and doing physics feels a little different. This is where I might get in trouble. It feels a little different than some of, like, the mathematical proof type things. Physicists are known to be more informal and, you know, hand-wavy. But also, on the other hand, connected to, in some sense, connected to the interplay between experiment and the sort of models that physicists study is maybe what saves them is that, you know, they have things that are informal and hand-wavy, but very explanatory. And then the mathematicians, it’s like we were saying earlier, the engineers discovered all the exciting industrial machines, and the physicists maybe cleaned up a bunch of the theory about how that works. And then the mathematicians come later and clean up, like, formalize everything and clean it up even more. And so there’s a mathematician or mathematical physicist that cleans up a lot of, you know, make proper and try and, you know, understand in formal ways some of the stuff that physicists do.
But rather than talking about–I mean, I think the key point there is that the sort of questions that are interesting to physicists maybe don’t look like proofs, and maybe it’s not about how do we, given a particular model, how do we actually solve it? Like once things are set up correctly, like it’s often, you know, senior or you know, people that are trained in the field are able to sort of figure out how to analyze those systems. It’s more the other stuff. Like, what is the right model to study? Does it capture the right sort of problems? Does this relate to the thing that you care about? What are the insights you can draw from it? And so for AI to help there, I think it would look different than the way we’re sort of trying to build AI systems for math. So rather than here’s, you know, here’s the word problem going, you know, solve this high school level problem or, you know, prove the Riemann hypothesis.
It’s like, you know, the questions in physics are like, “What is quantum gravity? What happens when something goes into a black hole?” And that’s not like, you know, start generating tokens on that. What does that even look like? And you know, if you go to a physics department, you know, people hang out at the blackboard. They chat about things. Like, maybe they sketch mathematical things, but you know, there’s a lot of other things that go into this. And so maybe the sort of data that you need to collect looks more like that. Or maybe it looks like the random emails and conversations on Slack and the scratch work. And so, I mean, there are definitely tools that we can use, like “Help me understand this new paper so I don’t have to spend two weeks trying to study it and understand it.” You know, maybe let me ask questions about it. I think there are problems with the way that’s currently implemented.
But, you know, I think there are a lot of tools that will help accelerate physicists just like Mathematica, which is a software package that does integrals, and it does a lot more than that. Sorry, Stephen Wolfram. But I use it to do integrals and, you know, sometimes it doesn’t know integrals and you can look them up in like these integral tables. Anyway. You know, I think, you know, and this applies to other branches of science too. Like, I think the ways in which the questions are asked and what it means to do science in different fields, maybe can look further and further from gains, let’s just say. And so to the extent that that’s true, I think we’ll need to. And not even clear that we’ll need lots of ideas or I mean, we we will need lots of ideas, but it’s more just like, we’ll have to, I think, we’ll just have to approach them all differently. And maybe not, like maybe eventually we’ll have a universal thing that knows how to do all of it. But initially, like at least to me, a lot of these things feel a little different from each other.
Sonya Huang: You’ll have a front row seat to it, in part because you’re also on the prize committee for the AI Math Olympiad, which is something I’m personally super interested in.
Maybe to your last point on, kind of like, maybe eventually this stuff generalizes. Like, why do you think people are so focused on solving the hardest problems today, like physics, math? Those were the subjects that everyone was terrified of in school, right? Where it feels like there’s a lot more other domains that are also unsolved for now. Like do you think, do you think going for the hardest domains first kind of lets you get towards a generalized intelligence? Like how does solving these different domains kind of fit together in the grander puzzle?
Dan Roberts: Yeah. The first thing that comes to mind when you said that is to just push back and say, “Well, it’s not hard. These are the easy domains.” I mean, I’m bad at biology. It doesn’t make any sense to me at all. My girlfriend actually is in bioengineering and is in biotech. And so, like, what she does just makes no sense to me. I can’t understand any of it, where physics makes complete sense to me. I think maybe a better answer or a less glib answer is that, like I was trying to say about math, there are constraints. And, you know, in particular with math, a lot of it is unembodied. You don’t have to go and do experiments in the real world. You know, they’re sort of self-sufficient and that’s close to, like, what generating text, like the way language models work, or even the way some reinforcement learning systems work for games. And so, I think the further that you go from that, the messier things become, the harder it probably is, and also the harder it is to get the right kind of data to train these systems.
If you want to build an AI–and people are trying to do this, but it seems difficult–if you want to build an AI system that solves biology, I guess you need to also make sure robotics works, you know, so that it can do those sorts of experiments and like it has to understand that sort of data. Or maybe it has humans do it. But, you know, there’s a lot, you know, for a self-sustaining AI biologist, it seems like there’s a lot of things that are going to go into it. I mean, on the way, we’ll have things like AlphaFold 3, which just came out and which I didn’t get a chance to read the details of, but, you know, I saw that they’re trying to use it for drug discovery. And so, you know, I think each of these fields will have things developed along the way. But I think the less constraints there are and the sort of messier and more embodied it is, the harder it will be to accomplish.
Sonya Huang: No, that makes sense. So, like hard for a human is not the same thing–doesn’t correlate to hard for machine.
Dan Roberts: Yeah. Plus, also maybe humans disagree about what’s hard or not.
Sonya Huang: Some of us think more like machines, I guess. And then I guess the second question was like, do you think it all coalesces into, like, one big model that understands everything? Because right now it seems like there’s a lot of domain-specific problem solving that’s happening.
Dan Roberts: Yeah. I mean, the way things are going, it seems like the answers should be yes. It’s really dangerous to speculate in this field because everything you say is wrong. Usually much sooner than later.
Sonya Huang: Good thing we’re on record. We’ll hold you to it.
Dan Roberts: Yeah, exactly. But also, like, what does it mean to be different? You know, like, there’s a trivial way to make both things–make the question meaningless–by, like you say, the model is the union of all those other models. But there’s also a sense in which mixture of experts was originally meant to be that, it’s not that in practice at all. But, you know, there is a sliding scale here. But, you know, it does seem like people, at least the big labs, are going for the one big model and have a belief that that’s, you know, well… I don’t know, but maybe I will in the future, understand what the philosophy is there.
Lightning round
Pat Grady: Dan, we have a handful of more general questions to kind of close things out here. So I’ll start with the high level one. If we think kind of short-term, medium-term, long-term and call it, you know, five months, five years, five decades, what are you most excited or optimistic about in the world of AI?
Dan Roberts: Five years ago was after the transformer model came out, but it was around maybe when GPT-2 came out. So it seems like, you know, for the last five years we’ve been doing scaling. I imagine within the next five years, we’ll see that scaling will terminate. And maybe it will terminate in, you know, a utopia of some kind, you know, that people are excited about where we’re all post-economic and so forth, and we’ll have to shut down all your funds and, you know, return monopoly money because money won’t matter. Or we’ll see that we need lots of ideas. Maybe there will be another AI winter.
I imagine that–and again, scary to really speculate–but I imagine, like something will be something will be interestingly different within five years about AI. And it might just be that AI is over and we’re on to the, you know, we’re on to the next exciting investment opportunity. And, you know, everyone else will shift elsewhere. And you know, I’m not saying that. That’s not what’s motivating me about AI, but, you know. So maybe five years is enough time to see that. And I think in, in one year, I mean, or there’s a five. I messed this up. Whatever. Maybe it was five months, I don’t remember. I’m sorry.
Pat Grady: It’s five months. It’s okay, it’s okay. These are approximations. I know you said physicists are very hand-wavy. Venture capitalists are very hand-wavy. These are approximations.
Dan Roberts: In physics there’s–I like to joke that there’s like three numbers. There’s zero, one and infinity. And, you know, those are the only numbers that matter. You know, things are either arbitrarily small, arbitrarily large, or about order one.
So. Okay. Good. Thanks for reminding me. But yeah, for five months, I mean, I’m excited to well, to learn what’s exciting at the forefront of a huge research lab like OpenAI. And I think one thing that will be interesting will be the delta between the next generation of models, right? Because there’s ways in which things are scaling up in terms of, it’s not really public, I guess, aside from Meta, but in terms of size of data, size of models. And we see scaling laws that relate to, you know, something like the loss and, you know, it’s hard to translate that into actual capabilities. And so what will it feel like to talk to the next generation model? What will it look like? Will it have a huge economic impact or not? And I think I, you know, in terms of estimating velocity, right, you need a few points. You can’t just have one point. And we sort of have, you know, we’re starting to have that with GPT-3 to GPT-4. .But, you know, I feel like with the next delta, we’ll get to really see what the velocity looks like and what it feels like going from model to model to model. And maybe I’ll be able to make a better prediction in five months from now, but then I guess I probably won’t be able to tell it to you guys.
Sonya Huang: Thanks, Dan. One thing that stood out to me is just like your writing is so accessible and light and funny, and that’s not what I’m used to when I read super technical stuff. Like, do you think all technical writing should be informal and funny? Like, is that deliberate?
Dan Roberts: It’s definitely deliberate. It goes into, I think in some sense it’s inherited. I mean, I definitely am a not serious person, but I also think it’s an inherited, sort of, from the style of the field that I came from. But I’ll tell you a story. I was at lunch. I was a postdoc at the Institute for Advanced Study in Princeton, and I was having lunch and joking around with this professor, Nati Seiberg, who’s a professor at the institute. And then we got into, I think we were talking about someone, someone asked a question about, like, “What is a good title?” And I was like, “Oh, the title has to be a joke.” And he was on board with that. And then I was explaining that for me, the reason to write a paper is for the jokes. Like you have a bunch of jokes in mind, and then you want people to read those jokes. And so you have to package it into the science product, and people want to read the science product, and they’re forced to suffer through the jokes. And Nati, who is this Israeli professor, he was like, “I don’t get it. Why? Why can’t you just do the science? Why do you need the, like… the jokes are great too, but like, you should write for the science, not for the jokes.”
And I was adamant that I write for the jokes. But I think it’s, I think it’s what you said that at some point, you know, you learn about the scientific method and, you know, the, the formal ways of doing things, and you learn all these rules and then you grow up a bit. Or maybe, I had a roommate who was a linguist, he’s now a professor of linguistics at UT Austin, and like, he emphasized that you can–he would tell me which rules that I could break or what, like where the rules come from and why they’re important or not. And you sort of realize that you can break these rules. And, like, the ultimate goal should be, is the reader going to read it and understand it and enjoy it. So you don’t want to do things that compromise their ability to read and understand. But you don’t want to obscure things. You want to make it, you know, if it’s more enjoyable, people are more likely to read it and take the point. It’s also more fun if you’re writing it. So I think that’s where that comes from.
Sonya Huang: Dan, thank you so much for joining us today. We learned a lot. We enjoyed your jokes and I hope you have. I hope you have a wonderful second-to-last day at Sequoia. Thank you for spending part of it with us. We really appreciate it.
Dan Roberts: Thanks. I was absolutely delighted to be here chatting with you guys. This was wonderful.
Noticias
Why Google’s search engine trial is about AI : NPR
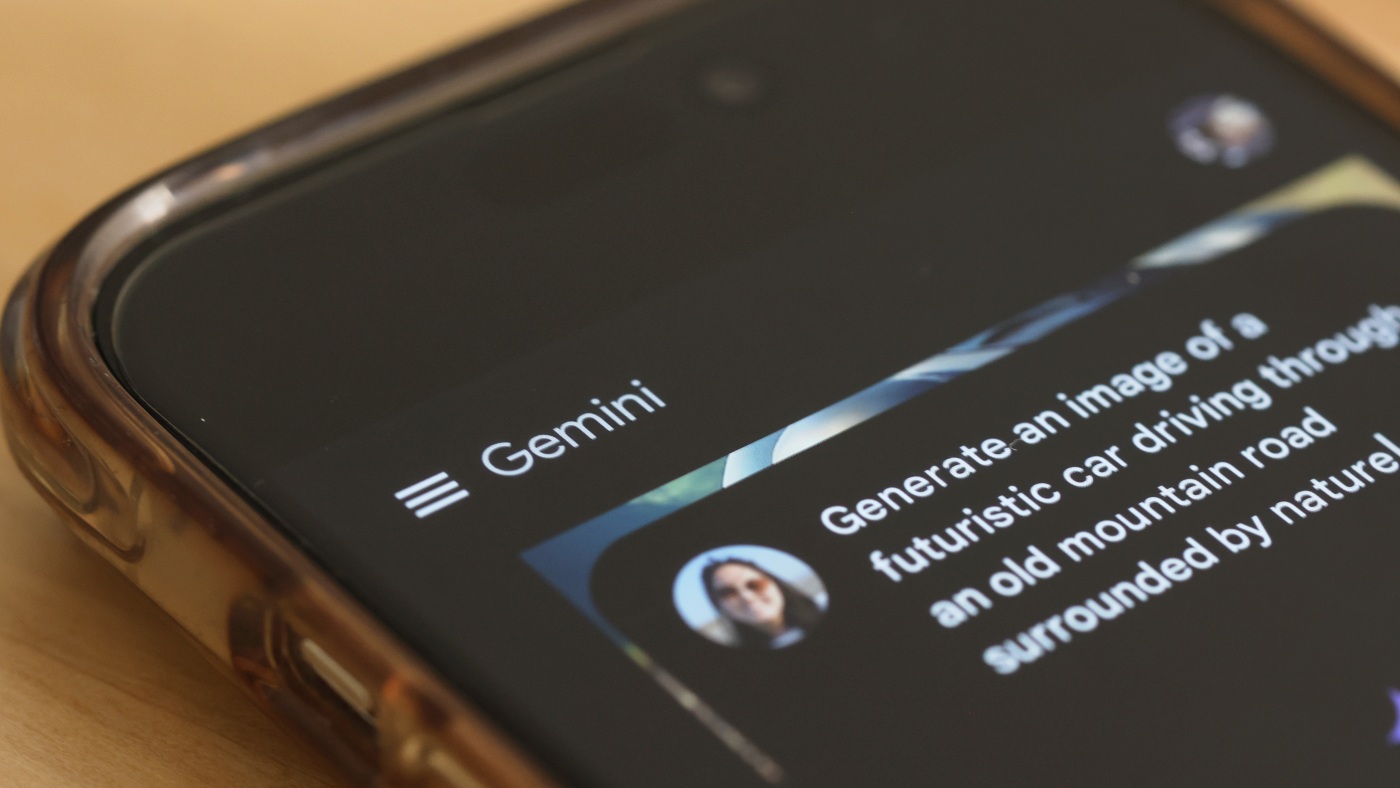
An illustration photograph taken on Feb. 20, 2025 shows Grok, DeepSeek and ChatGPT apps displayed on a phone screen. The Justice Department’s 2020 complaint against Google has few mentions of artificial intelligence or AI chatbots. But nearly five years later, as the remedy phase of the trial enters its second week of testimony, the focus has shifted to AI.
Michael M. Santiago/Getty Images/Getty Images North America
hide caption
toggle caption
Michael M. Santiago/Getty Images/Getty Images North America
When the U.S. Department of Justice originally brought — and then won — its case against Google, arguing that the tech behemoth monopolized the search engine market, the focus was on, well … search.
Back then, in 2020, the government’s antitrust complaint against Google had few mentions of artificial intelligence or AI chatbots. But nearly five years later, as the remedy phase of the trial enters its second week of testimony, the focus has shifted to AI, underscoring just how quickly this emerging technology has expanded.
In the past few days, before a federal judge who will assess penalties against Google, the DOJ has argued that the company could use its artificial intelligence products to strengthen its monopoly in online search — and to use the data from its powerful search index to become the dominant player in AI.
In his opening statements last Monday, David Dahlquist, the acting deputy director of the DOJ’s antitrust civil litigation division, argued that the court should consider remedies that could nip a potential Google AI monopoly in the bud. “This court’s remedy should be forward-looking and not ignore what is on the horizon,” he said.
Dahlquist argued that Google has created a system in which its control of search helps improve its AI products, sending more users back to Google search — creating a cycle that maintains the tech company’s dominance and blocks competitors out of both marketplaces.
The integration of search and Gemini, the company’s AI chatbot — which the DOJ sees as powerful fuel for this cycle — is a big focus of the government’s proposed remedies. The DOJ is arguing that to be most effective, those remedies must address all ways users access Google search, so any penalties approved by the court that don’t include Gemini (or other Google AI products now or in the future) would undermine their broader efforts.
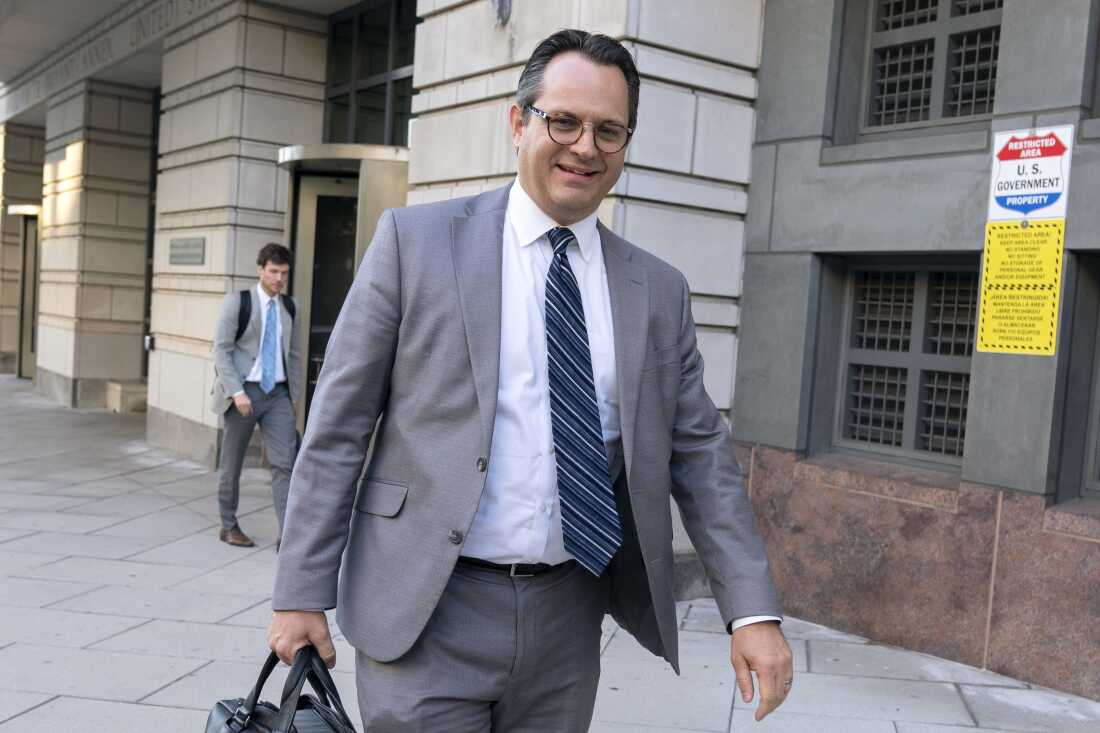
Department of Justice lawyer David Dahlquist leaves the Washington, D.C. federal courthouse on Sept. 20, 2023 during the original trial phase of the antitrust case against Google.
Jose Luis Magana/AP/FR159526 AP
hide caption
toggle caption
Jose Luis Magana/AP/FR159526 AP
AI and search are connected like this: Search engine indices are essentially giant databases of pages and information on the web. Google has its own such index, which contains hundreds of billions of webpages and is over 100,000,000 gigabytes, according to court documents. This is the data Google’s search engine scans when responding to a user’s query.
AI developers use these kinds of databases to build and train the models used to power chatbots. In court, attorneys for the DOJ have argued that Google’s Gemini pulls information from the company’s search index, including citing search links and results, extending what they say is a self-serving cycle. They argue that Google’s ability to monopolize the search market gives it user data, at a huge scale — an advantage over other AI developers.
The Justice Department argues Google’s monopoly over search could have a direct effect on the development of generative AI, a type of artificial intelligence that uses existing data to create new content like text, videos or photos, based on a user’s prompts or questions. Last week, the government called executives from several major AI companies, like OpenAI and Perplexity, in an attempt to argue that Google’s stranglehold on search is preventing some of those companies from truly growing.
The government argues that to level the playing field, Google should be forced to open its search data — like users’ search queries, clicks and results — and license it to other competitors at a cost.
This is on top of demands related to Google’s search engine business, most notably that it should be forced to sell off its Chrome browser.
Google flatly rejects the argument that it could monopolize the field of generative AI, saying competition in the AI race is healthy. In a recent blog post on Google’s website, Lee-Anne Mulholland, the company’s vice president of regulatory affairs, wrote that since the federal judge first ruled against Google over a year ago, “AI has already rapidly reshaped the industry, with new entrants and new ways of finding information, making it even more competitive.”
In court, Google’s lawyers have argued that there are a host of AI companies with chatbots — some of which are outperforming Gemini. OpenAI has ChatGPT, Meta has MetaAI and Perplexity has Perplexity AI.
“There is no shortage of competition in that market, and ChatGPT and Meta are way ahead of everybody in terms of the distribution and usage at this point,” said John E. Schmidtlein, a lawyer for Google, during his opening statement. “But don’t take my word for it. Look at the data. Hundreds and hundreds of millions of downloads by ChatGPT.”
Competing in a growing AI field
It should be no surprise that AI is coming up so much at this point in the trial, said Alissa Cooper, the executive director of the Knight-Georgetown Institute, a nonpartisan tech research and policy center at Georgetown University focusing on AI, disinformation and data privacy.
“If you look at search as a product today, you can’t really think about search without thinking about AI,” she said. “I think the case is a really great opportunity to try to … analyze how Google has benefited specifically from the monopoly that it has in search, and ensure that the behavior that led to that can’t be used to gain an unfair advantage in these other markets which are more nascent.”
Having access to Google’s data, she said, “would provide them with the ability to build better chatbots, build better search engines, and potentially build other products that we haven’t even thought of.”
To make that point, the DOJ called Nick Turley, OpenAI’s head of product for ChatGPT, to the stand last Tuesday. During a long day of testimony, Turley detailed how without access to Google’s search index and data, engineers for the growing company tried to build their own.
ChatGPT, a large language model that can generate human-like responses, engage in conversations and perform tasks like explaining a tough-to-understand math lesson, was never intended to be a product for OpenAI, Turley said. But once it launched and went viral, the company found that people were using it for a host of needs.
Though popular, ChatGPT had its drawbacks, like the bot’s limited “knowledge,” Turley said. Early on, ChatGPT was not connected to the internet and could only use information that it had been fed up to a certain point in its training. For example, Turley said, if a user asked “Who is the president?” the program would give a 2022 answer — from when its “knowledge” effectively stopped.
OpenAI couldn’t build their own index fast enough to address their problems; they found that process incredibly expensive, time consuming and potentially years from coming to fruition, Turley said.
So instead, they sought a partnership with a third party search provider. At one point, OpenAI tried to make a deal with Google to gain access to their search, but Google declined, seeing OpenAI as a direct competitor, Turley testified.
But Google says companies like OpenAI are doing just fine without gaining access to the tech giant’s own technology — which it spent decades developing. These companies just want “handouts,” said Schmidtlein.
On the third day of the remedy trial, internal Google documents shared in court by the company’s lawyers compared how many people are using Gemini versus its competitors. According to those documents, ChatGPT and MetaAI are the two leaders, with Gemini coming in third.
They showed that this March, Gemini saw 35 million active daily users and 350 million monthly active users worldwide. That was up from 9 million daily active users in October 2024. But according to those documents, Gemini was still lagging behind ChatGPT, which reached 160 million daily users and around 600 million active users in March.
These numbers show that competitors have no need to use Google’s search data, valuable intellectual property that the tech giant spent decades building and maintaining, the company argues.
“The notion that somehow ChatGPT can’t get distribution is absurd,” Schmidtlein said in court last week. “They have more distribution than anyone.”
Google’s exclusive deals
In his ruling last year, U.S. District Judge Amit Mehta said Google’s exclusive agreements with device makers, like Apple and Samsung, to make its search engine the default on those companies’ phones helped maintain its monopoly. It remains a core issue for this remedy trial.
Now, the DOJ is arguing that Google’s deals with device manufacturers are also directly affecting AI companies and AI tech.
In court, the DOJ argued that Google has replicated this kind of distribution deal by agreeing to pay Samsung what Dahlquist called a monthly “enormous sum” for Gemini to be installed on smartphones and other devices.
Last Wednesday, the DOJ also called Dmitry Shevelenko, Perplexity’s chief business officer, to testify that Google has effectively cut his company out from making deals with manufacturers and mobile carriers.
Perplexity AIs not preloaded on any mobile devices in the U.S., despite many efforts to get phone companies to establish Perplexity as a default or exclusive app on devices, Shevelenko said. He compared Google’s control in that space to that of a “mob boss.”
But Google’s attorney, Christopher Yeager, noted in questioning Shevelenko that Perplexity has reached a valuation of over $9 billion — insinuating the company is doing just fine in the marketplace.
Despite testifying in court (for which he was subpoenaed, Shevelenko noted), he and other leaders at Perplexity are against the breakup of Google. In a statement on the company’s website, the Perplexity team wrote that neither forcing Google to sell off Chrome nor to license search data to its competitors are the best solutions. “Neither of these address the root issue: consumers deserve choice,” they wrote.
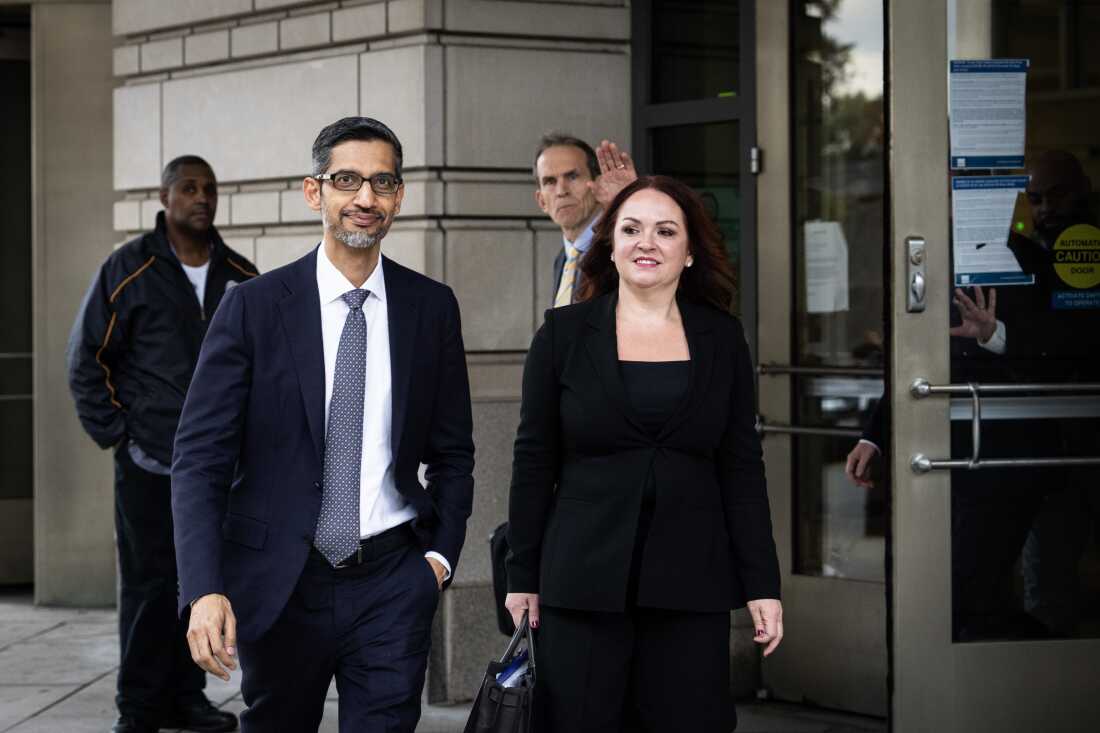
Google and Alphabet CEO Sundar Pichai departs federal court after testifying in October 2023 in Washington, DC. Pichai testified to defend his company in the original antitrust trial. Pichai is expected to testify again during the remedy phase of the legal proceedings.
Drew Angerer/Getty Images/Getty Images North America
hide caption
toggle caption
Drew Angerer/Getty Images/Getty Images North America
What to expect next
This week the trial continues, with the DOJ calling its final witnesses this morning to testify about the feasibility of a Chrome divestiture and how the government’s proposed remedies would help rivals compete. On Tuesday afternoon, Google will begin presenting its case, which is expected to feature the testimony of CEO Sundar Pichai, although the date of his appearance has not been specified.
Closing arguments are expected at the end of May, and then Mehta will make his ruling. Google says once this phase is settled the company will appeal Mehta’s ruling in the underlying case.
Whatever Mehta decides in this remedy phase, Cooper thinks it will have effects beyond just the business of search engines. No matter what it is, she said, “it will be having some kind of impact on AI.”
Google is a financial supporter of NPR.
Noticias
API de Meta Oleleshes Llama que se ejecuta 18 veces más rápido que OpenAI: Cerebras Partnership ofrece 2.600 tokens por segundo

Únase a nuestros boletines diarios y semanales para obtener las últimas actualizaciones y contenido exclusivo sobre la cobertura de IA líder de la industria. Obtenga más información
Meta anunció hoy una asociación con Cerebras Systems para alimentar su nueva API de LLAMA, ofreciendo a los desarrolladores acceso a velocidades de inferencia hasta 18 veces más rápido que las soluciones tradicionales basadas en GPU.
El anuncio, realizado en la Conferencia inaugural de desarrolladores de Llamacon de Meta en Menlo Park, posiciona a la compañía para competir directamente con Operai, Anthrope y Google en el mercado de servicios de inferencia de IA en rápido crecimiento, donde los desarrolladores compran tokens por miles de millones para impulsar sus aplicaciones.
“Meta ha seleccionado a Cerebras para colaborar para ofrecer la inferencia ultra rápida que necesitan para servir a los desarrolladores a través de su nueva API de LLAMA”, dijo Julie Shin Choi, directora de marketing de Cerebras, durante una sesión de prensa. “En Cerebras estamos muy, muy emocionados de anunciar nuestra primera asociación HyperScaler CSP para ofrecer una inferencia ultra rápida a todos los desarrolladores”.
La asociación marca la entrada formal de Meta en el negocio de la venta de AI Computation, transformando sus populares modelos de llama de código abierto en un servicio comercial. Si bien los modelos de LLAMA de Meta se han acumulado en mil millones de descargas, hasta ahora la compañía no había ofrecido una infraestructura en la nube de primera parte para que los desarrolladores creen aplicaciones con ellos.
“Esto es muy emocionante, incluso sin hablar sobre cerebras específicamente”, dijo James Wang, un ejecutivo senior de Cerebras. “Openai, Anthrope, Google: han construido un nuevo negocio de IA completamente nuevo desde cero, que es el negocio de inferencia de IA. Los desarrolladores que están construyendo aplicaciones de IA comprarán tokens por millones, a veces por miles de millones. Y estas son como las nuevas instrucciones de cómputo que las personas necesitan para construir aplicaciones AI”.
Breaking the Speed Barrier: Cómo modelos de Llama de Cerebras Supercharges
Lo que distingue a la oferta de Meta es el aumento de la velocidad dramática proporcionado por los chips de IA especializados de Cerebras. El sistema de cerebras ofrece más de 2.600 fichas por segundo para Llama 4 Scout, en comparación con aproximadamente 130 tokens por segundo para ChatGPT y alrededor de 25 tokens por segundo para Deepseek, según puntos de referencia del análisis artificial.
“Si solo se compara con API a API, Gemini y GPT, todos son grandes modelos, pero todos se ejecutan a velocidades de GPU, que son aproximadamente 100 tokens por segundo”, explicó Wang. “Y 100 tokens por segundo están bien para el chat, pero es muy lento para el razonamiento. Es muy lento para los agentes. Y la gente está luchando con eso hoy”.
Esta ventaja de velocidad permite categorías completamente nuevas de aplicaciones que antes no eran prácticas, incluidos los agentes en tiempo real, los sistemas de voz de baja latencia conversacional, la generación de código interactivo y el razonamiento instantáneo de múltiples pasos, todos los cuales requieren encadenamiento de múltiples llamadas de modelo de lenguaje grandes que ahora se pueden completar en segundos en lugar de minutos.
La API de LLAMA representa un cambio significativo en la estrategia de IA de Meta, en la transición de ser un proveedor de modelos a convertirse en una compañía de infraestructura de IA de servicio completo. Al ofrecer un servicio API, Meta está creando un flujo de ingresos a partir de sus inversiones de IA mientras mantiene su compromiso de abrir modelos.
“Meta ahora está en el negocio de vender tokens, y es excelente para el tipo de ecosistema de IA estadounidense”, señaló Wang durante la conferencia de prensa. “Traen mucho a la mesa”.
La API ofrecerá herramientas para el ajuste y la evaluación, comenzando con el modelo LLAMA 3.3 8B, permitiendo a los desarrolladores generar datos, entrenar y probar la calidad de sus modelos personalizados. Meta enfatiza que no utilizará datos de clientes para capacitar a sus propios modelos, y los modelos construidos con la API de LLAMA se pueden transferir a otros hosts, una clara diferenciación de los enfoques más cerrados de algunos competidores.
Las cerebras alimentarán el nuevo servicio de Meta a través de su red de centros de datos ubicados en toda América del Norte, incluidas las instalaciones en Dallas, Oklahoma, Minnesota, Montreal y California.
“Todos nuestros centros de datos que sirven a la inferencia están en América del Norte en este momento”, explicó Choi. “Serviremos Meta con toda la capacidad de las cerebras. La carga de trabajo se equilibrará en todos estos diferentes centros de datos”.
El arreglo comercial sigue lo que Choi describió como “el proveedor de cómputo clásico para un modelo hiperscalador”, similar a la forma en que NVIDIA proporciona hardware a los principales proveedores de la nube. “Están reservando bloques de nuestro cómputo para que puedan servir a su población de desarrolladores”, dijo.
Más allá de las cerebras, Meta también ha anunciado una asociación con Groq para proporcionar opciones de inferencia rápida, brindando a los desarrolladores múltiples alternativas de alto rendimiento más allá de la inferencia tradicional basada en GPU.
La entrada de Meta en el mercado de API de inferencia con métricas de rendimiento superiores podría potencialmente alterar el orden establecido dominado por Operai, Google y Anthrope. Al combinar la popularidad de sus modelos de código abierto con capacidades de inferencia dramáticamente más rápidas, Meta se está posicionando como un competidor formidable en el espacio comercial de IA.
“Meta está en una posición única con 3 mil millones de usuarios, centros de datos de hiper escala y un gran ecosistema de desarrolladores”, según los materiales de presentación de Cerebras. La integración de la tecnología de cerebras “ayuda a Meta Leapfrog OpenAi y Google en rendimiento en aproximadamente 20X”.
Para las cerebras, esta asociación representa un hito importante y la validación de su enfoque especializado de hardware de IA. “Hemos estado construyendo este motor a escala de obleas durante años, y siempre supimos que la primera tarifa de la tecnología, pero en última instancia tiene que terminar como parte de la nube de hiperescala de otra persona. Ese fue el objetivo final desde una perspectiva de estrategia comercial, y finalmente hemos alcanzado ese hito”, dijo Wang.
La API de LLAMA está actualmente disponible como una vista previa limitada, con Meta planifica un despliegue más amplio en las próximas semanas y meses. Los desarrolladores interesados en acceder a la inferencia Ultra-Fast Llama 4 pueden solicitar el acceso temprano seleccionando cerebras de las opciones del modelo dentro de la API de LLAMA.
“Si te imaginas a un desarrollador que no sabe nada sobre cerebras porque somos una empresa relativamente pequeña, solo pueden hacer clic en dos botones en el SDK estándar de SDK estándar de Meta, generar una tecla API, seleccionar la bandera de cerebras y luego, de repente, sus tokens se procesan en un motor gigante a escala de dafers”, explicó las cejas. “Ese tipo de hacernos estar en el back -end del ecosistema de desarrolladores de Meta todo el ecosistema es tremendo para nosotros”.
La elección de Meta de silicio especializada señala algo profundo: en la siguiente fase de la IA, no es solo lo que saben sus modelos, sino lo rápido que pueden pensarlo. En ese futuro, la velocidad no es solo una característica, es todo el punto.
Noticias
Todo lo que necesitas saber
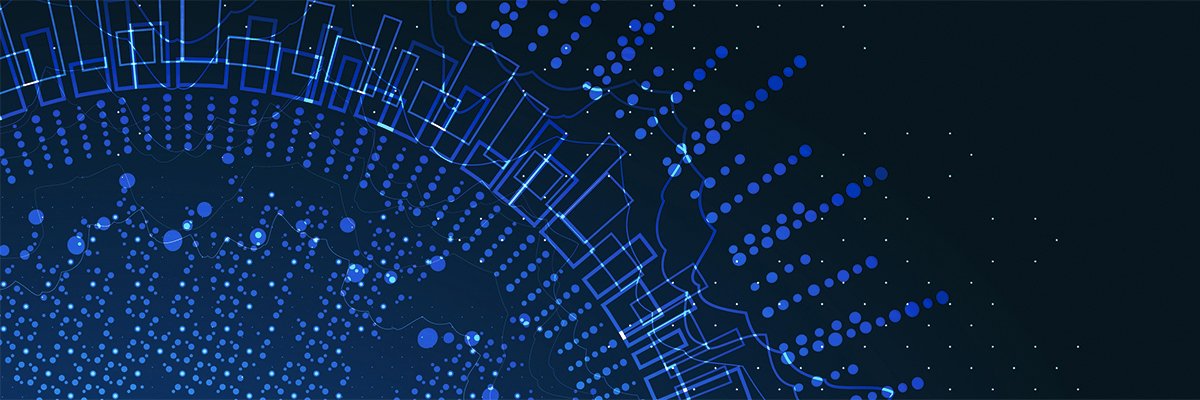
El ritmo rápido de la innovación generativa de IA coloca en los proveedores que empujan nuevos modelos de idiomas grandes (LLM) aparentemente sin pausa.
Entre estos destacados proveedores de LLM está Google. Su familia Gemini Model es el modelo de lenguaje sucesor de Pathways (Palm). Google Gemini debutó en diciembre de 2023 con el lanzamiento de 1.0, y Gemini 1.5 Pro siguió en febrero de 2024. Gemini 2.0, anunciado en diciembre de 2024, estuvo disponible en febrero de 2025. El 25 de marzo de 2025, Google anunció Gemini 2.5 Pro Experimental, continuó el ritmo rápido de la innovación.
El modelo Google Gemini 2.5 Pro ingresó al panorama de LLM a medida que su mercado cambia hacia modelos de razonamiento, como Deepseek R1 y Open AI’s O3, así como modelos de razonamiento híbridos, incluido el soneto 3.7 de Anthrope’s Claude.
¿Qué es Gemini 2.5 Pro?
Gemini 2.5 Pro es un LLM desarrollado por Google Deepmind. Cuando debutó en marzo de 2025, fue el modelo de IA más avanzado de Google, superando las capacidades y el rendimiento de las iteraciones anteriores de Gemini.
Al igual que con Gemini 2.0, Gemini 2.5 Pro es un LLM multimodal, lo que significa que no es simplemente para texto. Procesa y analiza texto, imágenes, audio y video. El modelo también tiene fuertes capacidades de codificación, superando los modelos de Géminis anteriores.
El modelo Gemini 2.5 Pro es el primero de la serie Gemini en ser construido especialmente como un “modelo de pensamiento” con la funcionalidad de razonamiento avanzado como capacidad central. En algunos aspectos, el modelo Gemini 2.5 Pro se basa en una versión de Gemini 2.0, Flash Thinking, que proporciona capacidades de razonamiento limitadas. Los modelos avanzados como Gemini 2.5 Pro usan un razonamiento de más tiempo a través de o “pensar” a través de los pasos requeridos para ejecutar un aviso, superando la mera institución de la cadena de pensamiento para permitir una producción más matizada, a menudo con mayor profundidad y precisión.
Google aplicó técnicas avanzadas, incluidos el aprendizaje de refuerzo y el mejor entrenamiento posterior, para aumentar el rendimiento de Gemini 2.5 Pro sobre los modelos anteriores. El modelo se lanzó con una ventana de contexto de un millón, con planes de expandirse a 2 millones de tokens.
¿Qué hay de nuevo en Gemini 2.5 Pro?
Las nuevas capacidades de Gemini 2.5 Pro y la funcionalidad mejorada elevan a la familia Google Gemini LLM.
Las mejoras clave incluyen lo siguiente:
- Razonamiento mejorado. La función principal de Gemini 2.5 Pro es su capacidad de razonamiento mejorada. Según Google, Gemini 2.5 Pro supera a Openai O3, el soneto antrópico Claude 3.7 y Deepseek R1 sobre los puntos de referencia de razonamiento y conocimiento, incluido el último examen de la humanidad.
- Capacidades de codificación avanzadas. Según Google, Gemini 2.5 Pro también supera las iteraciones anteriores en términos de capacidades de codificación. Similar a sus predecesores, este modelo genera y depura el código y crea aplicaciones visualmente atractivas. El modelo admite la generación y ejecución del código, lo que le permite probar y refinar sus soluciones. Gemini 2.5 Pro calificó 63.8% en SWE-Bench Verified, un estándar de la industria para evaluaciones de código de agente, con una configuración de agente personalizado que supera el soneto de Claude 3.7 de OpenAI GPT-4.5.
- Habilidades avanzadas de matemáticas y ciencias. Google también afirma mejorar las capacidades de matemáticas y ciencias. En el punto de referencia de matemáticas AIME 2025, Gemini 2.5 Pro obtuvo un 86.7%; En el referencia de GPQA Diamond Science, logró un 84%. Ambos puntajes superaron a sus rivales.
- Multimodalidad nativa. Sobre la base de fortalezas familiares, Gemini 2.5 Pro mantiene capacidades multimodales nativas, lo que permite la comprensión y el trabajo con textos, audio, imágenes, video y repositorios completos de código.
- Procesamiento en tiempo real. A pesar del aumento de las capacidades, el modelo mantiene una latencia razonable, lo que lo hace adecuado para aplicaciones en tiempo real y casos de uso interactivo.
¿Cómo mejora Gemini 2.5 Pro Google?
El modelo Gemini 2.5 Pro mejora los servicios de Google, y su posición entre los compañeros, de las siguientes maneras:
Liderazgo competitivo
El altamente competitivo LLM Market presenta a los principales competidores globales: Meta’s Llama Family, OpenAI’s GPT-4O y O3, Claude de Anthrope y Xai’s Grok, además de profundos de China, todos compiten por la participación de mercado. En su lanzamiento, Gemini 2.5 Pro inmediatamente se disparó a la cima de la clasificación de LLM Arena para la evaluación comparativa de IA, mejorando su posición como desarrollador líder de LLM para que las organizaciones lo consideren.
Mejores resultados en las aplicaciones de Google
En el lanzamiento, Gemini 2.5 Pro no se integró en el suite de productos de Google, incluidas las aplicaciones de búsqueda de búsqueda y Google Works. Sin embargo, su integración exitosa promete mejorar múltiples servicios. Para la búsqueda de Google, las capacidades de razonamiento mejoradas proporcionan respuestas más matizadas y precisas a consultas complejas. En Google Docs y otras aplicaciones del espacio de trabajo, la comprensión mejorada del contexto del modelo permite un análisis de documentos y generación de contenido más sofisticados.
Enfoque de desarrollador
Las habilidades de ejecución y generación de códigos avanzados del modelo también fortalecen la posición de Google en las herramientas y servicios del desarrollador, mejorando las llamadas de funciones y la automatización del flujo de trabajo en los servicios en la nube de Google.
Usos para Gemini 2.5 Pro
Gemini 2.5 Pro admite una variedad de tareas, que incluyen:
- Pregunta y respuesta. Gemini es un recurso para las interacciones de conocimiento de preguntas y respuestas fundamentales, basándose en los datos de capacitación de Google.
- Resumen de contenido multimodal. Como modelo multimodal, Gemini 2.5 Pro resume el contenido de texto, audio o video de forma larga.
- Respuesta de preguntas multimodales. El modelo combina información de texto, imágenes, audio y video para responder preguntas que abarcan múltiples modalidades.
- Generación de contenido de texto. Similar a sus predecesores, Gemini 2.5 Pro maneja la generación de texto.
- Resolución compleja de problemas. Con sus capacidades de razonamiento avanzado, Gemini 2.5 Pro administra tareas que requieren razonamiento lógico, como matemáticas, ciencias y análisis estructurado.
- Investigación profunda. La ventana de contexto extendida del modelo y las capacidades de razonamiento lo hacen ideal para analizar documentos largos, sintetizar información de múltiples fuentes y realizar investigaciones en profundidad.
- Tareas de codificación avanzadas. Gemini 2.5 Pro genera y depura el código que admite tareas de desarrollo de aplicaciones.
- Ai de agente. El razonamiento avanzado, las llamadas de funciones y el uso de la herramienta del modelo respaldan su valor como parte de un flujo de trabajo de AI agente.
¿Qué plataformas aceptan la integración Gemini 2.5 Pro?
Siguiendo los pasos de la familia Gemini, Gemini 2.5 está establecido para la integración en una serie de servicios de Google, que incluyen:
- Google AI Studio. En el lanzamiento, el nuevo modelo está disponible con Google AI Studio, una herramienta basada en la web que permite a los desarrolladores probar modelos directamente en el navegador.
- Aplicación Géminis. En el menú de selección del modelo desplegable, los suscriptores del servicio avanzado de Gemini pueden acceder al modelo a través de la aplicación Gemini en las plataformas de escritorio y móviles.
- Vértice ai. Google planea poner a disposición Gemini 2.5 Pro con su plataforma Vertex AI, lo que permite a las empresas utilizar el modelo para implementaciones a mayor escala.
- API GEMINI. Aunque no estaba disponible en el lanzamiento, todas las versiones anteriores de Gemini estaban disponibles utilizando una interfaz de programación de aplicaciones que permite a los desarrolladores integrar el modelo directamente en sus aplicaciones.
Sean Michael Kerner es un consultor de TI, entusiasta de la tecnología y tinkerer. Ha sacado el anillo de tokens, configurado NetWare y se sabe que compiló su propio kernel Linux. Consulta con organizaciones de la industria y los medios de comunicación sobre temas de tecnología.
-
Startups11 meses ago
Remove.bg: La Revolución en la Edición de Imágenes que Debes Conocer
-
Tutoriales12 meses ago
Cómo Comenzar a Utilizar ChatGPT: Una Guía Completa para Principiantes
-
Recursos12 meses ago
Cómo Empezar con Popai.pro: Tu Espacio Personal de IA – Guía Completa, Instalación, Versiones y Precios
-
Startups10 meses ago
Startups de IA en EE.UU. que han recaudado más de $100M en 2024
-
Startups12 meses ago
Deepgram: Revolucionando el Reconocimiento de Voz con IA
-
Recursos11 meses ago
Perplexity aplicado al Marketing Digital y Estrategias SEO
-
Recursos12 meses ago
Suno.com: La Revolución en la Creación Musical con Inteligencia Artificial
-
Noticias10 meses ago
Dos periodistas octogenarios deman a ChatGPT por robar su trabajo