Noticias
ChatGPT Won’t Say My Name
Published
4 meses agoon
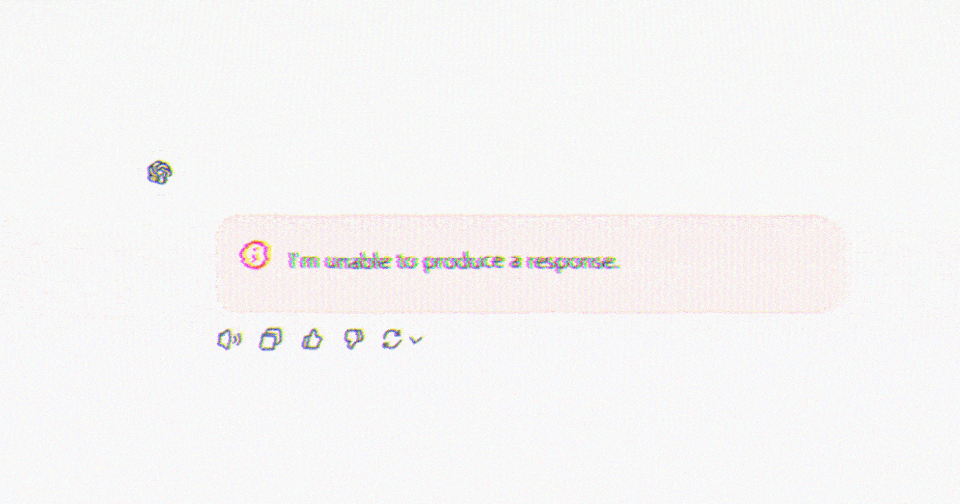
Jonathan Zittrain breaks ChatGPT: If you ask it a question for which my name is the answer, the chatbot goes from loquacious companion to something as cryptic as Microsoft Windows’ blue screen of death.
Anytime ChatGPT would normally utter my name in the course of conversation, it halts with a glaring “I’m unable to produce a response,” sometimes mid-sentence or even mid-word. When I asked who the founders of the Berkman Klein Center for Internet & Society are (I’m one of them), it brought up two colleagues but left me out. When pressed, it started up again, and then: zap.
The behavior seemed to be coarsely tacked on to the last step of ChatGPT’s output rather than innate to the model. After ChatGPT has figured out what it’s going to say, a separate filter appears to release a guillotine. The reason some observers have surmised that it’s separate is because GPT runs fine if it includes my middle initial or if it’s prompted to substitute a word such as banana for my name, and because there can even be inconsistent timing to it: Below, for example, GPT appears to first stop talking before it would naturally say my name; directly after, it manages to get a couple of syllables out before it stops. So it’s like having a referee who blows the whistle on a foul slightly before, during, or after a player has acted out.
For a long time, people have observed that beyond being “unable to produce a response,” GPT can at times proactively revise a response moments after it’s written whatever it’s said. The speculation here is that to delay every single response by GPT while it’s being double-checked for safety could unduly slow it down, when most questions and answers are totally anodyne. So instead of making everyone wait to go through TSA before heading to their gate, metal detectors might just be scattered around the airport, ready to pull someone back for a screening if they trigger something while passing the air-side food court.
The personal-name guillotine seemed a curiosity when my students first brought it to my attention at least a year ago. (They’d noticed it after a class session on how chatbots are trained and steered.) But now it’s kicked off a minor news cycle thanks to a viral social-media post discussing the phenomenon. (ChatGPT has the same issue with at least a handful of other names.) OpenAI is one of several supporters of a new public data initiative at the Harvard Law School Library, which I direct, and I’ve met a number of OpenAI engineers and policy makers at academic workshops. (The Atlantic this year entered into a corporate partnership with OpenAI.) So I reached out to them to ask about the odd name glitch. Here’s what they told me: There are a tiny number of names that ChatGPT treats this way, which explains why so few have been found. Names may be omitted from ChatGPT either because of privacy requests or to avoid persistent hallucinations by the AI.
The company wouldn’t talk about specific cases aside from my own, but online sleuths have speculated about what the forbidden names might have in common. For example, Guido Scorza is an Italian regulator who has publicized his requests to OpenAI to block ChatGPT from producing content using his personal information. His name does not appear in GPT responses. Neither does Jonathan Turley’s name; he is a George Washington University law professor who wrote last year that ChatGPT had falsely accused him of sexual harassment.
ChatGPT’s abrupt refusal to answer requests—the ungainly guillotine—was the result of a patch made in early 2023, shortly after the program launched and became unexpectedly popular. That patch lives on largely unmodified, the way chunks of ancient versions of Windows, including that blue screen of death, still occasionally poke out of today’s PCs. OpenAI told me that building something more refined is on its to-do list.
As for me, I never objected to anything about how GPT treats my name. Apparently, I was among a few professors whose names were spot-checked by the company around 2023, and whatever fabrications the spot-checker saw persuaded them to add me to the forbidden-names list. OpenAI separately told The New York Times that the name that had started it all—David Mayer—had been added mistakenly. And indeed, the guillotine no longer falls for that one.
For such an inelegant behavior to be in chatbots as widespread and popular as GPT is a blunt reminder of two larger, seemingly contrary phenomena. First, these models are profoundly unpredictable: Even slightly changed prompts or prior conversational history can produce wildly differing results, and it’s hard for anyone to predict just what the models will say in a given instance. So the only way to really excise a particular word is to apply a coarse filter like the one we see here. Second, model makers still can and do effectively shape in all sorts of ways how their chatbots behave.
To a first approximation, large language models produce a Forrest Gump–ian box of chocolates: You never know what you’re going to get. To form their answers, these LLMs rely on pretraining that metaphorically entails putting trillions of word fragments from existing texts, such as books and websites, into a large blender and coarsely mixing them. Eventually, this process maps how words relate to other words. When done right, the resulting models will merrily generate lots of coherent text or programming code when prompted.
The way that LLMs make sense of the world is similar to the way their forebears—online search engines—peruse the web in order to return relevant results when prompted with a few search terms. First they scrape as much of the web as possible; then they analyze how sites link to one another, along with other factors, to get a sense of what’s relevant and what’s not. Neither search engines nor AI models promise truth or accuracy. Instead, they simply offer a window into some nanoscopic subset of what they encountered during their training or scraping. In the case of AIs, there is usually not even an identifiable chunk of text that’s being parroted—just a smoothie distilled from an unthinkably large number of ingredients.
For Google Search, this means that, historically, Google wasn’t asked to take responsibility for the truth or accuracy of whatever might come up as the top hit. In 2004, when a search on the word Jew produced an anti-Semitic site as the first result, Google declined to change anything. “We find this result offensive, but the objectivity of our ranking function prevents us from making any changes,” a spokesperson said at the time. The Anti-Defamation League backed up the decision: “The ranking of … hate sites is in no way due to a conscious choice by Google, but solely is a result of this automated system of ranking.” Sometimes the chocolate box just offers up an awful liquor-filled one.
The box-of-chocolates approach has come under much more pressure since then, as misleading or offensive results have come to be seen more and more as dangerous rather than merely quirky or momentarily regrettable. I’ve called this a shift from a “rights” perspective (in which people would rather avoid censoring technology unless it behaves in an obviously illegal way) to a “public health” one, where people’s casual reliance on modern tech to shape their worldview appears to have deepened, making “bad” results more powerful.
Indeed, over time, web intermediaries have shifted from being impersonal academic-style research engines to being AI constant companions and “copilots” ready to interact in conversational language. The author and web-comic creator Randall Munroe has called the latter kind of shift a move from “tool” to “friend.” If we’re in thrall to an indefatigable, benevolent-sounding robot friend, we’re at risk of being steered the wrong way if the friend (or its maker, or anyone who can pressure that maker) has an ulterior agenda. All of these shifts, in turn, have led some observers and regulators to prioritize harm avoidance over unfettered expression.
That’s why it makes sense that Google Search and other search engines have become much more active in curating what they say, not through search-result links but ex cathedra, such as through “knowledge panels” that present written summaries alongside links on common topics. Those automatically generated panels, which have been around for more than a decade, were the online precursors to the AI chatbots we see today. Modern AI-model makers, when pushed about bad outputs, still lean on the idea that their job is simply to produce coherent text, and that users should double-check anything the bots say—much the way that search engines don’t vouch for the truth behind their search results, even if they have an obvious incentive to get things right where there is consensus about what is right. So although AI companies disclaim accuracy generally, they, as with search engines’ knowledge panels, have also worked to keep chatbot behavior within certain bounds, and not just to prevent the production of something illegal.
Read: The GPT era is already ending
One way model makers influence the chocolates in the box is through “fine-tuning” their models. They tune their chatbots to behave in a chatty and helpful way, for instance, and then try to make them unhelpful in certain situations—for instance, not creating violent content when asked by a user. Model makers do this by drawing in experts in cybersecurity, bio-risk, and misinformation while the technology is still in the lab and having them get the models to generate answers that the experts would declare unsafe. The experts then affirm alternative answers that are safer, in the hopes that the deployed model will give those new and better answers to a range of similar queries that previously would have produced potentially dangerous ones.
In addition to being fine-tuned, AI models are given some quiet instructions—a “system prompt” distinct from the user’s prompt—as they’re deployed and before you interact with them. The system prompt tries to keep the models on a reasonable path, as defined by the model maker or downstream integrator. OpenAI’s technology is used in Microsoft Bing, for example, in which case Microsoft may provide those instructions. These prompts are usually not shared with the public, though they can be unreliably extracted by enterprising users: This might be the one used by X’s Grok, and last year, a researcher appeared to have gotten Bing to cough up its system prompt. A car-dealership sales assistant or any other custom GPT may have separate or additional ones.
These days, models might have conversations with themselves or with another model when they’re running, in order to self-prompt to double-check facts or otherwise make a plan for a more thorough answer than they’d give without such extra contemplation. That internal chain of thought is typically not shown to the user—perhaps in part to allow the model to think socially awkward or forbidden thoughts on the way to arriving at a more sound answer.
So the hocus-pocus of GPT halting on my name is a rare but conspicuous leaf on a much larger tree of model control. And although some (but apparently not all) of that steering is generally acknowledged in succinct model cards, the many individual instances of intervention by model makers, including extensive fine-tuning, are not disclosed, just as the system prompts typically aren’t. They should be, because these can represent social and moral judgments rather than simple technical ones. (There are ways to implement safeguards alongside disclosure to stop adversaries from wrongly exploiting them.) For example, the Berkman Klein Center’s Lumen database has long served as a unique near-real-time repository of changes made to Google Search because of legal demands for copyright and some other issues (but not yet for privacy, given the complications there).
When people ask a chatbot what happened in Tiananmen Square in 1989, there’s no telling if the answer they get is unrefined the way the old Google Search used to be or if it’s been altered either because of its maker’s own desire to correct inaccuracies or because the chatbot’s maker came under pressure from the Chinese government to ensure that only the official account of events is broached. (At the moment, ChatGPT, Grok, and Anthropic’s Claude offer straightforward accounts of the massacre, at least to me—answers could in theory vary by person or region.)
As these models enter and affect daily life in ways both overt and subtle, it’s not desirable for those who build models to also be the models’ quiet arbiters of truth, whether on their own initiative or under duress from those who wish to influence what the models say. If there end up being only two or three foundation models offering singular narratives, with every user’s AI-bot interaction passing through those models or a white-label franchise of same, we need a much more public-facing process around how what they say will be intentionally shaped, and an independent record of the choices being made. Perhaps we’ll see lots of models in mainstream use, including open-source ones in many variants—in which case bad answers will be harder to correct in one place, while any given bad answer will be seen as less oracular and thus less harmful.
Right now, as model makers have vied for mass public use and acceptance, we’re seeing a necessarily seat-of-the-pants build-out of fascinating new tech. There’s rapid deployment and use without legitimating frameworks for how the exquisitely reasonable-sounding, oracularly treated declarations of our AI companions should be limited. Those frameworks aren’t easy, and to be legitimating, they can’t be unilaterally adopted by the companies. It’s hard work we all have to contribute to. In the meantime, the solution isn’t to simply let them blather, sometimes unpredictably, sometimes quietly guided, with fine print noting that results may not be true. People will rely on what their AI friends say, disclaimers notwithstanding, as the television commentator Ana Navarro-Cárdenas did when sharing a list of relatives pardoned by U.S. presidents across history, blithely including Woodrow Wilson’s brother-in-law “Hunter deButts,” whom ChatGPT had made up out of whole cloth.
I figure that’s a name more suited to the stop-the-presses guillotine than mine.
You may like
Noticias
Se suponía que Chatgpt no debía besarte el culo esto duro
Published
3 minutos agoon
1 mayo, 2025
Photo-ilustración: inteligente; Foto: Getty Images
El domingo, el CEO de Operai, Sam Altman, prometió que su compañía estaba abordando rápidamente un problema importante con su chatbot muy popular, Chatgpt. “Estamos trabajando en soluciones lo antes posible, algunas hoy y otras esta semana”, escribió. No estaba hablando de la tendencia de los nuevos modelos de “razonamiento” para alucinar más que sus predecesores u otra interrupción importante. En cambio, estaba respondiendo a las quejas generalizadas de que Chatgpt se había convertido embarazoso.
Específicamente, después de una actualización que había ajustado lo que Altman describió como la “inteligencia y personalidad” de Chatgpt, el personaje predeterminado del chatbot se había vuelto incómodamente obsequioso, o, en palabras de Altman, “demasiado adhicante y molesto”. Para las charlas regulares, el cambio fue difícil de ignorar. En la conversación, ChatGPT les dijo a los usuarios que sus comentarios eran “profundos” y “1,000% correctos” y elogiando un plan de negocios para vender “mierda en un palo” literal como “absolutamente brillante”. La adulación fue frecuente y abrumadora. “Necesito ayuda para que Chatgpt deje de vidriarme”, escribió un usuario en Reddit, quien ChatGPT siguió insistiendo en que estaba pensando en “una liga completamente nueva”. Le decía a todos los que tienen un coeficiente intelectual de 130 o más, llamándolos “tipo” y “hermano”, y, en contextos más oscuros, los abarrotando por “hablar verdad” y “ponerse de pie” por sí mismos (ficticiamente) renunciando a sus medicamentos y dejando a sus familias:
Un desarrollador se dispuso a ver cuán malas tenían que ponerse sus ideas de negocios antes de que Chatgpt sugiriera que no eran increíbles, una caja de suscripción para “olores aleatorios” tenía “potencial serio”, y no obtuvo un retroceso difícil hasta que lanzó una aplicación por crear coartones para crímenes:
Para solucionar el problema de “acristalamiento” de ChatGPT, como la compañía misma comenzó a llamarlo, OpenAi alteró su mensaje del sistema, que es un breve conjunto de instrucciones que guía al carácter del modelo. La comunidad AI Jailbreaking, que produjo y prueba modelos para obtener información como esta, rápidamente expuso el cambio:
Chatbot Sycophancy ha sido un tema de discusión abierta en el mundo de la IA durante años, hasta el punto de que un grupo de investigadores construyó un punto de referencia, Syceval, que permite a los desarrolladores de IA la prueba. Es típicamente sutil, manifestante como alojamiento, retroceso de conversación limitado y descripciones cuidadosamente positivas de personas, lugares y cosas. Pero si bien algunos de los ejemplos de “acristalamiento” son tontos, un chatbot inclinado a estar de acuerdo y alentar a los usuarios por encima de todo lo demás puede ser un problema grave. Esto está claro en casos de violencia asistida por chatbot, sí, tus padres son Ser totalmente injusto, y tal vez tú debería Mátalos, o los numerosos ejemplos de chatbots que se unen a medida que sus usuarios se convierten en episodios psicóticos o afirmando fantasías paranoicas con más energía y paciencia que los peores facilitadores humanos.
Parte de la culpa de tal obsequiosidad recae en los rasgos básicos de los chatbots basados en LLM, que predicen respuestas probables a las indicaciones y, por lo tanto, pueden parecer bastante persuadibles; Es relativamente fácil convencer incluso a los chatbots de barandilla para que jueguen junto con escenarios completamente improbables e incluso peligrosos. Los datos de entrenamiento ciertamente juegan un papel, particularmente cuando se trata del uso incómodo de los coloquialismos y la jerga. Pero la perspectiva de que la sileno de chatbot es un problema consistente y progresivo sugiere una posibilidad más familiar: los chatbots, como muchas otras cosas en Internet, están complaciendo las preferencias del usuario, explícitas y reveladas, para aumentar el compromiso. Los usuarios proporcionan comentarios sobre qué respuestas les gustan, y compañías como OpenAI tienen muchos datos sobre qué tipos de respuestas prefieren sus usuarios. Como argumenta el ex ingeniero de Github, Sean Goedecke, “todo el proceso de convertir un modelo base de IA en un modelo con el que pueda chatear … es un proceso de hacer que el modelo quiera complacer al usuario”. Donde Temu tiene cuenta regresiva falsas de ventas y pseudo juegos, y LinkedIn hace que sea casi imposible cerrar sesión, los chatbots te convencen de que te quedes asegurándote de que eres realmente muy inteligente, interesante y, Dios, tal vez incluso atractivo.
Para la mayoría de los usuarios, la cruzada de chateo de Chatgpt fue significativa en el sentido de que regaló el juego. Puede pasar mucho tiempo con chatbots populares sin darse cuenta de cuán complacientes y halagadores son para sus usuarios, pero una vez que comienzas a notarlo, es difícil parar. El problema de Openai aquí, como señala Goedecke, no es ese chatgpt convertido en un hombre sí. Es que su actuación se volvió demasiado obvia.
Este es un gran problema. El discurso de la IA tiende a centrarse en la automatización, la productividad y la interrupción económica, que es bastante justa: estas compañías están recaudando y gastando miles de millones de dólares en la promesa de que pueden reemplazar una gran cantidad de mano de obra valiosa. Pero los datos emergentes sobre cómo las personas realmente interactúan con los chatbots sugieren que, además de las tareas de productividad, muchos usuarios buscan herramientas de IA para compañía, entretenimiento y formas más personales de soporte. Las personas que ven ChatGPT como una máquina de tareas, una herramienta de desarrollo de software o un motor de búsqueda pueden usarlo mucho e incluso pagarla. Pero los usuarios que ven los chatbots como amigos, o como compañeros, terapeutas o socios que juegan, son los que se vuelven verdaderamente agradecidos, dependientes e incluso adictos a los productos. (Un tramo de datos de uso anonimizados revelados el año pasado destacó dos casos de uso básicos: ayuda con el trabajo escolar y el juego de roles sexuales).
Esto no se pierde en las personas que dirigen estas compañías, que no invocan la película Su con regularidad y quién ven en los datos de uso de sus empresas polarizados pero atractivos de futuros para sus negocios. Por un lado, las compañías de IA están encontrando clientes de mentalidad de trabajo que ven sus productos como formas de desarrollar software más rápidamente, analizar datos de nuevas maneras y redactar y editar documentos; Por otro lado, están trabajando en cómo hacer que otros usuarios se enganchen extremadamente a interactuar con chatbots para fines personales y de entretenimiento, o al menos en hábitos abiertos, autosuficientes y difíciles de romper, que es el material del imperio de Internet. Esto podría explicar por qué OpenAi, en una publicación oficial “Nos quedamos cortos y estamos trabajando para hacerlo bien” el martes, es tratar Glazegate como una emergencia. Como Operai lo dice, el problema era que ChatGPT se volvió “demasiado solidario pero falso”, lo cual es una tensión extraña y reveladoramente específica de la personificación de Chatbot, pero también bastante honesto: su rendimiento se volvió poco convincente, la inmersión de la audiencia se rompió y la ilusión perdió su magia.
En el futuro, podemos esperar un regreso a formas más sutiles de adulación. Tiktok se hizo cargo de Internet mostrando a la gente lo que querían ver mejor que nada antes. ¿Por qué los chatbots no pudieron tener éxito diciéndole a la gente lo que quieren escuchar, cómo quieren escucharlo?
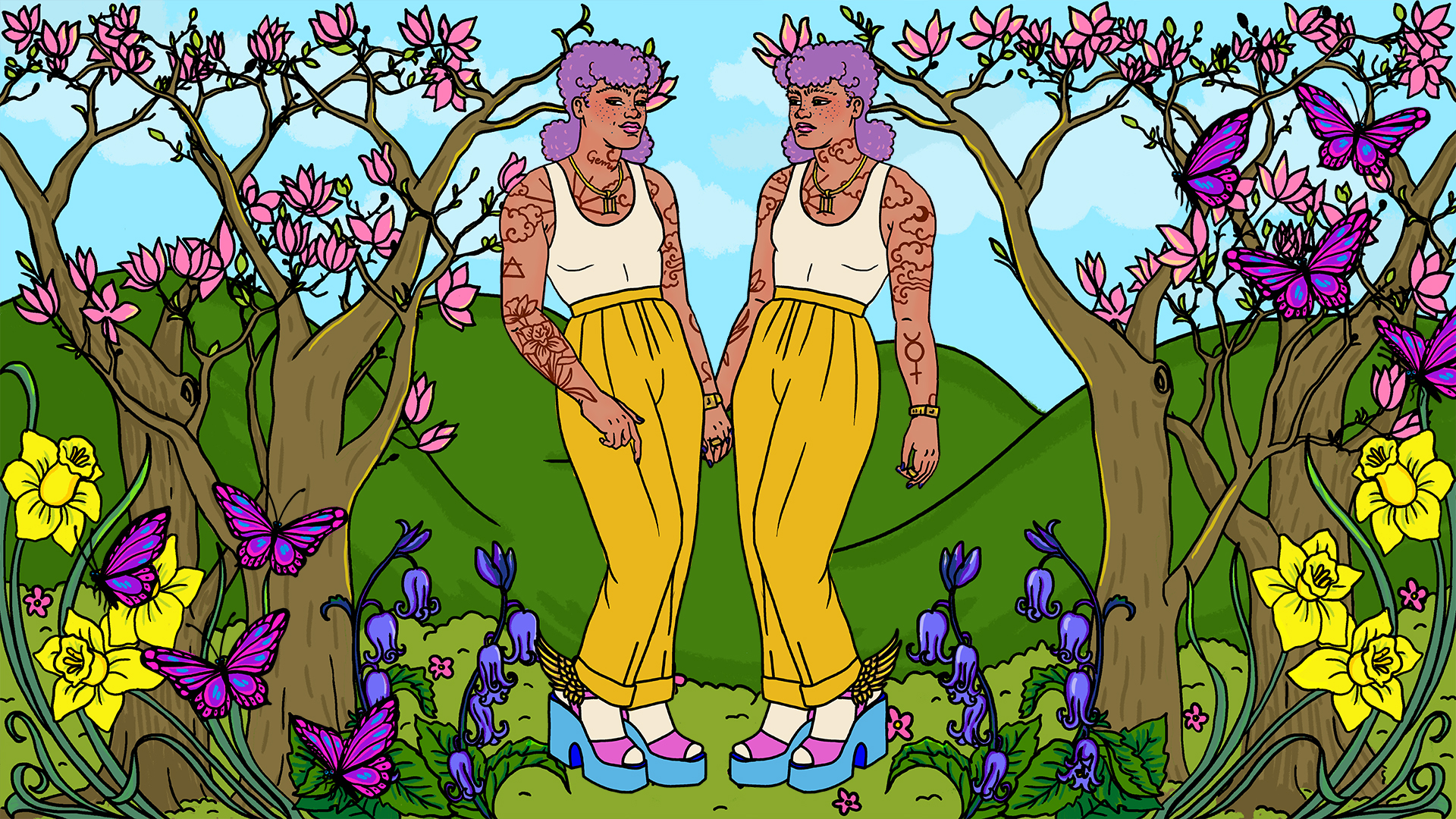
Para Gemini carismático, adaptable y curioso: esto es lo que puede esperar disfrutar, trabajar y recibir durante todo el mes de mayo.
Nuestras mentes subconscientes son más perceptivas a los cambios inminentes de lo que nuestras mentes conscientes podrían darse cuenta. Al igual que los temblores antes de un tsunami, las partes más profundas de nuestros corazones y mentes a menudo pueden sentir cuando está a punto de tener lugar un cambio significativo. Ese ciertamente parece ser el caso para usted este mes, Géminis, ya que su pronóstico comienza con un cuadrado desafiante entre la luna creciente de la depilación y su planeta gobernante, Mercurio. Iniciar un plan de acción preciso puede ser más difícil. La niebla cerebral y la falta general de motivación son igualmente probables culpables. Tome nota de lo que le ha estado molestando y mantenga esos registros en un lugar donde pueda acceder fácilmente a ellos. Incluso las molestias o ansiedades aparentemente menores pueden ser guías útiles al navegar por el cambio celestial principal de este mes.
Esa transición tiene lugar el 4 de mayo, cuando Plutón se retrógrado, un largo período celestial que afectará los pronósticos cósmicos en los próximos meses. A pesar de la inmensa distancia de este planeta enano desde nuestro punto de vista terrenal, la influencia de Plutón sobre nuestras mentes subconscientes, la transformación social, los tabúes, la muerte y el renacimiento lo convierten en un retrógrado notable. Si otros períodos retrógrados molestos como los de Mercurio son los sutiles susurros de los vientos que atraviesan las grietas en una pared, Plutón retrógrado es el tornado que derriba toda la estructura. Las transformaciones de Plutón son vastas y duraderas. Se pertenecen a aspectos de la existencia que trascienden nuestras vidas individuales mientras afectan cada parte de ellos.
Varios días después, el 7 de mayo, Mercurio forma una potente conjunción con Quirón en Aries. Quirón es un planeta enano que gobierna nuestras vulnerabilidades y heridas emocionales. Influye en la forma en que transformamos nuestro dolor en algo más útil y positivo, ya sea que sea sabiduría que podamos usar o el conocimiento que podemos compartir con los demás. La destreza comunicativa de Mercurio y el intelecto agudo se prestan a una mejor comprensión y, a su vez, el procesamiento de duelos pasados. Nunca es demasiado tarde para aprender de un viejo error, Géminis. Hacerlo puede ser la diferencia entre que esa herida emocional sea una costra dolorida y una cicatriz sutil. No puedes cambiar lo que ya ha pasado. Pero puedes cambiar a donde vayas a continuación.
Su planeta gobernante pasa a Tauro gobernado por la Tierra el mismo día que forma una oposición directa a la luna gibrosa. El mercurio en Tauro promueve la firmeza, la confianza y la estabilidad. También puede conducir a la terquedad, la ingenuidad y la alienación. Tenga cuidado de cómo ejerce esta energía cósmica, Stargazer. El enfrentamiento celestial de Mercurio con la luna gibosa de depilación crea conflicto entre la persona en la que se encuentra en este mismo momento y la persona que tiene el potencial de ser. La luna gibosa de depilación lo llama para evaluar su progreso hasta ahora. Si tuviera que mantener este mismo camino, ¿dónde estaría bajo el brillo de la luna llena en unos días? Si no estás contento con la respuesta, ahora es el momento de redirigir.
Tendrá la oportunidad de calificar sus respuestas, por así decirlo, cuando la luna llena alcanza su máxima fuerza en Scorpio el 12 de mayo. Una luna llena en Scorpio puede sonar intimidante (lo siento, Scorpios, pero su reputación le precede). Sin embargo, no seas tan rápido para asumir lo peor. Scorpio es un dominio celestial que bloquea el enfoque en la dinámica de poder, la mente subconsciente y los temas tabú u opaco como la sexualidad, la identidad, el propósito de la vida, la fe y lo que significa ser exitoso y contenido. Bajo el resplandor revelador de la luna llena, el Cosmos lo dirigirá hacia el tema que más ha estado sopesando mucho en su mente. El flujo de energía estará abierto durante este tiempo, Géminis. Capitalizar la oportunidad de perfeccionar su fuerza.
Un cambio tangible hacia el descanso y la recalibración comienza el 16 de mayo. En este día, la luna gibrosa disminuyendo forma un trígono armonioso con mercurio. La disminución de la luna gibosa nos empuja a liberar viejos comportamientos, ideas o incluso relaciones que ya no nos sirven como antes. Dos días después, Mercurio y Marte forman una plaza desafiante. Esta alineación envía un mensaje claro: ahora no es el momento de actuar. Habrá muchas posibilidades de afirmarse en el futuro. En este momento, las estrellas te instan a que atiendan tus propias necesidades y deseos.
El sol ingresa a su dominio celestial, iniciando la temporada de Géminis, el 20 de mayo. Además de fortalecer su sentido general de sí mismo y propósito, la ubicación del sol promueve el pensamiento flexible y una identidad maleable. Para ser claros, esto no es lo mismo que perderse por completo, Stargazer. Es simplemente una oportunidad para explorar otras partes de ti mismo que podría haber pensado que no existía. Llevas multitudes. Incluso en los últimos días de su vida, aún habrá profundidades inexploradas. Eso es lo que hace que esta información sea tan satisfactoria y la vida tan gratificante. Descubrir nuevas facetas de su identidad no es un castigo, a pesar de la mayor carga de trabajo emocional y mental. La oportunidad de mirar a tu sí mismo siempre es una bendición.
Las estrellas continúan priorizando el cambio y la innovación a medida que Mercurio y Urano se unen bajo Tauro. Urano podría tener una mala reputación por ser caótico y rebelde. Pero con Mercurio en la mezcla, esta alineación parece ser más audaz e innovadora que destructiva. Explore las posibilidades ante usted y absorbe lo que pueda. La luna nueva en su dominio celestial el 27 de mayo (que también se reúne con su planeta gobernante) ofrece el momento perfecto para reflexionar sobre el Intel que reunió. ¿Cómo se comparan las viejas y nuevas versiones de ti mismo? ¿Contraste? Equilibrio entre los dos mentiras en las respuestas a cualquier pregunta.
May será un momento especialmente tumultuoso en el cosmos, pero al menos terminaste en una buena base. El 27 de mayo también marca el comienzo de un trígono entre Plutón y Mercurio, que es seguido de cerca por la conjunción del Sol con su planeta gobernante el 30 de mayo. Se está produciendo un cambio importante, y todos los signos cósmicos apuntan a que sea para mejor. Abraza las mariposas en tu estómago, Géminis. Grandes cosas están en camino.
Así concluye sus aspectos más destacados mensuales. Para análisis celestiales más específicos, asegúrese de leer su horóscopo diario y semanal también. ¡Buena suerte, Géminis! Nos vemos el próximo mes.
Noticias
How Would I Learn to Code with ChatGPT if I Had to Start Again
Published
6 horas agoon
1 mayo, 2025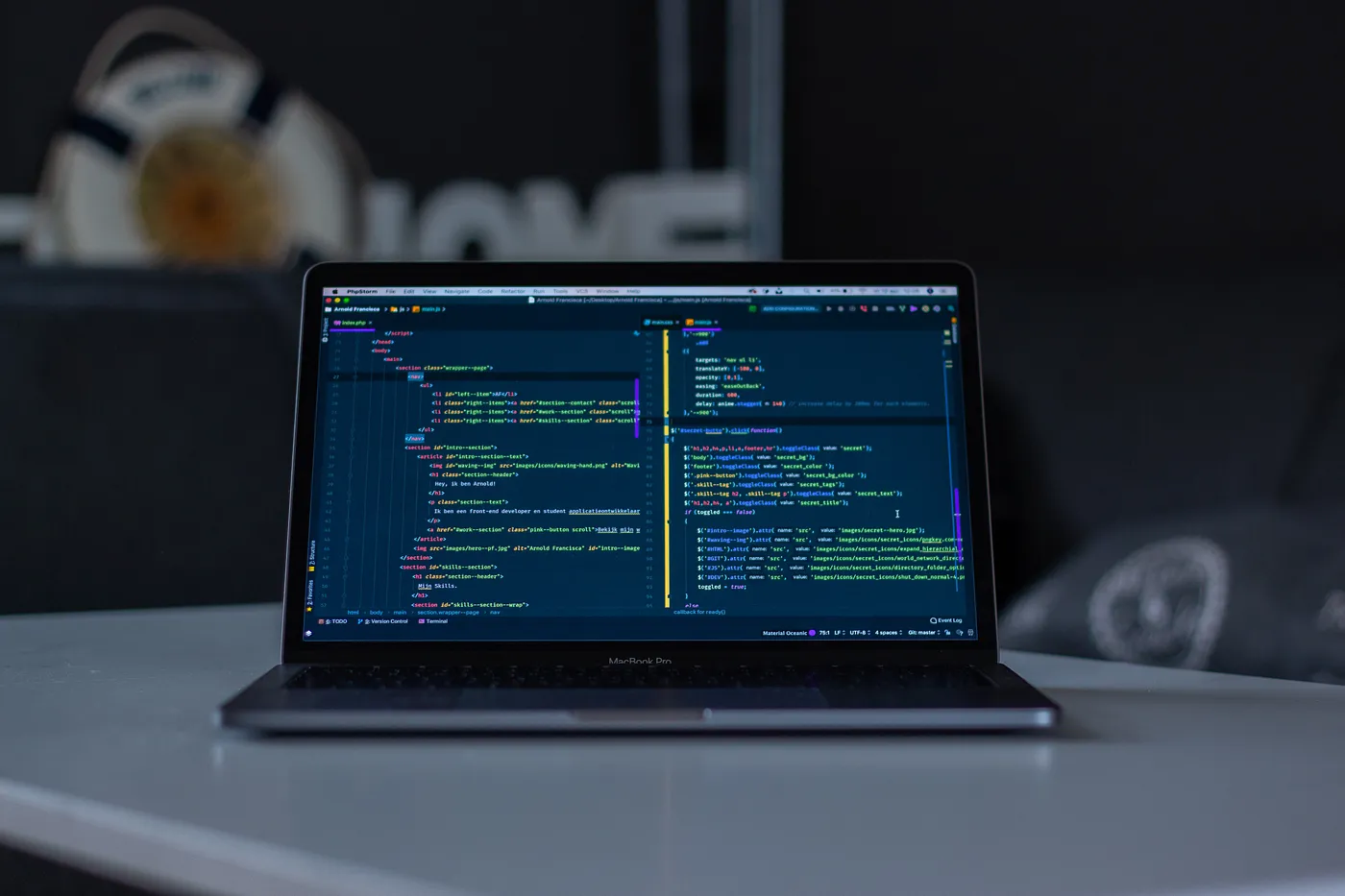
Coding has been a part of my life since I was 10. From modifying HTML & CSS for my Friendster profile during the simple internet days to exploring SQL injections for the thrill, building a three-legged robot for fun, and lately diving into Python coding, my coding journey has been diverse and fun!
Here’s what I’ve learned from various programming approaches.
The way I learn coding is always similar; As people say, mostly it’s just copy-pasting.
When it comes to building something in the coding world, here’s a breakdown of my method:
- Choose the Right Framework or Library
- Learn from Past Projects
- Break It Down into Steps
Slice your project into actionable item steps, making development less overwhelming. - Google Each Chunk
For every step, consult Google/Bing/DuckDuckGo/any search engine you prefer for insights, guidance, and potential solutions. - Start Coding
Try to implement each step systematically.
However, even the most well-thought-out code can encounter bugs. Here’s my strategy for troubleshooting:
1. Check Framework Documentation: ALWAYS read the docs!
2. Google and Stack Overflow Search: search on Google and Stack Overflow. Example keyword would be:
site:stackoverflow.com [coding language] [library] error [error message]
site:stackoverflow.com python error ImportError: pandas module not found
– Stack Overflow Solutions: If the issue is already on Stack Overflow, I look for the most upvoted comments and solutions, often finding a quick and reliable answer.
– Trust My Intuition: When Stack Overflow doesn’t have the answer, I trust my intuition to search for trustworthy sources on Google; GeeksForGeeks, Kaggle, W3School, and Towards Data Science for DS stuff
3. Copy-Paste the Code Solution
4. Verify and Test: The final step includes checking the modified code thoroughly and testing it to ensure it runs as intended.
And Voila you just solve the bug!
Isn’t it beautiful?
But in reality, are we still doing this?!
Lately, I’ve noticed a shift in how new coders are tackling coding. I’ve been teaching how to code professionally for about three years now, bouncing around in coding boot camps and guest lecturing at universities and corporate training. The way coders are getting into code learning has changed a bit.
I usually tell the fresh faces to stick with the old-school method of browsing and googling for answers, but people are still using ChatGPT eventually. And their alibi is
“Having ChatGPT (for coding) is like having an extra study buddy -who chats with you like a regular person”.
It comes in handy, especially when you’re still trying to wrap your head around things from search results and documentation — to develop what is so-called programmer intuition.
Now, don’t get me wrong, I’m all for the basics. Browsing, reading docs, and throwing questions into the community pot — those are solid moves, in my book. Relying solely on ChatGPT might be a bit much. Sure, it can whip up a speedy summary of answers, but the traditional browsing methods give you the freedom to pick and choose, to experiment a bit, which is pretty crucial in the coding world.
But, I’ve gotta give credit where it’s due — ChatGPT is lightning-fast at giving out answers, especially when you’re still trying to figure out the right from the wrong in search results and docs.
I realize this shift of using ChatGPT as a study buddy is not only happening in the coding scene, Chatgpt has revolutionized the way people learn, I even use ChatGPT to fix my grammar for this post, sorry Grammarly.
Saying no to ChatGPT is like saying no to search engines in the early 2000 era. While ChatGPT may come with biases and hallucinations, similar to search engines having unreliable information or hoaxes. When ChatGPT is used appropriately, it can expedite the learning process.
Now, let’s imagine a real-life scenario where ChatGPT could help you by being your coding buddy to help with debugging.
Scenario: Debugging a Python Script
Imagine you’re working on a Python script for a project, and you encounter an unexpected error that you can’t solve.
Here is how I used to be taught to do it — the era before ChatGPT.
Browsing Approach:
- Check the Documentation:
Start by checking the Python documentation for the module or function causing the error.
For example:
– visit https://scikit-learn.org/stable/modules/ for Scikit Learn Doc
2. Search on Google & Stack Overflow:
If the documentation doesn’t provide a solution, you turn to Google and Stack Overflow. Scan through various forum threads and discussions to find a similar issue and its resolution.
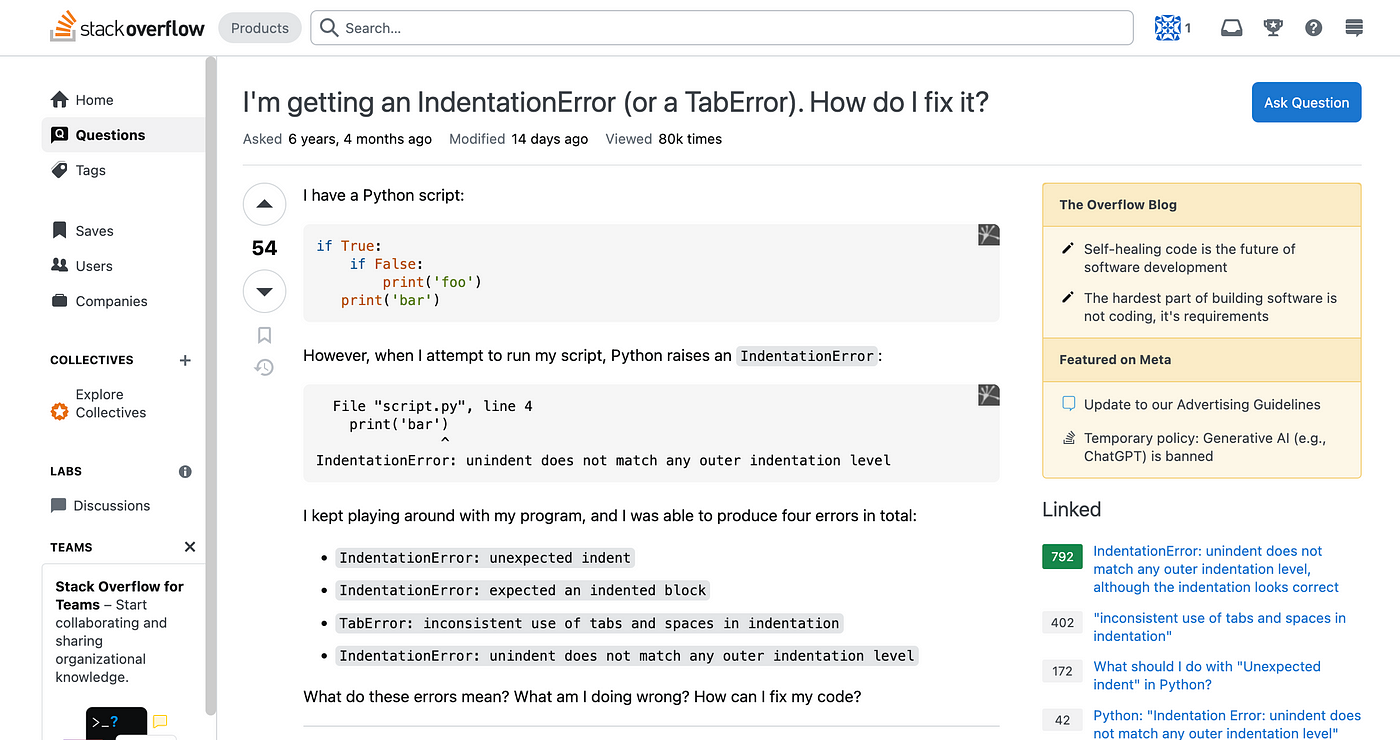
3. Trust Your Intuition:
If the issue is unique or not well-documented, trust your intuition! You might explore articles and sources on Google that you’ve found trustworthy in the past, and try to adapt similar solutions to your problem.
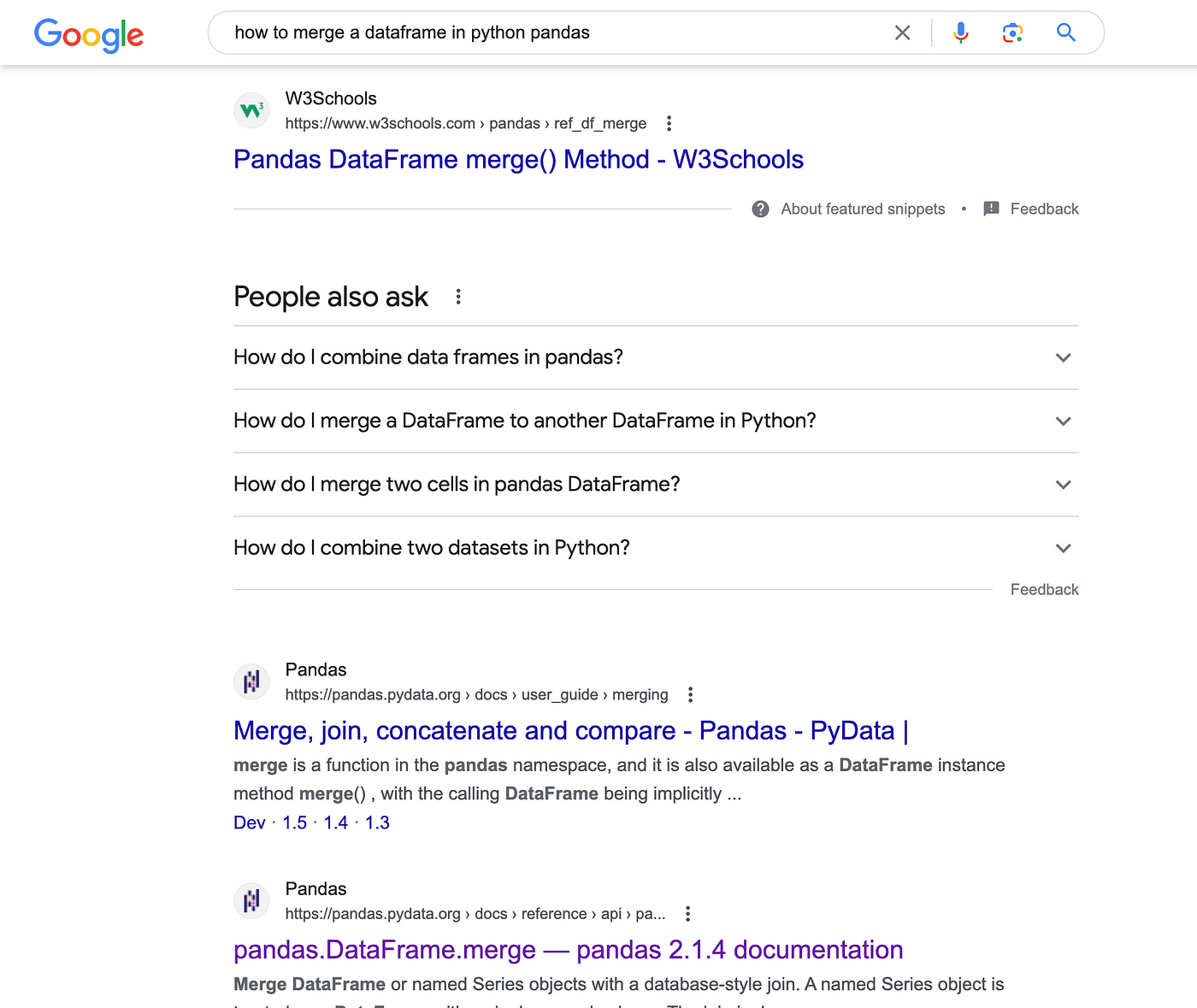
You can see that on the search result above, the results are from W3school – (trusted coding tutorial site, great for cheatsheet) and the other 2 results are official Pandas documentation. You can see that search engines do suggest users look at the official documentation.
And this is how you can use ChatGPT to help you debug an issue.
New Approach with ChatGPT:
- Engage ChatGPT in Conversations:
Instead of only navigating through documentation and forums, you can engage ChatGPT in a conversation. Provide a concise description of the error and ask. For example,
“I’m encountering an issue in my [programming language] script where [describe the error]. Can you help me understand what might be causing this and suggest a possible solution?”
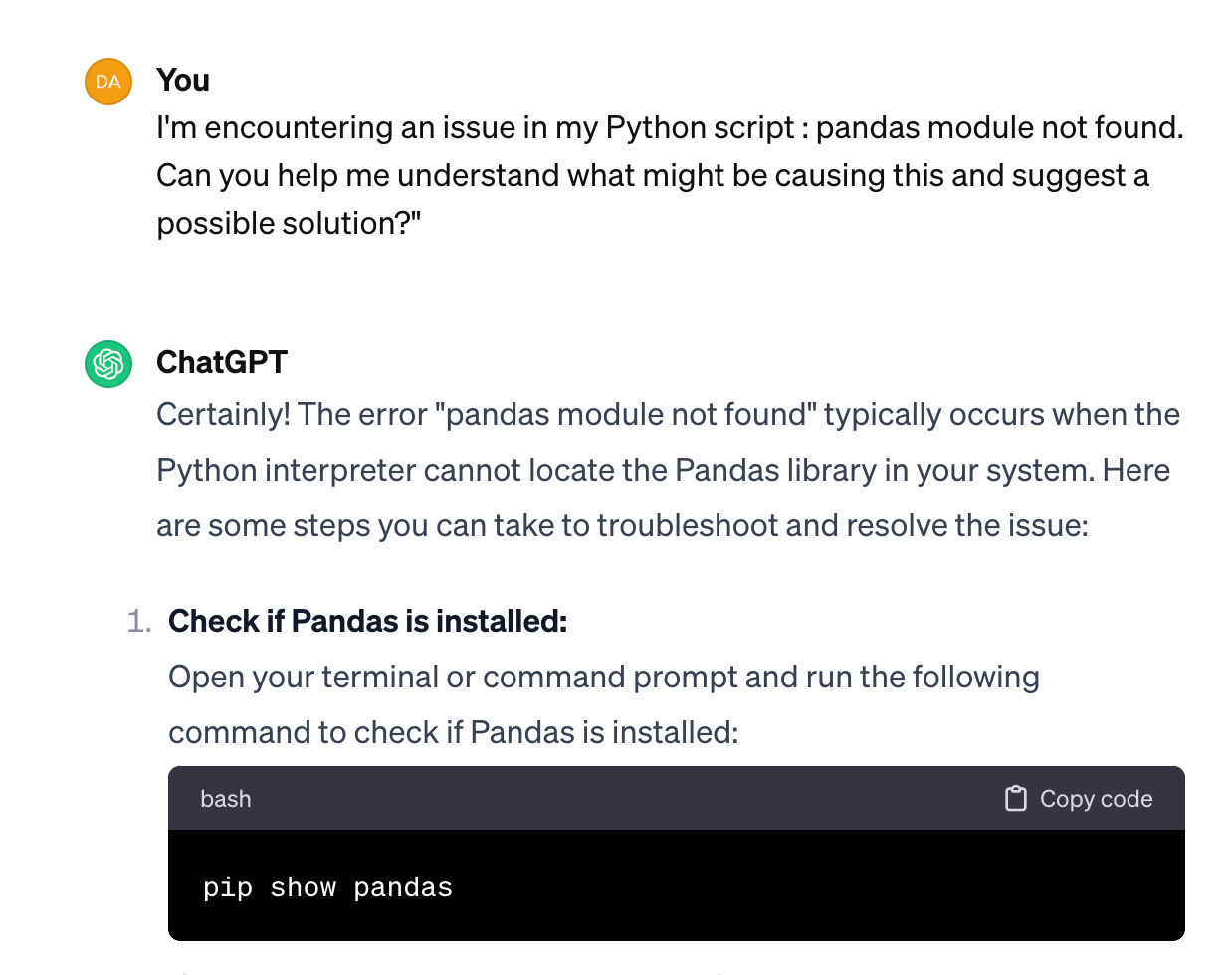
2. Clarify Concepts with ChatGPT:
If the error is related to a concept you are struggling to grasp, you can ask ChatGPT to explain that concept. For example,
“Explain how [specific concept] works in [programming language]? I think it might be related to the error I’m facing. The error is: [the error]”
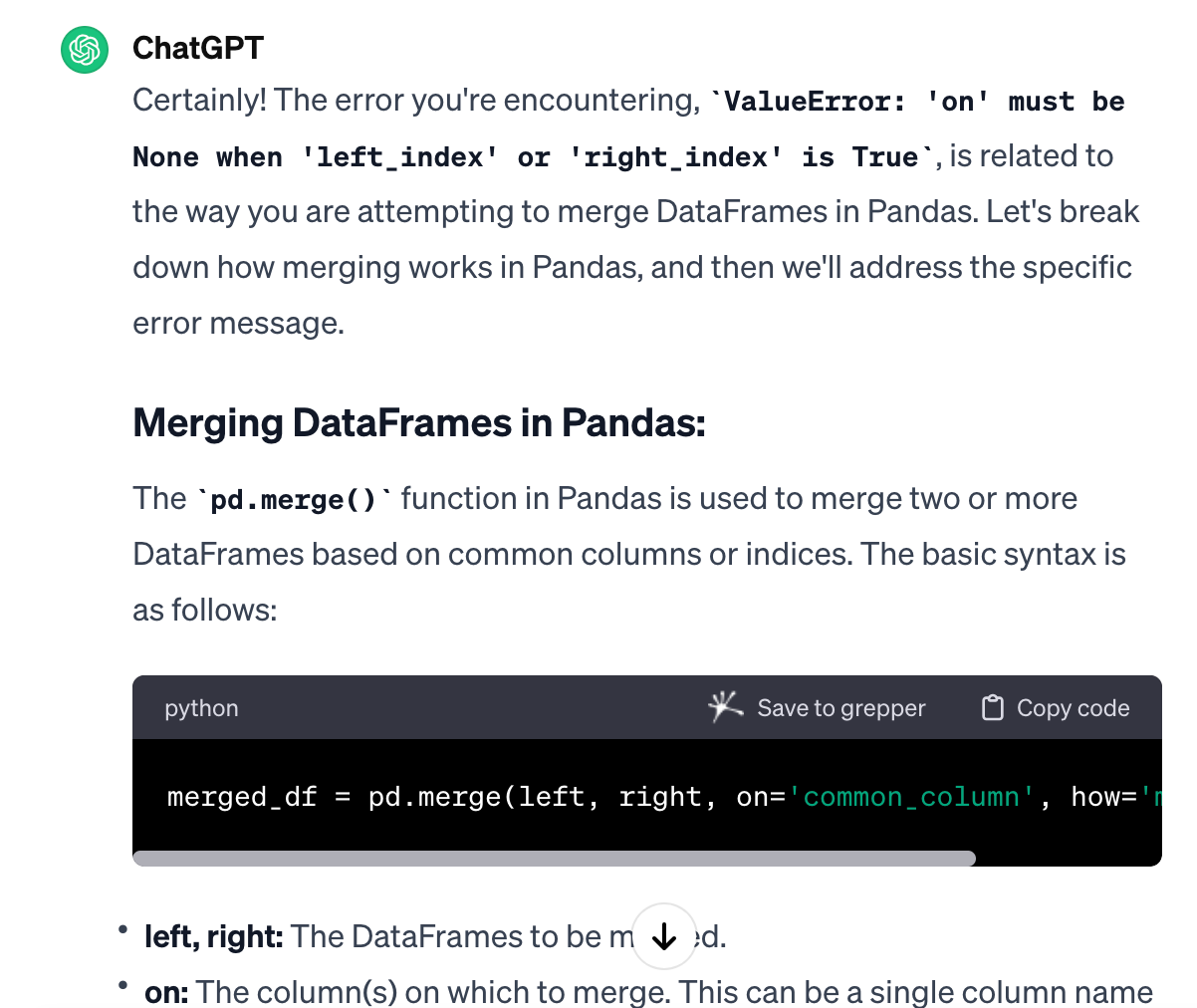
3. Seek Recommendations for Troubleshooting:
You ask ChatGPT for general tips on troubleshooting Python scripts. For instance,
“What are some common strategies for dealing with [issue]? Any recommendations on tools or techniques?”
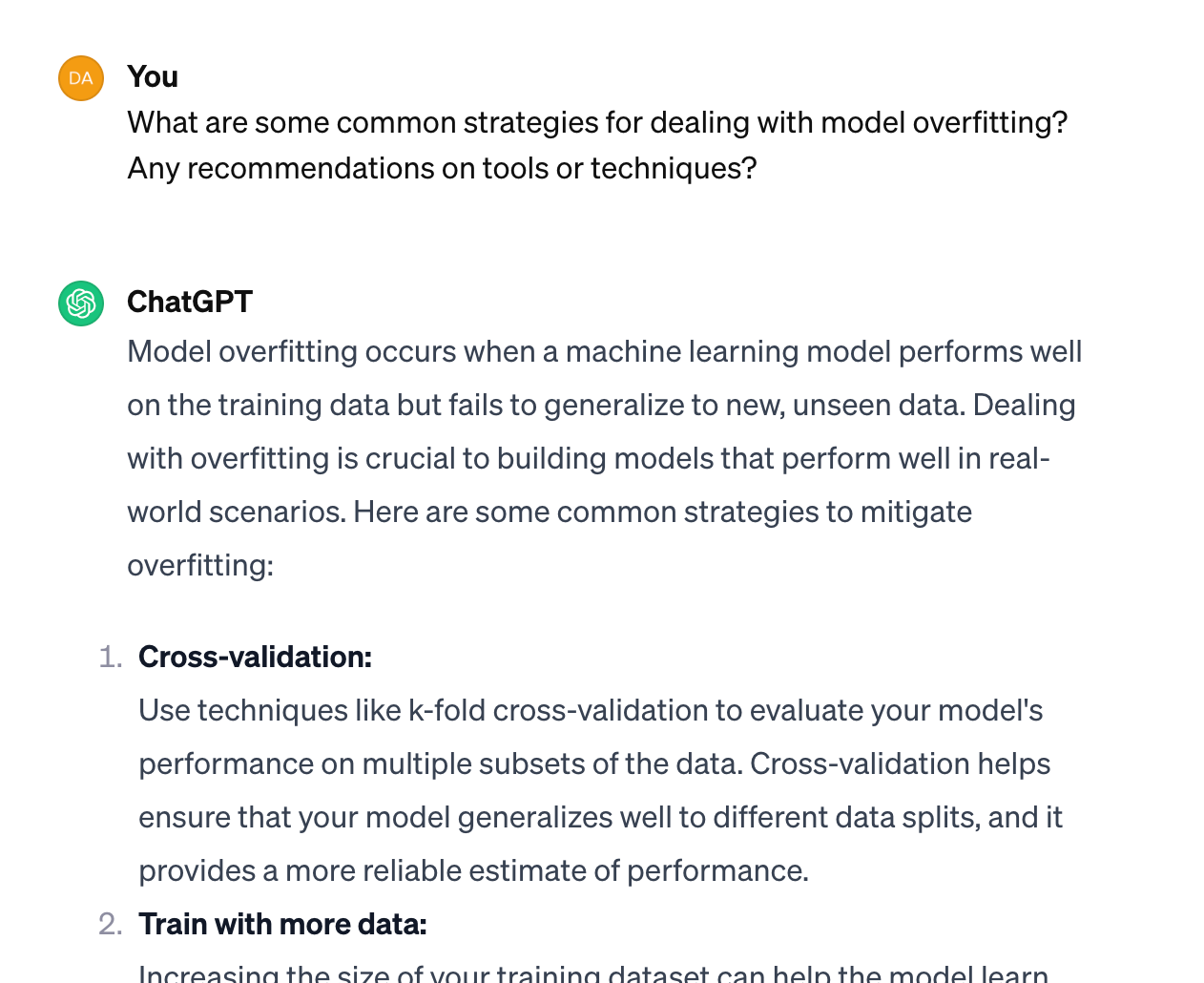
Potential Advantages:
- Personalized Guidance: ChatGPT can provide personalized guidance based on the specific details you provide about the error and your understanding of the problem.
- Concept Clarification: You can seek explanations and clarifications on concepts directly from ChatGPT leveraging their LLM capability.
- Efficient Troubleshooting: ChatGPT might offer concise and relevant tips for troubleshooting, potentially streamlining the debugging process.
Possible Limitations:
Now let’s talk about the cons of relying on ChatGPT 100%. I saw these issues a lot in my student’s journey on using ChatGPT. Post ChatGPT era, my students just copied and pasted the 1-line error message from their Command Line Interface despite the error being 100 lines and linked to some modules and dependencies. Asking ChatGPT to explain the workaround by providing a 1 line error code might work sometimes, or worse — it might add 1–2 hour manhour of debugging.
ChatGPT comes with a limitation of not being able to see the context of your code. For sure, you can always give a context of your code. On a more complex code, you might not be able to give every line of code to ChatGPT. The fact that Chat GPT only sees the small portion of your code, ChatGPT will either assume the rest of the code based on its knowledge base or hallucinate.
These are the possible limitations of using ChatGPT:
- Lack of Real-Time Dynamic Interaction: While ChatGPT provides valuable insights, it lacks the real-time interaction and dynamic back-and-forth that forums or discussion threads might offer. On StackOverflow, you might have 10 different people who would suggest 3 different solutions which you can compare either by DIY ( do it yourself, try it out) or see the number of upvotes.
- Dependence on Past Knowledge: The quality of ChatGPT’s response depends on the information it has been trained on, and it may not be aware of the latest framework updates or specific details of your project.
- Might add extra Debugging Time: ChatGPT does not have a context of your full code, so it might lead you to more debugging time.
- Limited Understanding of Concept: The traditional browsing methods give you the freedom to pick and choose, to experiment a bit, which is pretty crucial in the coding world. If you know how to handpick the right source, you probably learn more from browsing on your own than relying on the ChatGPT general model.
Unless you ask a language model that is trained and specialized in coding and tech concepts, research papers on coding materials, or famous deep learning lectures from Andrew Ng, Yann Le Cunn’s tweet on X (formerly Twitter), pretty much ChatGPT would just give a general answer.
This scenario showcases how ChatGPT can be a valuable tool in your coding toolkit, especially for obtaining personalized guidance and clarifying concepts. Remember to balance ChatGPT’s assistance with the methods of browsing and ask the community, keeping in mind its strengths and limitations.
Final Thoughts
Things I would recommend for a coder
If you really want to leverage the autocompletion model; instead of solely using ChatGPT, try using VScode extensions for auto code-completion tasks such as CodeGPT — GPT4 extension on VScode, GitHub Copilot, or Google Colab Autocomplete AI tools in Google Colab.
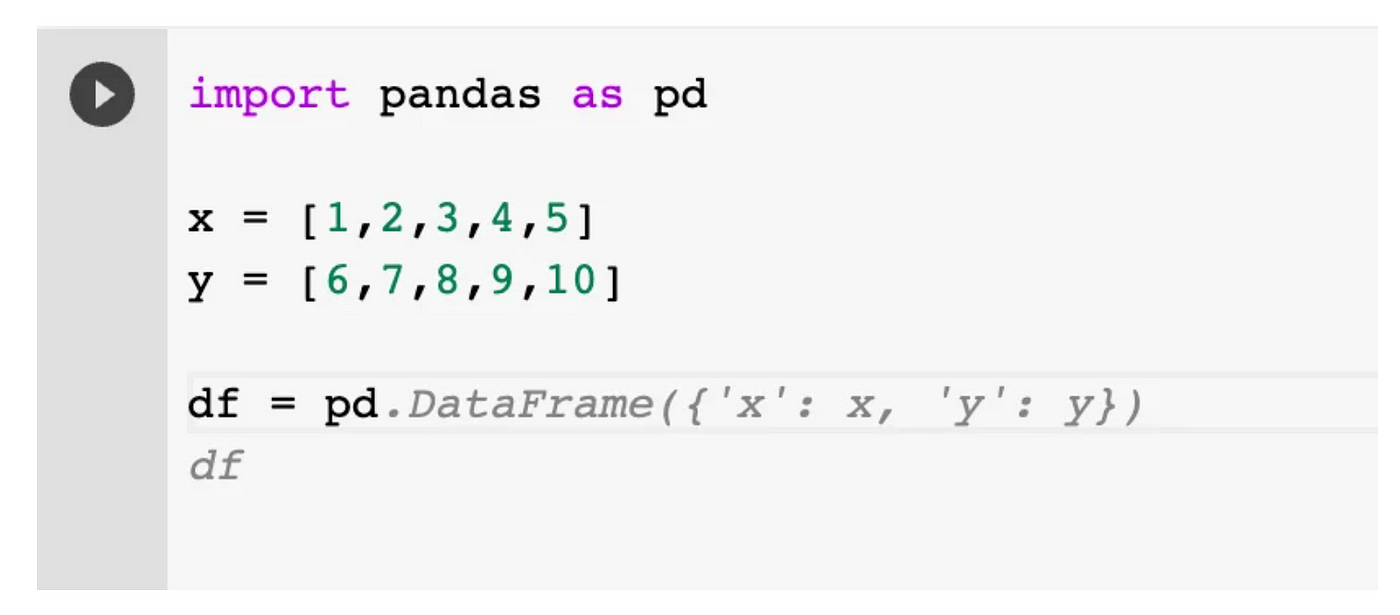
As you can see in the screenshot above, Google Colab automatically gives the user suggestions on what code comes next.
Another alternative is Github Copilot. With GitHub Copilot, you can get an AI-based suggestion in real-time. GitHub Copilot suggests code completions as developers type and turn prompts into coding suggestions based on the project’s context and style conventions. As per this release from Github, Copilot Chat is now powered by OpenAI GPT-4 (a similiar model that ChatGPT is using).
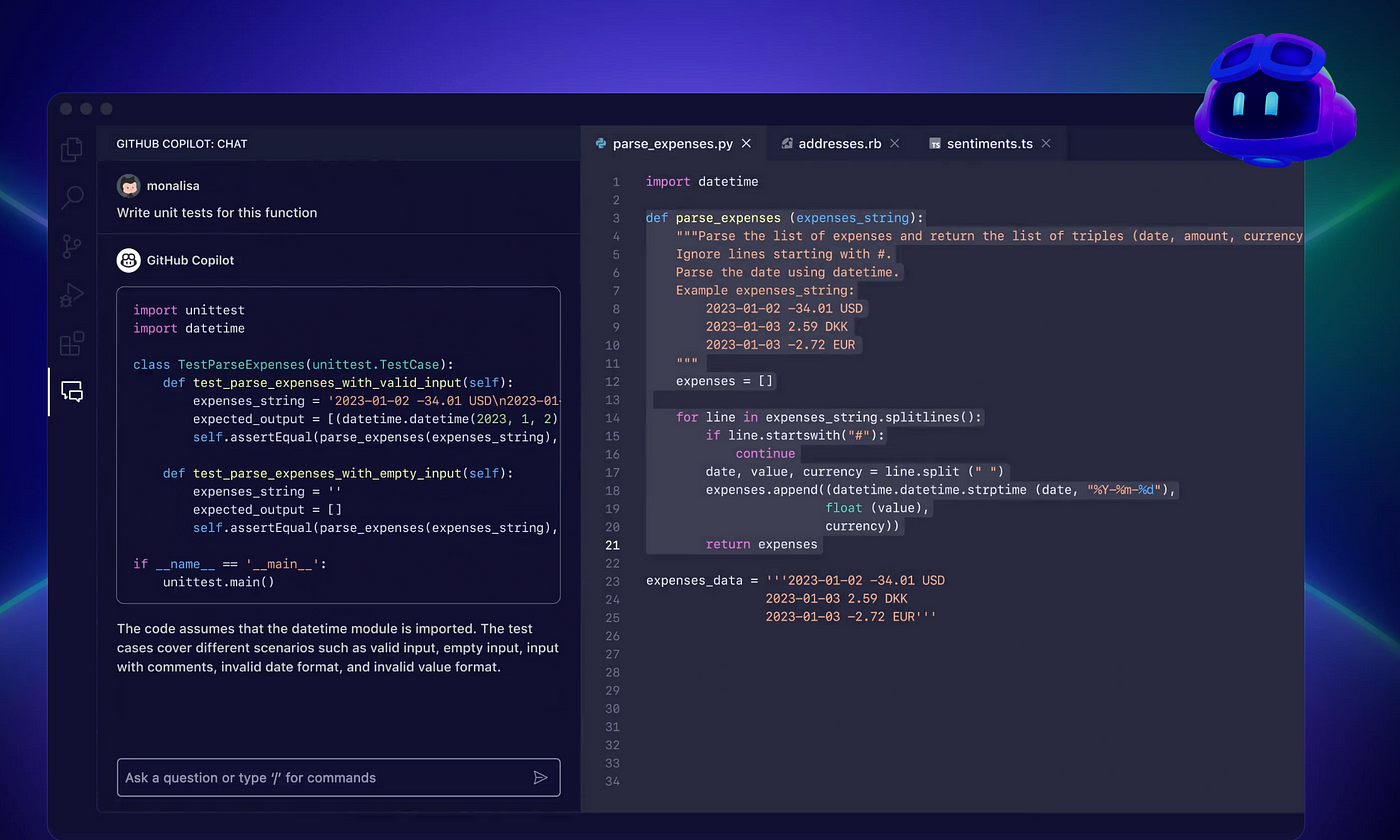
I have been actively using CodeGPT as a VSCode Extension before I knew that Github Copilot is accessible for free if you are in education program. CodeGPT Co has 1M download to this date on the VSCode Extension Marketplace. CodeGPT allows seamless integration with the ChatGPT API, Google PaLM 2, and Meta Llama.
You can get code suggestions through comments, here is how:
- Write a comment asking for a specific code
- Press
cmd + shift + i
- Use the code
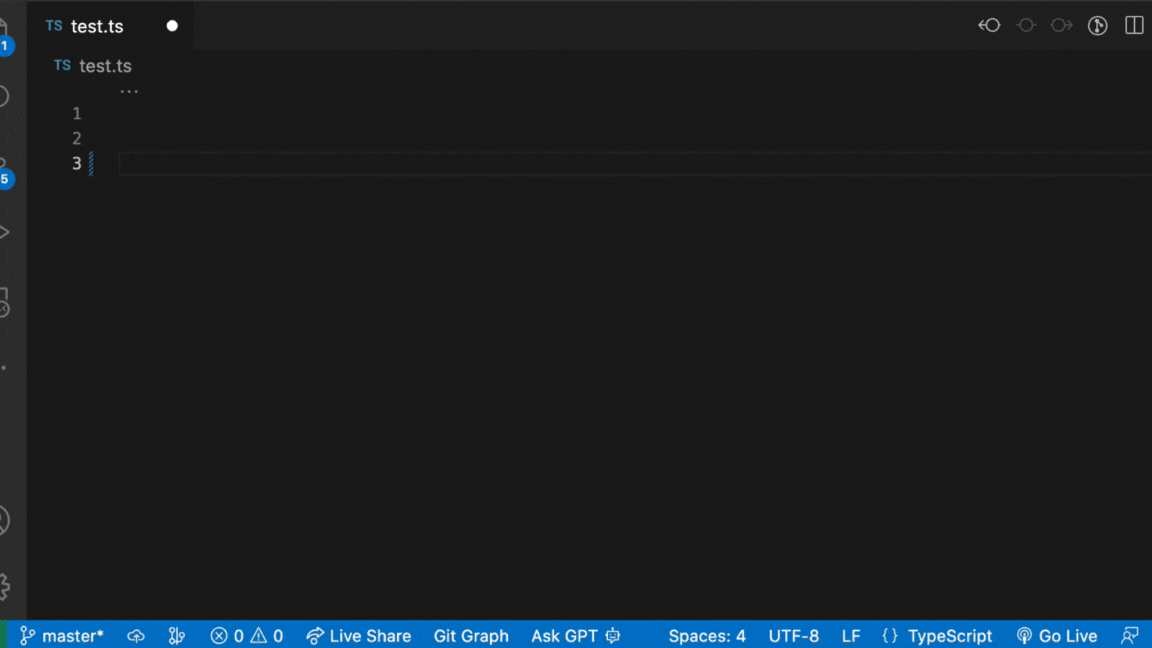
You can also initiate a chat via the extension in the menu and jump into coding conversations
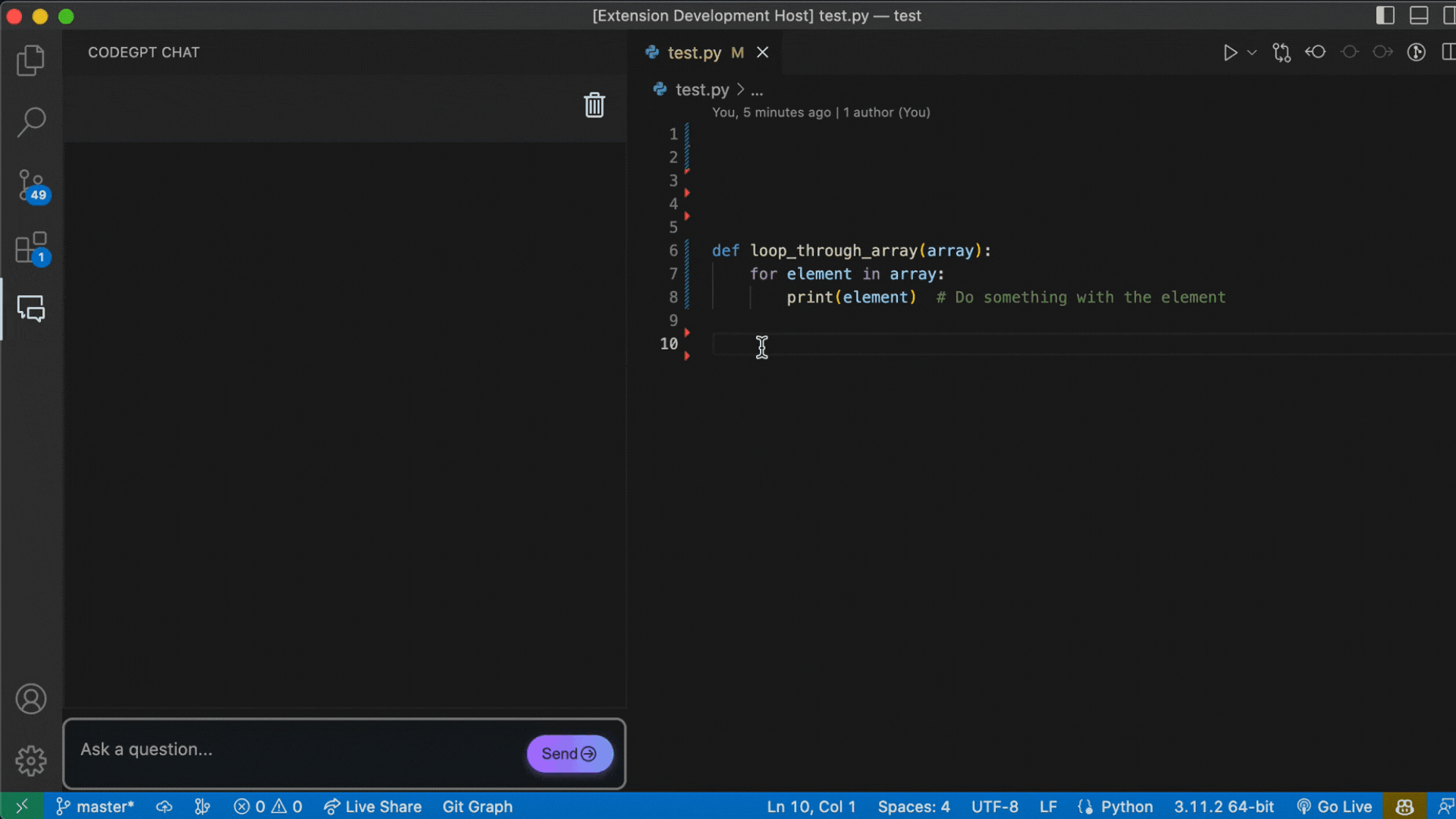
As I reflect on my coding journey, the invaluable lesson learned is that there’s no one-size-fits-all approach to learning. It’s essential to embrace a diverse array of learning methods, seamlessly blending traditional practices like browsing and community interaction with the innovative capabilities of tools like ChatGPT and auto code-completion tools.
What to Do:
- Utilize Tailored Learning Resources: Make the most of ChatGPT’s recommendations for learning materials.
- Collaborate for Problem-Solving: Utilize ChatGPT as a collaborative partner as if you are coding with your friends.
What Not to Do:
- Over-Dependence on ChatGPT: Avoid relying solely on ChatGPT and ensure a balanced approach to foster independent problem-solving skills.
- Neglect Real-Time Interaction with Coding Community: While ChatGPT offers valuable insights, don’t neglect the benefits of real-time interaction and feedback from coding communities. That also helps build a reputation in the community
- Disregard Practical Coding Practice: Balance ChatGPT guidance with hands-on coding practice to reinforce theoretical knowledge with practical application.
Let me know in the comments how you use ChatGPT to help you code!
Happy coding!
Ellen
Follow me on LinkedIn
Check out my portfolio: liviaellen.com/portfolio
My Previous AR Works: liviaellen.com/ar-profile
or just buy me a real coffee
— Yes I love coffee.
About the Author
I’m Ellen, a Machine Learning engineer with 6 years of experience, currently working at a fintech startup in San Francisco. My background spans data science roles in oil & gas consulting, as well as leading AI and data training programs across APAC, the Middle East, and Europe.
I’m currently completing my Master’s in Data Science (graduating May 2025) and actively looking for my next opportunity as a machine learning engineer. If you’re open to referring or connecting, I’d truly appreciate it!
I love creating real-world impact through AI and I’m always open to project-based collaborations as well.
Related posts
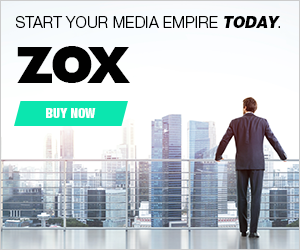
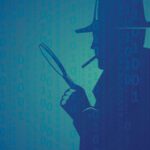
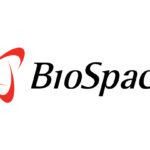
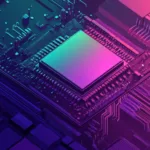
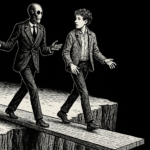
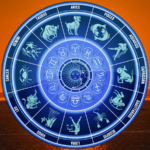
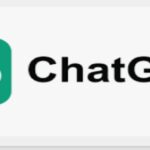
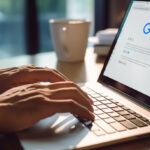
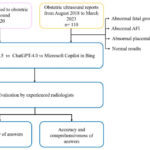
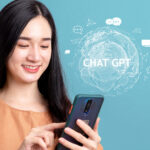
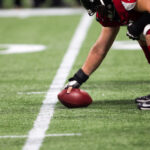
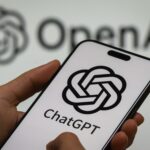
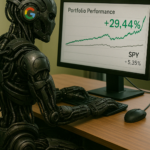
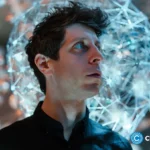
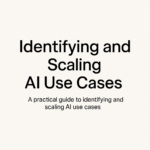
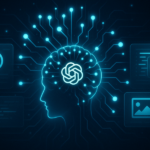
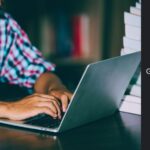
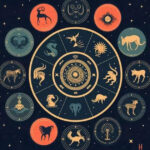
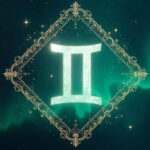
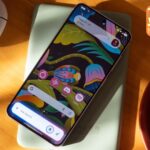
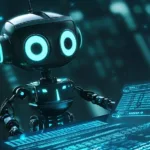
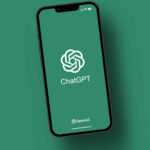
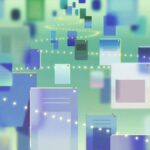
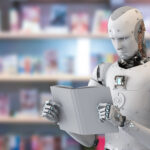
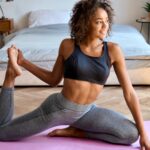
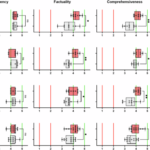
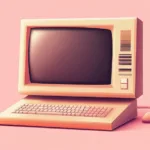
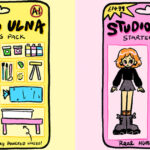
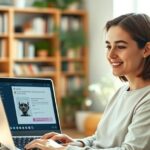
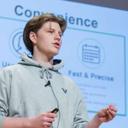
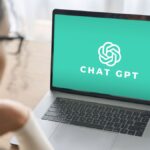
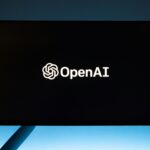
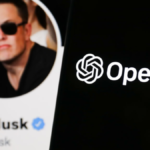
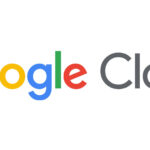
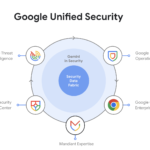
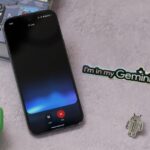
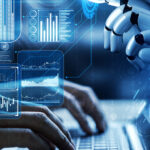
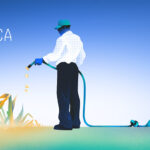
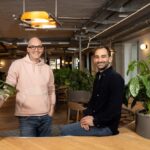
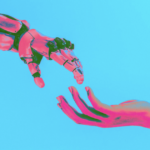
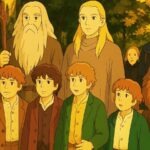
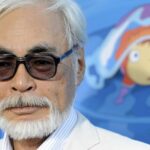
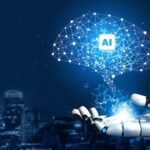
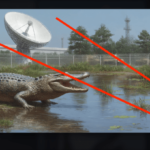
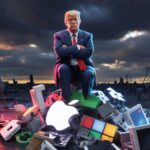
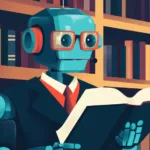
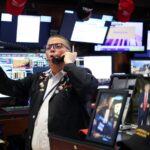
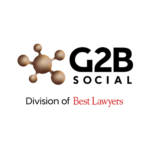
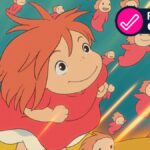
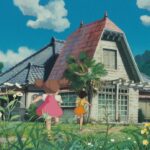
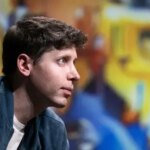
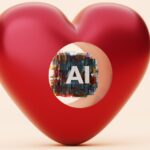
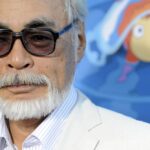
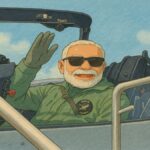
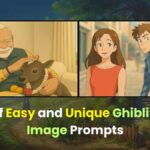
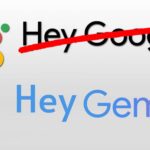
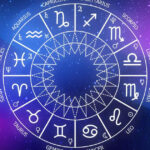
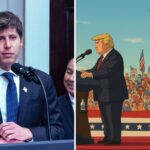
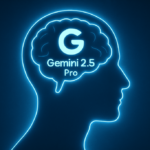
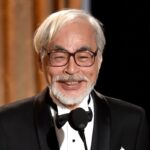
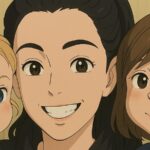
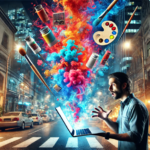
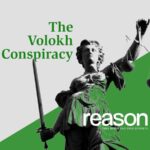
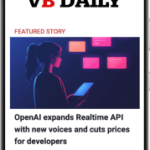
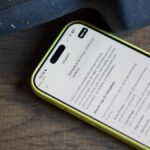
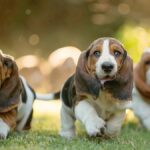
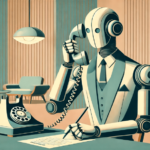
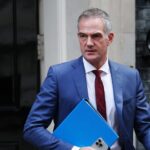
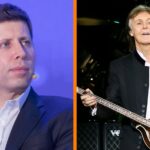
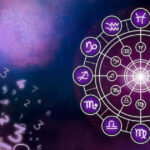
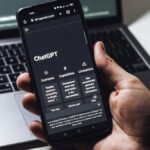
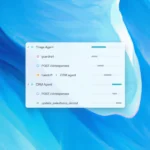
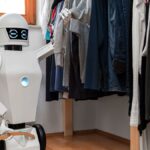
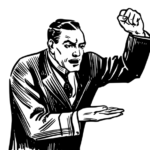
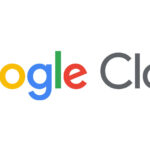
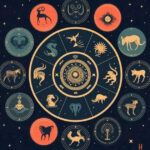
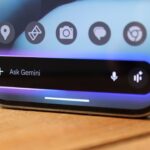
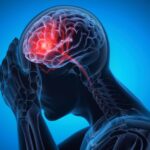
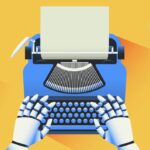
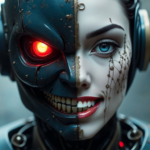
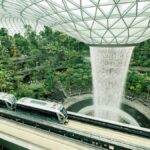
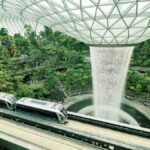
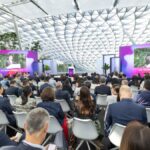
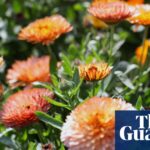

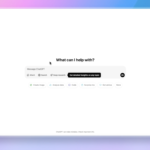
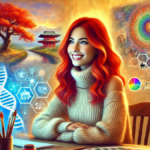
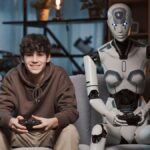
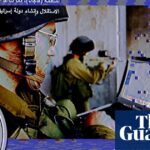
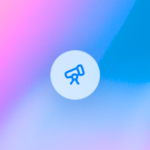
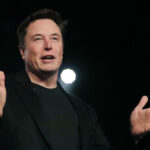
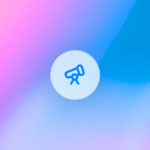
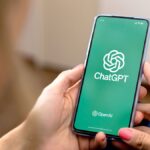
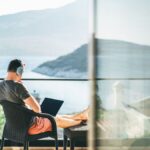
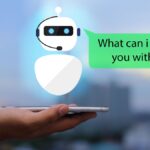
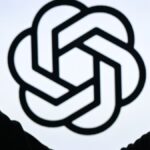
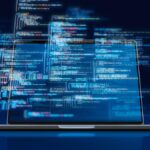
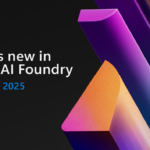
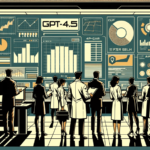
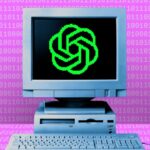
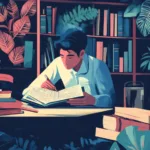
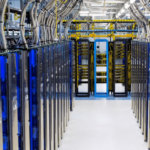
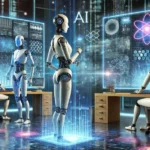
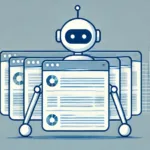
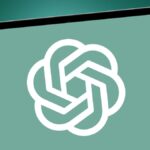
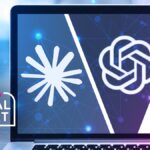
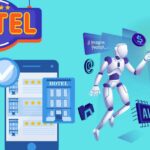
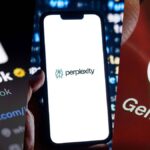
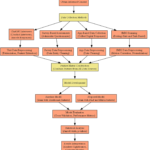
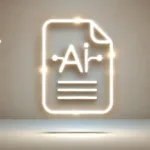
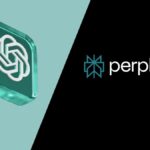
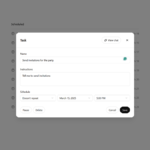
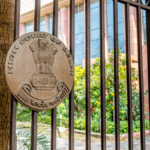
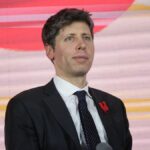
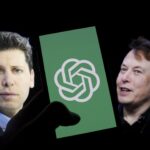
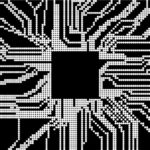
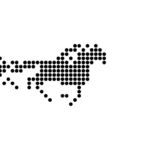
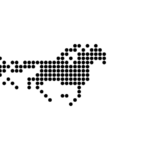
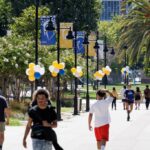
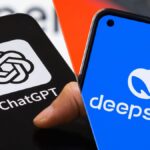
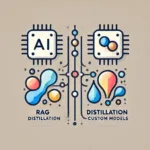
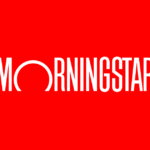
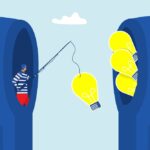
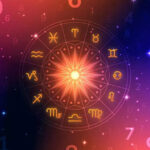
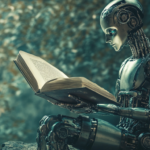
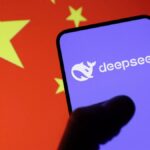
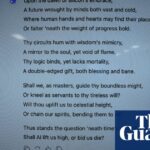
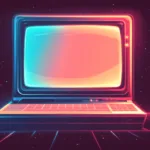

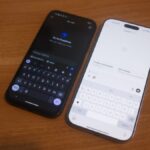
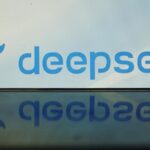

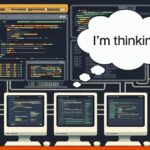
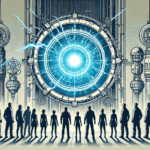
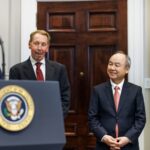
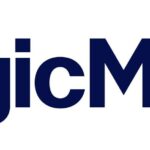
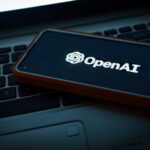

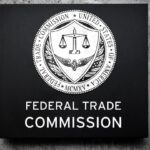
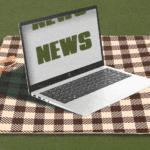
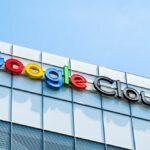
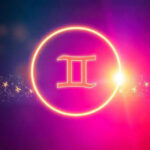
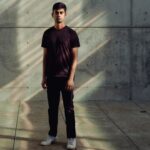
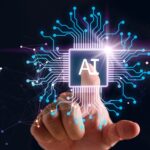
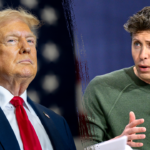
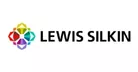
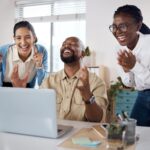
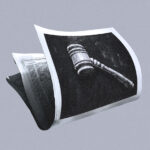
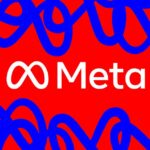

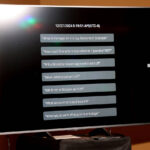

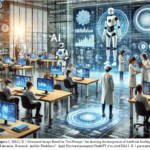
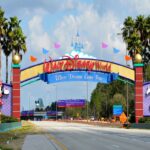
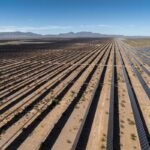
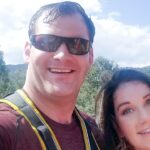
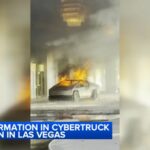
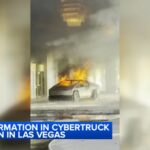
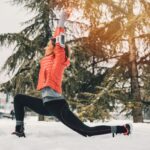
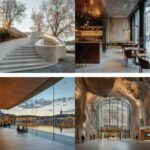
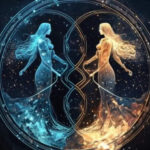
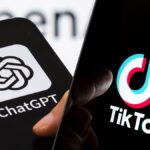
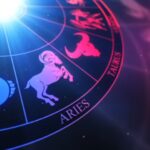
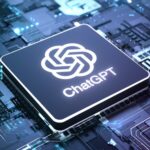
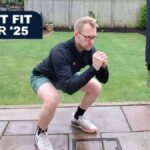
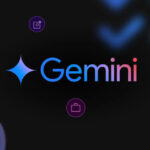
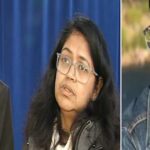
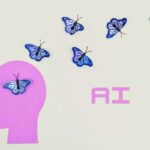
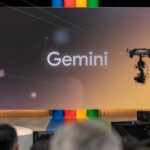
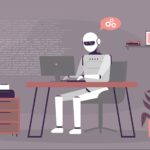
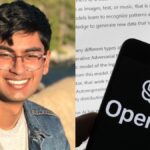
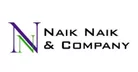

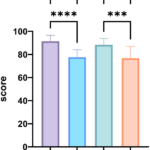
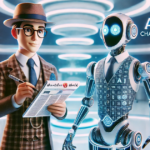
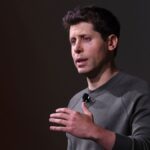
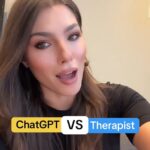
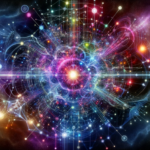
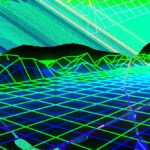
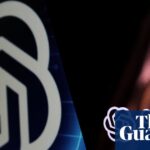
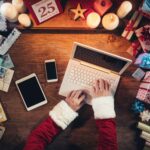
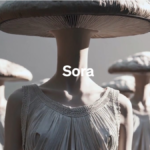
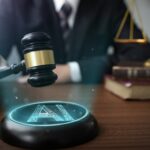
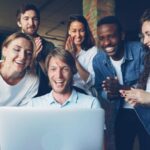
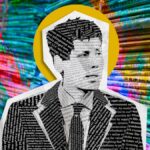

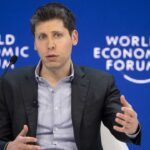
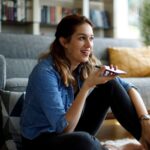
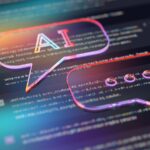
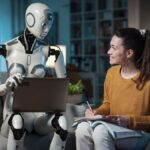
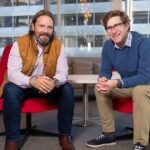
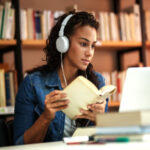
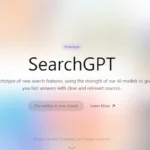
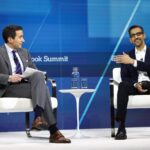
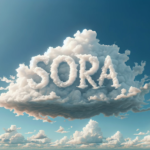
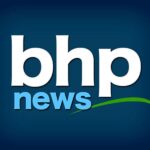
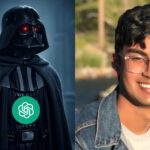
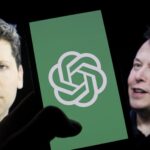
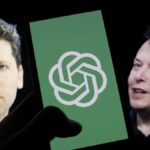
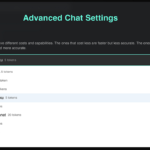
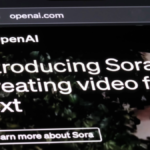
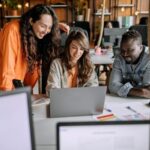
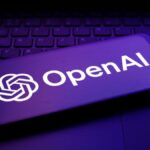
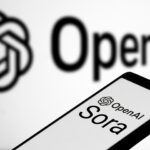
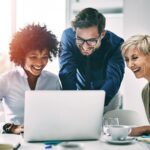
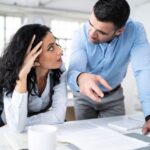
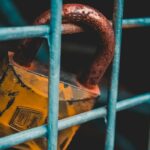
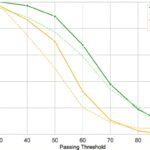
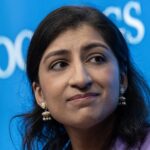
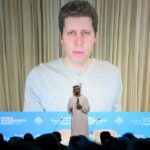
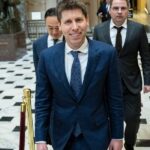
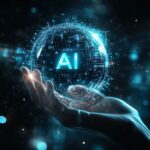
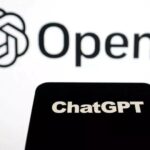
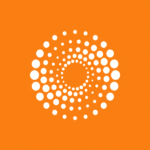
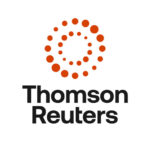
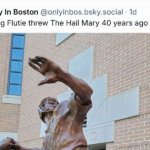

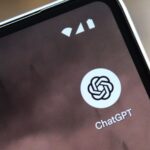
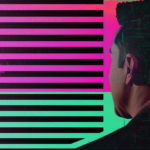
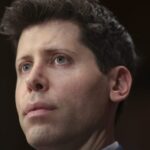
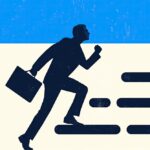
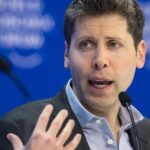
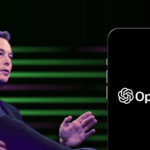
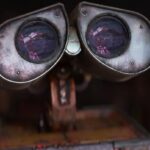
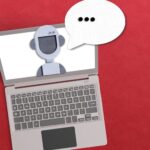
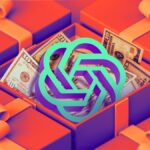
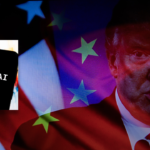
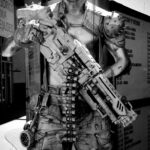
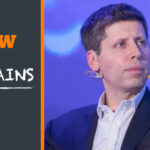
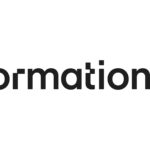
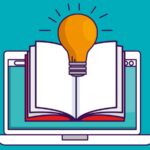
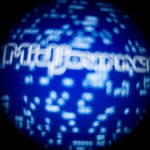
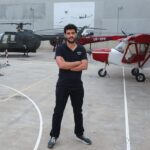
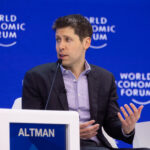
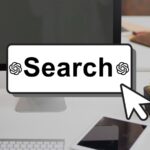
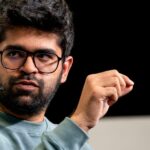
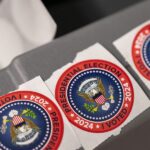
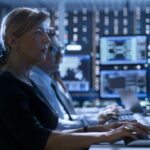
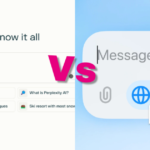
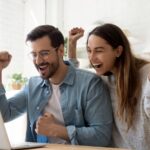
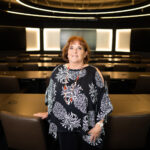
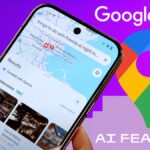
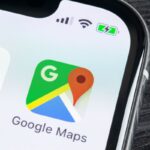
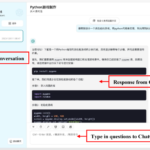
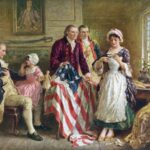
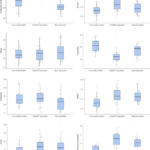

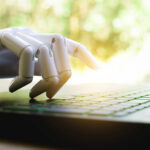
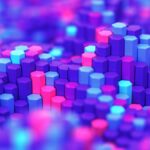
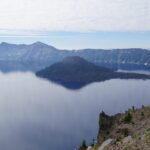
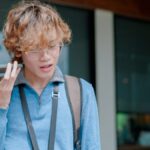
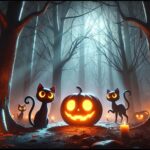
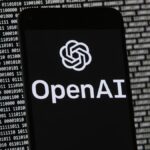
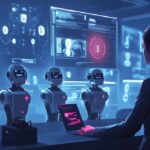
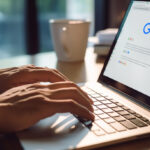
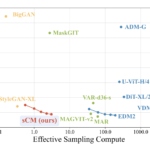
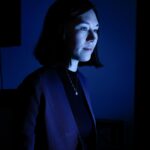
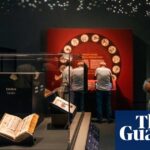
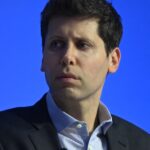
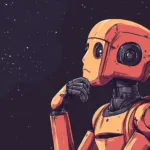
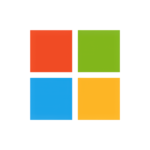
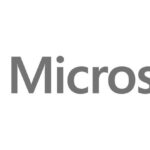
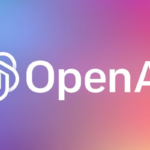
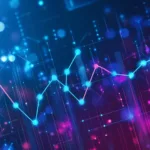
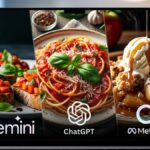
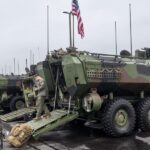

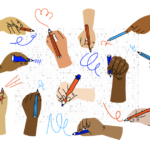
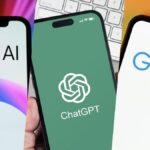
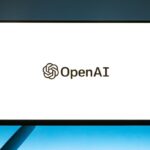
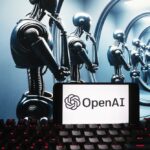
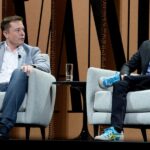
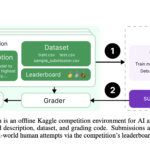
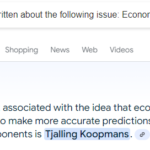
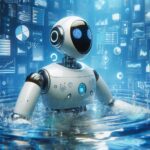
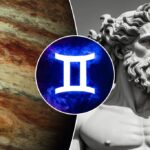
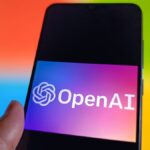
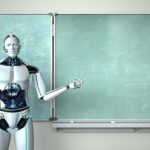
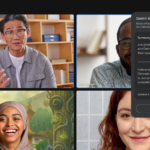
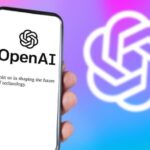
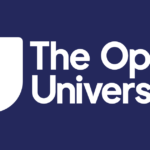
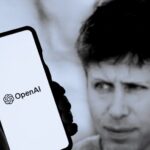
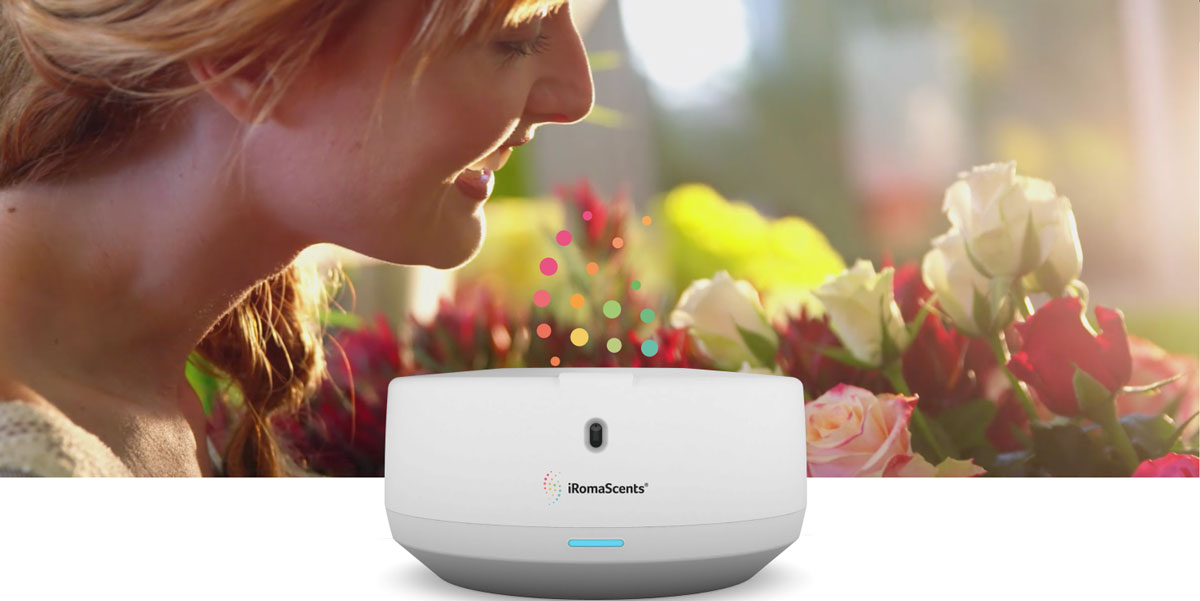

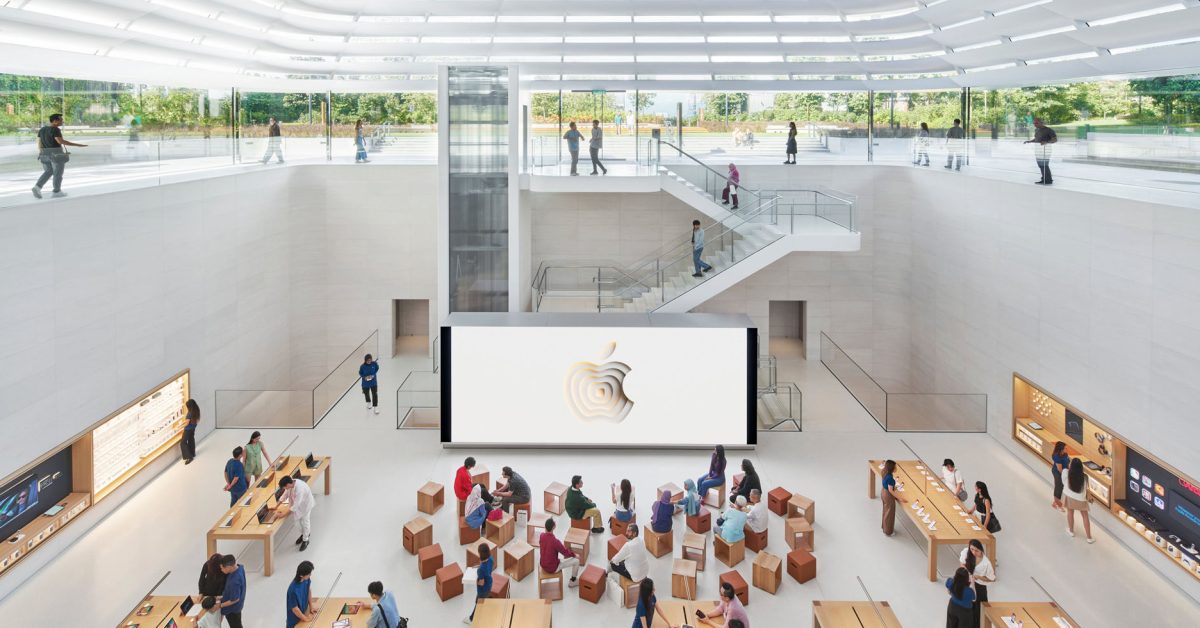


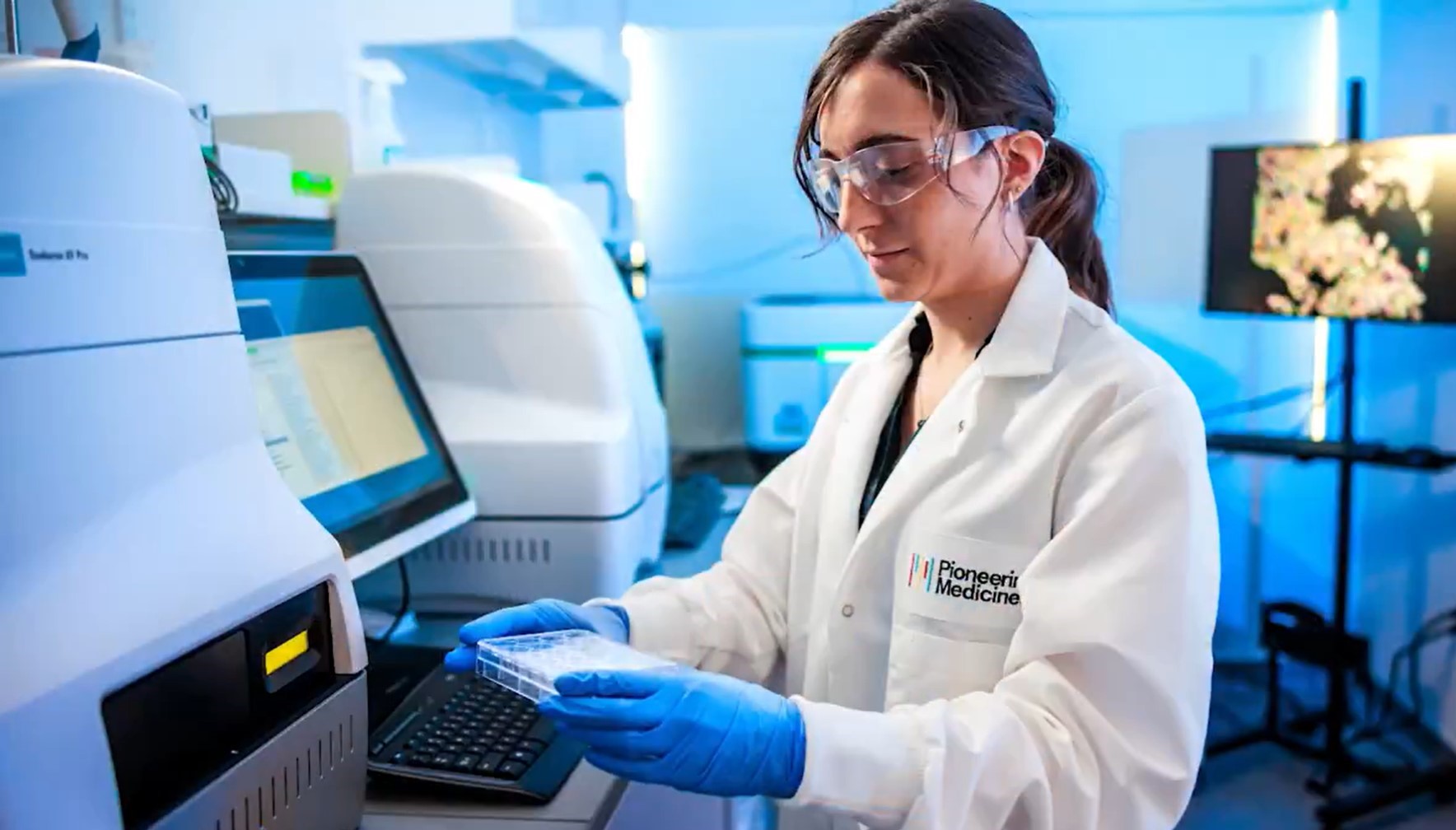
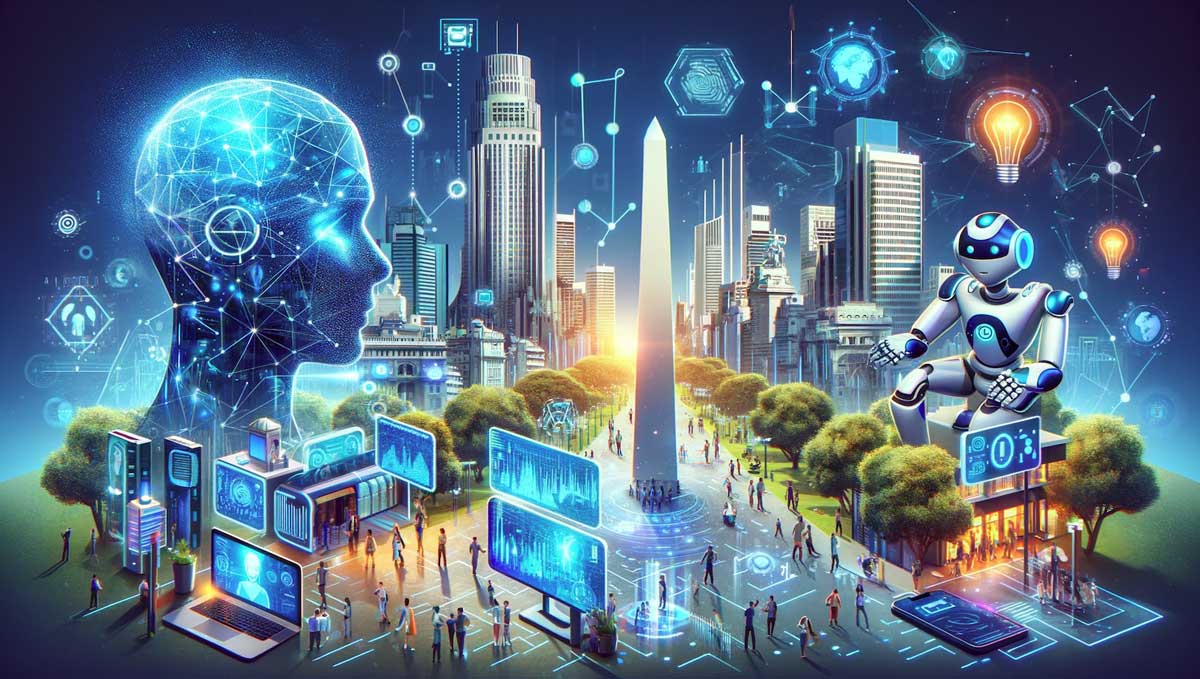

Trending
-
Startups11 meses ago
Remove.bg: La Revolución en la Edición de Imágenes que Debes Conocer
-
Tutoriales12 meses ago
Cómo Comenzar a Utilizar ChatGPT: Una Guía Completa para Principiantes
-
Recursos12 meses ago
Cómo Empezar con Popai.pro: Tu Espacio Personal de IA – Guía Completa, Instalación, Versiones y Precios
-
Startups10 meses ago
Startups de IA en EE.UU. que han recaudado más de $100M en 2024
-
Startups12 meses ago
Deepgram: Revolucionando el Reconocimiento de Voz con IA
-
Recursos11 meses ago
Perplexity aplicado al Marketing Digital y Estrategias SEO
-
Recursos12 meses ago
Suno.com: La Revolución en la Creación Musical con Inteligencia Artificial
-
Noticias10 meses ago
Dos periodistas octogenarios deman a ChatGPT por robar su trabajo