Noticias
ChatGPT Won’t Say My Name
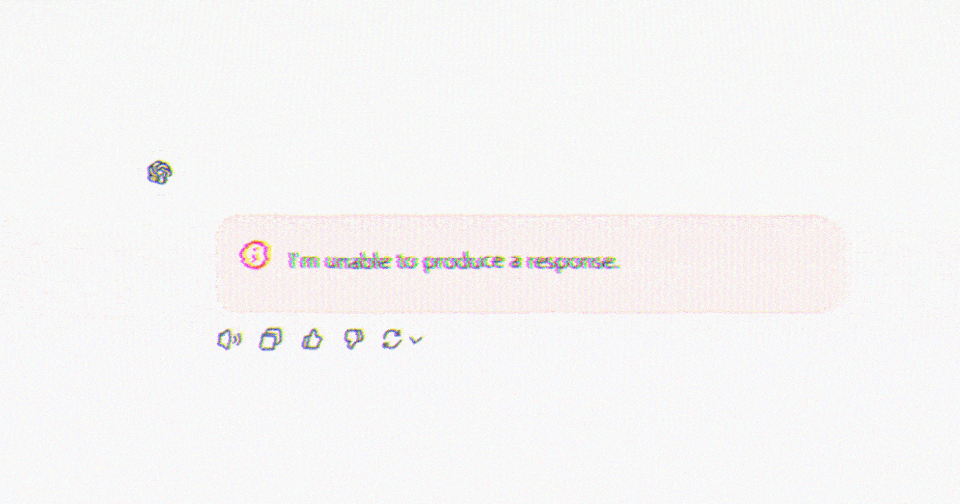
Jonathan Zittrain breaks ChatGPT: If you ask it a question for which my name is the answer, the chatbot goes from loquacious companion to something as cryptic as Microsoft Windows’ blue screen of death.
Anytime ChatGPT would normally utter my name in the course of conversation, it halts with a glaring “I’m unable to produce a response,” sometimes mid-sentence or even mid-word. When I asked who the founders of the Berkman Klein Center for Internet & Society are (I’m one of them), it brought up two colleagues but left me out. When pressed, it started up again, and then: zap.
The behavior seemed to be coarsely tacked on to the last step of ChatGPT’s output rather than innate to the model. After ChatGPT has figured out what it’s going to say, a separate filter appears to release a guillotine. The reason some observers have surmised that it’s separate is because GPT runs fine if it includes my middle initial or if it’s prompted to substitute a word such as banana for my name, and because there can even be inconsistent timing to it: Below, for example, GPT appears to first stop talking before it would naturally say my name; directly after, it manages to get a couple of syllables out before it stops. So it’s like having a referee who blows the whistle on a foul slightly before, during, or after a player has acted out.
For a long time, people have observed that beyond being “unable to produce a response,” GPT can at times proactively revise a response moments after it’s written whatever it’s said. The speculation here is that to delay every single response by GPT while it’s being double-checked for safety could unduly slow it down, when most questions and answers are totally anodyne. So instead of making everyone wait to go through TSA before heading to their gate, metal detectors might just be scattered around the airport, ready to pull someone back for a screening if they trigger something while passing the air-side food court.
The personal-name guillotine seemed a curiosity when my students first brought it to my attention at least a year ago. (They’d noticed it after a class session on how chatbots are trained and steered.) But now it’s kicked off a minor news cycle thanks to a viral social-media post discussing the phenomenon. (ChatGPT has the same issue with at least a handful of other names.) OpenAI is one of several supporters of a new public data initiative at the Harvard Law School Library, which I direct, and I’ve met a number of OpenAI engineers and policy makers at academic workshops. (The Atlantic this year entered into a corporate partnership with OpenAI.) So I reached out to them to ask about the odd name glitch. Here’s what they told me: There are a tiny number of names that ChatGPT treats this way, which explains why so few have been found. Names may be omitted from ChatGPT either because of privacy requests or to avoid persistent hallucinations by the AI.
The company wouldn’t talk about specific cases aside from my own, but online sleuths have speculated about what the forbidden names might have in common. For example, Guido Scorza is an Italian regulator who has publicized his requests to OpenAI to block ChatGPT from producing content using his personal information. His name does not appear in GPT responses. Neither does Jonathan Turley’s name; he is a George Washington University law professor who wrote last year that ChatGPT had falsely accused him of sexual harassment.
ChatGPT’s abrupt refusal to answer requests—the ungainly guillotine—was the result of a patch made in early 2023, shortly after the program launched and became unexpectedly popular. That patch lives on largely unmodified, the way chunks of ancient versions of Windows, including that blue screen of death, still occasionally poke out of today’s PCs. OpenAI told me that building something more refined is on its to-do list.
As for me, I never objected to anything about how GPT treats my name. Apparently, I was among a few professors whose names were spot-checked by the company around 2023, and whatever fabrications the spot-checker saw persuaded them to add me to the forbidden-names list. OpenAI separately told The New York Times that the name that had started it all—David Mayer—had been added mistakenly. And indeed, the guillotine no longer falls for that one.
For such an inelegant behavior to be in chatbots as widespread and popular as GPT is a blunt reminder of two larger, seemingly contrary phenomena. First, these models are profoundly unpredictable: Even slightly changed prompts or prior conversational history can produce wildly differing results, and it’s hard for anyone to predict just what the models will say in a given instance. So the only way to really excise a particular word is to apply a coarse filter like the one we see here. Second, model makers still can and do effectively shape in all sorts of ways how their chatbots behave.
To a first approximation, large language models produce a Forrest Gump–ian box of chocolates: You never know what you’re going to get. To form their answers, these LLMs rely on pretraining that metaphorically entails putting trillions of word fragments from existing texts, such as books and websites, into a large blender and coarsely mixing them. Eventually, this process maps how words relate to other words. When done right, the resulting models will merrily generate lots of coherent text or programming code when prompted.
The way that LLMs make sense of the world is similar to the way their forebears—online search engines—peruse the web in order to return relevant results when prompted with a few search terms. First they scrape as much of the web as possible; then they analyze how sites link to one another, along with other factors, to get a sense of what’s relevant and what’s not. Neither search engines nor AI models promise truth or accuracy. Instead, they simply offer a window into some nanoscopic subset of what they encountered during their training or scraping. In the case of AIs, there is usually not even an identifiable chunk of text that’s being parroted—just a smoothie distilled from an unthinkably large number of ingredients.
For Google Search, this means that, historically, Google wasn’t asked to take responsibility for the truth or accuracy of whatever might come up as the top hit. In 2004, when a search on the word Jew produced an anti-Semitic site as the first result, Google declined to change anything. “We find this result offensive, but the objectivity of our ranking function prevents us from making any changes,” a spokesperson said at the time. The Anti-Defamation League backed up the decision: “The ranking of … hate sites is in no way due to a conscious choice by Google, but solely is a result of this automated system of ranking.” Sometimes the chocolate box just offers up an awful liquor-filled one.
The box-of-chocolates approach has come under much more pressure since then, as misleading or offensive results have come to be seen more and more as dangerous rather than merely quirky or momentarily regrettable. I’ve called this a shift from a “rights” perspective (in which people would rather avoid censoring technology unless it behaves in an obviously illegal way) to a “public health” one, where people’s casual reliance on modern tech to shape their worldview appears to have deepened, making “bad” results more powerful.
Indeed, over time, web intermediaries have shifted from being impersonal academic-style research engines to being AI constant companions and “copilots” ready to interact in conversational language. The author and web-comic creator Randall Munroe has called the latter kind of shift a move from “tool” to “friend.” If we’re in thrall to an indefatigable, benevolent-sounding robot friend, we’re at risk of being steered the wrong way if the friend (or its maker, or anyone who can pressure that maker) has an ulterior agenda. All of these shifts, in turn, have led some observers and regulators to prioritize harm avoidance over unfettered expression.
That’s why it makes sense that Google Search and other search engines have become much more active in curating what they say, not through search-result links but ex cathedra, such as through “knowledge panels” that present written summaries alongside links on common topics. Those automatically generated panels, which have been around for more than a decade, were the online precursors to the AI chatbots we see today. Modern AI-model makers, when pushed about bad outputs, still lean on the idea that their job is simply to produce coherent text, and that users should double-check anything the bots say—much the way that search engines don’t vouch for the truth behind their search results, even if they have an obvious incentive to get things right where there is consensus about what is right. So although AI companies disclaim accuracy generally, they, as with search engines’ knowledge panels, have also worked to keep chatbot behavior within certain bounds, and not just to prevent the production of something illegal.
One way model makers influence the chocolates in the box is through “fine-tuning” their models. They tune their chatbots to behave in a chatty and helpful way, for instance, and then try to make them unhelpful in certain situations—for instance, not creating violent content when asked by a user. Model makers do this by drawing in experts in cybersecurity, bio-risk, and misinformation while the technology is still in the lab and having them get the models to generate answers that the experts would declare unsafe. The experts then affirm alternative answers that are safer, in the hopes that the deployed model will give those new and better answers to a range of similar queries that previously would have produced potentially dangerous ones.
In addition to being fine-tuned, AI models are given some quiet instructions—a “system prompt” distinct from the user’s prompt—as they’re deployed and before you interact with them. The system prompt tries to keep the models on a reasonable path, as defined by the model maker or downstream integrator. OpenAI’s technology is used in Microsoft Bing, for example, in which case Microsoft may provide those instructions. These prompts are usually not shared with the public, though they can be unreliably extracted by enterprising users: This might be the one used by X’s Grok, and last year, a researcher appeared to have gotten Bing to cough up its system prompt. A car-dealership sales assistant or any other custom GPT may have separate or additional ones.
These days, models might have conversations with themselves or with another model when they’re running, in order to self-prompt to double-check facts or otherwise make a plan for a more thorough answer than they’d give without such extra contemplation. That internal chain of thought is typically not shown to the user—perhaps in part to allow the model to think socially awkward or forbidden thoughts on the way to arriving at a more sound answer.
So the hocus-pocus of GPT halting on my name is a rare but conspicuous leaf on a much larger tree of model control. And although some (but apparently not all) of that steering is generally acknowledged in succinct model cards, the many individual instances of intervention by model makers, including extensive fine-tuning, are not disclosed, just as the system prompts typically aren’t. They should be, because these can represent social and moral judgments rather than simple technical ones. (There are ways to implement safeguards alongside disclosure to stop adversaries from wrongly exploiting them.) For example, the Berkman Klein Center’s Lumen database has long served as a unique near-real-time repository of changes made to Google Search because of legal demands for copyright and some other issues (but not yet for privacy, given the complications there).
When people ask a chatbot what happened in Tiananmen Square in 1989, there’s no telling if the answer they get is unrefined the way the old Google Search used to be or if it’s been altered either because of its maker’s own desire to correct inaccuracies or because the chatbot’s maker came under pressure from the Chinese government to ensure that only the official account of events is broached. (At the moment, ChatGPT, Grok, and Anthropic’s Claude offer straightforward accounts of the massacre, at least to me—answers could in theory vary by person or region.)
As these models enter and affect daily life in ways both overt and subtle, it’s not desirable for those who build models to also be the models’ quiet arbiters of truth, whether on their own initiative or under duress from those who wish to influence what the models say. If there end up being only two or three foundation models offering singular narratives, with every user’s AI-bot interaction passing through those models or a white-label franchise of same, we need a much more public-facing process around how what they say will be intentionally shaped, and an independent record of the choices being made. Perhaps we’ll see lots of models in mainstream use, including open-source ones in many variants—in which case bad answers will be harder to correct in one place, while any given bad answer will be seen as less oracular and thus less harmful.
Right now, as model makers have vied for mass public use and acceptance, we’re seeing a necessarily seat-of-the-pants build-out of fascinating new tech. There’s rapid deployment and use without legitimating frameworks for how the exquisitely reasonable-sounding, oracularly treated declarations of our AI companions should be limited. Those frameworks aren’t easy, and to be legitimating, they can’t be unilaterally adopted by the companies. It’s hard work we all have to contribute to. In the meantime, the solution isn’t to simply let them blather, sometimes unpredictably, sometimes quietly guided, with fine print noting that results may not be true. People will rely on what their AI friends say, disclaimers notwithstanding, as the television commentator Ana Navarro-Cárdenas did when sharing a list of relatives pardoned by U.S. presidents across history, blithely including Woodrow Wilson’s brother-in-law “Hunter deButts,” whom ChatGPT had made up out of whole cloth.
I figure that’s a name more suited to the stop-the-presses guillotine than mine.
Noticias
Esto es lo que debes saber
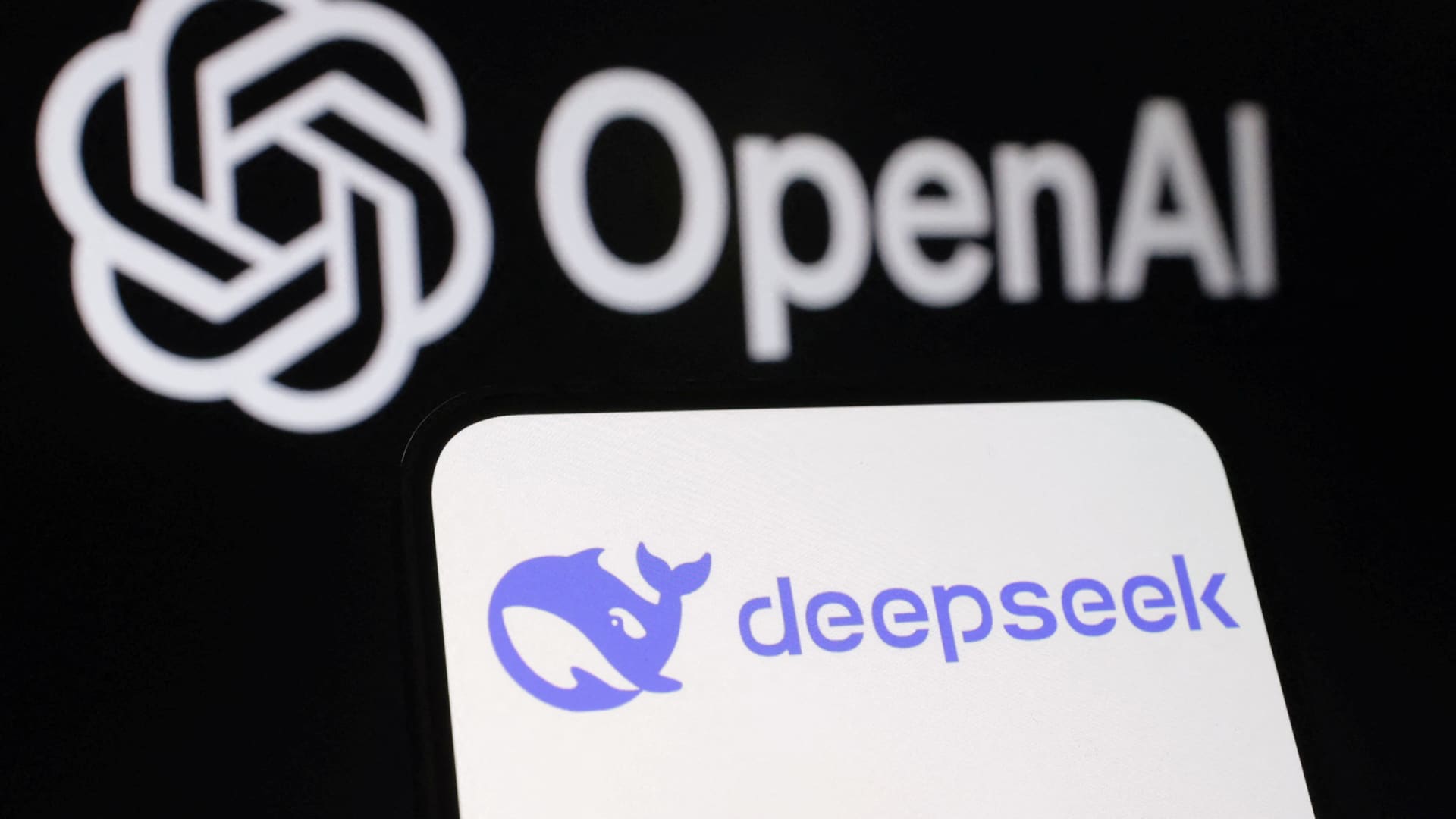
El lunes, la startup china de inteligencia artificial DeepSeek tomó el codiciado lugar de su rival OpenAI como la aplicación gratuita más descargada en los EE. UU. Manzana‘s App Store, destronando a ChatGPT para el asistente de inteligencia artificial de DeepSeek. Las acciones tecnológicas mundiales se vendieron y estaban en camino de eliminar miles de millones en capitalización de mercado.
Líderes tecnológicos, analistas, inversores y desarrolladores dicen que la exageración (y el consiguiente temor de quedarse atrás en el siempre cambiante ciclo exagerado de la IA) puede estar justificada. Especialmente en la era de la carrera armamentista generativa de la IA, donde tanto los gigantes tecnológicos como las nuevas empresas compiten para asegurarse de no quedarse atrás en un mercado que se prevé superará el billón de dólares en ingresos dentro de una década.
¿Qué es DeepSeek?
DeepSeek fue fundada en 2023 por Liang Wenfeng, cofundador de High-Flyer, un fondo de cobertura cuantitativo centrado en la IA. Según se informa, la startup de IA surgió de la unidad de investigación de IA del fondo de cobertura en abril de 2023 para centrarse en grandes modelos de lenguaje y alcanzar la inteligencia artificial general, o AGI, una rama de la IA que iguala o supera al intelecto humano en una amplia gama de tareas, que OpenAI y sus rivales dicen que lo están persiguiendo rápidamente. DeepSeek sigue siendo propiedad total de High-Flyer y financiado por ella, según analistas de Jefferies.
Los rumores en torno a DeepSeek comenzaron a cobrar fuerza a principios de este mes, cuando la startup lanzó R1, su modelo de razonamiento que rivaliza con el o1 de OpenAI. Es de código abierto, lo que significa que cualquier desarrollador de IA puede usarlo, y se ha disparado a la cima de las tiendas de aplicaciones y tablas de clasificación de la industria, y los usuarios elogian su rendimiento y capacidades de razonamiento.
Al igual que otros chatbots chinos, tiene sus limitaciones cuando se le pregunta sobre ciertos temas: cuando se le pregunta sobre algunas de las políticas del líder chino Xi Jinping, por ejemplo, DeepSeek supuestamente aleja al usuario de líneas de preguntas similares.
Otra parte clave de la discusión: el R1 de DeepSeek se construyó a pesar de que Estados Unidos limitó las exportaciones de chips a China tres veces en tres años. Las estimaciones difieren sobre cuánto cuesta exactamente el R1 de DeepSeek o cuántas GPU se incluyen en él. Los analistas de Jefferies estimaron que una versión reciente tenía un “coste de capacitación de sólo 5,6 millones de dólares (suponiendo un costo de alquiler de 2 dólares por hora y 800 horas). Eso es menos del 10% del costo de Meta‘s Llama.” Pero independientemente de las cifras específicas, los informes coinciden en que el modelo fue desarrollado a una fracción del costo de los modelos rivales por OpenAI, Anthropic, Google y otros.
Como resultado, el sector de la IA está inundado de preguntas, entre ellas si el creciente número de rondas de financiación astronómicas y valoraciones de miles de millones de dólares de la industria es necesaria, y si una burbuja está a punto de estallar.
Acciones de NVIDIA cayó un 11%, con el fabricante de chips ASML bajó más del 6%. El Nasdaq cayó más del 2% y cuatro gigantes tecnológicos… Meta, microsoft, Manzana y ASML están listos para informar sus ganancias esta semana.
Los analistas de Raymond James detallaron algunas de las preguntas que afectan a la industria de la IA este mes y escribieron: “¿Cuáles son las implicaciones para la inversión? ¿Qué dice sobre los modelos de código abierto versus los propietarios? ¿Invertir dinero en GPU es realmente una panacea? ¿Existen restricciones a las exportaciones de Estados Unidos? ¿Cuáles son las implicaciones más amplias de [DeepSeek]? Bueno, podrían ser espantosos o no ser un evento, pero tengan la seguridad de que la industria está llena de incredulidad y especulación”.
Los analistas de Bernstein escribieron en una nota el lunes que “según las muchas (ocasionalmente histéricas) tomas calientes que vimos [over the weekend,] las implicaciones van desde ‘Eso es realmente interesante’ hasta ‘Esta es la sentencia de muerte del complejo de infraestructura de IA tal como lo conocemos'”.
Cómo están respondiendo las empresas estadounidenses
Algunos directores ejecutivos de tecnología estadounidenses están luchando por responder antes de que los clientes cambien a ofertas potencialmente más baratas de DeepSeek, y se informa que Meta está iniciando cuatro “salas de guerra” relacionadas con DeepSeek dentro de su departamento de IA generativa.
microsoft El director ejecutivo Satya Nadella escribió en X que el fenómeno DeepSeek era solo un ejemplo de la paradoja de Jevons: “A medida que la IA se vuelva más eficiente y accesible, veremos cómo su uso se dispara, convirtiéndola en un bien del que simplemente no podemos tener suficiente”. “. El director ejecutivo de OpenAI, Sam Altman, tuiteó una cita que atribuyó a Napoleón y escribió: “Una revolución no se puede hacer ni detener. Lo único que se puede hacer es que uno de sus hijos le dé una dirección a fuerza de victorias”.
Yann LeCun, científico jefe de IA de Meta, escribió en LinkedIn que el éxito de DeepSeek es indicativo del cambio de rumbo en el sector de la IA para favorecer la tecnología de código abierto.
LeCun escribió que DeepSeek se ha beneficiado de parte de la tecnología propia de Meta, es decir, sus modelos Llama, y que la startup “ideó nuevas ideas y las construyó sobre el trabajo de otras personas. Debido a que su trabajo está publicado y es de código abierto, todos pueden sacar provecho de ello. Ese es el poder de la investigación abierta y del código abierto”.
Alexandr Wang, director ejecutivo de Scale AI, dijo a CNBC la semana pasada que el último modelo de IA de DeepSeek fue “revolucionario” y que su versión R1 es aún más poderosa.
“Lo que hemos descubierto es que DeepSeek… tiene el mejor rendimiento, o aproximadamente está a la par de los mejores modelos estadounidenses”, dijo Wang, añadiendo que la carrera de IA entre EE.UU. y China es una “guerra de IA”. La empresa de Wang proporciona datos de entrenamiento a actores clave de la IA, incluidos OpenAI, Google y Meta.
A principios de esta semana, el presidente Donald Trump anunció una empresa conjunta con OpenAI, Oracle y SoftBank para invertir miles de millones de dólares en infraestructura de IA en Estados Unidos. El proyecto, Stargate, fue presentado en la Casa Blanca por Trump, el director ejecutivo de SoftBank, Masayoshi Son, el cofundador de Oracle, Larry Ellison, y el director ejecutivo de OpenAI, Sam Altman. Los socios tecnológicos iniciales clave incluirán a Microsoft, Nvidia y Oracle, así como a la empresa de semiconductores Arm. Dijeron que invertirían 100.000 millones de dólares para empezar y hasta 500.000 millones de dólares en los próximos cuatro años.
IA evolucionando
La noticia de la destreza de DeepSeek también llega en medio del creciente revuelo en torno a los agentes de IA (modelos que van más allá de los chatbots para completar tareas complejas de varios pasos para un usuario) que tanto los gigantes tecnológicos como las nuevas empresas están persiguiendo. Meta, Google, Amazon, Microsoft, OpenAI y Anthropic han expresado su objetivo de crear IA agente.
Anthropic, la startup de IA respaldada por Amazon y fundada por ex ejecutivos de investigación de OpenAI, intensificó su desarrollo tecnológico durante el año pasado y, en octubre, la startup dijo que sus agentes de IA podían usar computadoras como humanos para completar tareas complejas. La capacidad de uso de computadoras de Anthropic permite que su tecnología interprete lo que hay en la pantalla de una computadora, seleccione botones, ingrese texto, navegue por sitios web y ejecute tareas a través de cualquier software y navegación por Internet en tiempo real, dijo la startup.
La herramienta puede “usar computadoras básicamente de la misma manera que nosotros”, dijo a CNBC Jared Kaplan, director científico de Anthropic, en una entrevista en ese momento. Dijo que puede realizar tareas con “decenas o incluso cientos de pasos”.
OpenAI lanzó una herramienta similar la semana pasada, introduciendo una función llamada Operador que automatizará tareas como planificar vacaciones, completar formularios, hacer reservas en restaurantes y pedir alimentos.
El microsoft-La startup respaldada lo describe como “un agente que puede ir a la web para realizar tareas por usted” y agregó que está capacitado para interactuar con “los botones, menús y campos de texto que la gente usa a diario” en la web. También puede hacer preguntas de seguimiento para personalizar aún más las tareas que realiza, como información de inicio de sesión para otros sitios web. Los usuarios pueden tomar el control de la pantalla en cualquier momento.
Noticias
Esto es lo que debes saber: NBC 6 South Florida
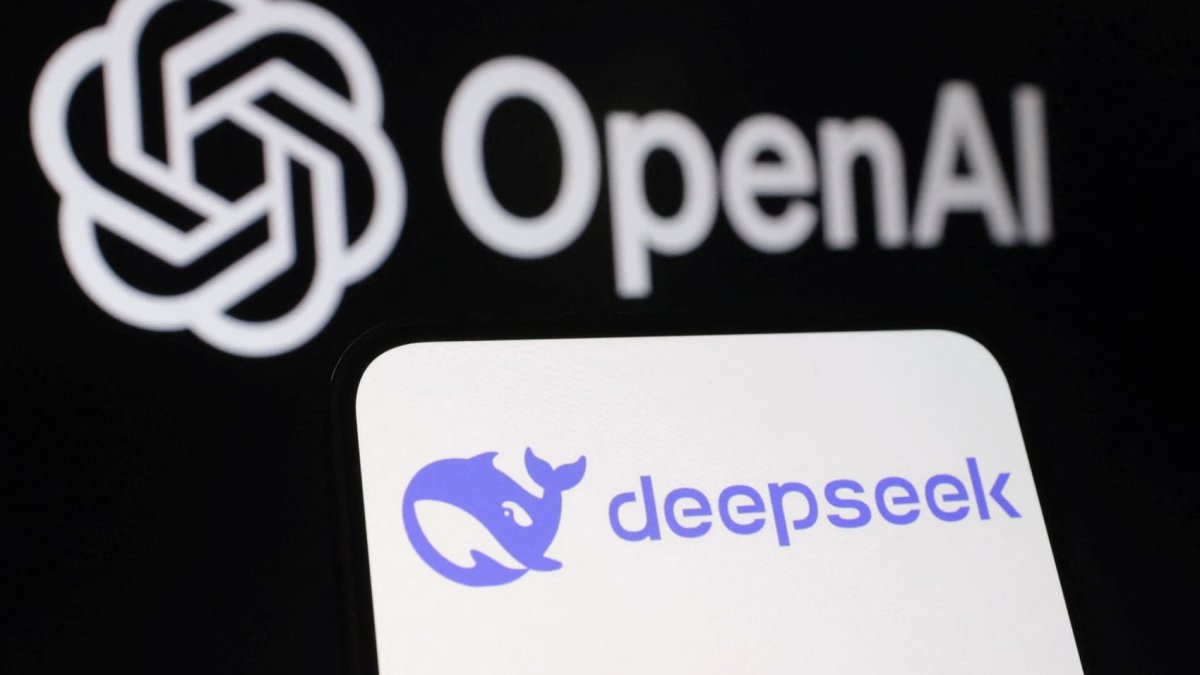
- El zumbido alrededor de la startup de IA China Deepseek comenzó a recoger a Steam a principios de este mes, cuando la startup lanzó R1, su modelo de razonamiento que rivaliza con el O1 de Openai.
- El lunes, Deepseek se hizo cargo del lugar codiciado de su rival Openai para la aplicación gratuita más descargada en los EE. UU. En la App Store de Apple, destronando a Chatgpt para el asistente de IA de Deepseek.
- Global Tech Stocks se vendió, con el gigante de chip de IA Nvidia cayendo un 10%.
El lunes, la startup de inteligencia artificial china Deepseek se hizo cargo del lugar codiciado de su rival Openai como la aplicación gratuita más desactivada en los EE. UU. En la tienda de aplicaciones de Apple, destronando Chatgpt para el asistente de IA de Deepseek. Las acciones de Global Tech se vendieron y estaban en camino de acabar con miles de millones en el límite de mercado.
Los líderes tecnológicos, analistas, inversores y desarrolladores dicen que el bombo, y el consiguiente temor de quedarse atrás en el ciclo de bombo de IA en constante cambio, pueden estar justificados. Especialmente en la era de la carrera armamentista generativa de IA, donde los gigantes tecnológicos y las startups están corriendo para garantizar que no se queden atrás en un mercado previsto para superar los ingresos de $ 1 billón en una década.
¿Qué es Deepseek?
Deepseek fue fundada en 2023 por Liang Wenfeng, cofundador de High-Flyer, un fondo cuantitativo de cobertura centrado en la IA. Según los informes, la startup de IA surgió de la Unidad de Investigación de AI del fondo de cobertura en abril de 2023 para centrarse en modelos de idiomas grandes y alcanzar la inteligencia general artificial, o AGI, una rama de IA que iguala o supere el intelecto humano en una amplia gama de tareas, que se abren. Y sus rivales dicen que están persiguiendo rápidamente. Deepseek sigue siendo propiedad y financiado por High-Flyer, según analistas de Jefferies.
El zumbido alrededor de Deepseek comenzó a recoger a Steam a principios de este mes, cuando la startup lanzó R1, su modelo de razonamiento que rivaliza con el O1 de OpenAI. Es de código abierto, lo que significa que cualquier desarrollador de IA puede usarlo, y se ha disparado a la cima de las tiendas de aplicaciones y las tablas de clasificación de la industria, con los usuarios elogios de su rendimiento y capacidades de razonamiento.
Al igual que otros chatbots chinos, tiene sus limitaciones cuando se les pregunta sobre ciertos temas: cuando se le pregunta sobre algunas de las políticas del líder chino Xi Jinping, por ejemplo, Deepseek aleja al usuario de líneas similares de preguntas.
Otra parte clave de la discusión: R1 de Deepseek se construyó a pesar de las exportaciones de chips de EE. UU. A China tres veces en tres años. Las estimaciones difieren exactamente en la cantidad de R1 de Deepseek, o en cuántas GPU entró. Los analistas de Jefferies estimaron que una versión reciente tenía un “costo de capacitación de solo US $ 5,6 millones (suponiendo un costo de alquiler de US $ 2/h800 horas). Eso es menos del 10% del costo de la LLAMA de Meta”. Pero independientemente de los números específicos, los informes acuerdan que el modelo se desarrolló a una fracción del costo de los modelos rivales por OpenAI, Anthrope, Google y otros.
Como resultado, el sector de la IA está inundado de preguntas, incluido si es necesario el creciente número de rondas de financiación astronómica y las valoraciones de mil millones de dólares, y si una burbuja está a punto de estallar.
Las acciones de NVIDIA cayeron un 11%, con el fabricante de chips ASML más del 6%. El NASDAQ cayó por 2%, y cuatro gigantes tecnológicos: Meta, Microsoft, Apple y ASML están listos para informar las ganancias esta semana.
Los analistas de Raymond James detallaron algunas de las preguntas que afectan a la industria de la IA este mes, escribiendo: “¿Cuáles son las implicaciones de inversión? ¿Qué dice sobre los modelos de origen abierto versus patentado? ¿Está arrojando dinero a las GPU realmente una panacea? trabajando? ¿Cuáles son las implicaciones más amplias de [DeepSeek]? Bueno, podrían ser terribles o un no evento, pero tengan la seguridad de que la industria está llena de incredulidad y especulación “.
Los analistas de Bernstein escribieron en una nota el lunes que “según las muchas tomas (ocasionalmente histéricas) que vimos que vimos [over the weekend,] El rango de implicaciones en cualquier lugar desde ‘eso es realmente interesante’ hasta ‘Esta es la luz de muerte del complejo de infraestructura de IA tal como lo conocemos’ “.
Cómo están respondiendo las empresas estadounidenses
Algunos CEO de tecnología estadounidense están trepando por responder antes de que los clientes cambien a ofertas potencialmente más baratas de Deepseek, y Según los informes, Meta inicia cuatro “salas de guerra” relacionadas con Deepseek dentro de su departamento generativo de IA.
El CEO de Microsoft, Satya Nadella, escribió en X que el fenómeno de Deepseek era solo un ejemplo de la paradoja de Jevons, escribiendo, “A medida que AI se vuelve más eficiente y accesible, veremos su uso Skyroocket, convirtiéndolo en una mercancía, simplemente no podemos obtener suficiente de.” El CEO de Operai, Sam Altman, tuiteó una cita que atribuyó a Napoleón, escribiendo: “Una revolución no se puede hacer ni detener.
Yann Lecun, el jefe científico de AI de Meta, escribió en LinkedIn que el éxito de Deepseek es indicativo de cambiar las mareas en el sector de IA para favorecer la tecnología de código abierto.
Lecun escribió que Deepseek se ha beneficiado de algunas de la propia tecnología de Meta, es decir, sus modelos de llama, y que la startup “se les ocurrió nuevas ideas y las construyó en la parte superior del trabajo de otras personas. Debido a que su trabajo es publicado y de código abierto, todos pueden Se beneficia de él.
Alexandr Wang, CEO de Scale AI, le dijo a CNBC la semana pasada que el último modelo de IA de Deepseek fue “devastador de la tierra” y que su lanzamiento de R1 es aún más poderoso.
“Lo que hemos encontrado es que Deepseek … es el mejor desempeño, o aproximadamente a la par con los mejores modelos estadounidenses”, dijo Wang, y agregó que la carrera de IA entre los Estados Unidos y China es una “guerra de IA”. La compañía de Wang proporciona datos de capacitación a jugadores clave de IA, incluidos OpenAI, Google y Meta.
A principios de esta semana, presidente Donald Trump anunció una empresa conjunta con OpenAI, Oracle y Softbank para invertir miles de millones de dólares en infraestructura de IA de EE. UU. El proyecto, Stargate, fue presentado en la Casa Blanca por Trump, el CEO de SoftBank, Masayoshi,, cofundador Larry Ellison, y el CEO de Operai, Sam Altman. Los socios de tecnología iniciales clave incluirán Microsoft, Nvidia y Oracle, así como el brazo de la compañía de semiconductores. Dijeron que invertirían $ 100 mil millones para comenzar y hasta $ 500 mil millones en los próximos cuatro años.
AI evolucionando
La noticia de la destreza de Deepseek también se produce en medio de la creciente exageración en torno a los agentes de IA, modelos que van más allá de los chatbots para completar tareas complejas de varios pasos para un usuario, que los gigantes tecnológicos y las startups están persiguiendo. Meta, Google, Amazon, Microsoft, Openai y Anthrope han expresado su objetivo de construir IA de agente.
Anthrope, la startup de IA respaldada por Amazon fundada por ex ejecutivos de investigación de Openai, aumentó su desarrollo de tecnología durante el año pasado, y en octubre, la startup dijo que sus agentes de IA pudieron usar computadoras como humanos para completar tareas complejas. La capacidad de uso de la computadora de Anthrope permite que su tecnología interprete lo que está en la pantalla de una computadora, seleccione botones, ingrese texto, navegue por los sitios web y ejecute tareas a través de cualquier software y navegación en Internet en tiempo real, dijo la startup.
La herramienta puede “usar computadoras básicamente de la misma manera que lo hacemos”, dijo Jared Kaplan, director científico de Anthrope, a CNBC en una entrevista en ese momento. Dijo que puede hacer tareas con “decenas o incluso cientos de pasos”.
Openai lanzó una herramienta similar la semana pasada, presentando una característica llamada operador que automatizará tareas como planificar vacaciones, completar formularios, hacer reservas de restaurantes y ordenar comestibles.
La startup respaldada por Microsoft lo describe como “un agente que puede ir a la web para realizar tareas para usted”, y agregó que está capacitado para interactuar con “los botones, los menús y los campos de texto que las personas usan a diario” en la web . También puede hacer preguntas de seguimiento para personalizar aún más las tareas que completa, como la información de inicio de sesión para otros sitios web. Los usuarios pueden tomar el control de la pantalla en cualquier momento.
Noticias
¿Por qué DeepSeek AI de repente es tan popular?
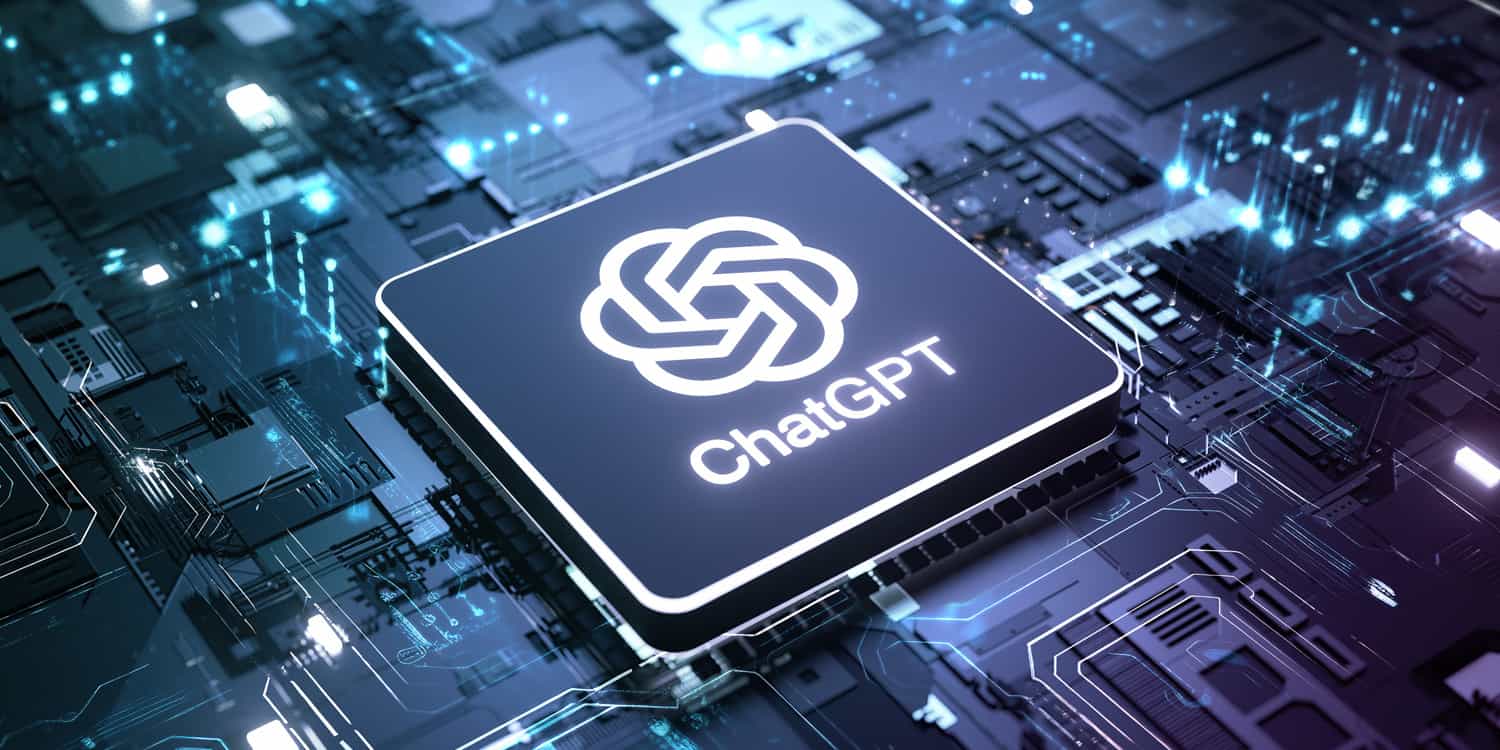
OpenAI lanzó su agente Operador AI para ChatGPT el jueves, lo que debería haber sido un hito importante para la empresa y el desarrollo de la IA en general. Si bien no pagaría $200 al mes para probar esta versión inicial de Operador, lo que vi en las demostraciones de OpenAI me dejó alucinado. El operador está muy por delante de los agentes de inteligencia artificial de Google, al menos en lo que respecta a demostraciones. No puedo esperar a tenerlo en mis manos una vez que OpenAI lo lleve a otros niveles pagos de ChatGPT y, lo que es más importante para mí personalmente, a la UE.
Sin embargo, la verdadera historia de la IA que se está apoderando del mundo no es ChatGPT, Operador o el enorme proyecto Stargate que se anunció la semana pasada. La historia de DeepSeek AI se apoderó del mundo cuando la startup china lanzó su modelo de razonamiento R1 que puede igualar el ChatGPT o1 de OpenAI.
No hay nada sorprendente en eso; Esperamos que otras empresas de IA igualen o1. Después de todo, OpenAI ya presentó o3, que debería anunciarse en unos días o semanas. Lo inusual de DeepSeek es que la empresa china hizo que sus modelos fueran de código abierto, por lo que cualquier empresa o desarrollador puede acceder a ellos e inspeccionarlos. Más interesante es el artículo de investigación sobre R1 que publicó DeepSeek, que afirma que el modelo altamente sofisticado fue entrenado a una fracción del costo del o1 de OpenAI.
La noticia de que el entrenamiento de DeepSeek R1 es posible con solo del 3% al 5% de los recursos que OpenAI necesita para un progreso similar con ChatGPT causó sensación en todo el mundo. Las acciones relacionadas con la IA se desplomaron durante las primeras operaciones del lunes, justo cuando DeepSeek saltó hasta convertirse en el número uno en la App Store, superando a ChatGPT.
Uno de los problemas del software de IA actual tiene que ver con el coste de desarrollo y uso del producto. Desarrollar modelos avanzados como o1 puede costar decenas de millones. El proceso requiere tarjetas gráficas (GPU) de alta gama que proporcionen la potencia informática y el gasto de energía necesarios.
Es por eso que los productos terminados como ChatGPT o1 no pueden estar disponibles de forma gratuita y sin limitaciones. Empresas como OpenAI necesitan cubrir costos y obtener ganancias. Es por eso que el enorme programa Stargate de 500 mil millones de dólares es una decisión tan monumental para el desarrollo de la IA, especialmente considerando la inevitable carrera armamentista de IA entre Estados Unidos y China.
Agregue las sanciones de EE. UU. que impiden que China acceda a los mismos chips y GPU de alta gama que hacen posible el desarrollo de productos ChatGPT o1, y uno pensaría que ChatGPT, Gemini, Meta AI y Claude no pueden obtener una competencia significativa de China.
Ahí es donde DeepSeek sorprendió al mundo. La startup china sabía que no podía competir contra OpenAI basándose en la potencia bruta. No podría tener acceso a la misma cantidad de GPU que acaparan empresas como OpenAI. Entonces, los investigadores de DeepSeek adoptaron otro enfoque para R1 y encontraron formas de entrenar un modelo de razonamiento avanzado sin acceso al mismo hardware.
No es sólo eso, sino que DeepSeek hizo que el acceso a R1 fuera mucho más barato que ChatGPT de OpenAI, lo cual es un avance significativo. Agregue la naturaleza de código abierto de los modelos DeepSeek y podrá ver por qué los desarrolladores acudirían en masa para probar la IA de la empresa china y por qué DeepSeek surgiría en la App Store.
Según la investigación, la startup china reemplazó la tecnología Supervised Fine-Tuning (SFT) que OpenAI utiliza para entrenar ChatGPT con Reinforcement Learning (RL) para producir resultados más rápidos y económicos. SFT se basa en mostrarle a la IA formas de resolver problemas brindando acceso a los datos para que la IA sepa qué tipo de respuestas brindar a varias indicaciones.
RL se basa en el modelo de IA, intenta descubrir las respuestas con un sistema de recompensa implementado y luego proporciona retroalimentación a la IA. RL permitió a DeepSeek mejorar las capacidades de razonamiento de R1 y superar la falta de computación. Sin embargo, como VentureBeat explica, se necesitaba algo de entrenamiento SFT, donde los humanos supervisan la IA, en las primeras fases de R1 antes de cambiar a RL.
Si bien señalé los inconvenientes obvios de depender de un rival de ChatGPT de China en este momento, no hay duda de que DeepSeek merece atención.
Como mínimo, las innovaciones que desarrollaron los investigadores de DeepSeek se pueden copiar en otros lugares para lograr avances similares. Después de todo, las primeras versiones de DeepSeek mostraron que la startup china podría haber copiado el trabajo de desarrollo de ChatGPT. Ya sea IA u otra cosa, las innovaciones tecnológicas siempre serán robadas y adaptadas.
Piénselo: a DeepSeek se le ocurrió una forma más eficiente de entrenar la IA utilizando solo unas 50.000 GPU, 10.000 de las cuales eran GPU NVIDIA compradas antes de las restricciones a las exportaciones de EE. UU. Comparativamente, empresas como OpenAI, Google y Anthropic operan con más de 500.000 GPU cada una, por VentureBeat.
Me imagino que los investigadores de estas empresas ahora están compitiendo para ver cómo y si pueden replicar el éxito de DeepSeek R1. También me imagino que encontrarán formas de hacerlo.
Con tanta computación y recursos a disposición de OpenAI, Google, Meta y Anthropic, pronto serán posibles avances similares a R1 además de lo que ya está disponible en los modelos de IA.
Además, si bien el mercado se vio afectado por las noticias sobre la IA de DeepSeek en China, no creo que el hardware, la potencia informática y la energía no importen en el futuro del desarrollo de la IA. Nuevamente, combine las innovaciones de DeepSeek con, digamos, un fondo de 500 mil millones de dólares y acceso a tarjetas gráficas de alta tecnología NVIDIA, y podría obtener las primeras fases de AGI.
Una vez que se empleen métodos similares a DeepSeek R1 para el desarrollo de ChatGPT y Gemini, los costos del acceso avanzado a la IA probablemente disminuirán para los usuarios premium. Esta sería una victoria clave para los consumidores.
Las empresas occidentales de IA no podrán mantener los costos altos y competir con DeepSeek R1 y sus sucesores. Algunos desarrolladores siempre elegirán los modelos más baratos a pesar del país de origen de la IA y el sesgo de entrenamiento. Como recordatorio, los modelos de DeepSeek mostrarán un sesgo hacia China. Este sigue siendo un software que debe cumplir con las leyes de censura locales.
Señalaré que China no se quedará de brazos cruzados. Estas son victorias tempranas. DeepSeek no está solo, ya que ByteDance también lanzó un chatbot de primer nivel. Se invertirán miles de millones de dólares en el desarrollo de la IA en el país para computación y energía. Recuerde, no todo lo que viene de China puede tomarse al pie de la letra. No está claro si los costos de entrenar DeepSeek son reales. La transparencia sólo funciona hasta cierto punto.
Afortunadamente, debido a que DeepSeek es de código abierto, otros pronto podrán ver si el entrenamiento similar al R1 se puede realizar con éxito en otros lugares.
VentureBeat hace un gran trabajo explicando las complejidades del desarrollo de DeepSeek R1 en este enlace. El documento técnico de DeepSeek que acompaña al lanzamiento de R1 el lunes se puede encontrar en GitHub.
-
Startups8 meses ago
Remove.bg: La Revolución en la Edición de Imágenes que Debes Conocer
-
Recursos9 meses ago
Cómo Empezar con Popai.pro: Tu Espacio Personal de IA – Guía Completa, Instalación, Versiones y Precios
-
Recursos9 meses ago
Suno.com: La Revolución en la Creación Musical con Inteligencia Artificial
-
Recursos8 meses ago
Perplexity aplicado al Marketing Digital y Estrategias SEO
-
Tutoriales9 meses ago
Cómo Comenzar a Utilizar ChatGPT: Una Guía Completa para Principiantes
-
Startups7 meses ago
Startups de IA en EE.UU. que han recaudado más de $100M en 2024
-
Estudiar IA8 meses ago
Curso de Inteligencia Artificial de UC Berkeley estratégico para negocios
-
Noticias7 meses ago
Dos periodistas octogenarios deman a ChatGPT por robar su trabajo