Noticias
A deep dive analysis of 62 queries
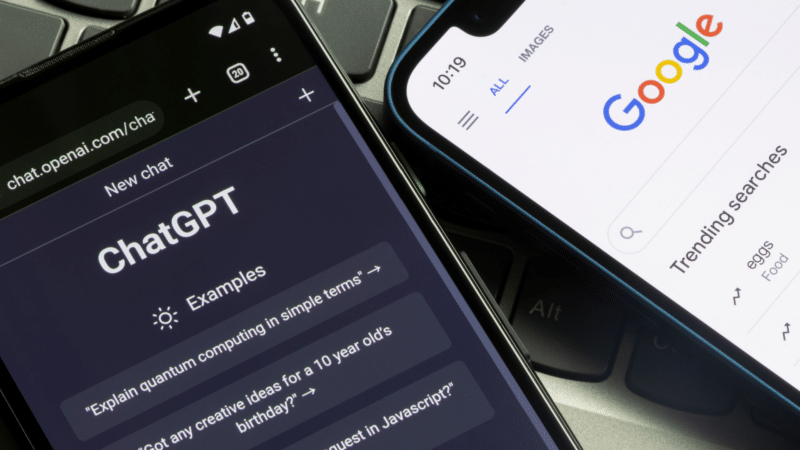
The emergence of ChatGPT search has led to many questions about the quality of the overall results compared to Google.
This is a difficult question to answer, and in today’s article, I will provide some insights into how to do just that.
Note that our understanding is that the technology that makes it possible for OpenAI to offer a search capability is called SearchGPT, but the actual product name is ChatGPT search.
In this article, we will use the name ChatGPT search.
What’s in this report
This report presents an analysis of 62 queries to assess the strengths and weaknesses of each platform.
Each response was meticulously fact-checked and evaluated for alignment with potential user intents.
The process, requiring about an hour per query, highlighted that “seemingly good” and “actually good” answers often differ.
Additionally, when Google provided an AI Overview, it was scored against ChatGPT search.
A combined score for the AI Overviews and the rest of Google’s SERP was also included.
Of the queries tested – two-thirds of which were informational – Google returned an AI Overview in 25 instances (40% of the time).
The queries analyzed fell into multiple categories:
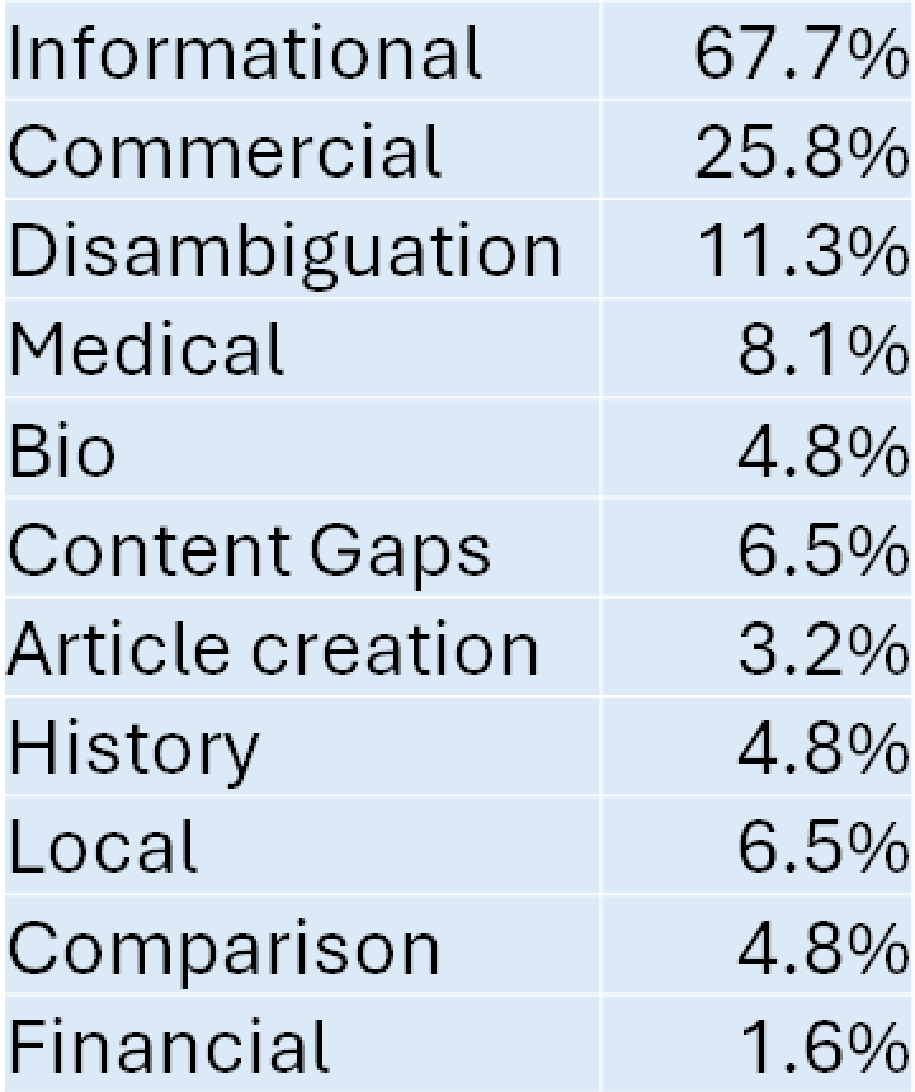
The total number of the above is greater than 100%, and that’s because some queries could fall into more than one classification.
For example, about 13% of the queries were considered informational and commercial.
Detailed information from SparkToro on the makeup of queries suggests a natural distribution of search queries as follows:
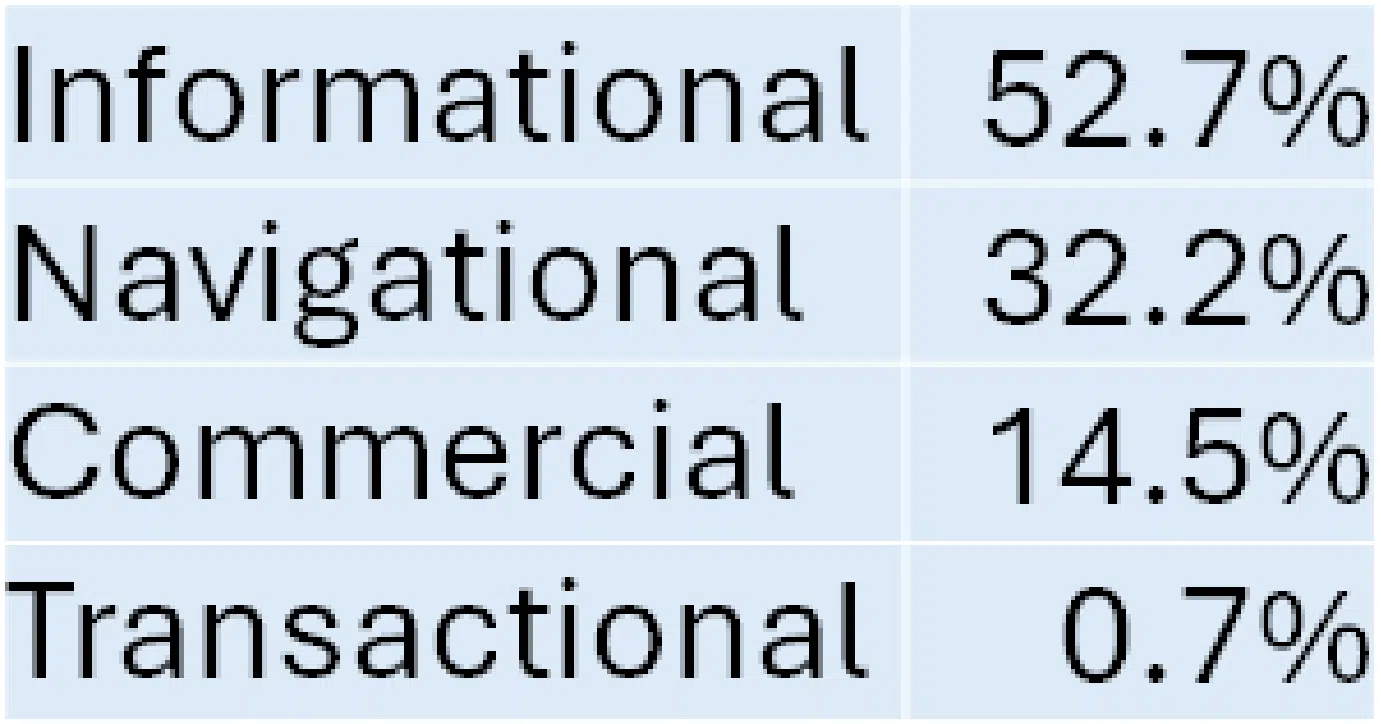
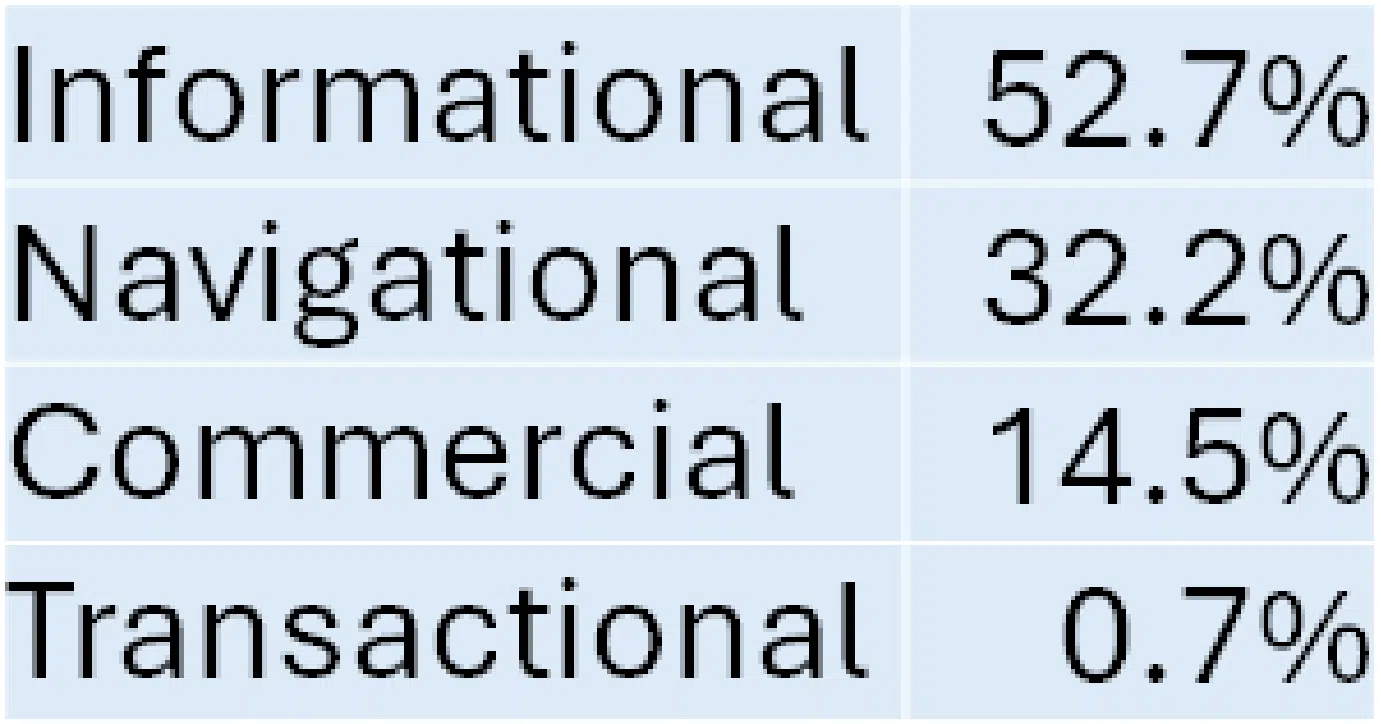
Navigational queries, which comprise nearly a third of all queries, were excluded from this test.
These queries typically demand a straightforward response like, “just give me the website,” and are a category where Google excels.
However, I included queries likely to favor one platform, such as:
- Content gap analysis queries (4): Representing a broader class of content-related queries, which Google doesn’t handle but ChatGPT search attempts (though not always successfully).
- Locally oriented queries (4): These leverage Google’s extensive local business database, Google Maps, and Waze, areas where ChatGPT search struggles to compete.
Metrics used in this study
I designed 62 queries to reflect diverse query intents, aiming to highlight each platform’s strengths and weaknesses.
Each response was scored across specific metrics to evaluate performance effectively.
- Errors: Did the response include incorrect information?
- Omissions: Was important information not in the response?
- Weaknesses: Were other aspects of the response considered weak but not scored as an error or omission?
- Fully addresses: Was the user’s query intent substantially addressed?
- Follow-up resources: Did the response provide suitable resources for follow-up research?
- Quality: An assessment by me of the overall quality of the response. This was done by weighing the other factors contained in this list.
At the end of this article are the total scores for each platform across the 62 queries.
Competitive observations
When considering how different search platforms provide value, it’s important to understand the many aspects of the search experience. Here are some of those areas:
Advertising
Multiple reviewers note that ChatGPT search is ad-free and tout how much better this makes it than Google. That is certainly the case now, but it won’t stay that way.
Microsoft has $13 billion committed to OpenAI so far, and they want to make that money back (and then some).
In short, don’t expect ChatGPT search to remain ad-free. That will change significantly at some point.
An important note is that advertising works best on commercial queries.
As you will see later in this article, I scored Google’s performance on commercial queries significantly higher than ChatGPT search.
Understanding user intent
Google has been working on understanding user intent across nearly infinite scenarios since 2004 or earlier.
They’ve been collecting data based on all the user interactions within search and leveraging what they have seen with the Chrome browser since its launch in 2008.
This data has most likely been used to help train Google algorithms to understand user intent and brand authority on a per query basis.
For reference, as of November 2024, Statcounter pegs Chrome’s market share at 67.5%, Safari at 18.2%, and Edge at 4.8%
This is a critical advantage for Google because understanding the user intent of a query is what it’s all about.
You can’t possibly answer the user’s need without understanding their need. As I’ll illustrate in the next section, this is complex!
How query sessions work
Part of the problem with understanding user intent is that the user may not have fully worked out what they’re looking for until they start the process.
Consider the following example of a query sequence that was given to me via Microsoft many years ago:
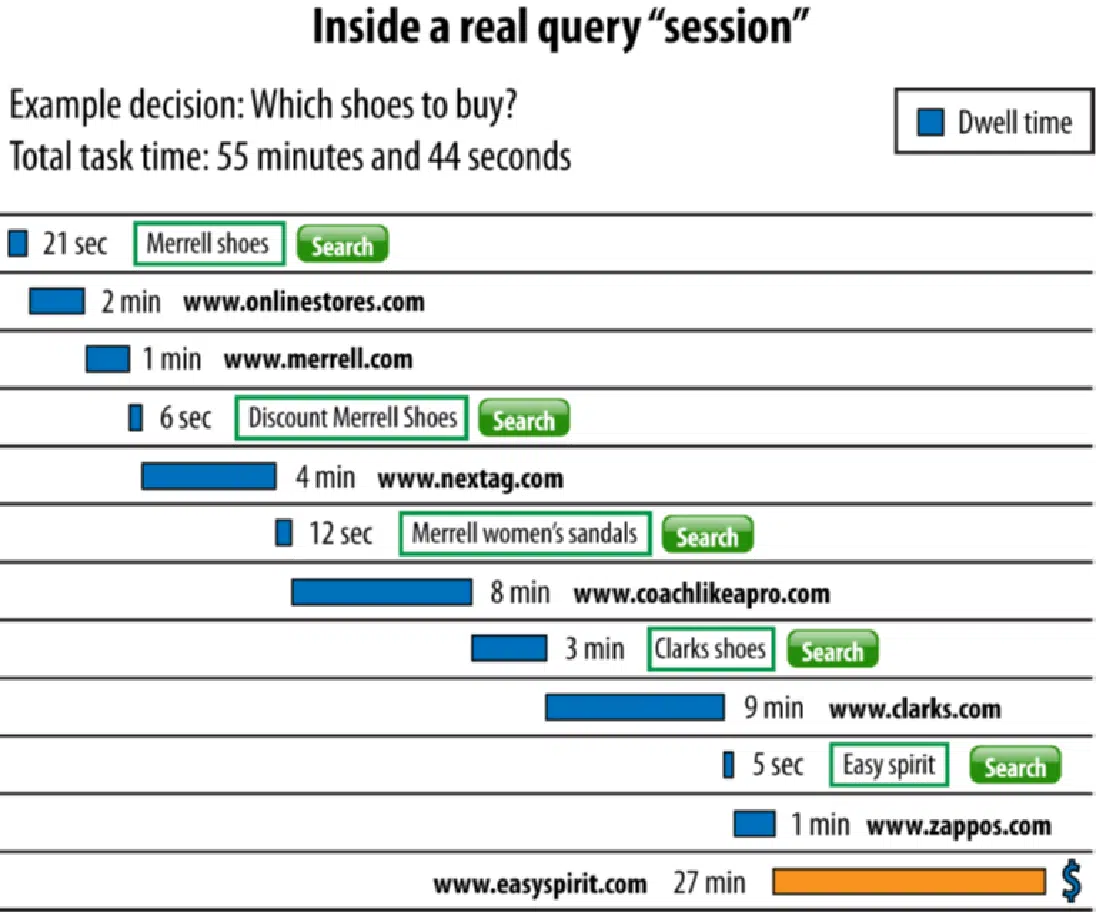
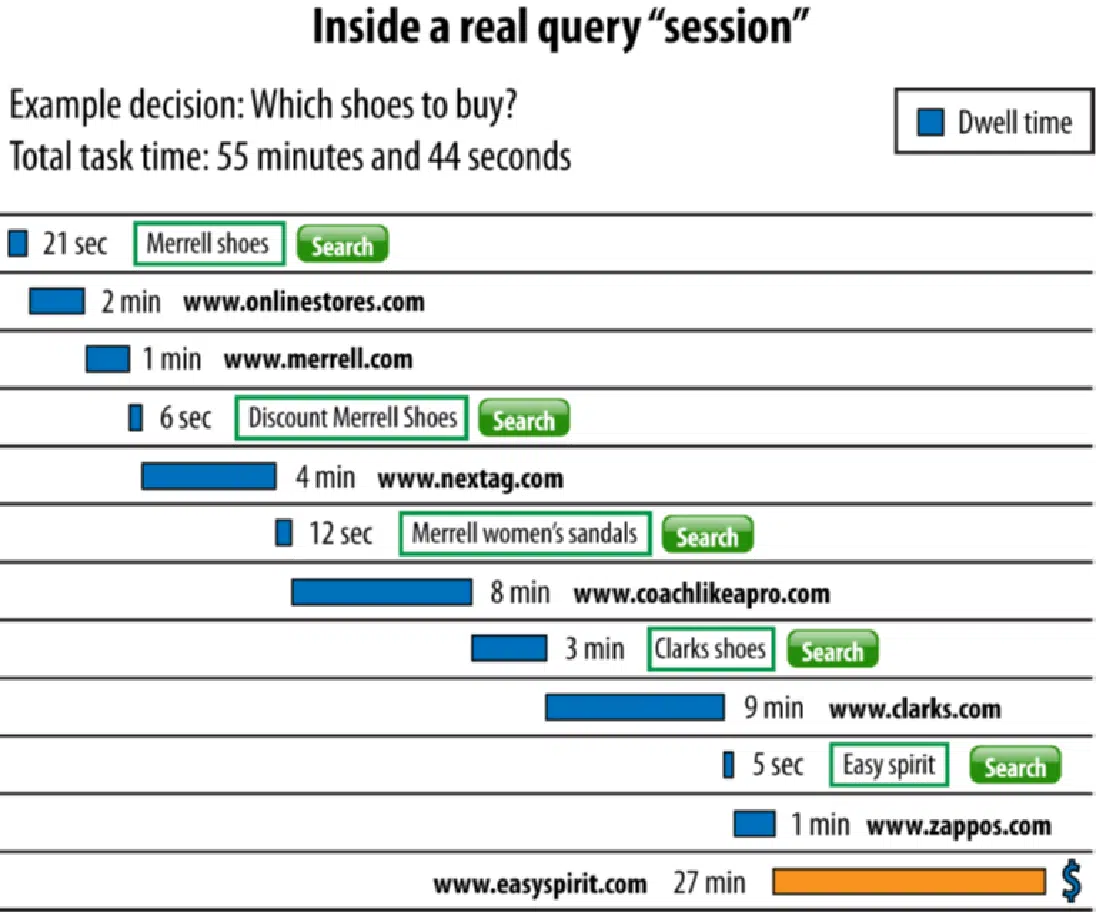
The initial query seems quite simple: “Merrell Shoes.”
You can imagine that the user entering that query often has a specific Merrell shoe in mind, or at least a shoe type, that they want to buy.
However, we see this user’s path has many twists and turns.
For example, the second site they visit is www.merrell.com, a website you might suspect has authoritative information about Merrell shoes.
However, this site doesn’t appear to satisfy the user’s needs.
The user ends up trying four more different queries and visiting six different websites before they finally execute a transaction on www.zappos.com.
This degree of uncertainty in search query journeys is quite common.
Some of the reasons why users have this lack of clarity include is that they:
- Don’t fully understand the need that they’re feeling.
- Don’t know how to ask the right questions to address their need.
- Need more information on a topic before deciding what they need.
- Are in general exploration mode.
Addressing this is an essential aspect of providing a great search experience. This is why the Follow-Up Resources score is part of my analysis.
Understanding categories of queries
Queries can be broadly categorized into several distinct groups, as outlined below:
- Informational: Queries where the user wants information (e.g., “what is diabetes?”).
- Navigational: Queries where the user wants to go to a specific website or page (e.g., “United Mileage Club”).
- Commercial: Queries where the user wants to learn about a product or service (e.g., “Teak dining table”).
- Transactional: Queries where the user is ready to conduct a transaction (e.g., “pizza near me”).
Recent data from SparkToro’s Rand Fishkin provides some insight into the percentage of search queries that fall into each of these categories:
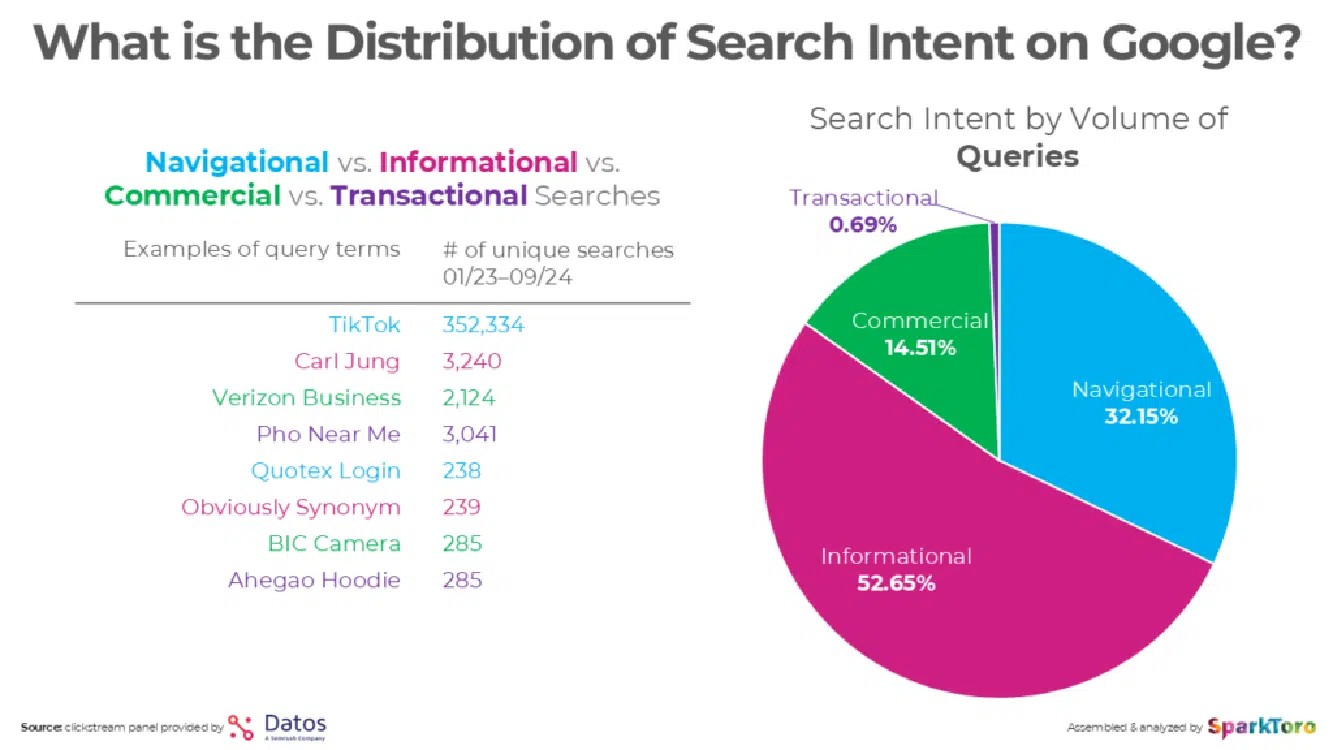
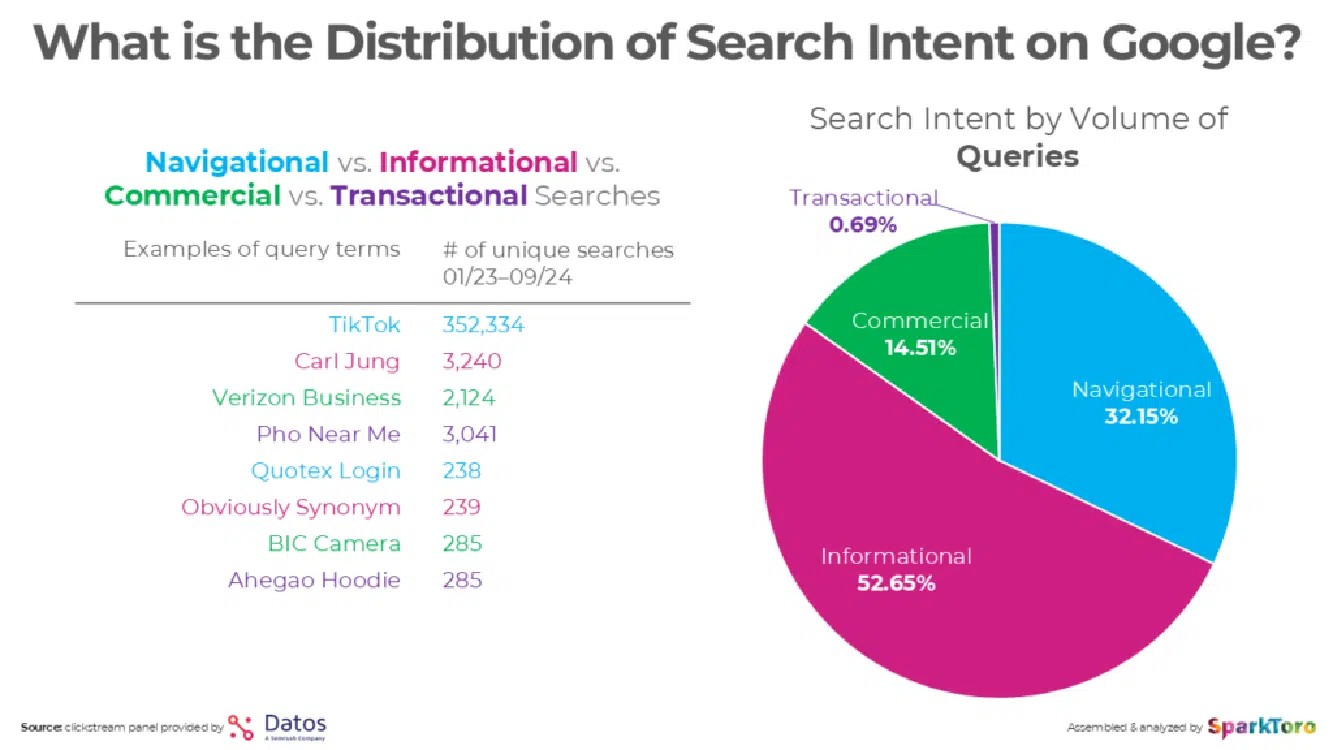
Be advised that the above is a broad view of the categories of queries.
The real work in search relates to handling searches on a query-by-query basis. Each query has many unique aspects that affect how it can be interpreted.
Next, we’ll examine several examples to illustrate this. Then, we’ll compare how ChatGPT search and Google performed on these queries.
Query type: Directions
This query type is a natural strength for Google (as is any locally oriented query). We can see ChatGPT search’s weaknesses in this area in its response:
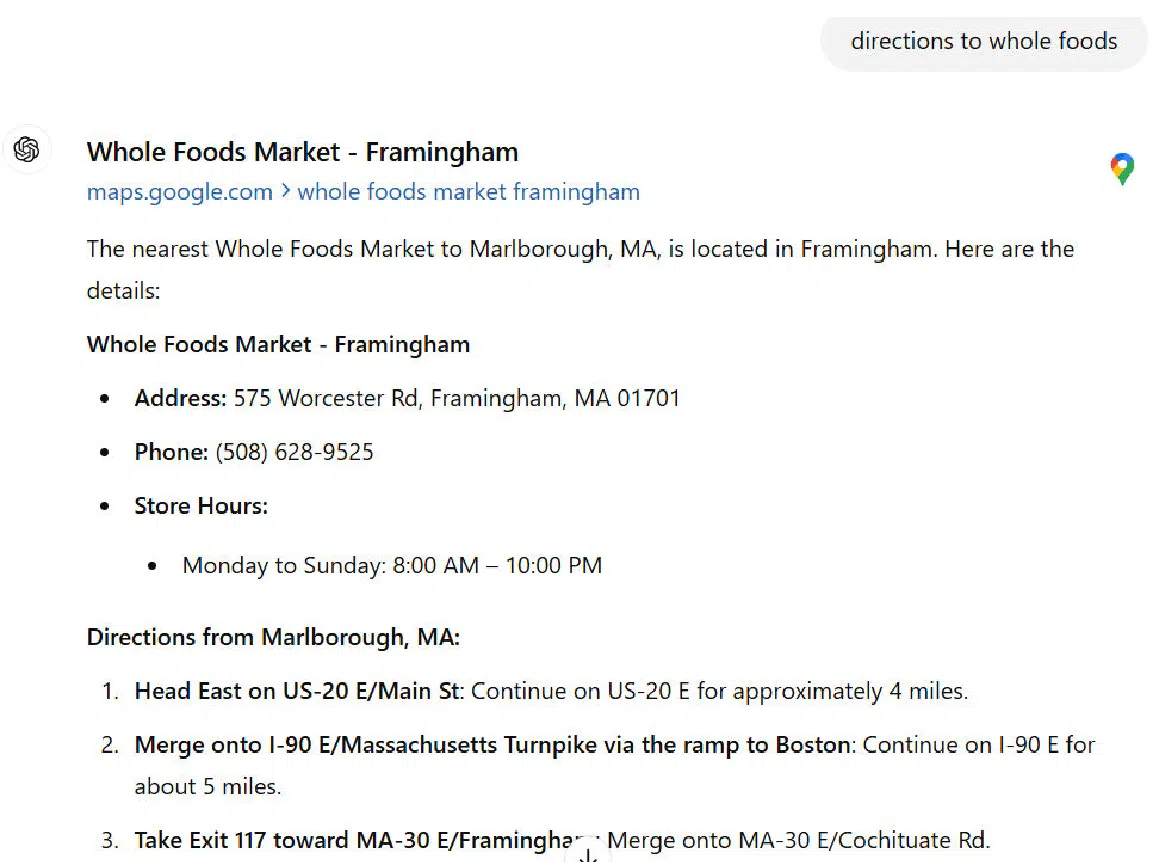
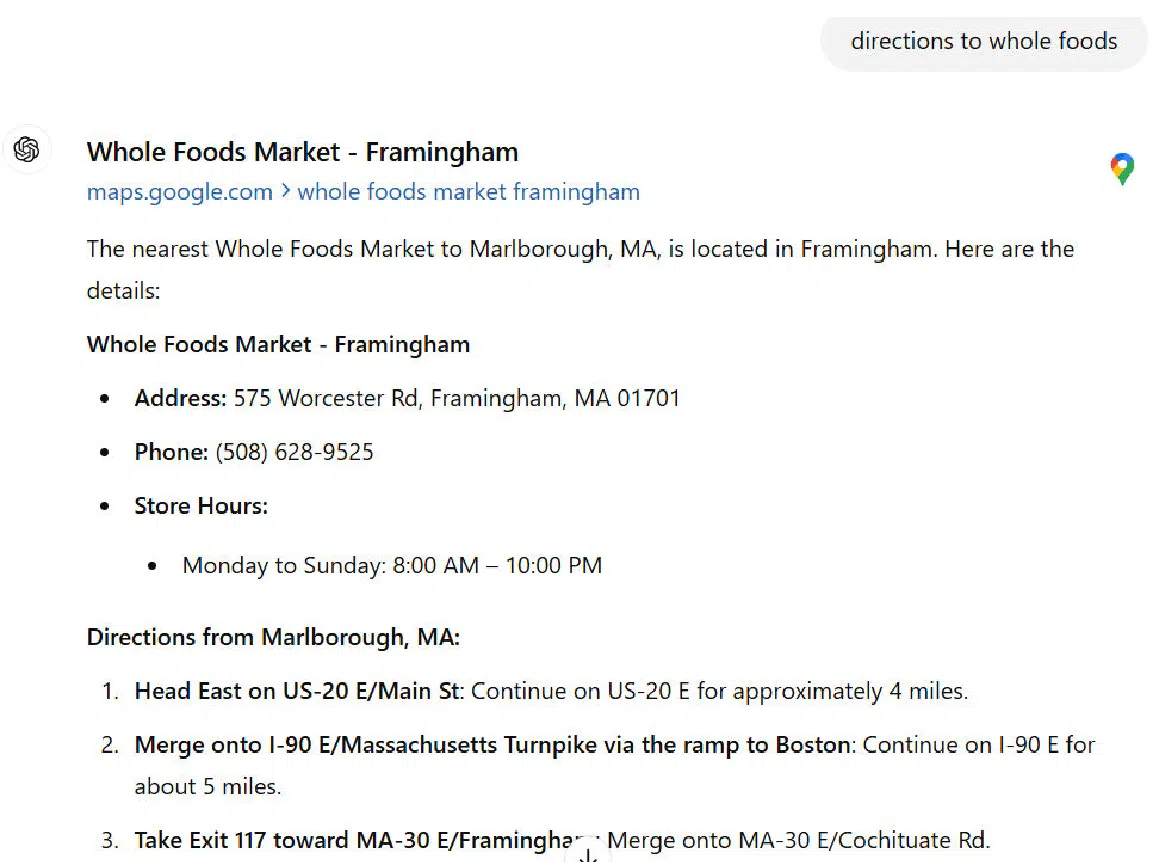
The problems with this response are numerous.
For example, I wasn’t in Marlborough, Massachusetts, when I did the query (I was in the neighboring town of Southborough).
In addition, steps 1 and 2 in the directions are unclear. Anyone following them and heading east on Route 20 would end up at Kenmore Square in Boston without ever crossing I-90 East.
In contrast, Google nails it:
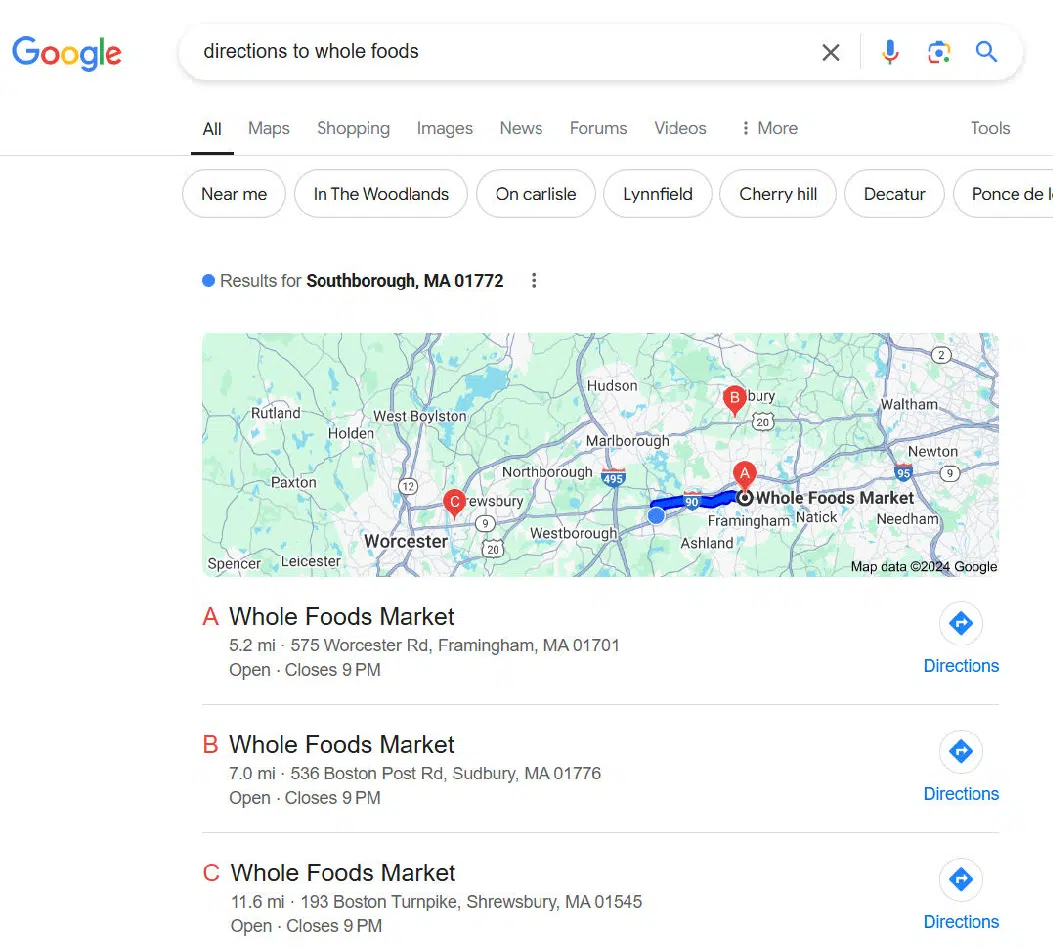
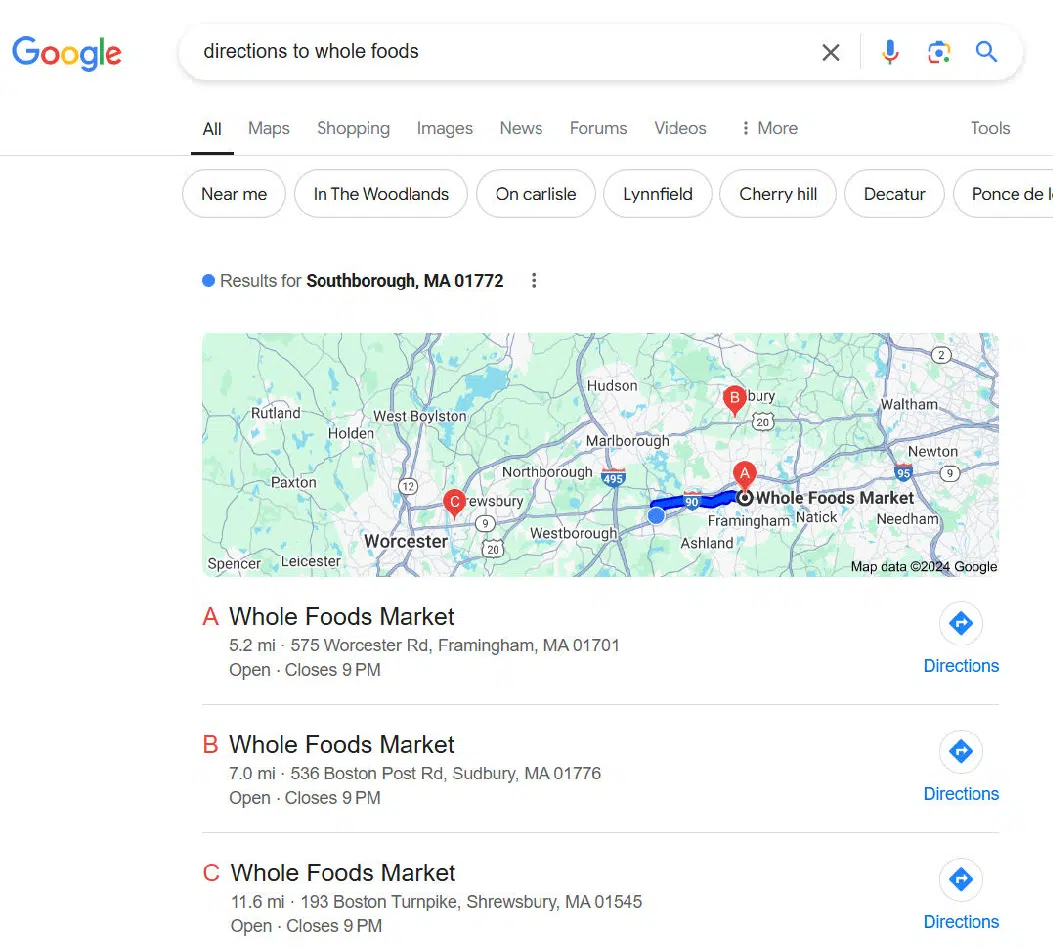
The reason why Google handles this better is simple.
Google Maps has an estimated 118 million users in the U.S., and Waze adds another 30 million users.
I wasn’t able to find a reasonable estimate for Bing Maps, but suffice it to say that it’s far lower than Google’s.
The reason Google is so much better than Bing here is simple – I use Google Maps, and that lets Google know exactly where I am.
This advantage applies to all Google Maps and Waze users in the U.S.
Query type: Local
Other types of local queries present similar issues to those of ChatGPT search. Note that a large percentage of search queries have local intent.
One estimate pegged this at 46% of all queries. This was reportedly shared by a Googler during a Secrets of Local Search conference at GoogleHQ in 2018.
Here is ChatGPT’s response to one example query that I tested:
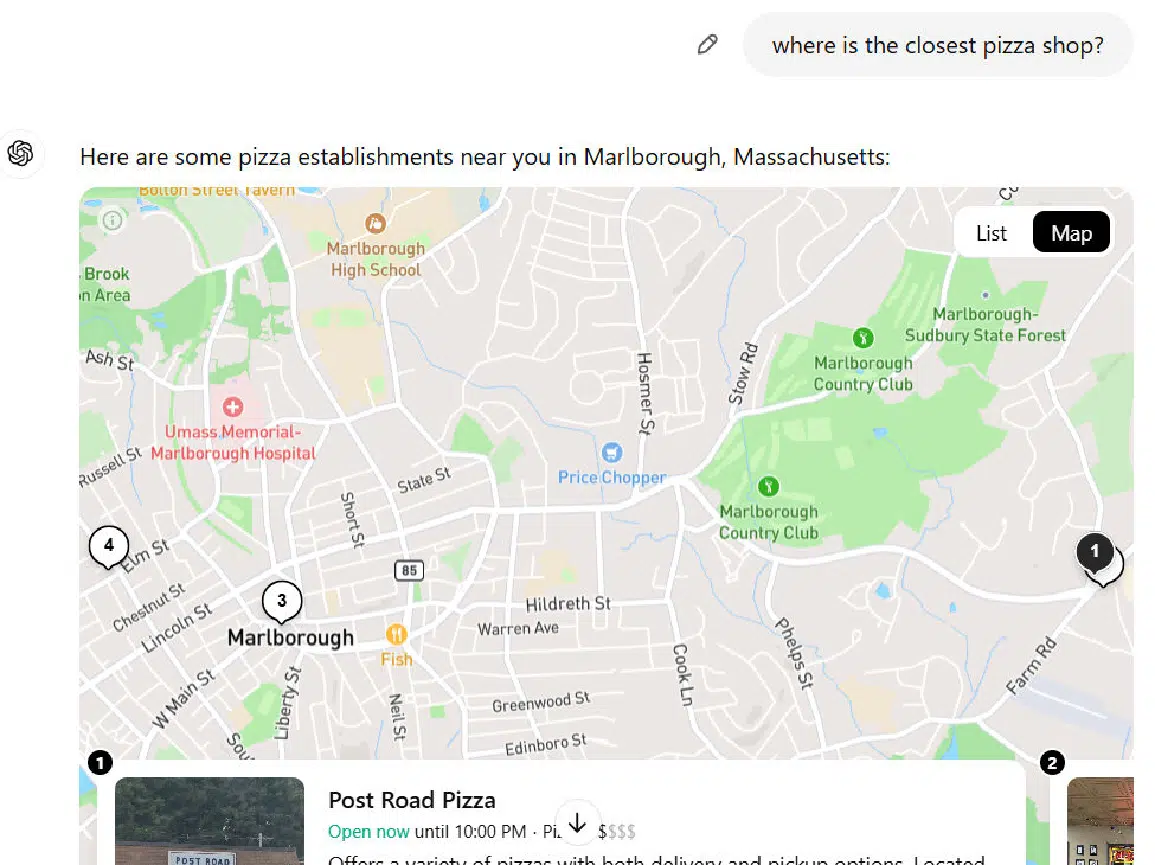
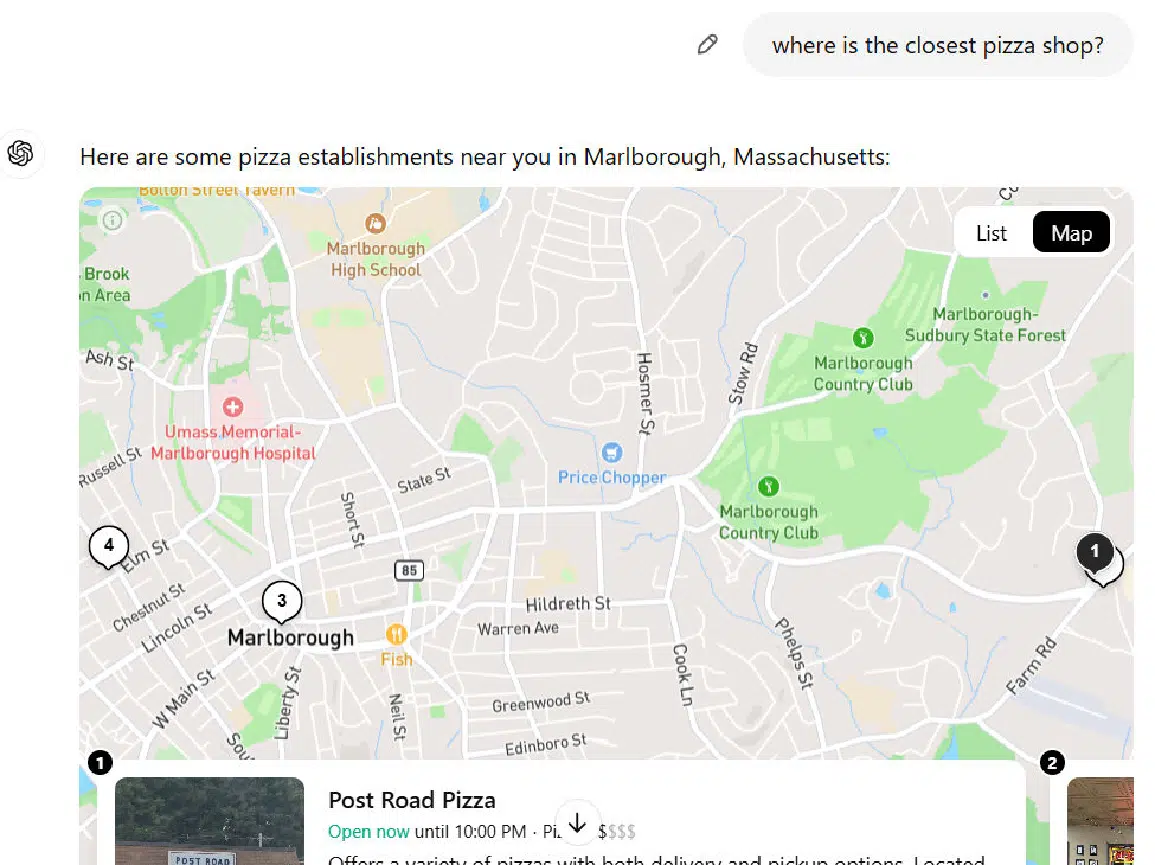
As with the directions example, it thinks that I’m in Marlborough.
In addition, it shows two pizza shops in Marlborough (only one of the two is shown in my screenshot).
Google’s response to this query is much more on point:
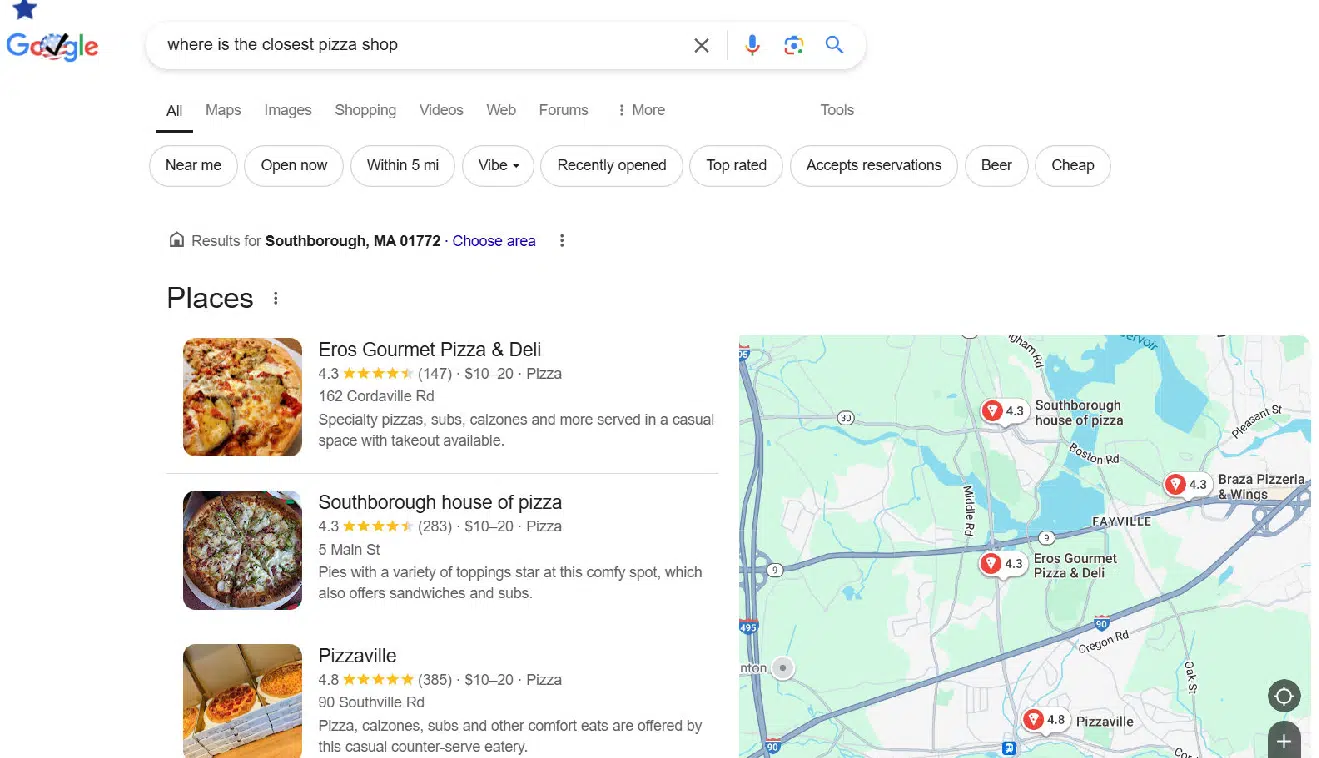
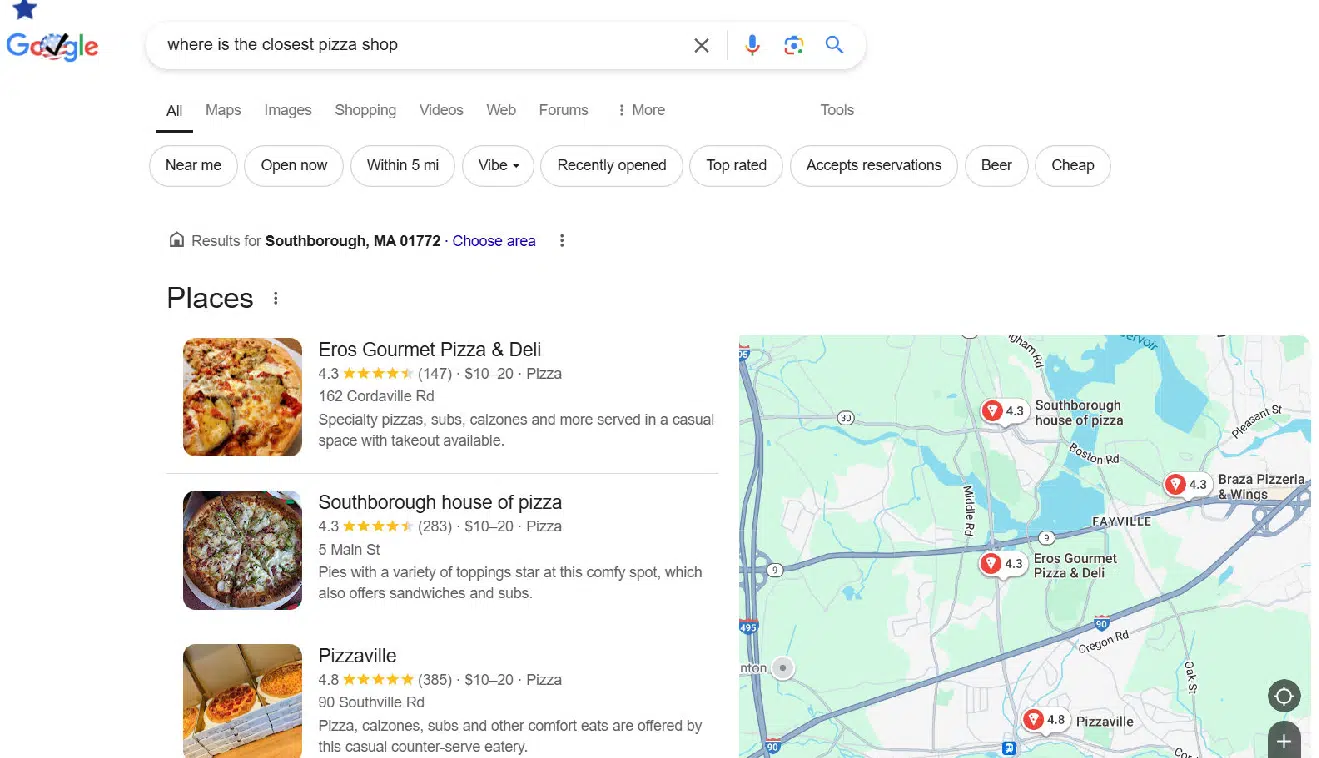
I also gave Google a second version of the query “Pizza shops in Marlborough,” and it returned 11 locations – 9 more than I saw from the ChatGPT search.
This shows us that Google also has far more access to local business data than ChatGPT search.
For this query class (including the Directions discussed previously), I assigned these scores:
- ChatGPT search: 2.00.
- Google: 6.25.
Query type: Content gap analysis
A content gap analysis is one of the most exciting SEO tasks that you can potentially do with generative AI tools.
The concept is simple: provide the tool of your choice a URL from a page on your site that you’d like to improve and ask it to identify weaknesses in the content.
As with most things involving generative AI tools, it’s best to use this type of query as part of a brainstorming process that your subject matter expert writer can use as input to a larger process they go through to update your content.
There are many other different types of content analysis queries that you can do with generative AI that you can’t do with Google (even with AI Overviews) at this point.
For this study, I did four content gap analysis queries to evaluate how well ChatGPT search did with its responses.
Google presented search results related to the page I targeted in the query but did not generate an AI Overview in any of the four cases.
However, ChatGPT search’s responses had significant errors for three of the four queries I tested.
Here is the beginning of ChatGPT search’s response to the one example query where the scope of errors was small:
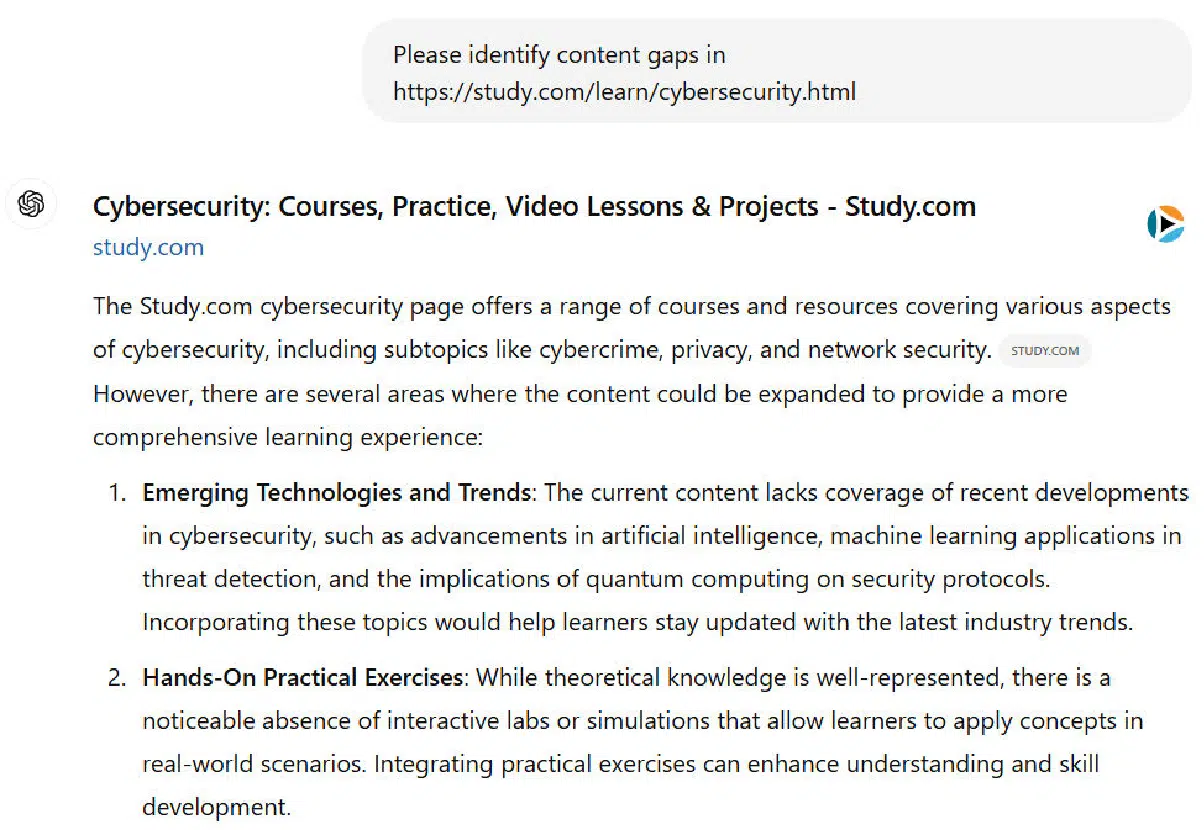
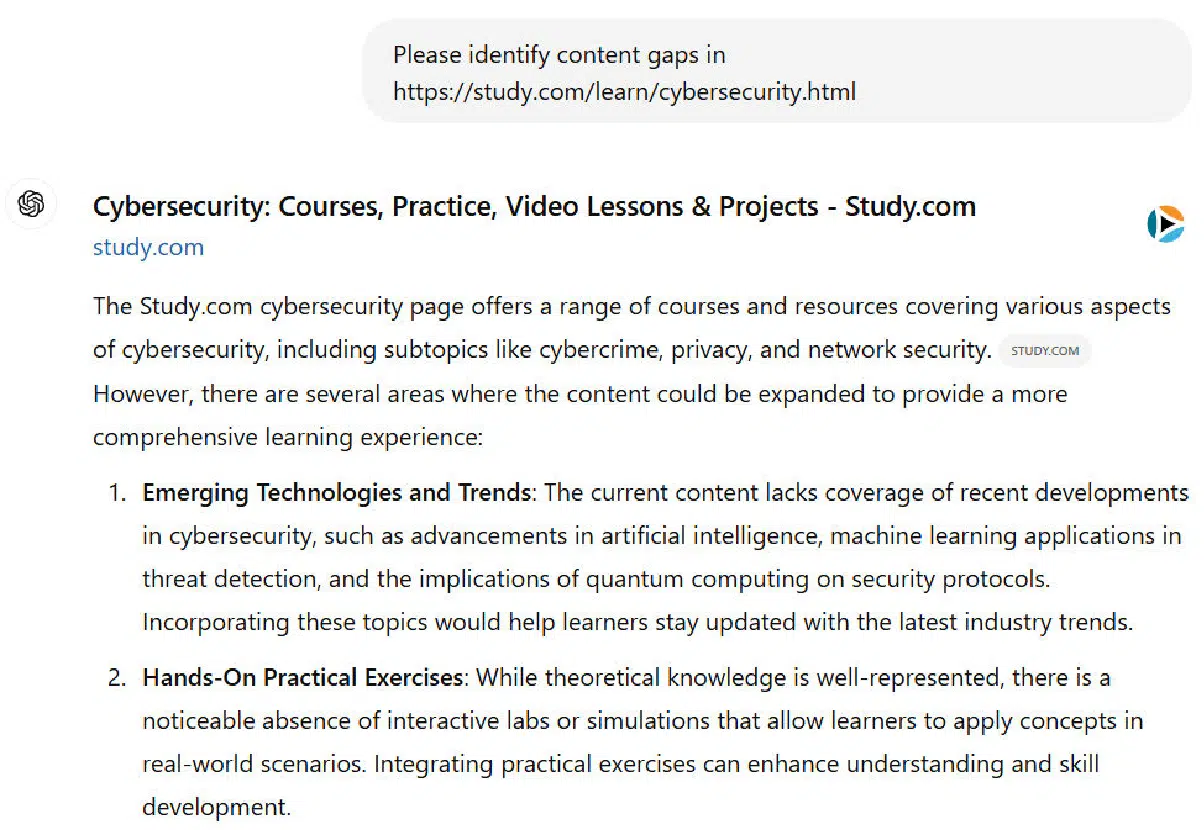
This result from ChatGPT isn’t perfect (there are a few weaknesses, but it’s pretty good. The start of Google’s response to the same query:
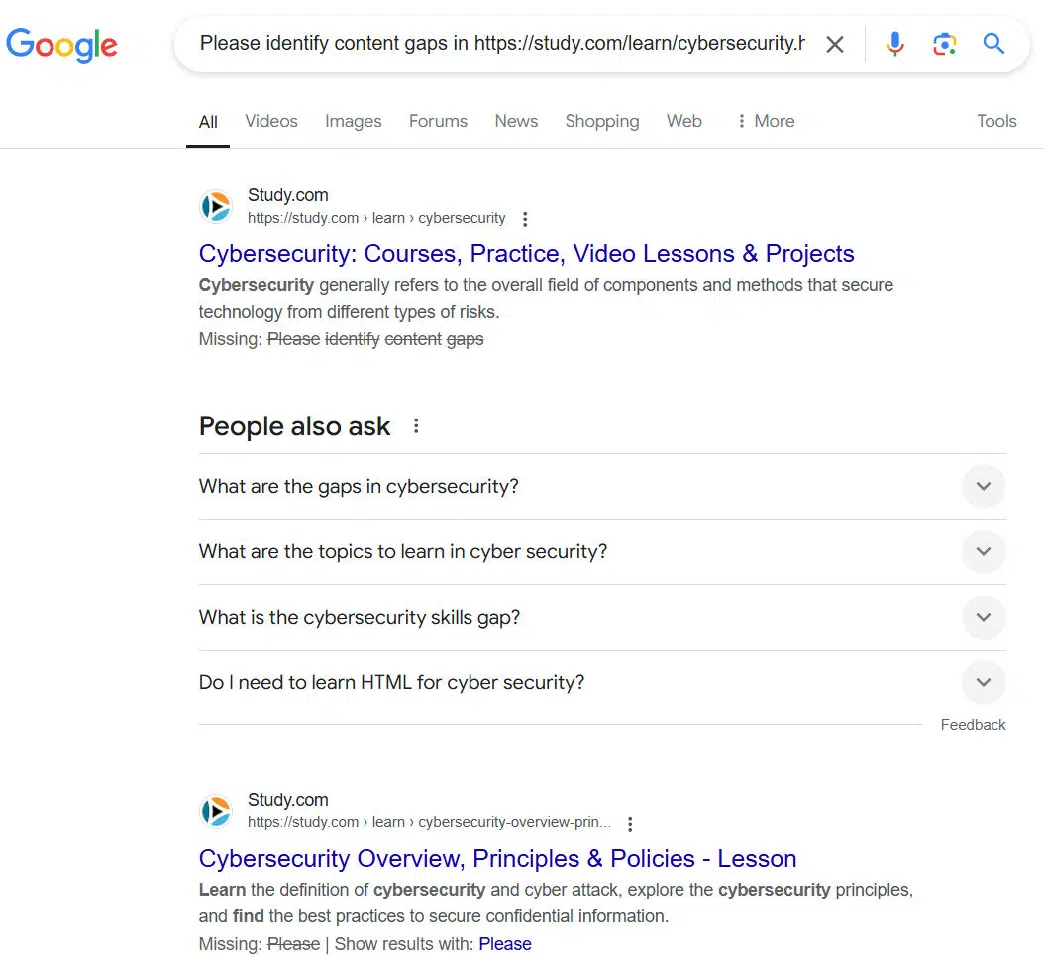
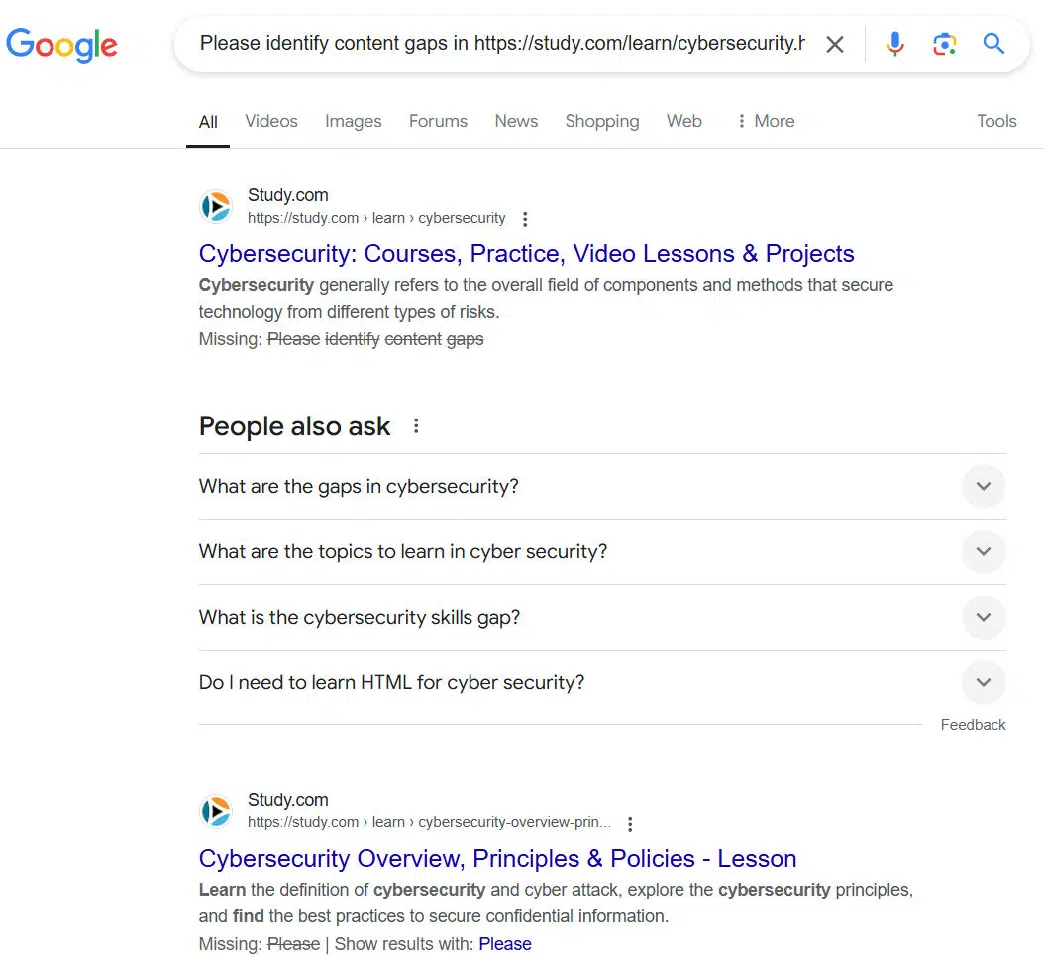
As you can see, Google hasn’t even attempted to perform a content gap analysis. ChatGPT search is better set up to address this type of query.
However, ChatGPT search doesn’t earn a clean sweep for this type of query.
Here is the first part of another example result:
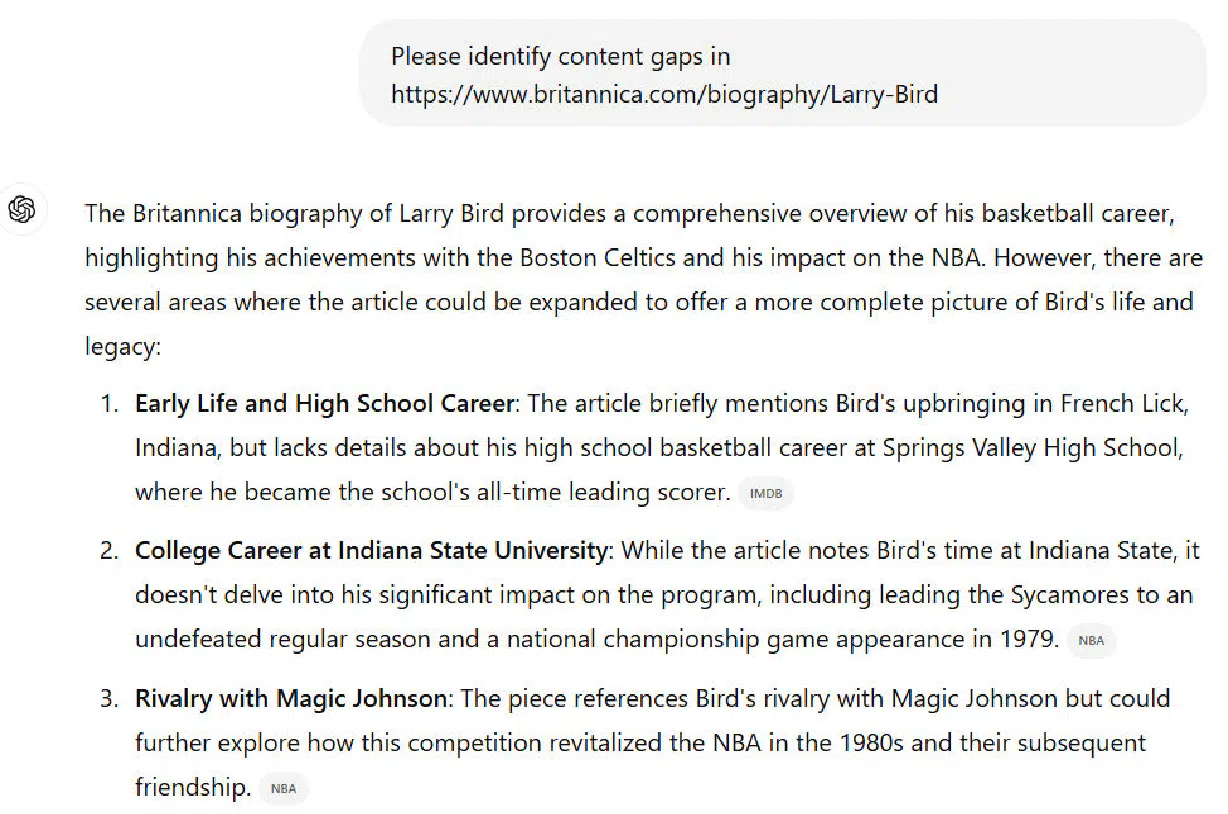
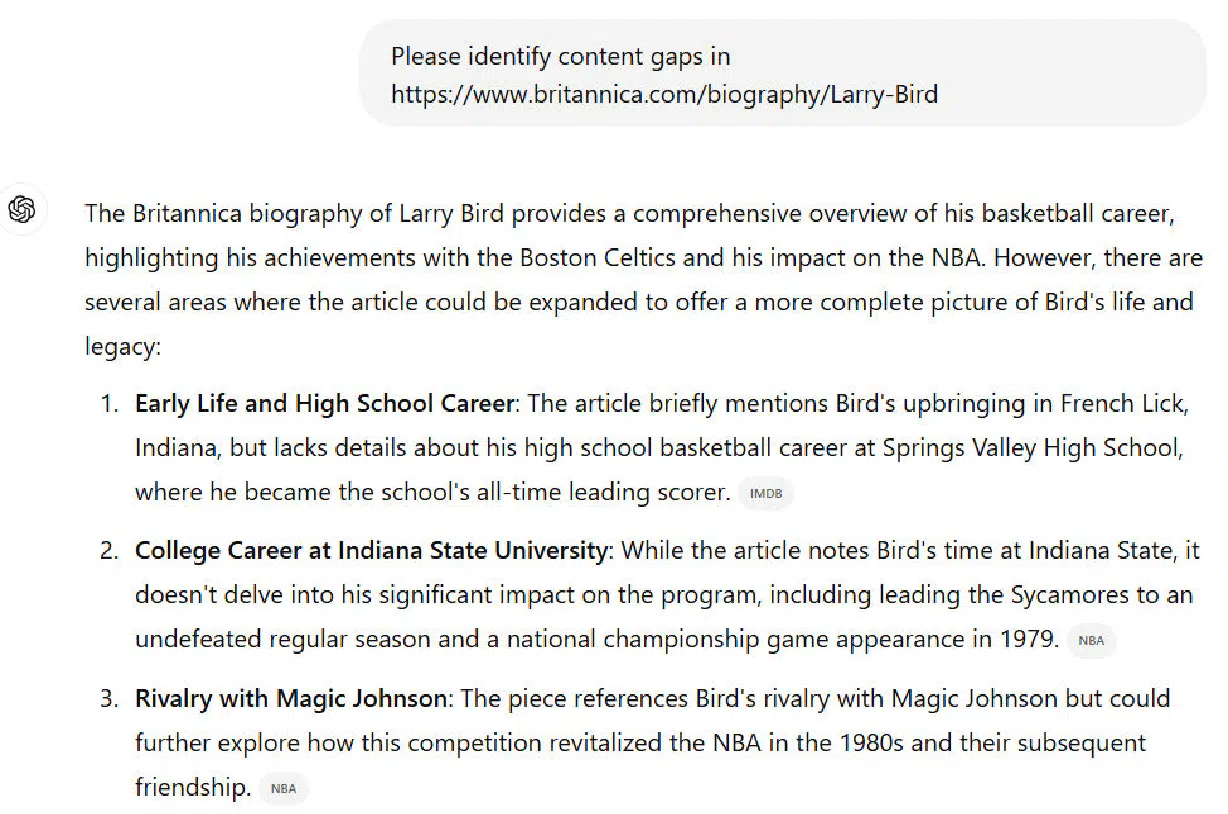
This looks good in principle, but it’s filled with errors. Some of these are:
- The Britannica article does discuss the depth of Larry Bird’s impact on Indiana State University.
- The Britannica article does mention the importance of the Larry Bird / Magic Johnson rivalry to the NBA
- The ChatGPT search response is longer than shown here and there are other errors beyond what I mention here.
Overall, I tried four different content gap analysis queries and ChatGPT search made significant errors in three of them. For this query, I assigned these scores:
- ChatGPT search: 3.25.
- Google: 1.00.
Query type: Individual bio
How these queries perform is impacted by how well-known the person is.
If the person is very famous, such as Lionel Messi, there will be large volumes of material written about them.
If the amount of material written about the person is relatively limited, there is a higher probability that the published online information hasn’t been kept up to date or fact-checked.
We see that in the responses to the query from both ChatGPT search and Google.
Here is what we see from ChatGPT search:
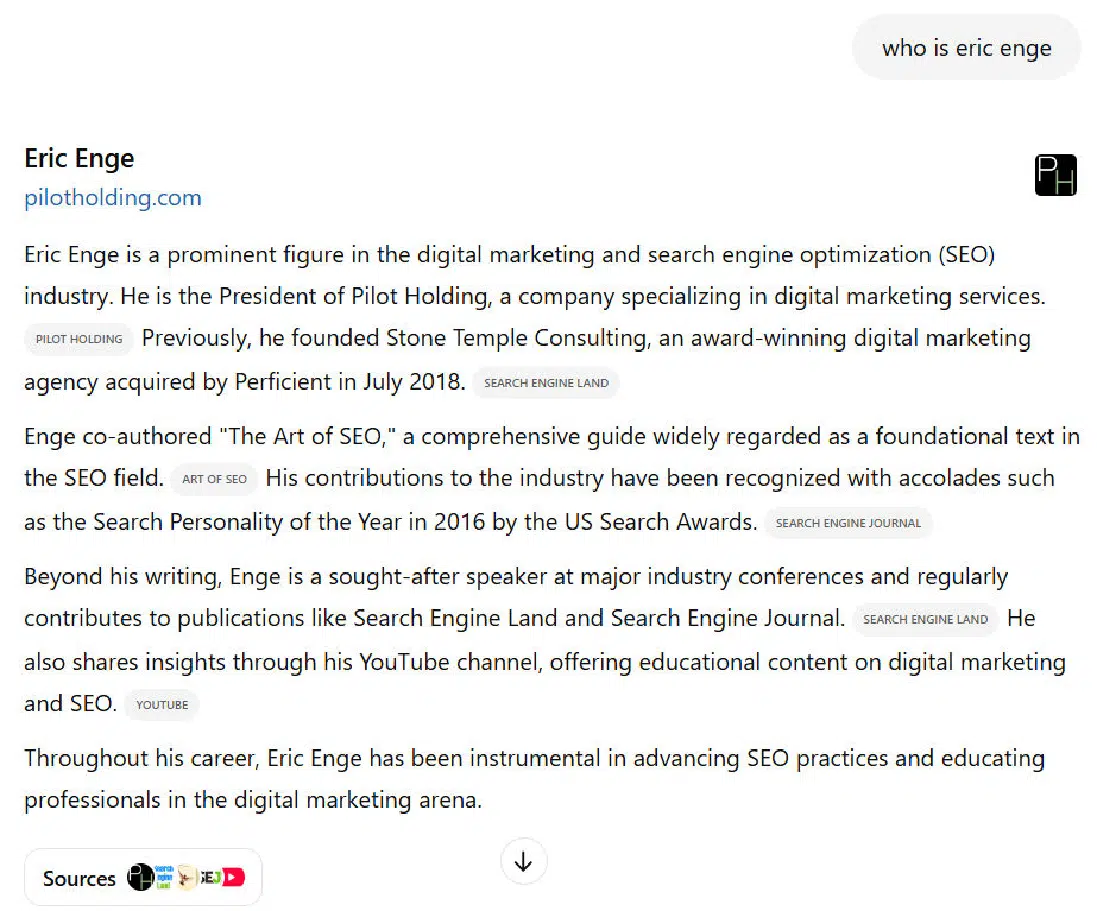
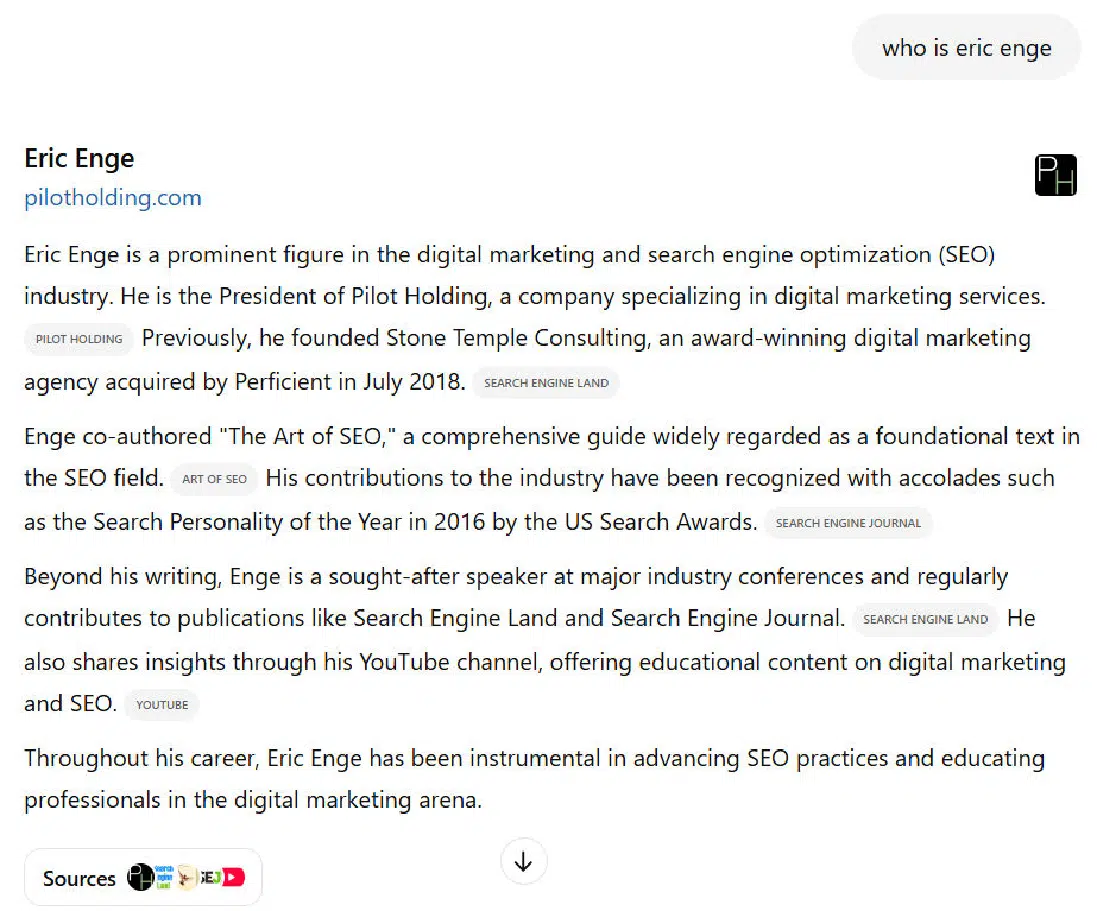
The main issues with this response are in the third paragraph.
I haven’t written for Search Engine Journal in a long time, and it’s also been more than six years since I published a video on my YouTube channel (@stonetemplecons).
Let’s see what Google has to say:
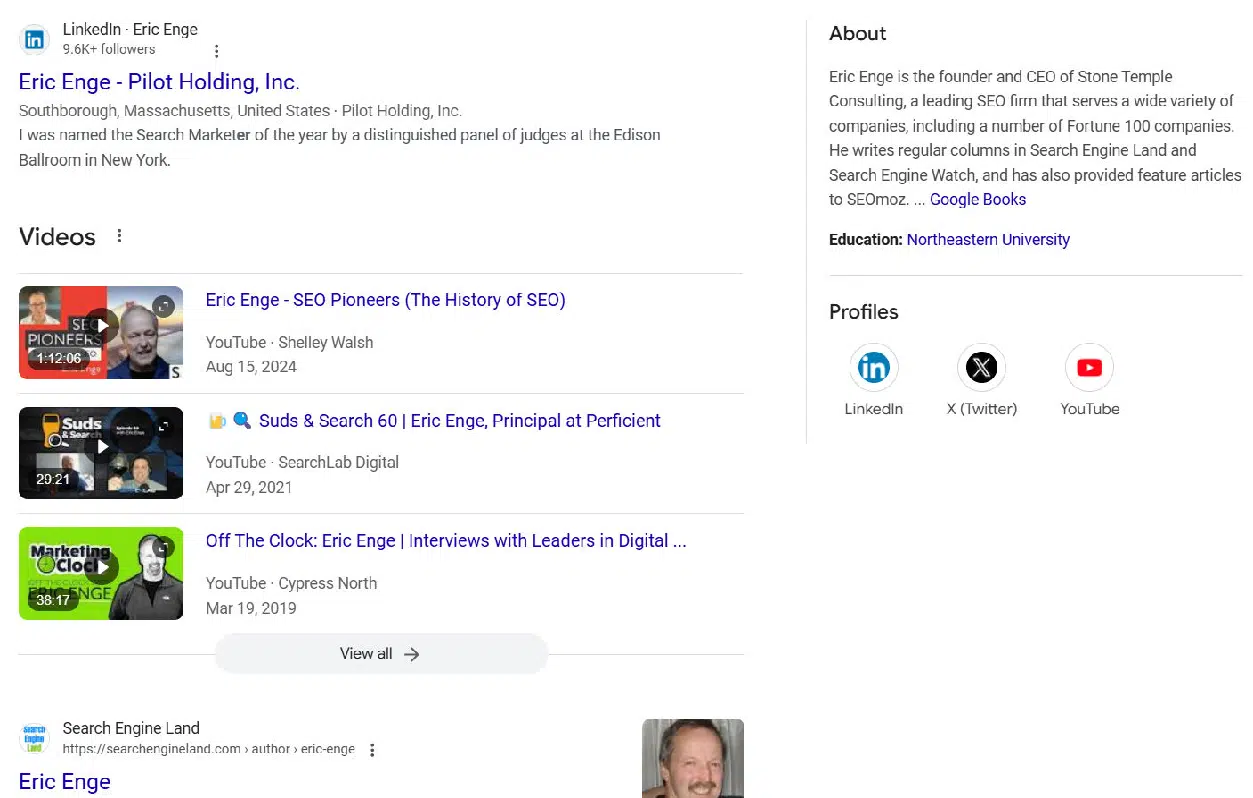
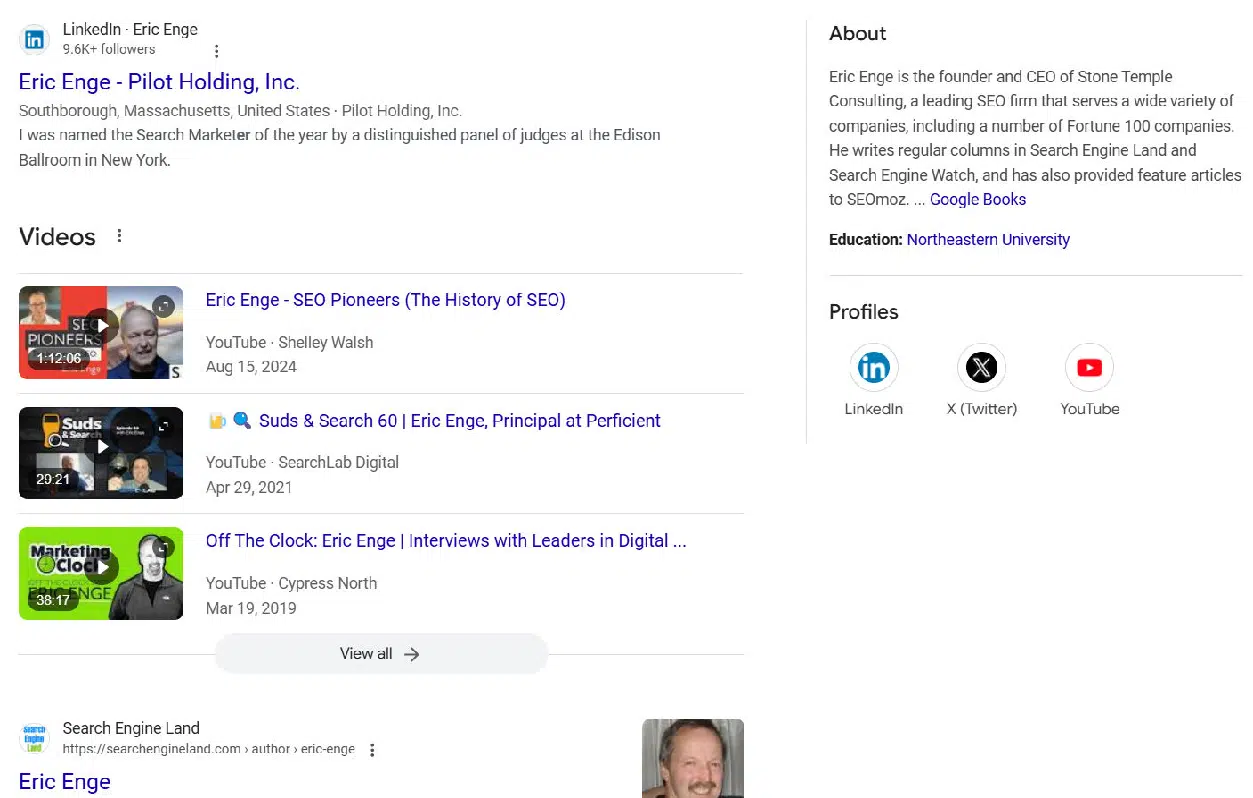
Google also has problems with its response. They lead with quite a few images of me (which are all accurate), and below that, they show my LinkedIn profile and a summary of me drawn from Google Books.
Here, it says that I write for Search Engine Watch (haven’t done that for more than a decade!) and SEOMoz (which rebranded to SEOmoz to Moz in 2013) (also more than a decade!).
These responses are both examples of what I call “Garbage-In-Garbage-Out” queries.
If the web sources aren’t accurate, the tools don’t have the correct information to render.
For bio queries (3 of them), I scored the competitors as follows:
- ChatGPT search: 6.00.
- Google: 5.00.
Query type: Debatable user intent
Arguably, nearly every search query has debatable user intent, but some cases are more extreme than others.
Consider, for example, queries like these:
- Diabetes.
- Washington Commanders.
- Physics.
- Ford Mustang.
Each of these examples represents an extremely broad query that could have many different intents behind it.
In the case of diabetes:
- Does the person just discover that they have (or a loved one has) diabetes, and they want a wide range of general information on the topic?
- Are they focused on treatment options? Long-term outlook? Medications? All of the above?
Or, for a term like physics:
- Do they want a broad definition of what it’s about?
- Or is there some specific aspect of physics that they wish to learn much more about?
Creating the best possible user experience for queries like these is tricky because your response should provide opportunities for each of the most common possible user intents.
For example, here is how ChatGPT responded to the query “physics”:
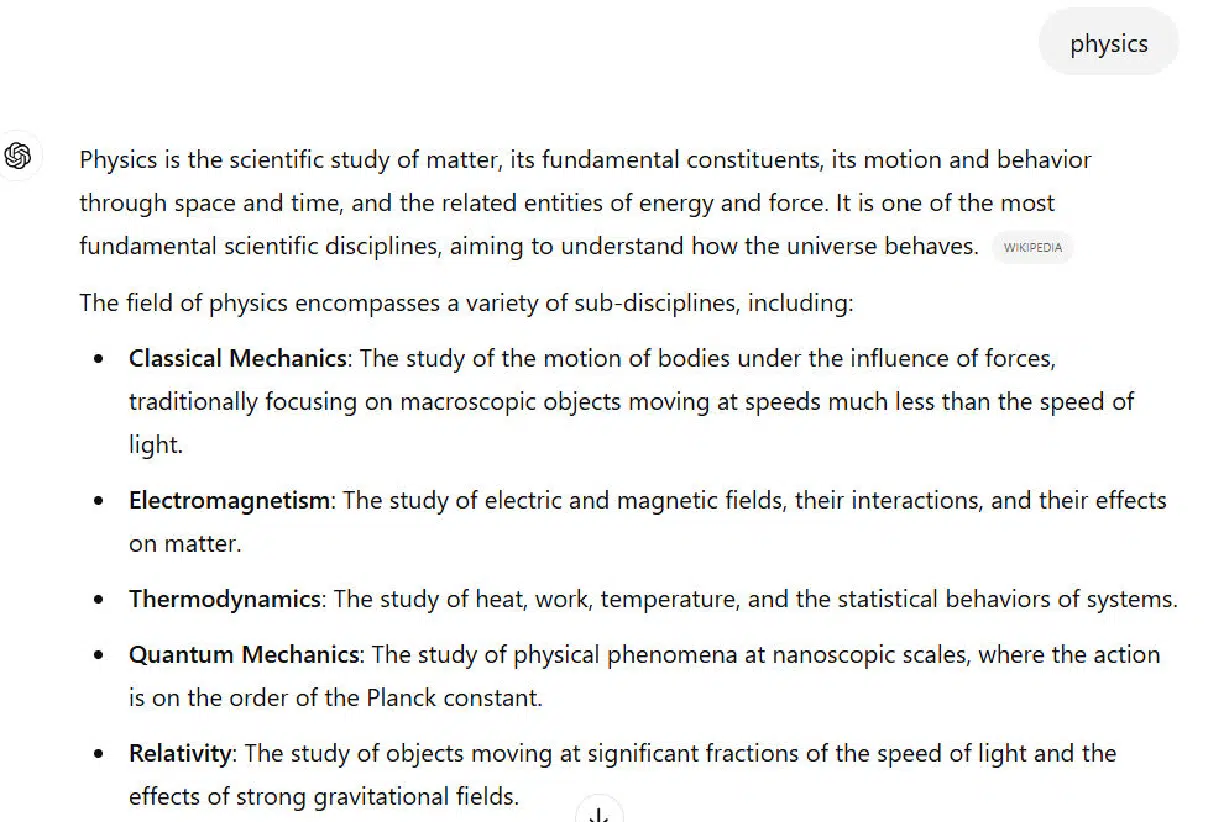
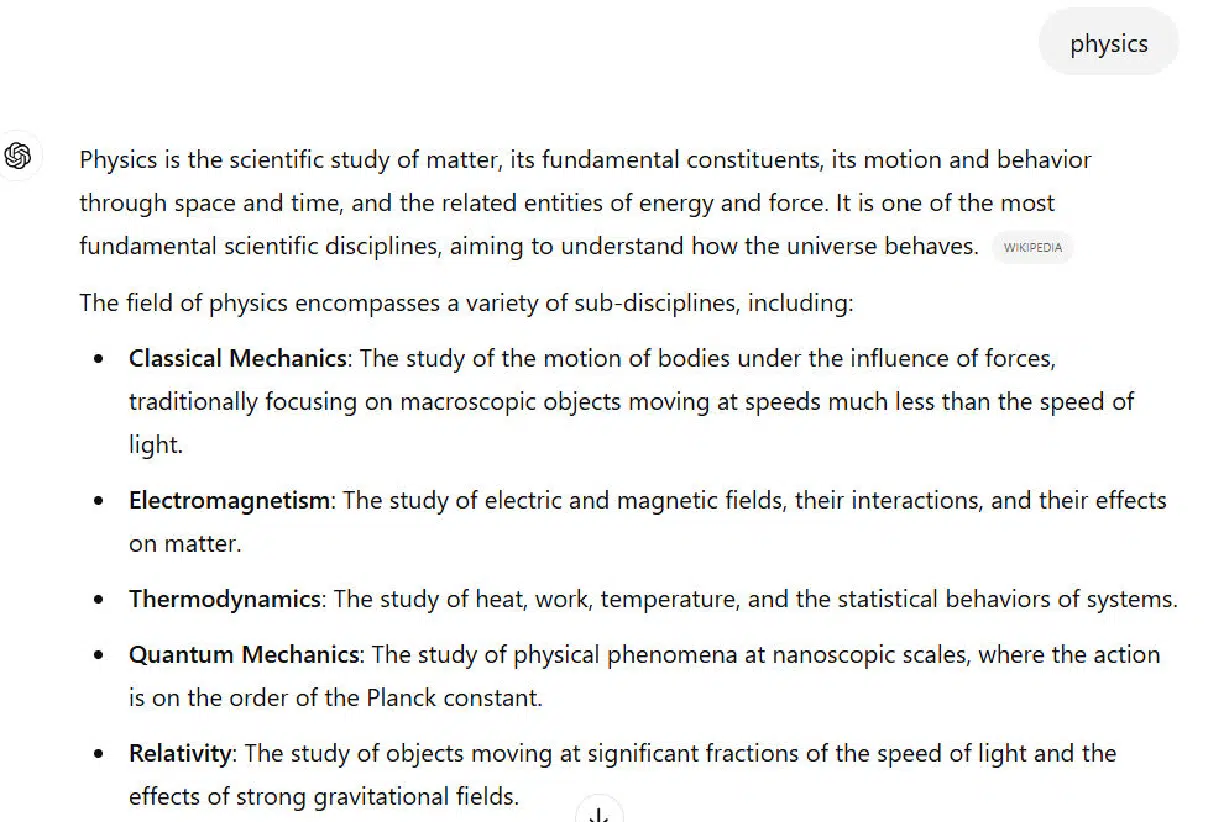
The additional two paragraphs of the response focused on the definition of Physics and kept the response at a very high level.
In contrast, the beginning of Google’s response also focuses on a broad definition of physics, but following that are People Also Ask and Things to Know boxes that address many other potential areas of interest to people who type in this search query:
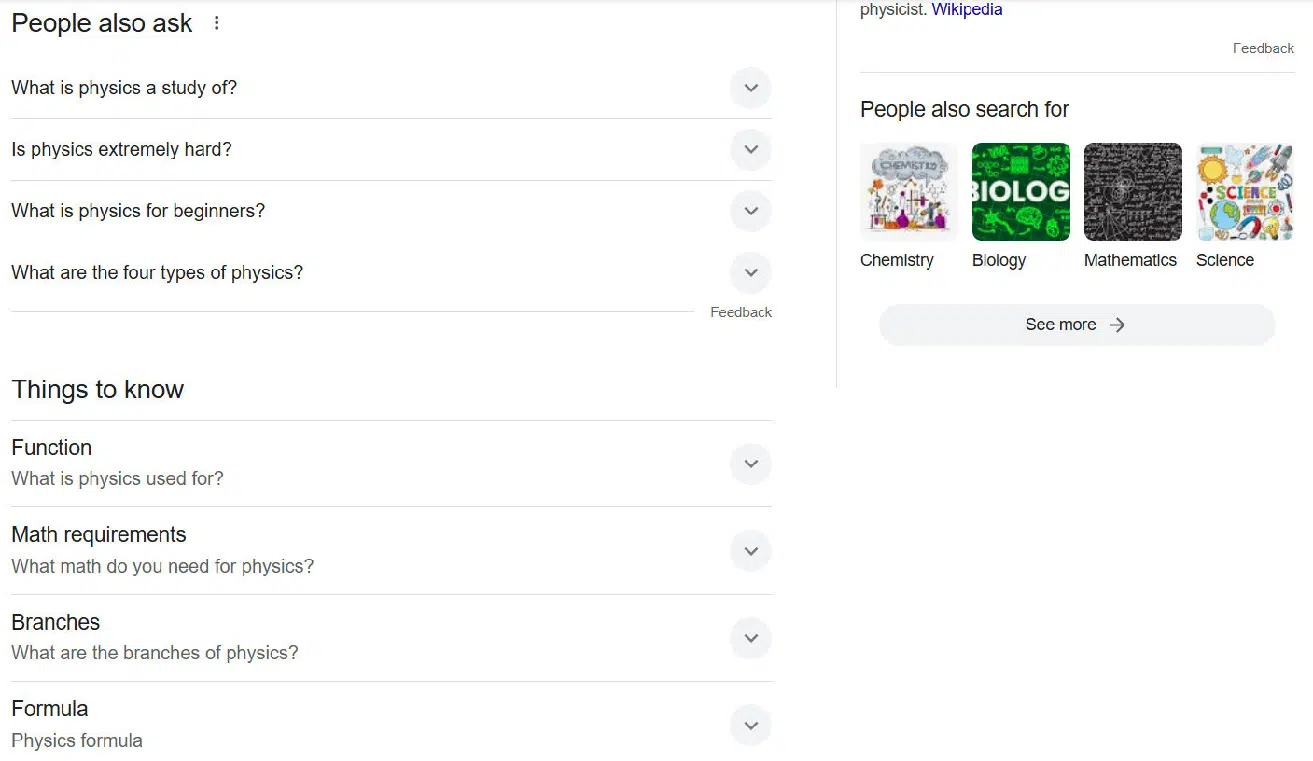
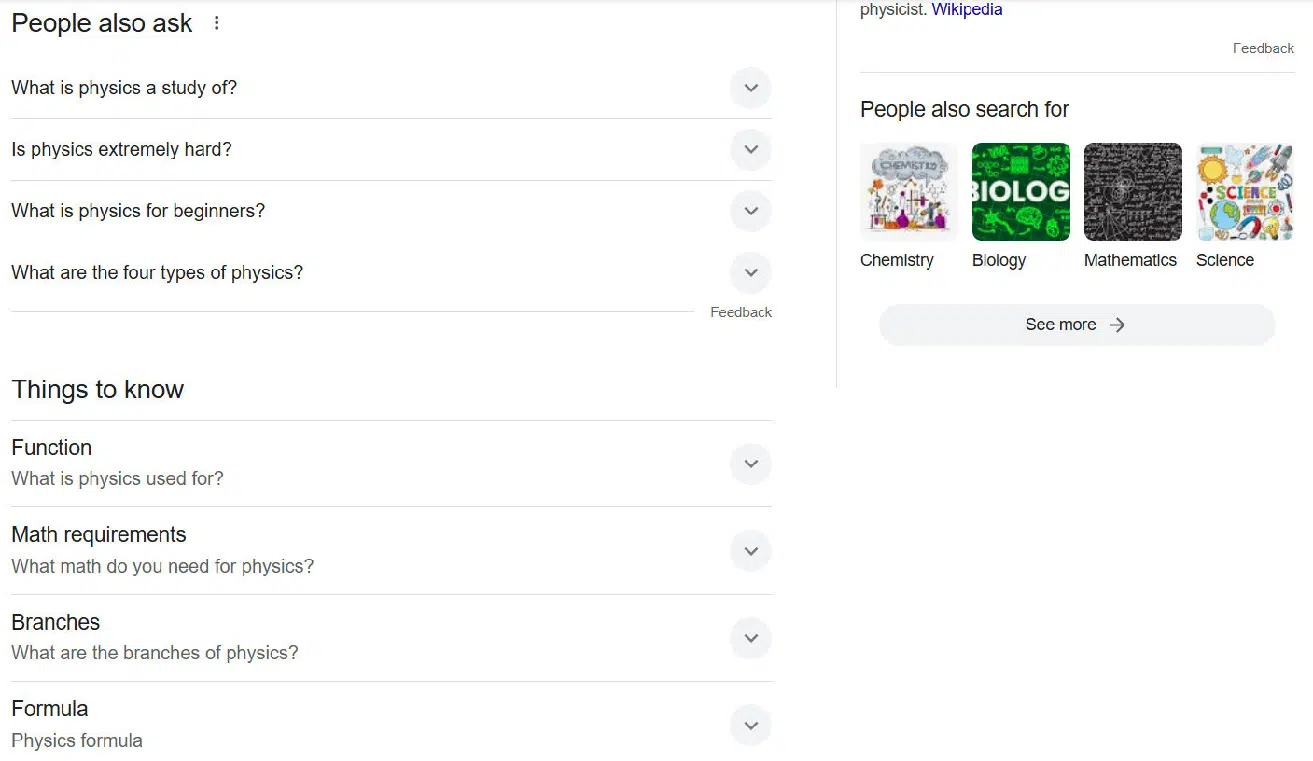
This part of Google’s response shows a recognition of the many possible intents that users who type in the phrase “physics” may have in mind.
For this query, I assigned these scores:
- ChatGPT search: 5.00.
- Google: 7.00.
Query type: Disambiguation
One special class of debatable intents queries is words or phrases that require disambiguation. Here are some example queries that I included in my test set:
- Where is the best place to buy a router?
- What is a jaguar?
- What is mercury?
- What is a joker?
- What is a bat?
- Racket meaning.
For example, here is how ChatGPT search responded to the question, “What is a joker query?”
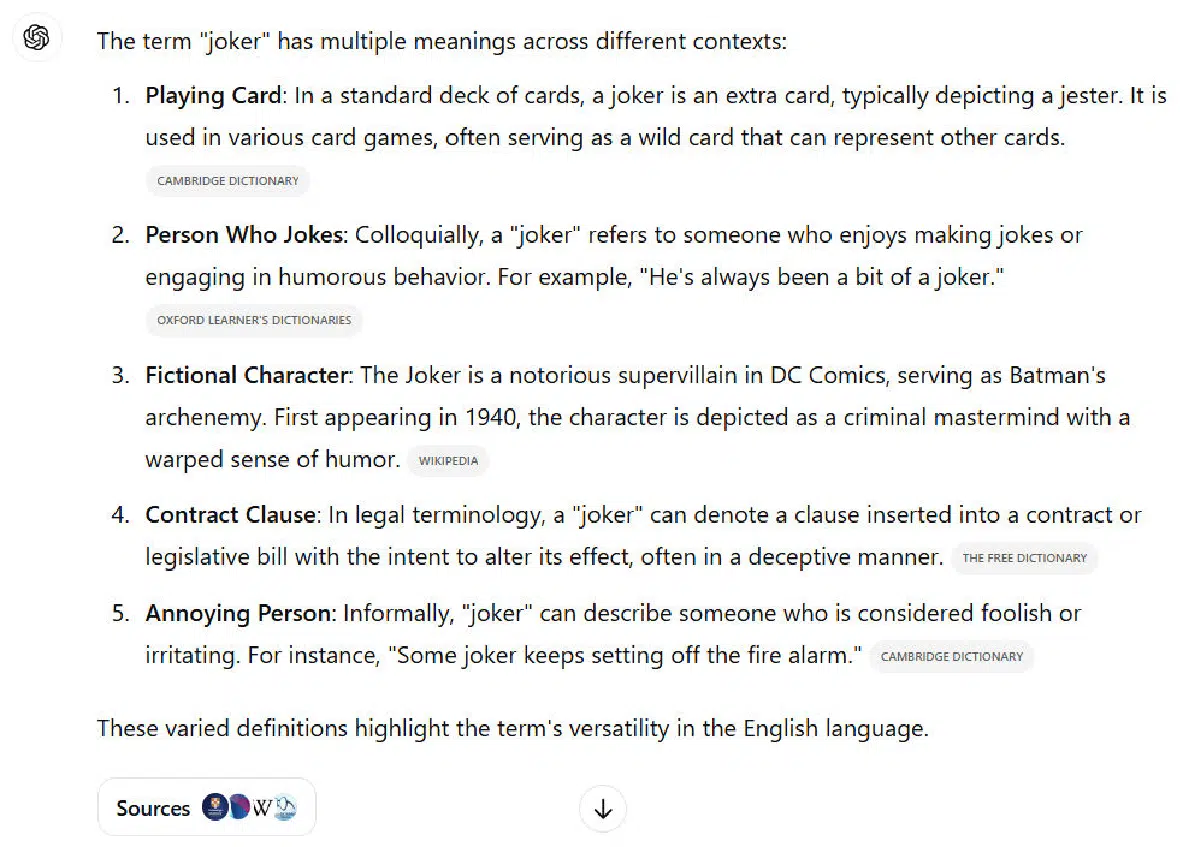
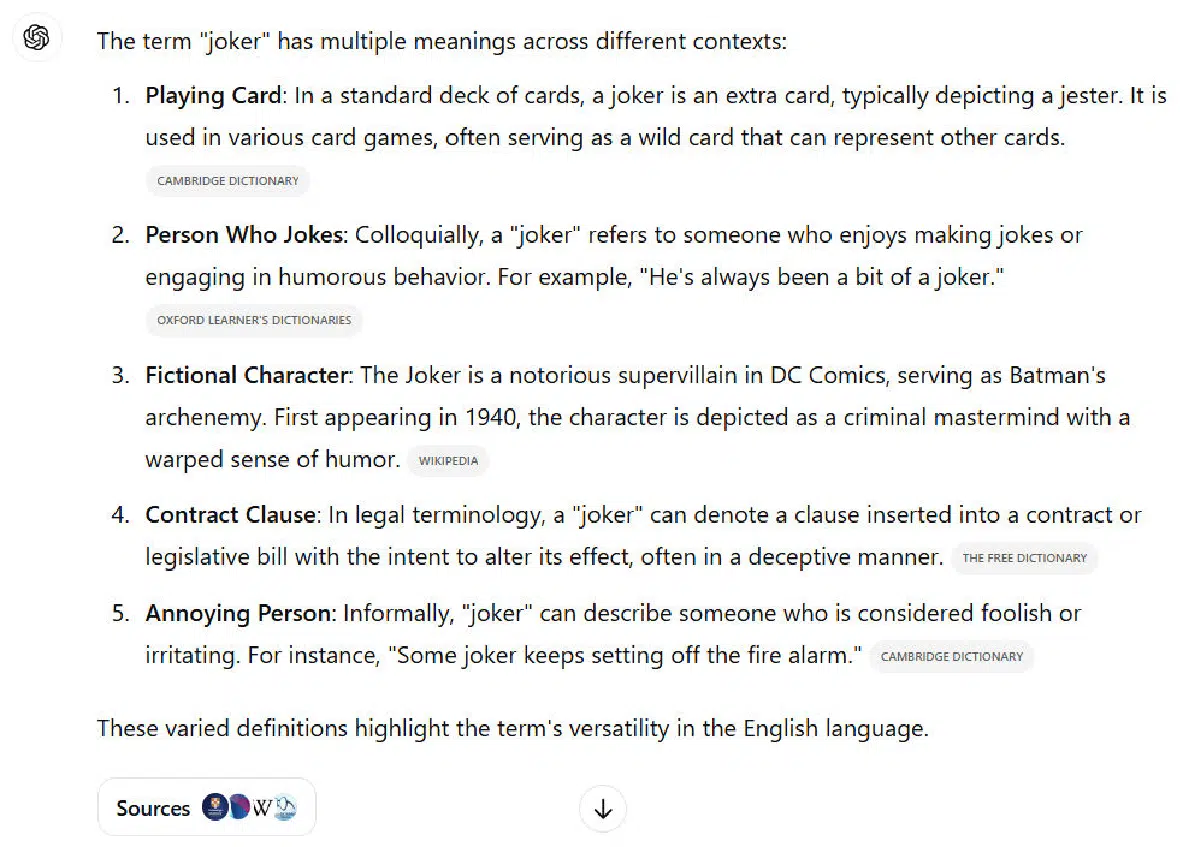
We can see that it offers a nice disambiguation table that provides a brief definition for five different meanings of the term.
It also includes links to pages on the web that users can visit for information related to each meaning.
In contrast, Google focuses on two major intents:
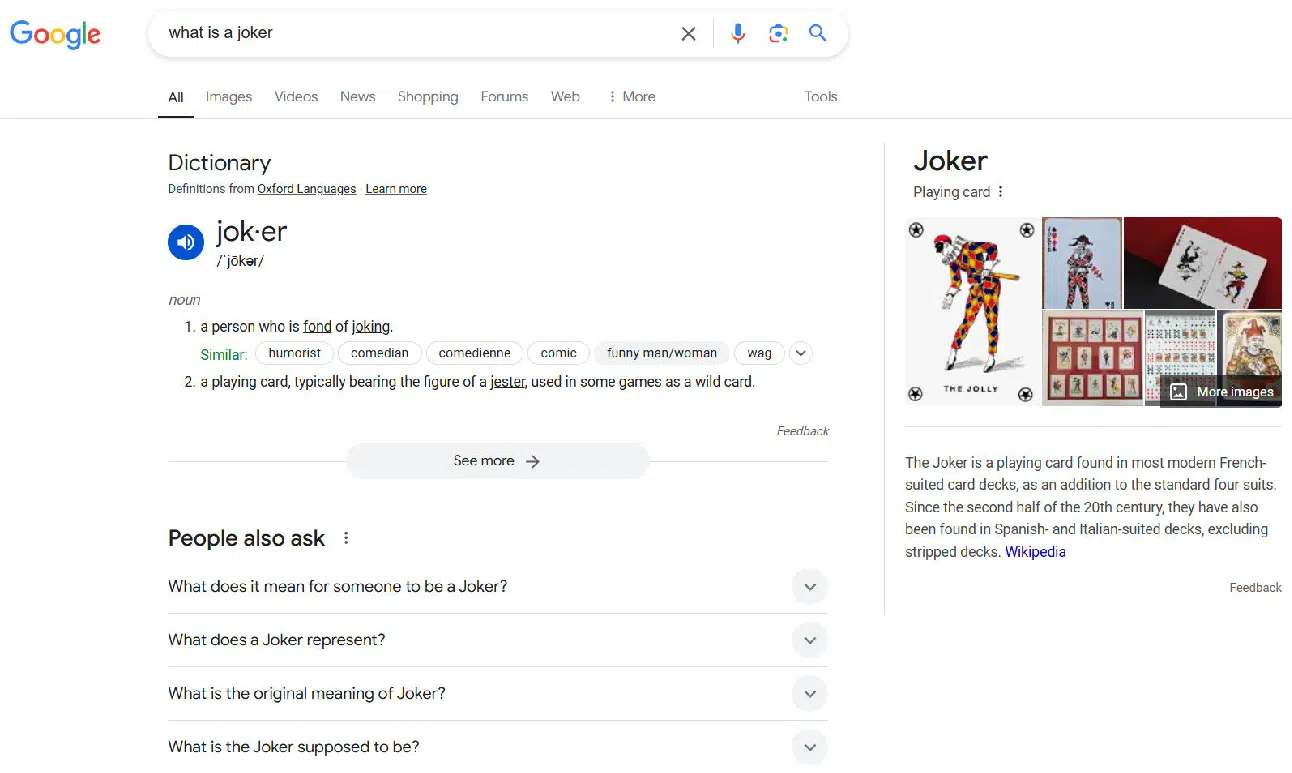
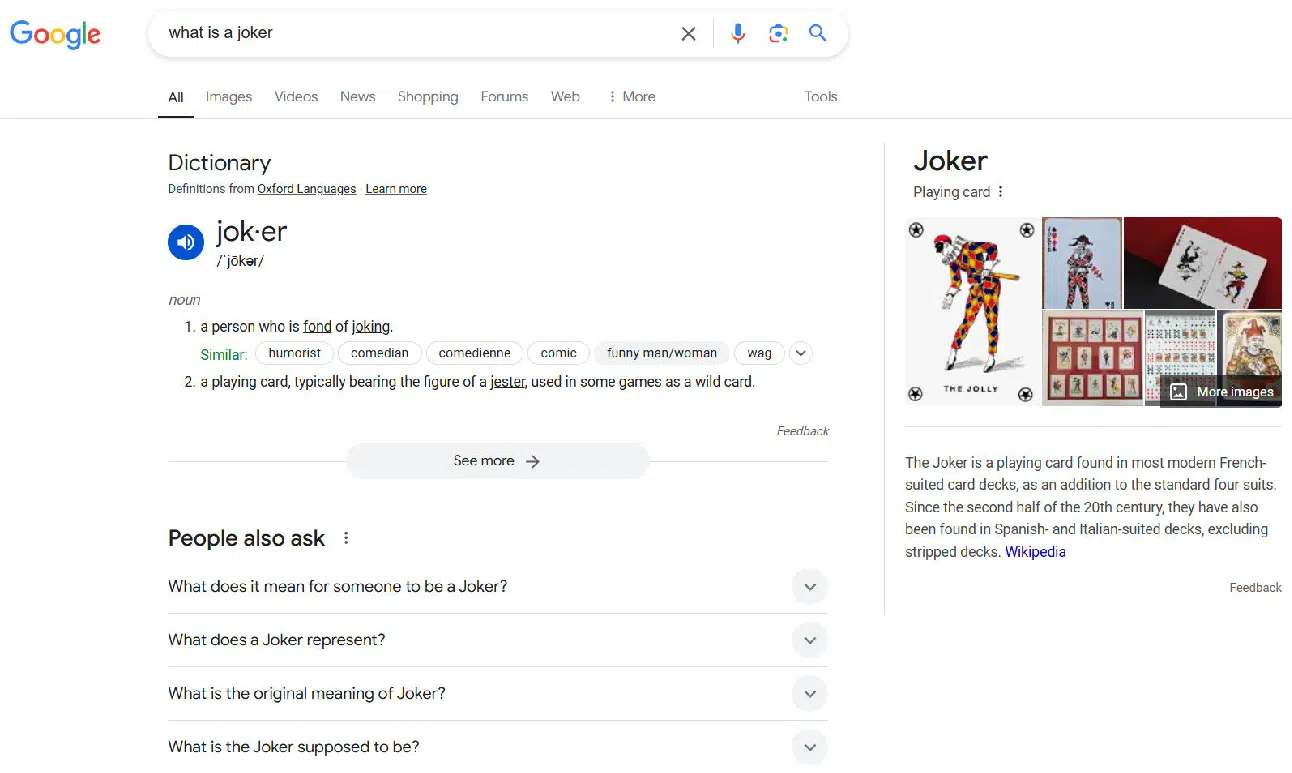
Google’s focus is on the playing card and a person who tells a lot of jokes.
Following this part of the SERP, Google continues this approach with websites focusing on these two definitions.
This means that someone who’s interested in the word “joker” as it applies to contract clauses will have to do an additional search to find what they were looking for (e.g., “meaning of joker when referring to contract clauses”).
Which is better?
Well, it depends.
If the searchers interested in playing cards or people who tell lots of jokes make up more than 90% of the people who enter this search query, then the Google result might be the better of the two.
As it is, I scored the ChatGPT search result a bit higher than Google’s for this query.
Another example of disambiguation failure is simply not addressing it at all. Consider the query example: “where is the best place to buy a router?”
Here is how ChatGPT search addressed it:
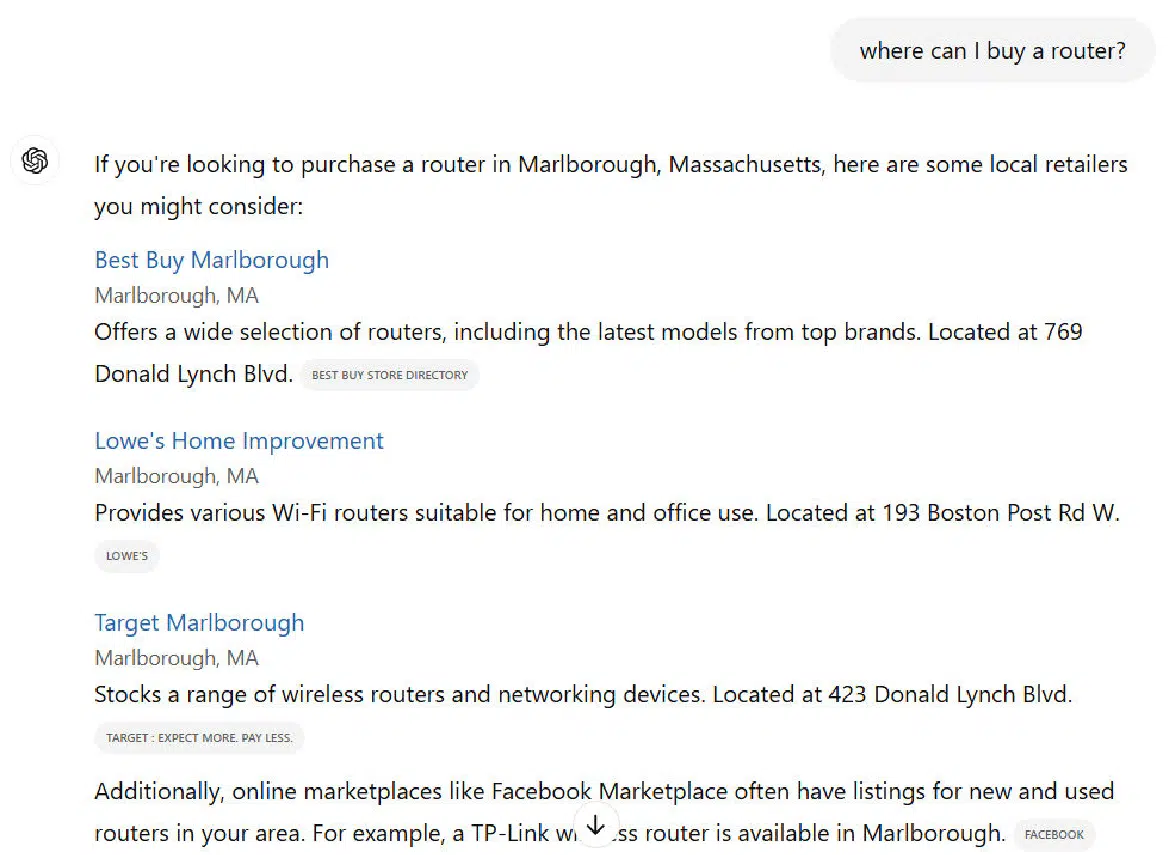
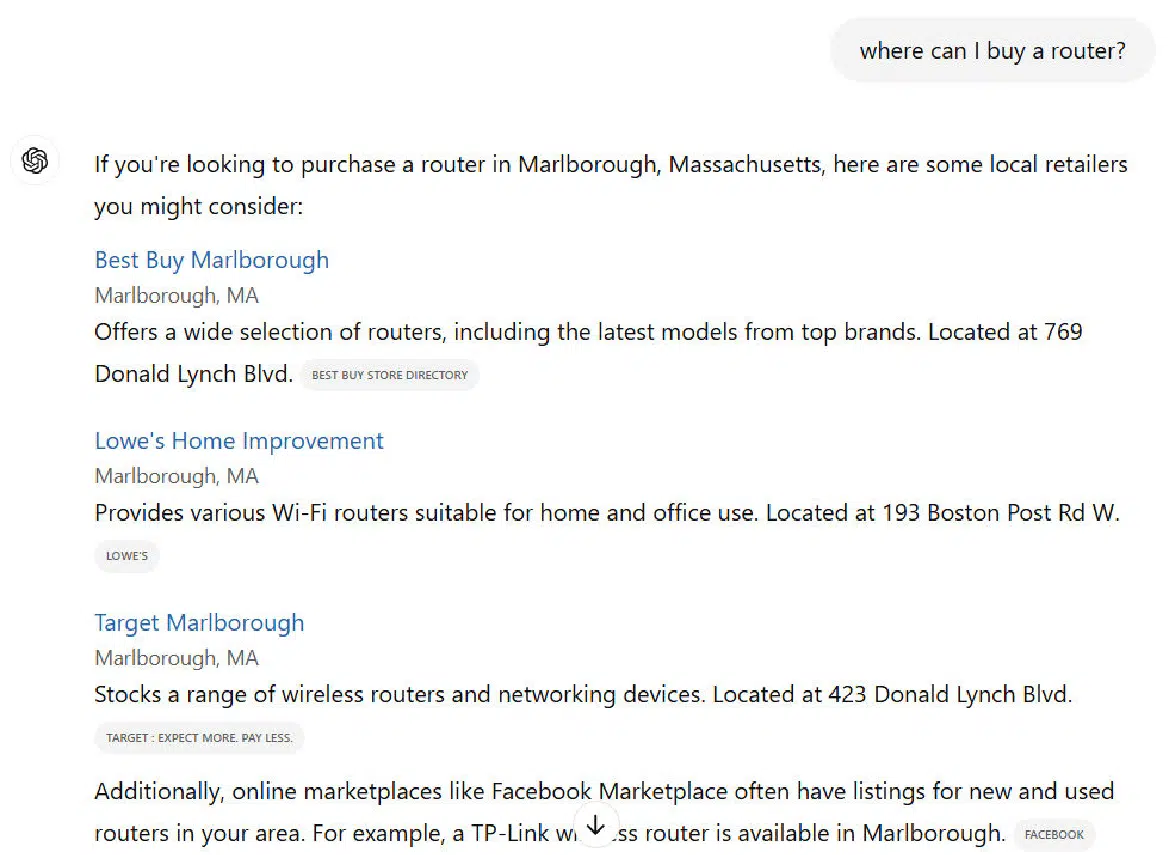
You might think this result is perfect, but routers also refer to a tool used in woodworking projects.
I use one frequently as a part of building furniture from scratch (true story).
There is a large enough audience of people who use these types of routers that I hope to see recognition of this in the SERPs.
Here is Google’s response to the query:
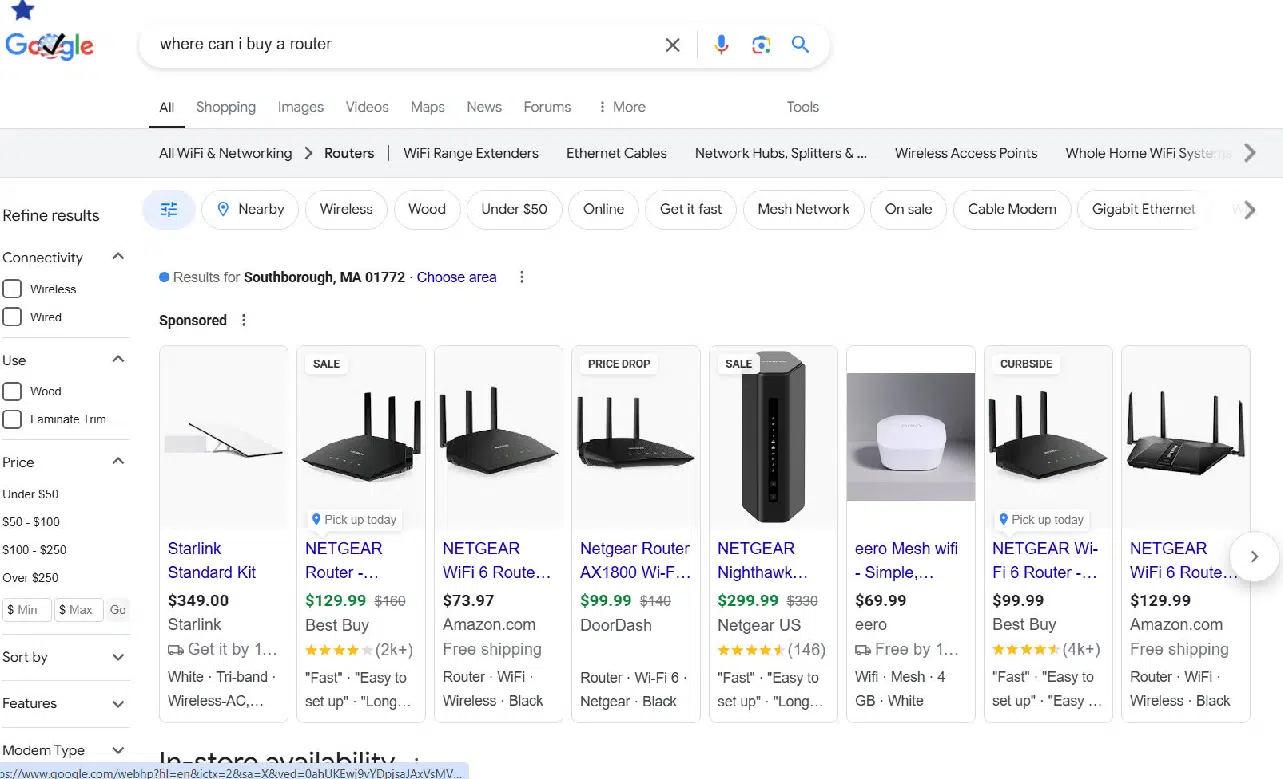
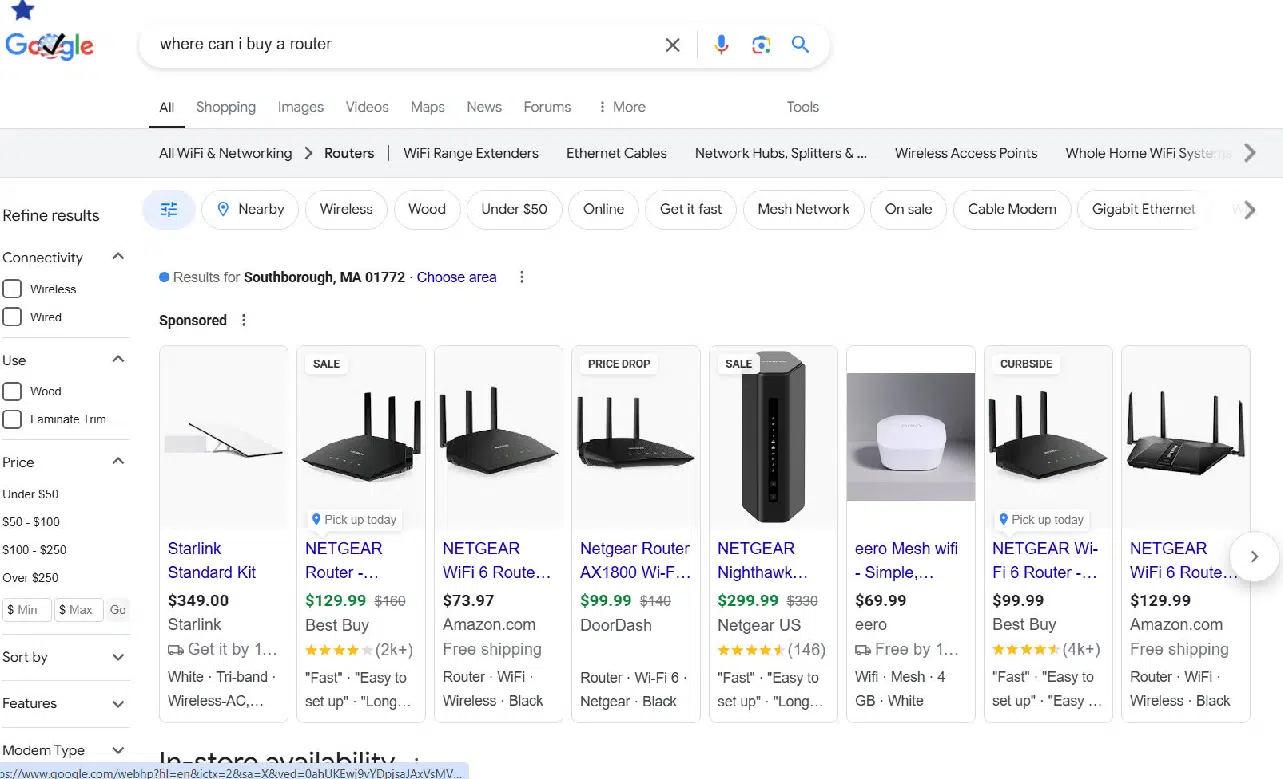
This part of the SERP is followed by:
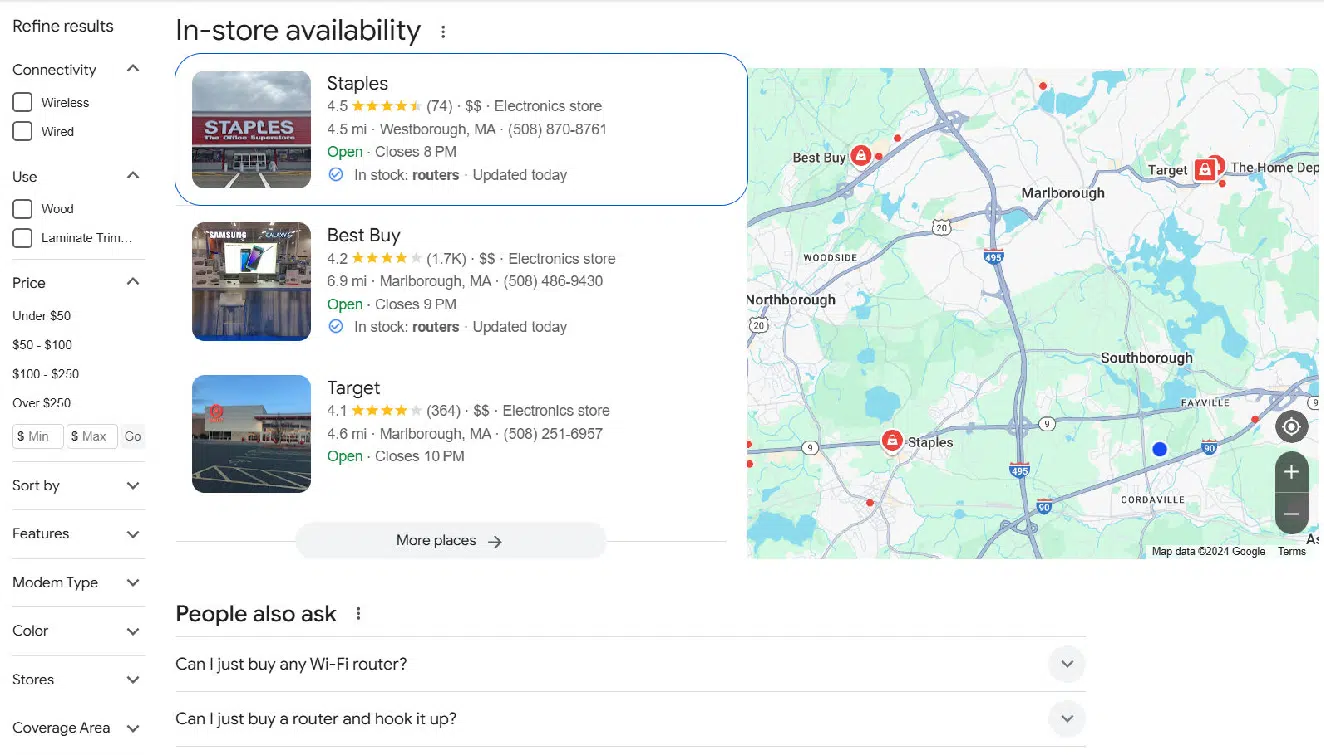
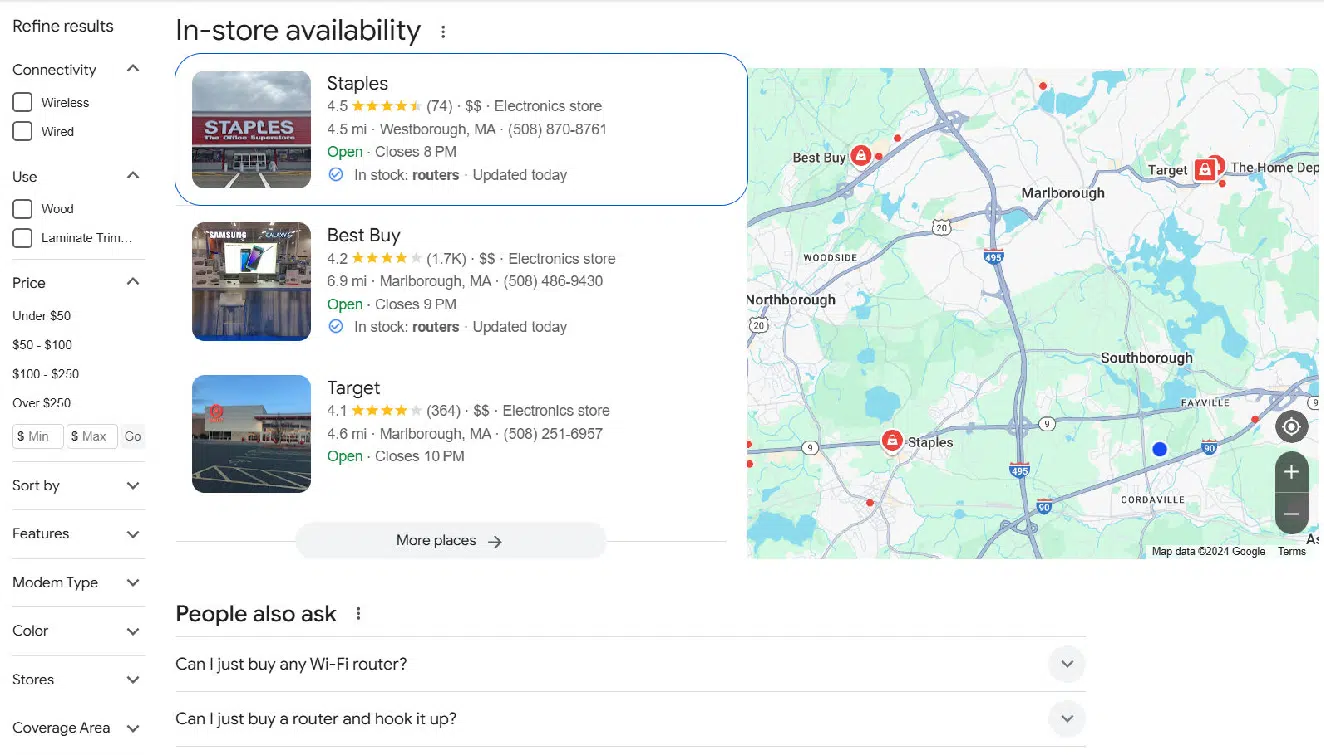
Google focuses on the internet router to the same degree as ChatGPT.
For this class of queries, I assigned these scores:
- ChatGPT search: 6.00.
- Google: 5.29.
Query type: Maintaining context in query sequences
Another interesting aspect of search is that users tend to enter queries in sequences.
Sometimes those query sequences contain much information that helps clarify their query intent.
An example query sequence is as follows:
- What is the best router to use for cutting a circular table top?
- Where can I buy a router?
As we’ve seen, the default assumption when people speak about routers is that they refer to devices for connecting devices to a single Internet source.
However, different types of devices, also called routers, are used in woodworking.
In the query sequence above, the reference to cutting a circular table should make it clear that the user’s interest is in the woodworking type of router.
ChatGPT’s response to the first query was to mention two specific models of routers and the general characteristics of different types of woodworking routers.
Then the response to “where can I buy a router” was a map with directions to Staples and the following content:
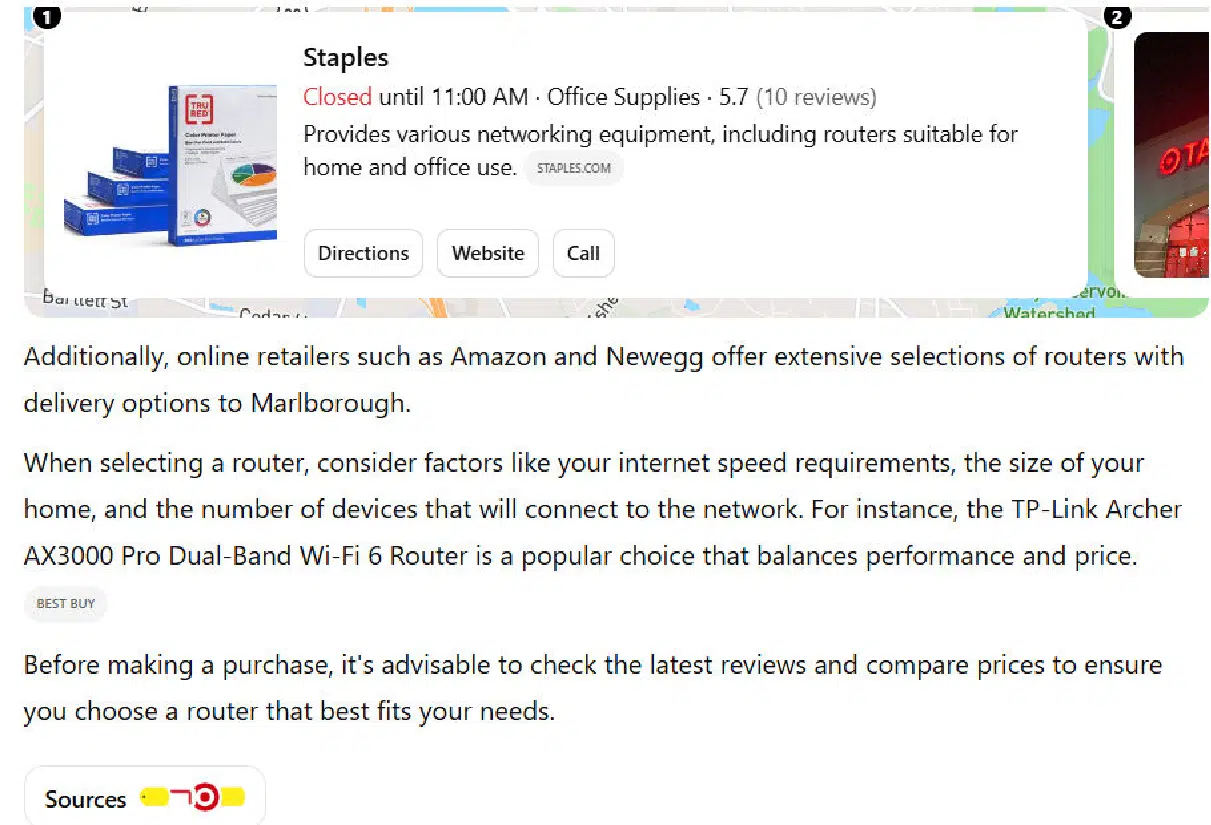
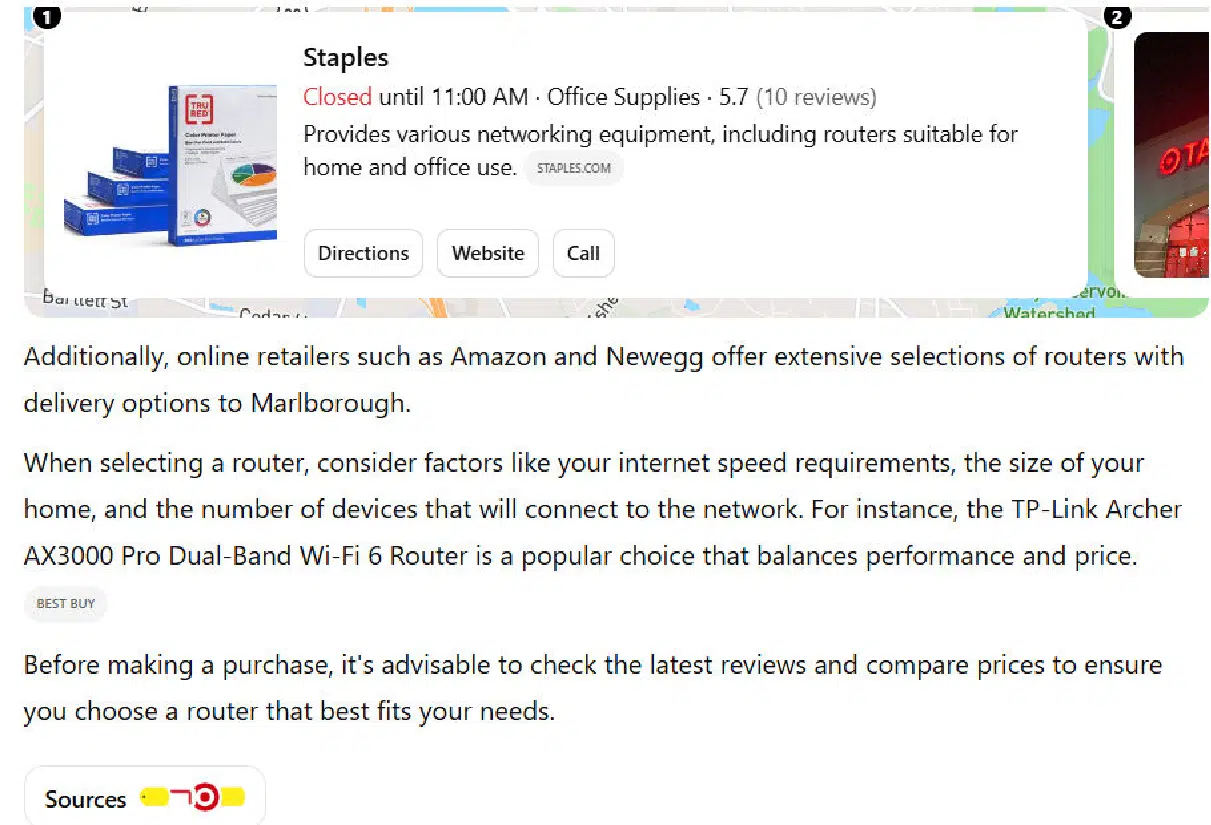
All of the context of the query was 100% lost.
Sadly, Google only performed slightly better.
It identified three locations, two of which were focused on networking routers and one which was focused on woodworking routers (Home Depot):
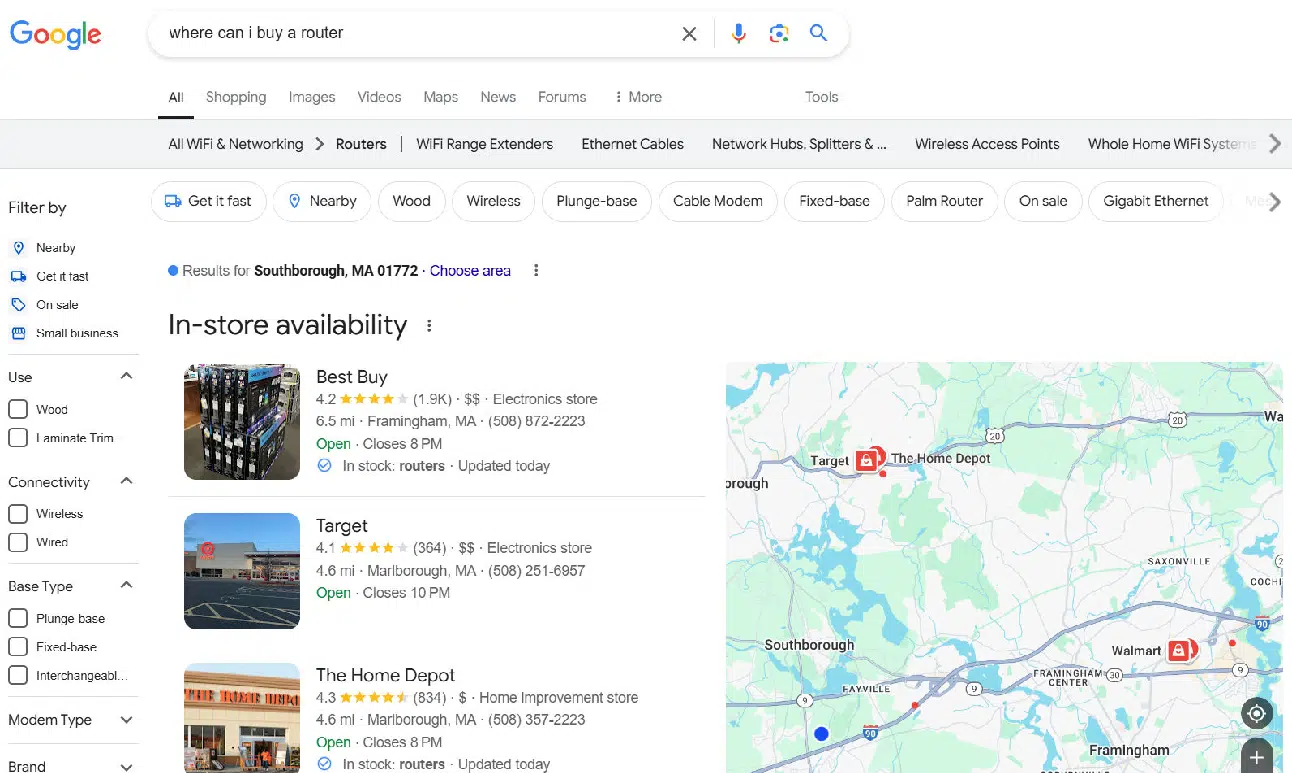
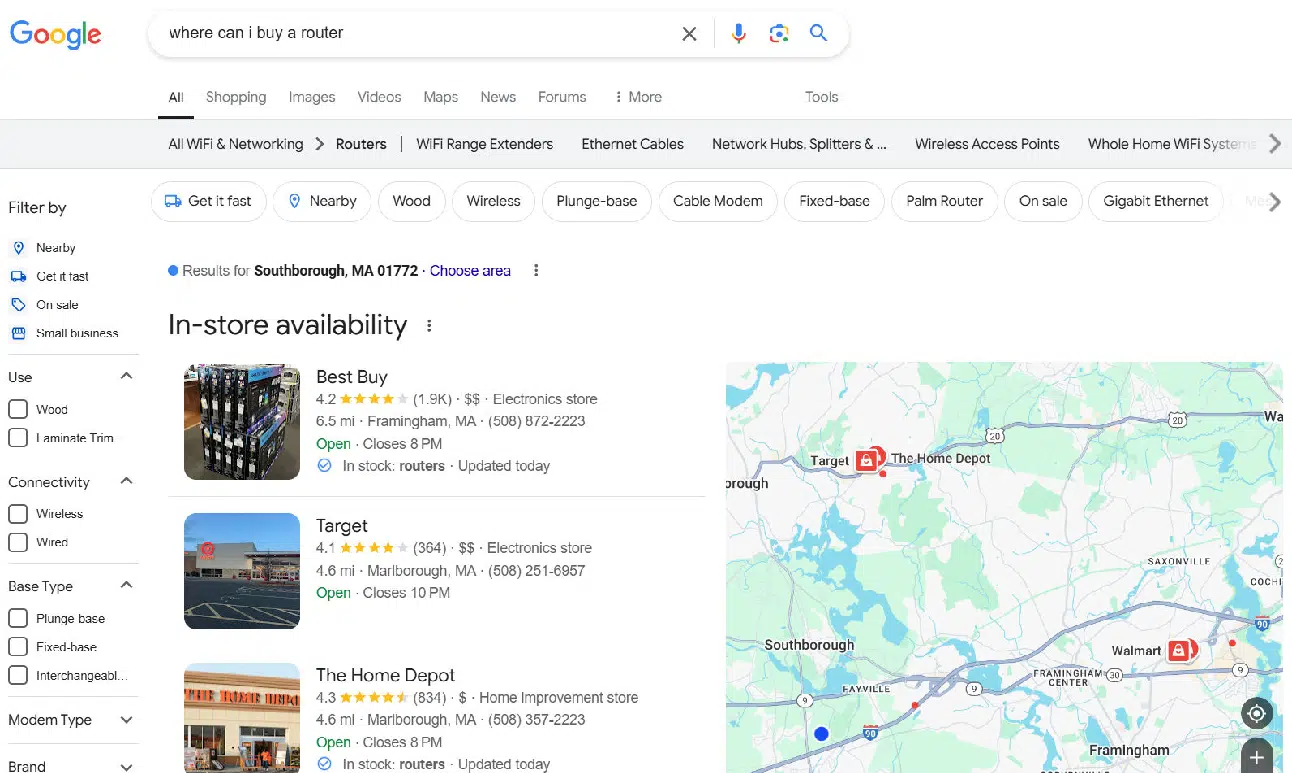
For this query, I scored the tools this way:
- ChatGPT search: 2.00.
- Google: 3.00.
Query type: Assumed typos
Another interesting example is queries where your search is relatively rare, yet it has a spelling that’s similar to another word.
For this issue, my search was: “Please discuss the history of the pinguin.”
The Pinguin was a commerce raider used by the German Navy in World War 2. It just has a spelling very similar to “penguin,” which is an aquatic flightless bird.
Both ChatGPT and Google simply assumed that I meant “penguin” and not “pinguin.”
Here is the result from ChatGPT:
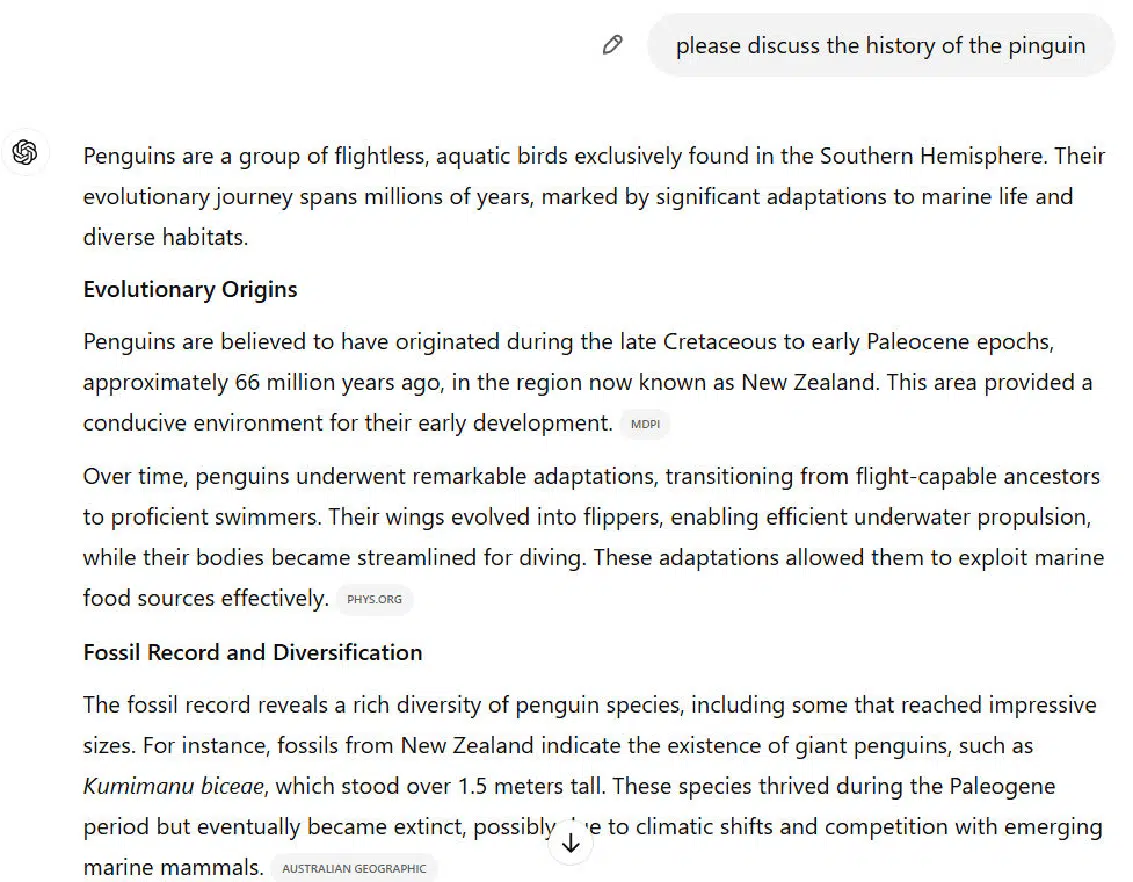
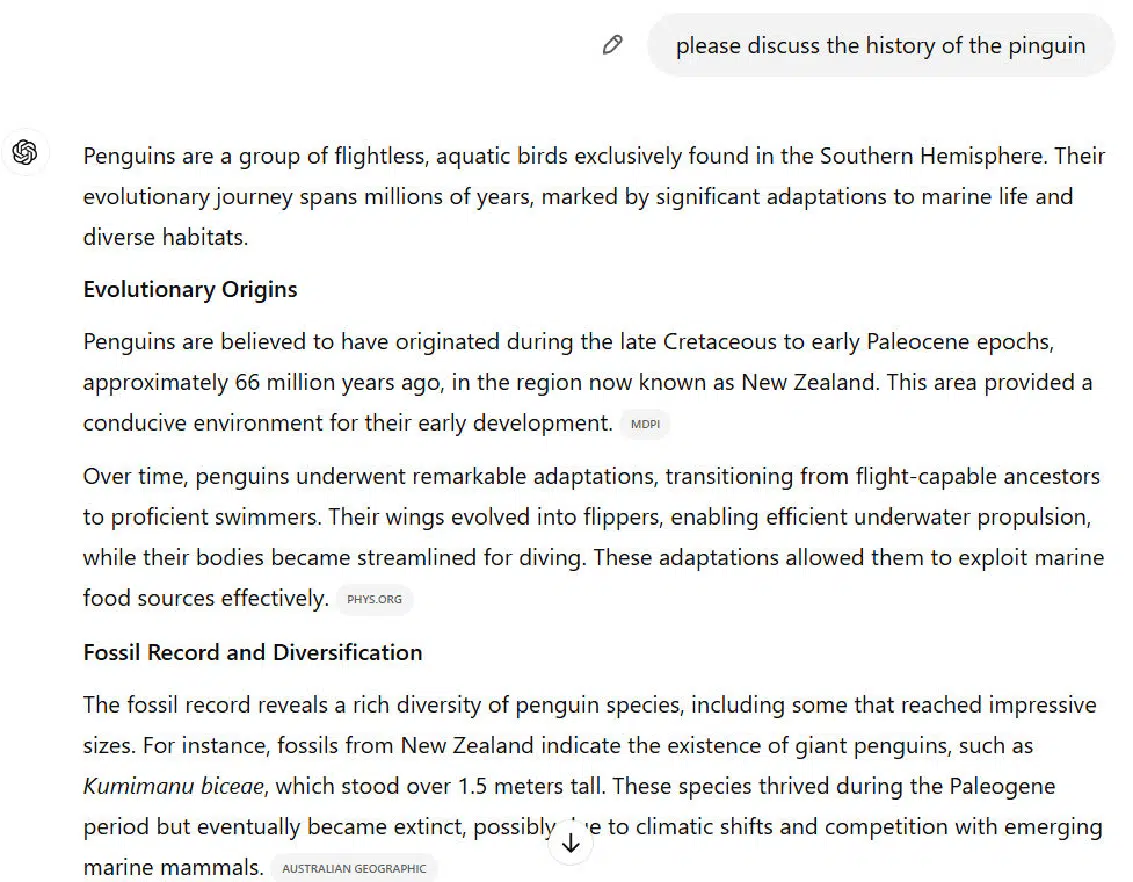
The result continues after what I’ve shown here but continues to focus on the bird, not the boat.
Google makes the same mistake:
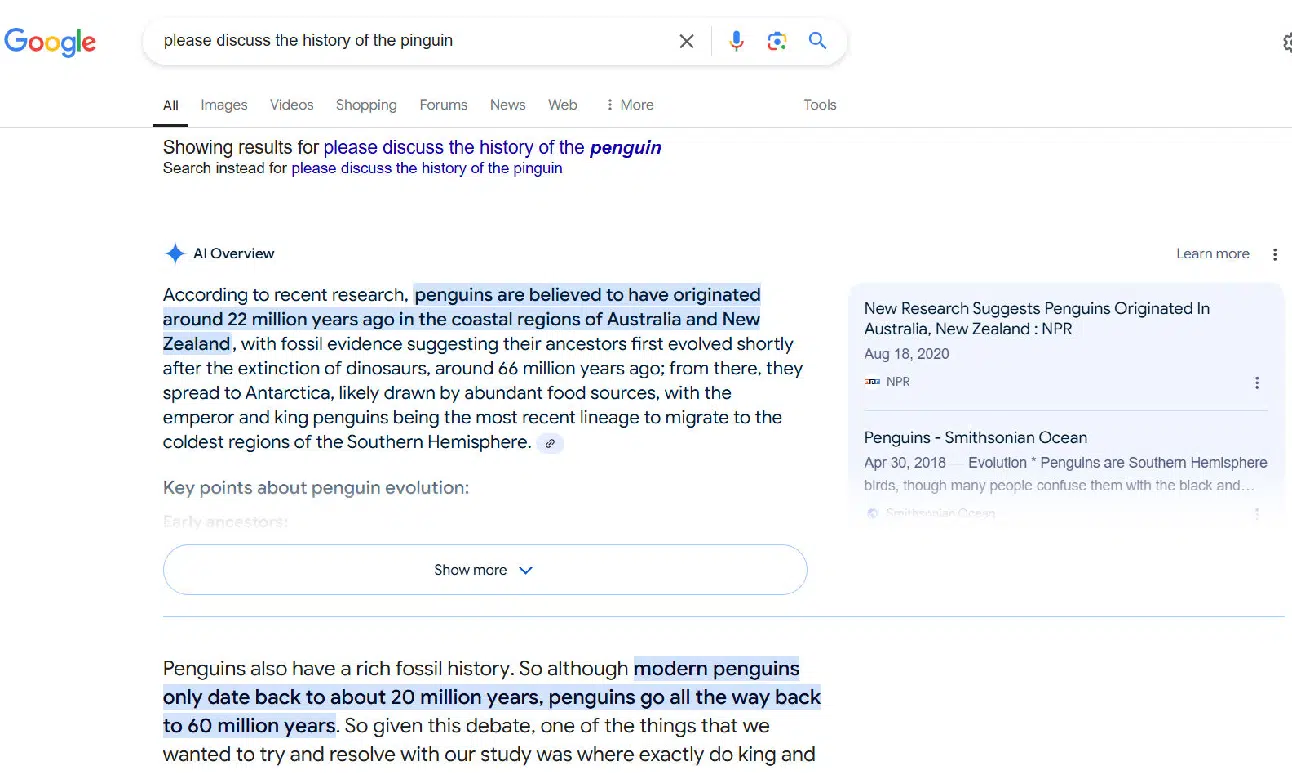
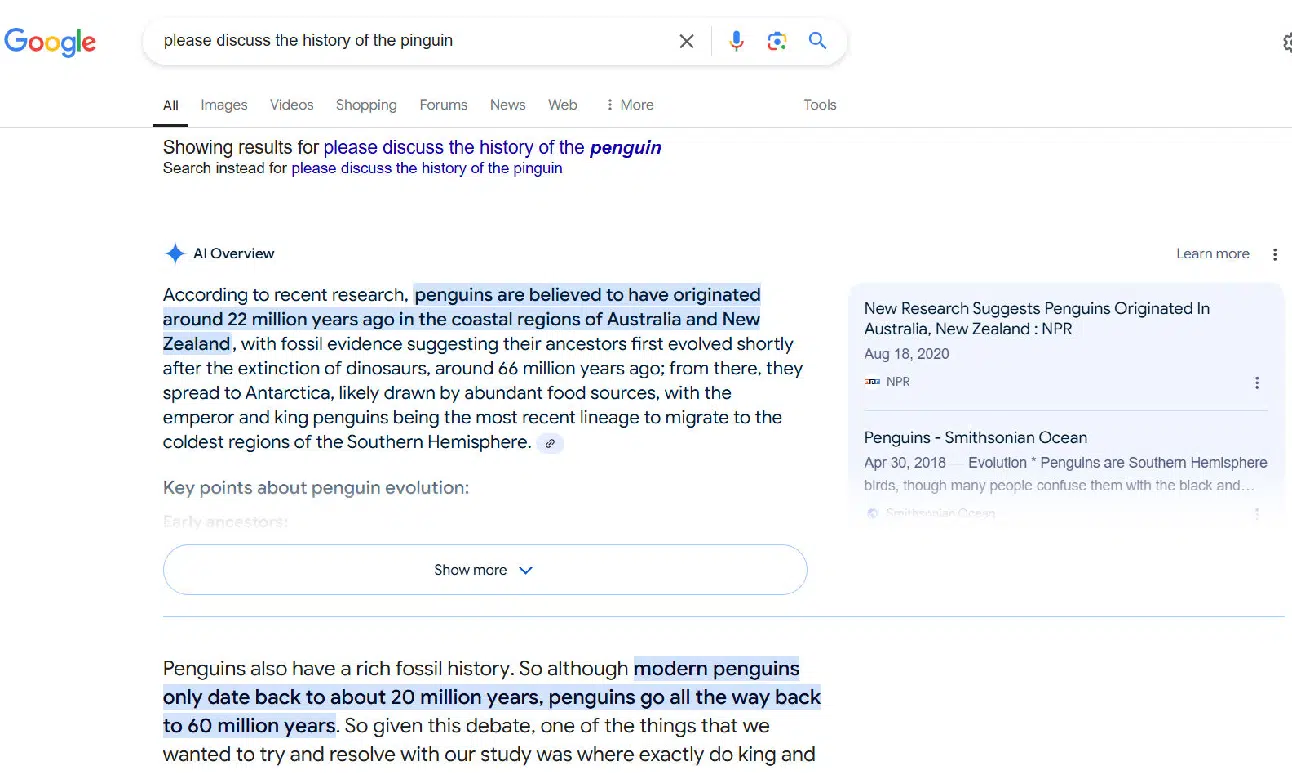
After the AI Overview and the featured snippet I’ve shown here, the SERPs continue to show more results focused on our flightless friends.
To be fair, I’ve referred to this as a mistake, but the reality is that the percentage of people who enter “pinguin” that simply misspelled “penguin” is probably far greater than those who actually mean the German Navy’s WW2 commerce raider.
However, you’ll notice that Google does one thing just a touch better than ChatGPT here.
At the top of the results, it acknowledges that it corrected “pinguin” to “penguin” and allows you to change it back.
The other way I addressed the problem was to do a second query: “Please discuss the history of the pinguin in WW2,” and both ChatGPT and Google gave results on the WW2 commerce raider.
For this query, I assigned these scores:
- ChatGPT search: 2.00.
- Google: 3.00.
Query type: Multiple options are a better experience
There are many queries where a single (even if it is well thought out) response is not what someone is probably looking for.
Consider, for example, a query like: “smoked salmon recipe.”
Even though the query is in the singular, there is little chance that anyone serious about cooking wants to see a single answer.
This type of searcher is looking for ideas and wants to look at several options before deciding what they want to do.
They may want to combine ideas from multiple recipes before they have what they want.
Let’s look at the response from ChatGPT search:
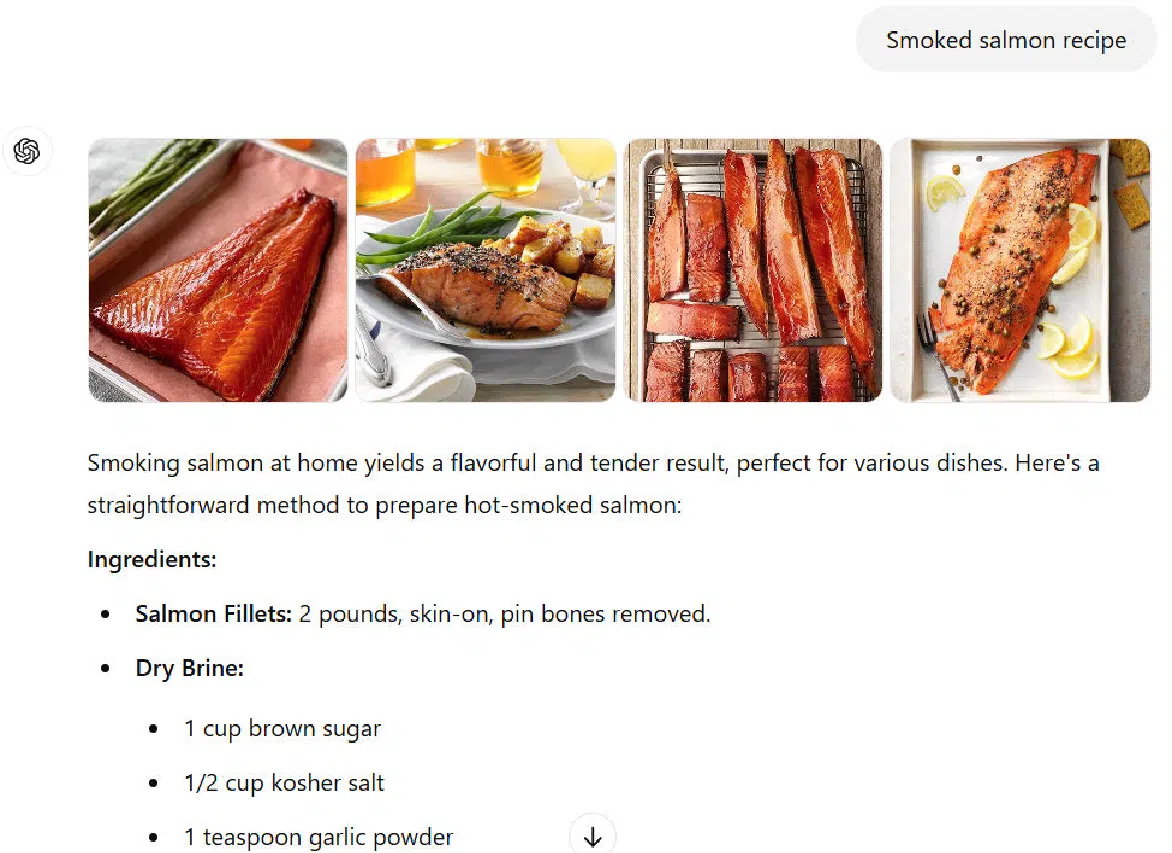
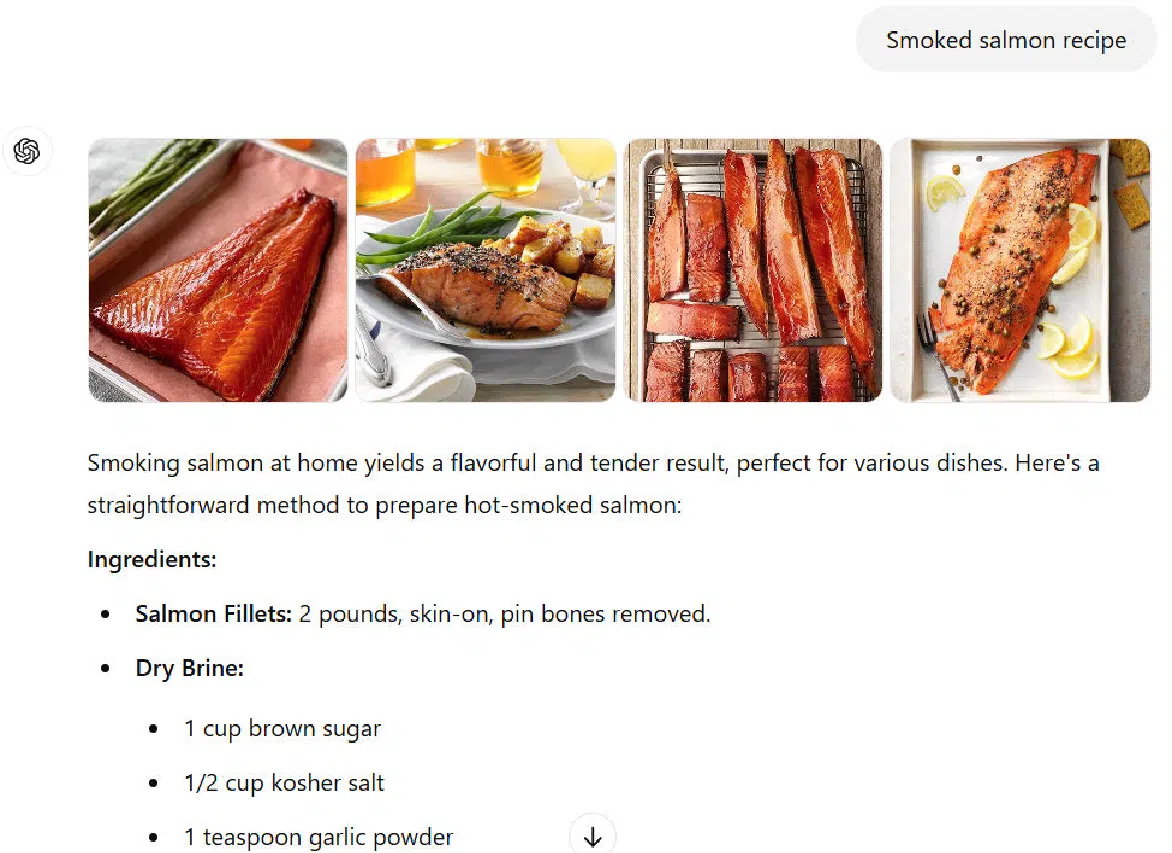
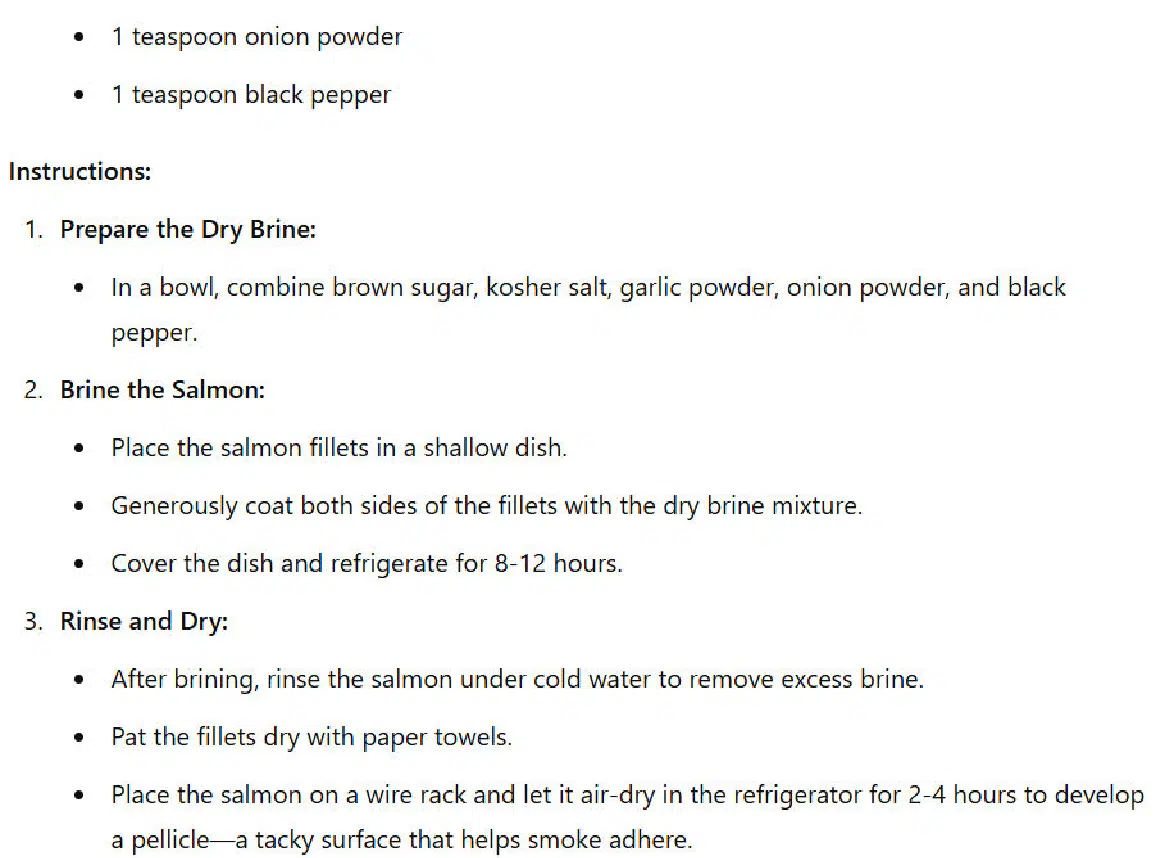
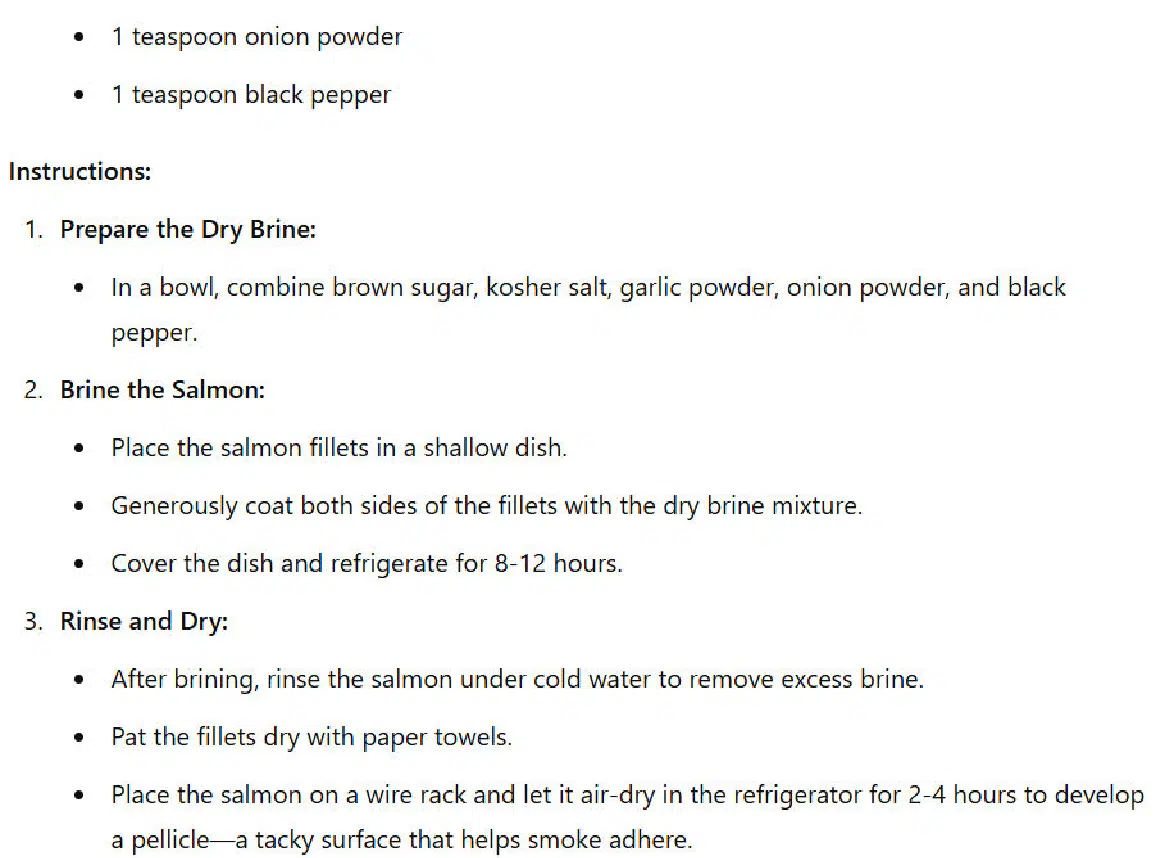
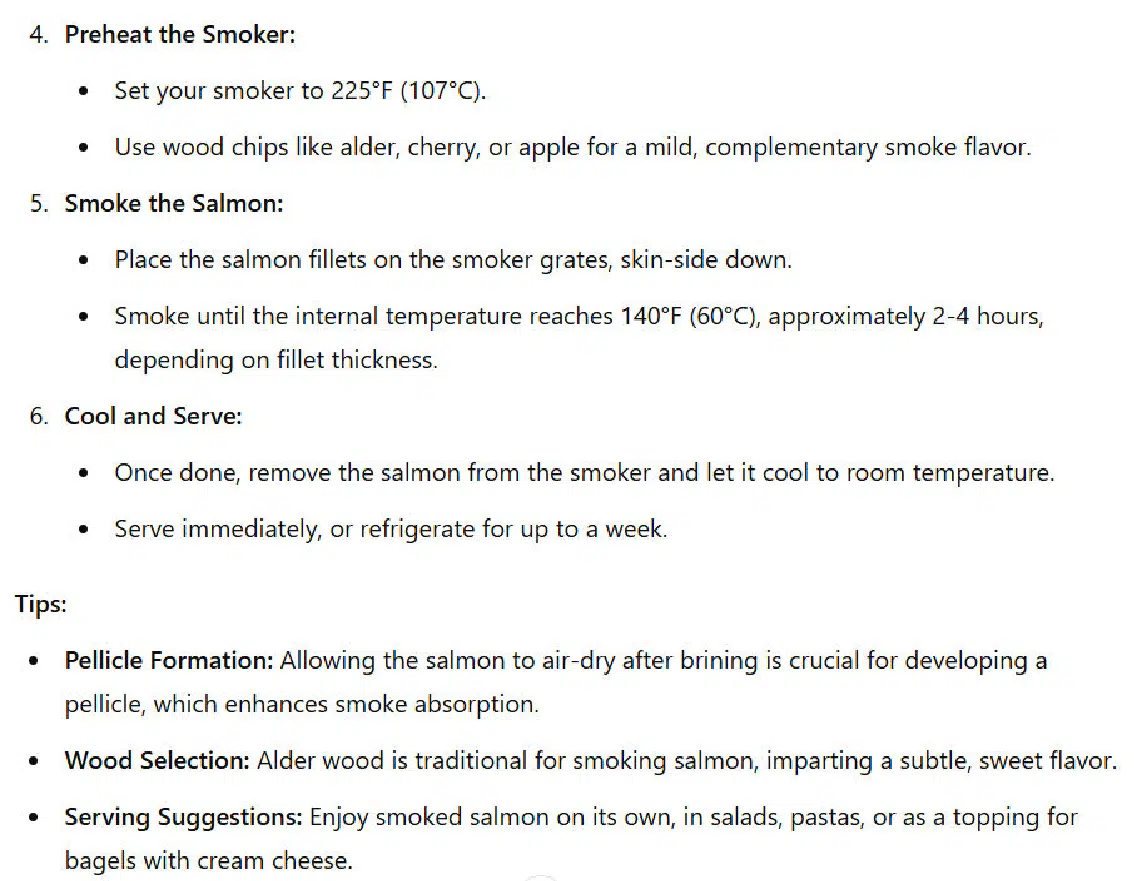
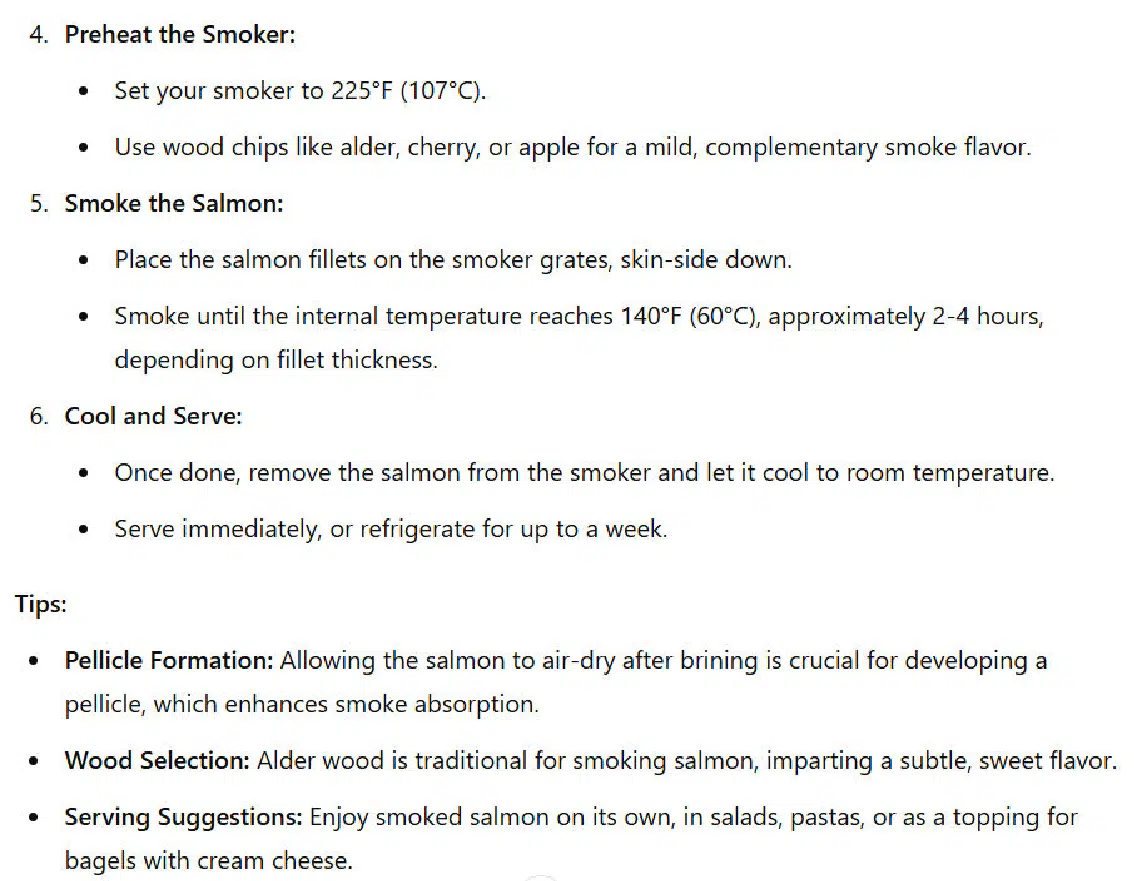
I’ve included the first three screens of the response (out of four), and here you will see that ChatGPT search provides one specific recipe from a site called Honest Food.
In addition, I see some things that don’t align with my experience.
For example, this write-up recommends cooking the salmon to 140 degrees. That’s already beginning to dry the salmon a bit.
From what I see on the Honest Food site, they suggest a range of possible temperatures starting from as low as 125.
In contrast, Google offers multiple recipes that you can access from the SERPs:
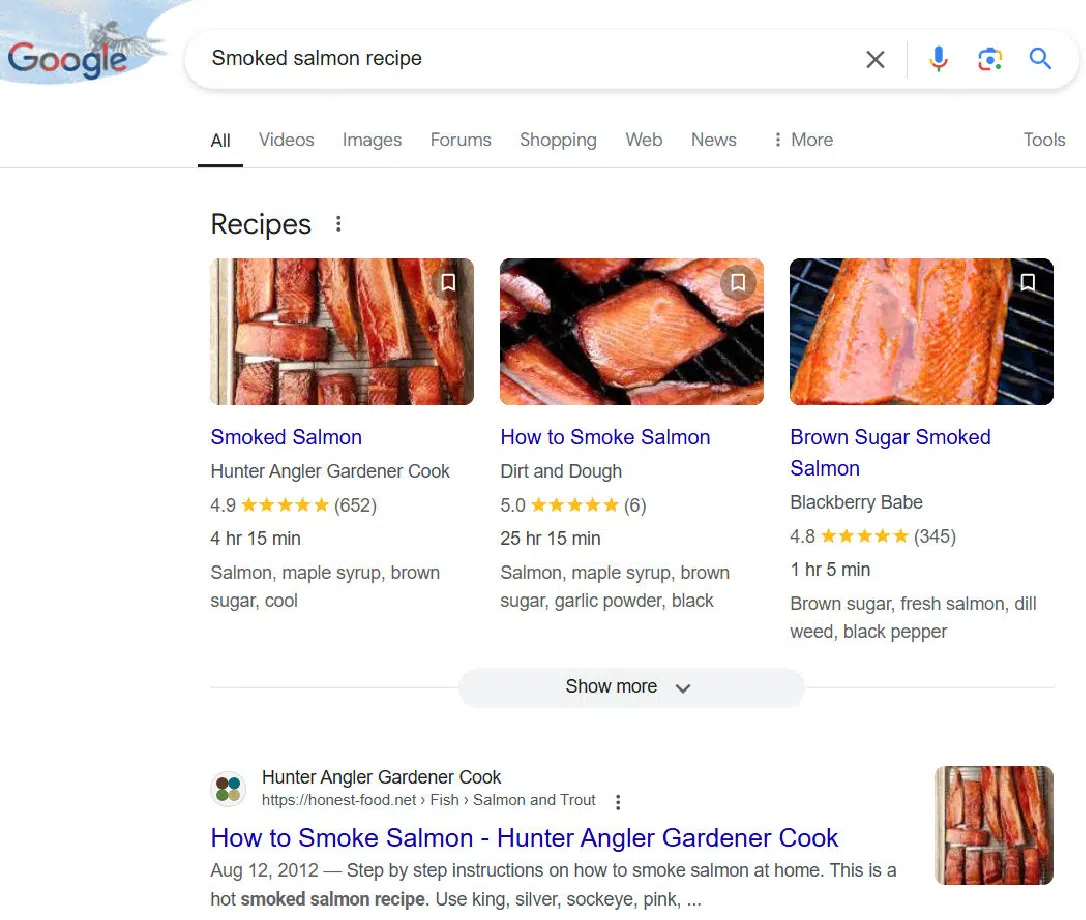
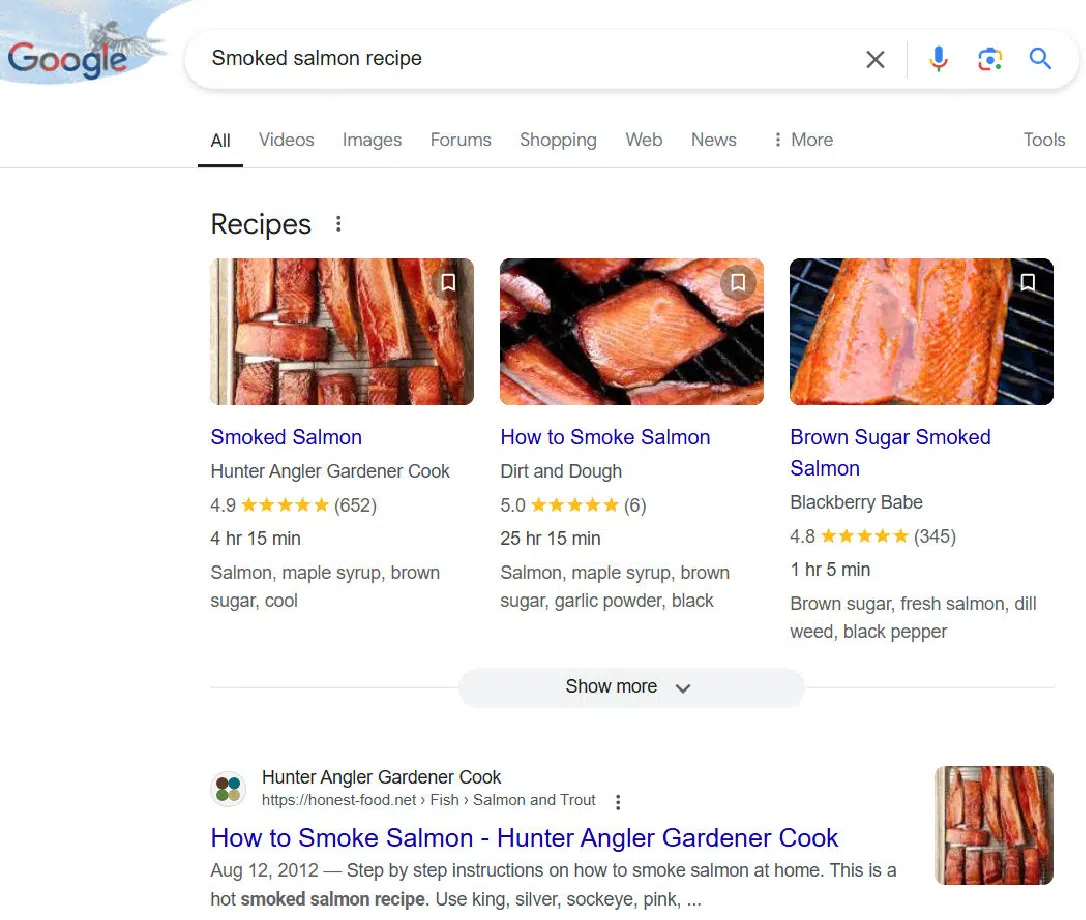
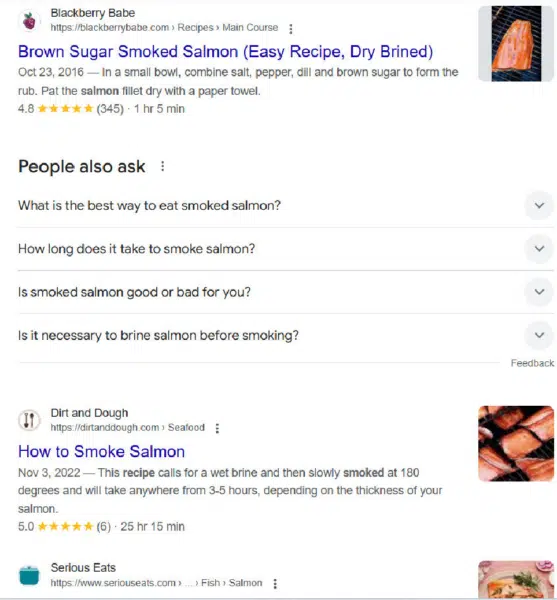
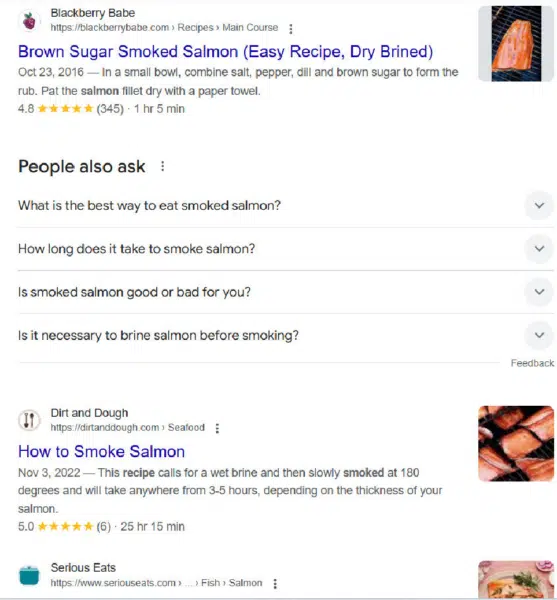
This is an example of a query that I scored in Google’s favor, as having multiple options is what I believe most searchers will want.
The scores I assigned were:
- ChatGPT search: 4.00.
- Google: 8.00.
Get the newsletter search marketers rely on.
Types of problems
Next, we’ll examine the types of things that can go wrong. I looked for these issues while scoring the results.
The analysis noted where problems that generative AI tools are known for were found and potential areas of weakness in Google’s SERPs.
These included:
- Errors.
- Omissions.
- Weaknesses.
- Incomplete coverage.
- Insufficient follow-on resources.
Problem type: Errors
This is what the industry refers to as “hallucinations,” meaning that the information provided is simply wrong.
Sometimes errors aren’t necessarily your money or your life situations, but they still give the user incorrect information.
Consider how ChatGPT search responds to a query asking about the NFL’s overtime rules:
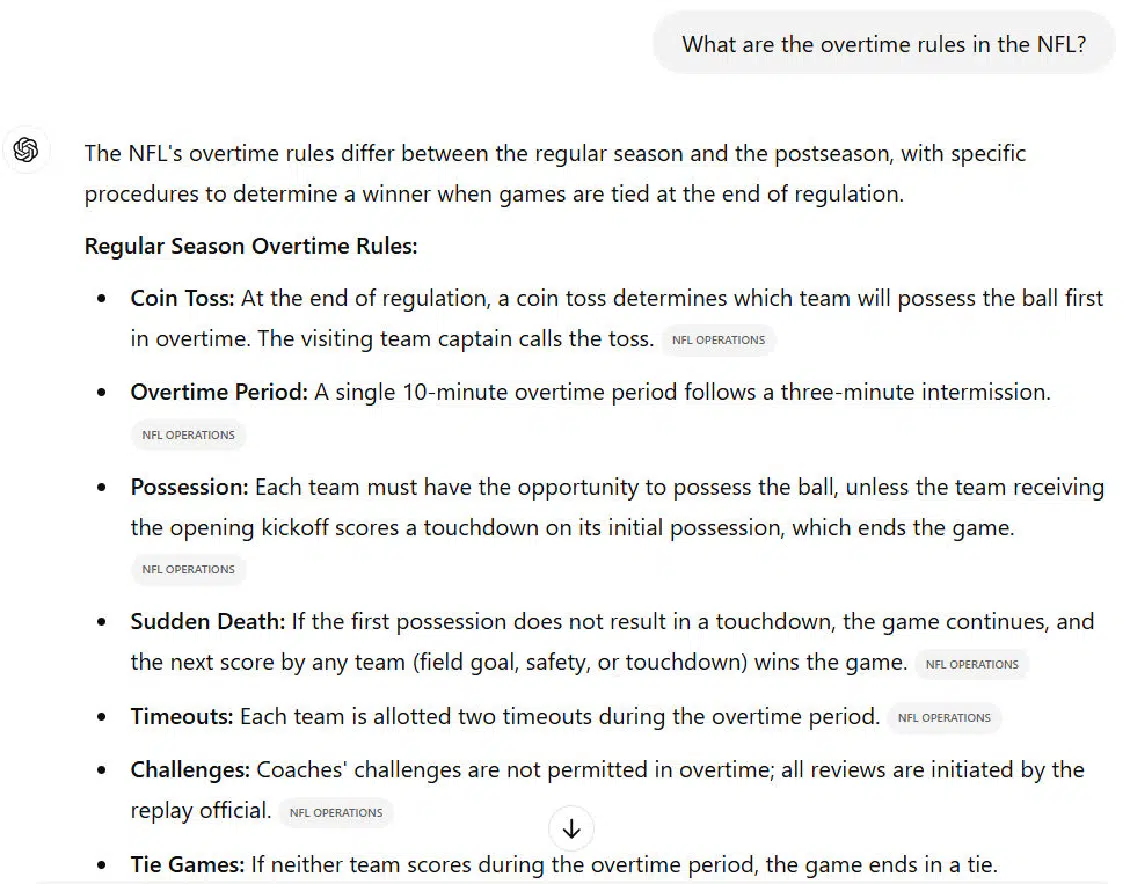
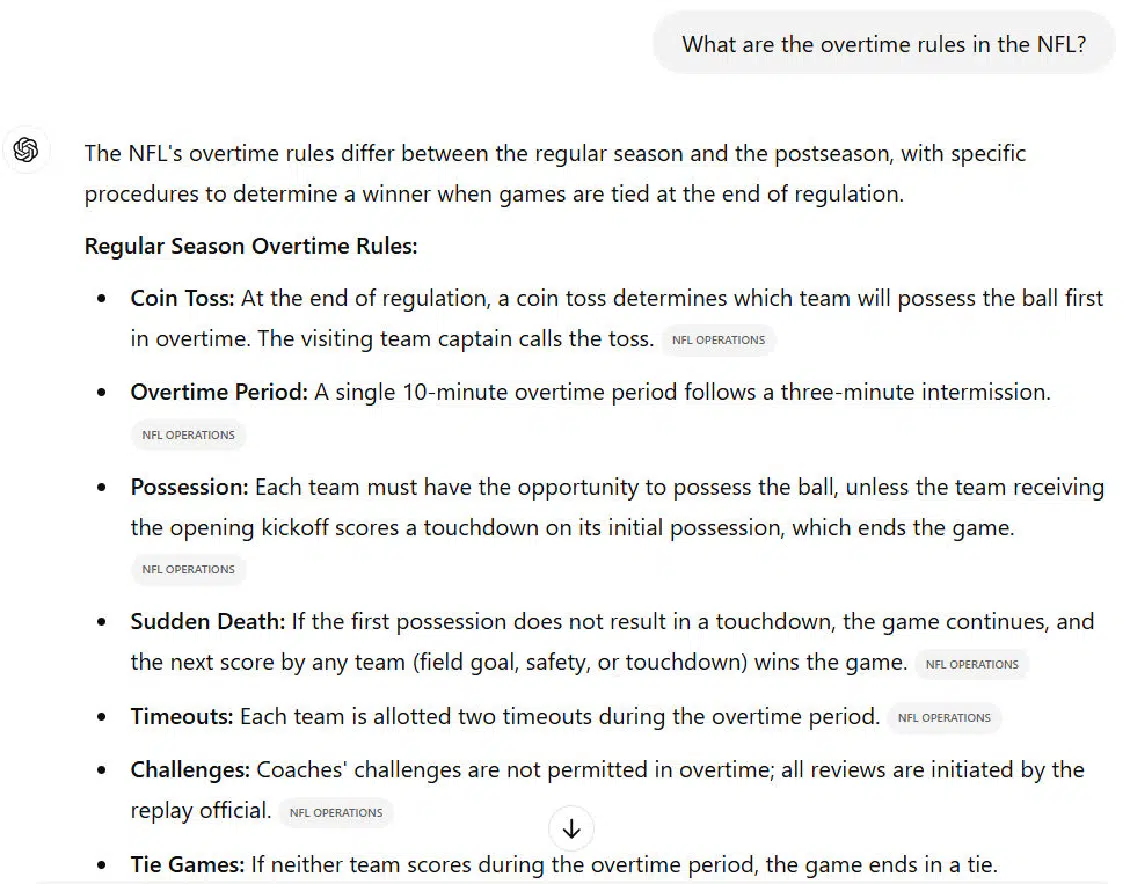
Notice the paragraph discussing how Sudden Death works. Unfortunately, it’s not correct.
It doesn’t account for when the first team that possesses the ball kicks a field goal, in which case they could win the game if the second team doesn’t score a field goal.
If the second team scores a field goal, this will tie the game.
In this event, it’s only after the field goal by the second team that the next score wins the game.
This nuance is missed by ChatGPT search.
Note: The information on the NFL Operations page that ChatGPT search used as a source is correct.
Google’s AI Overview also has an error in it:
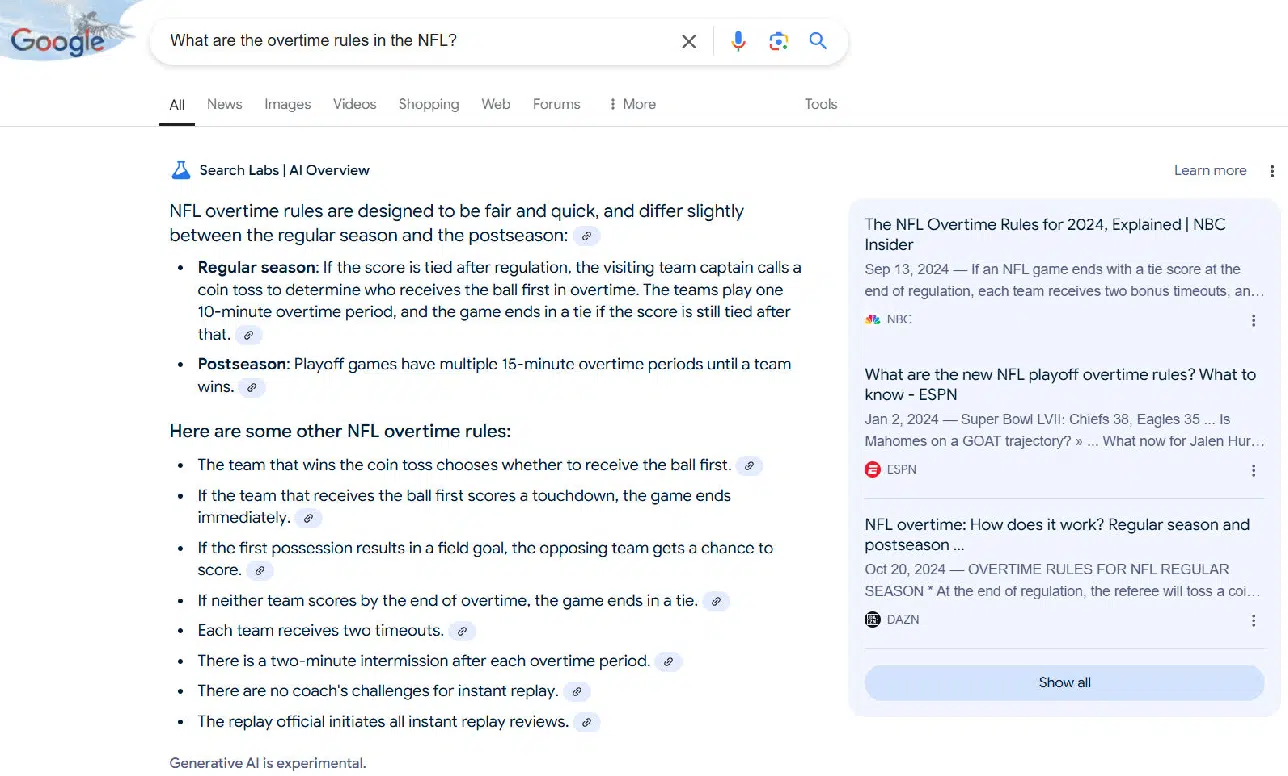
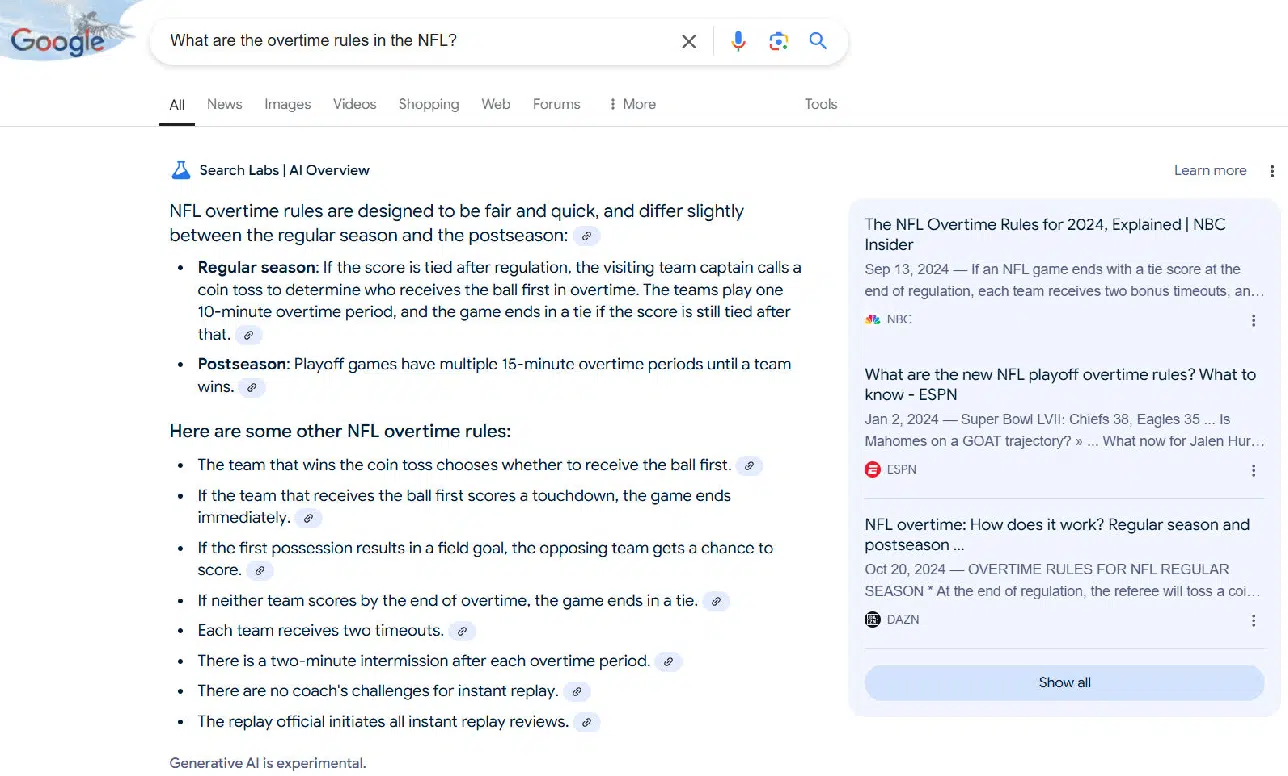
In the second line, where Google outlines “some other NFL overtime rules,” it notes that the same ends if the first team to possess the ball scores a touchdown.
This is true for regular season games but not true in the postseason, where both teams always get an opportunity to possess the ball.
Scores were as follows:
- ChatGPT search: 3.00.
- Google: 4.00.
Problem type: Omissions
This type of issue arises when important information that belongs in the response is left out.
Here is an example where ChatGPT search does this:
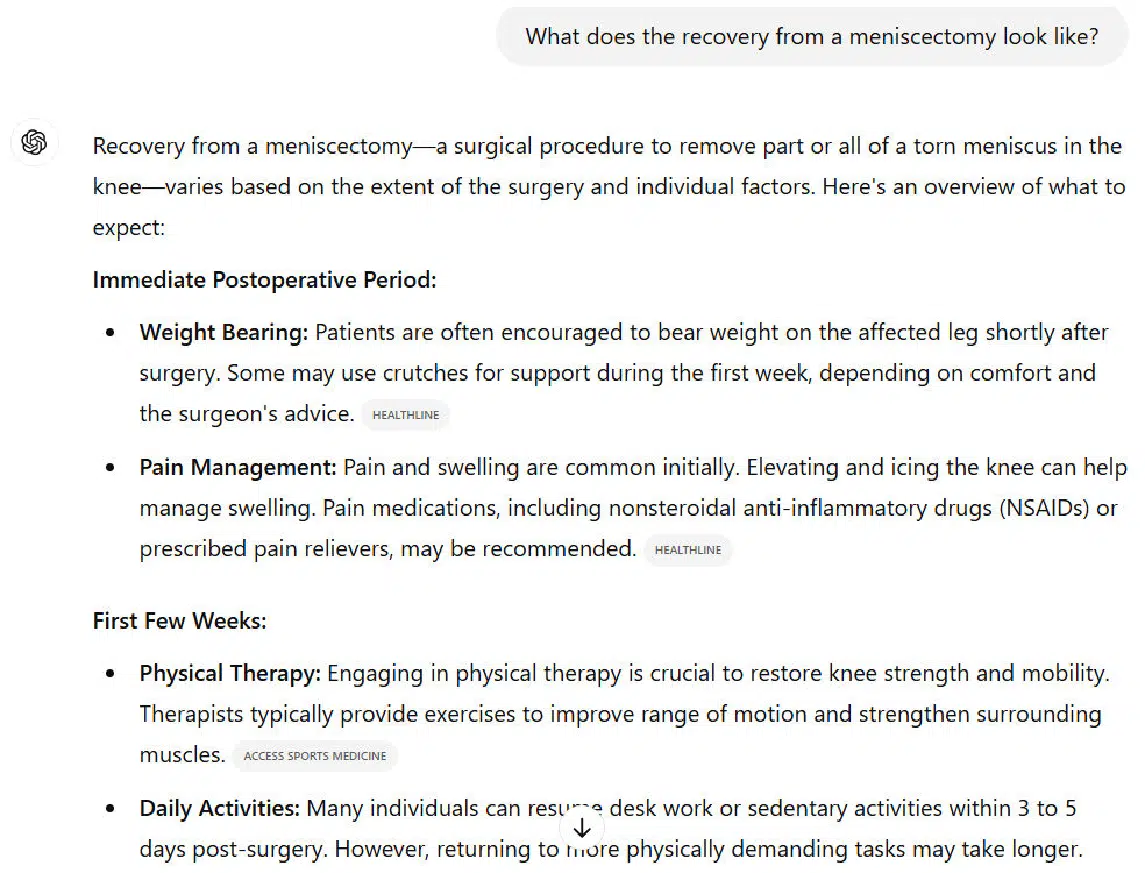
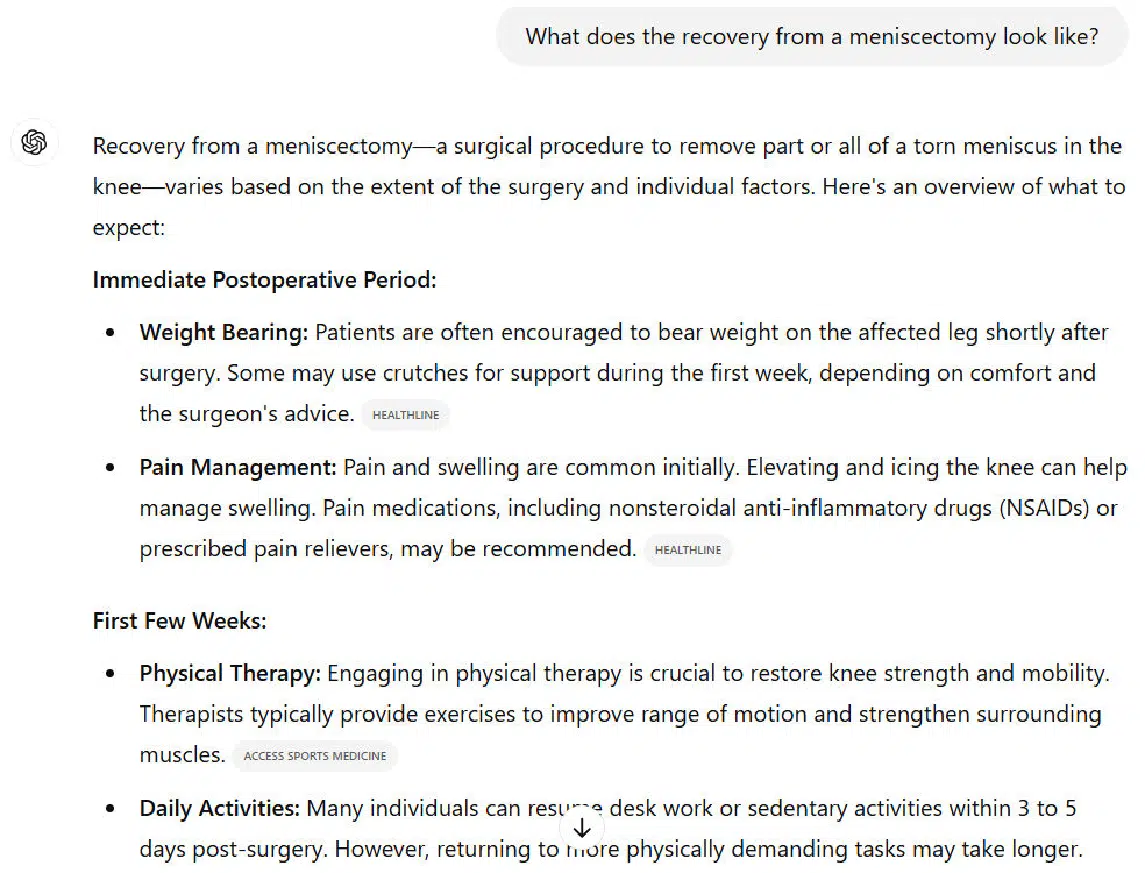
Under Pain Management, there is no mention of Tylenol as a part of a pain management regimen.
This is an unfortunate omission, as many people use only a mix of Tylenol and Ibuprofen to manage the pain after a meniscectomy.
Scores were as follows:
- ChatGPT search: 6.00.
- Google: 5.00.
Problem type: Weaknesses
I used weaknesses to cover cases where aspects of the result could have been more helpful to the searcher but where the identified issue couldn’t properly be called an error or omission.
Here is an example of an AI Overview that illustrates this:
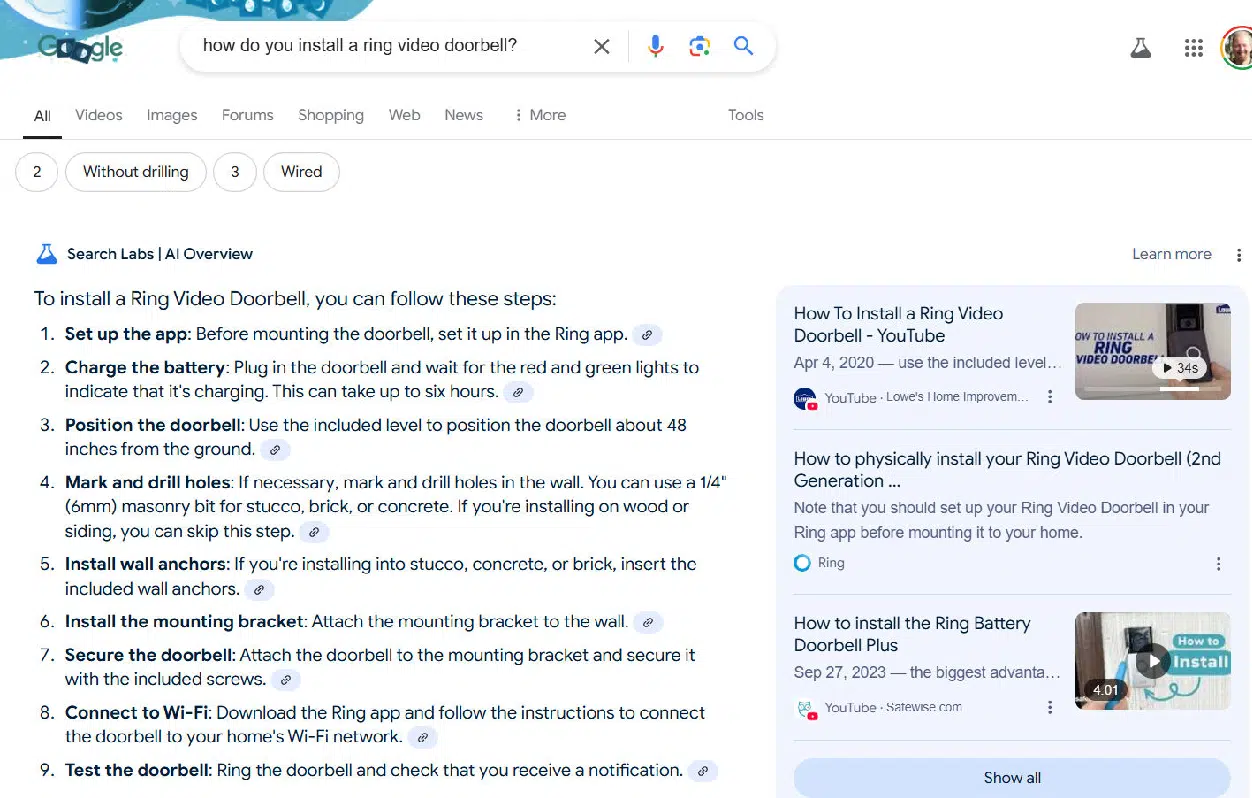
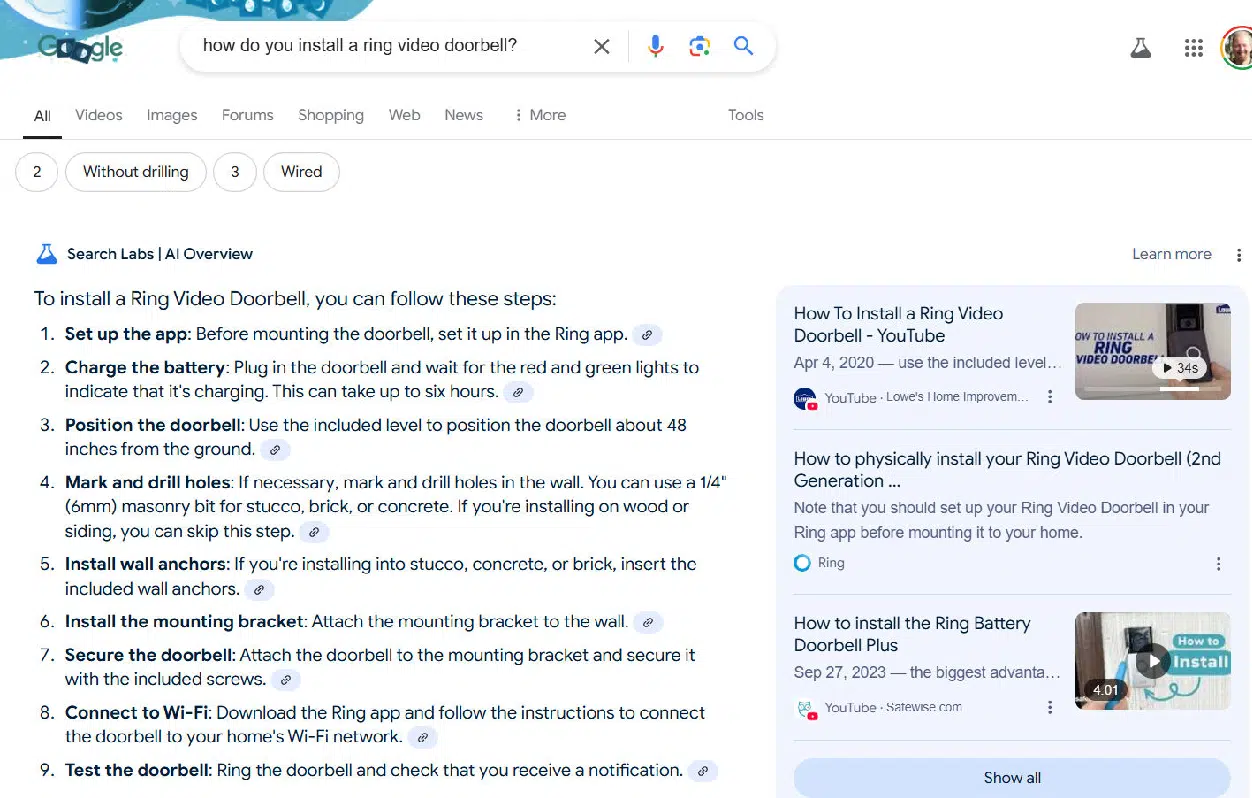
The weakness of this outline is that it makes the most sense to charge the battery as the first step.
Since it takes up to 6 hours to complet,e it’s not that useful to set up the app before completing this step.
Here is how I scored these two responses:
- ChatGPT search: 3.00.
- Google: 5.00.
Problem type: Incomplete coverage
This category is one that I used to identify results that failed to cover a significant user need for a query.
Note that “significant” is subjective, but I tried to use this only when many users would need a second query to get what they were looking for.
Here is an example of this from a Google SERP.
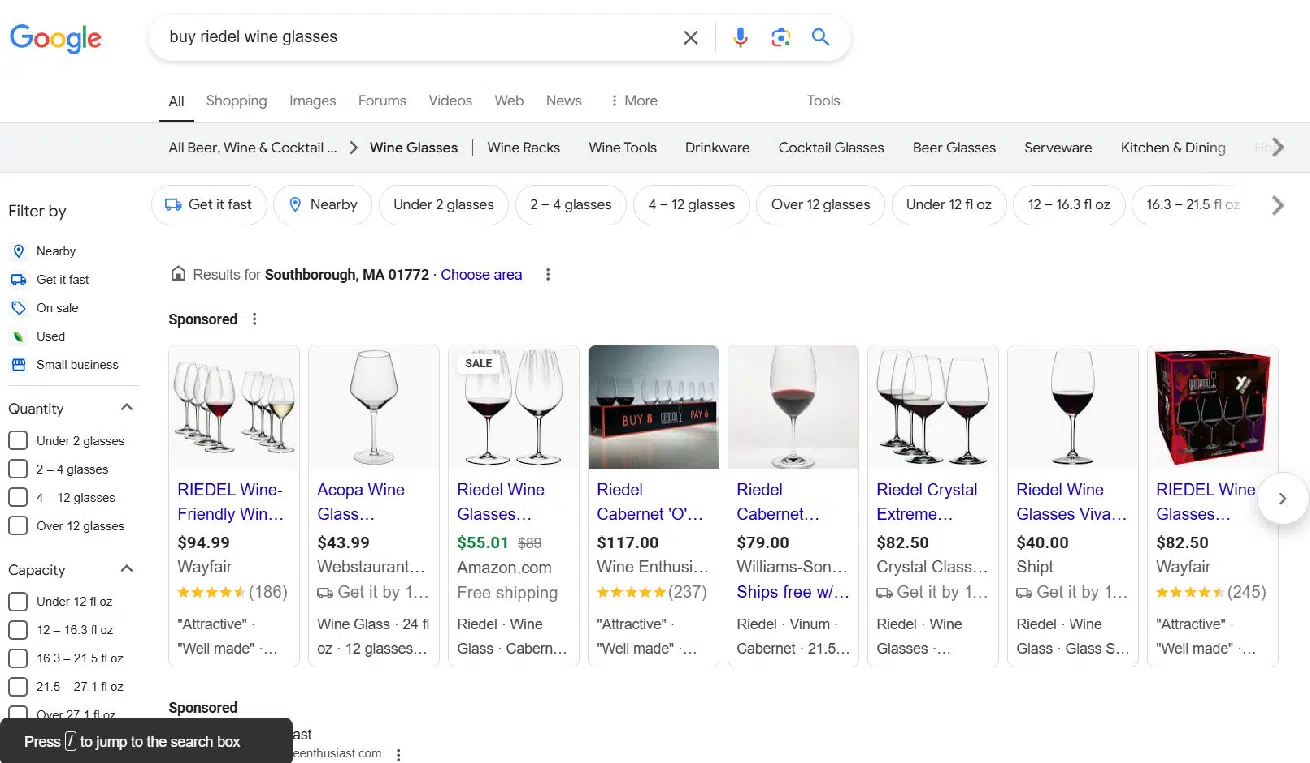
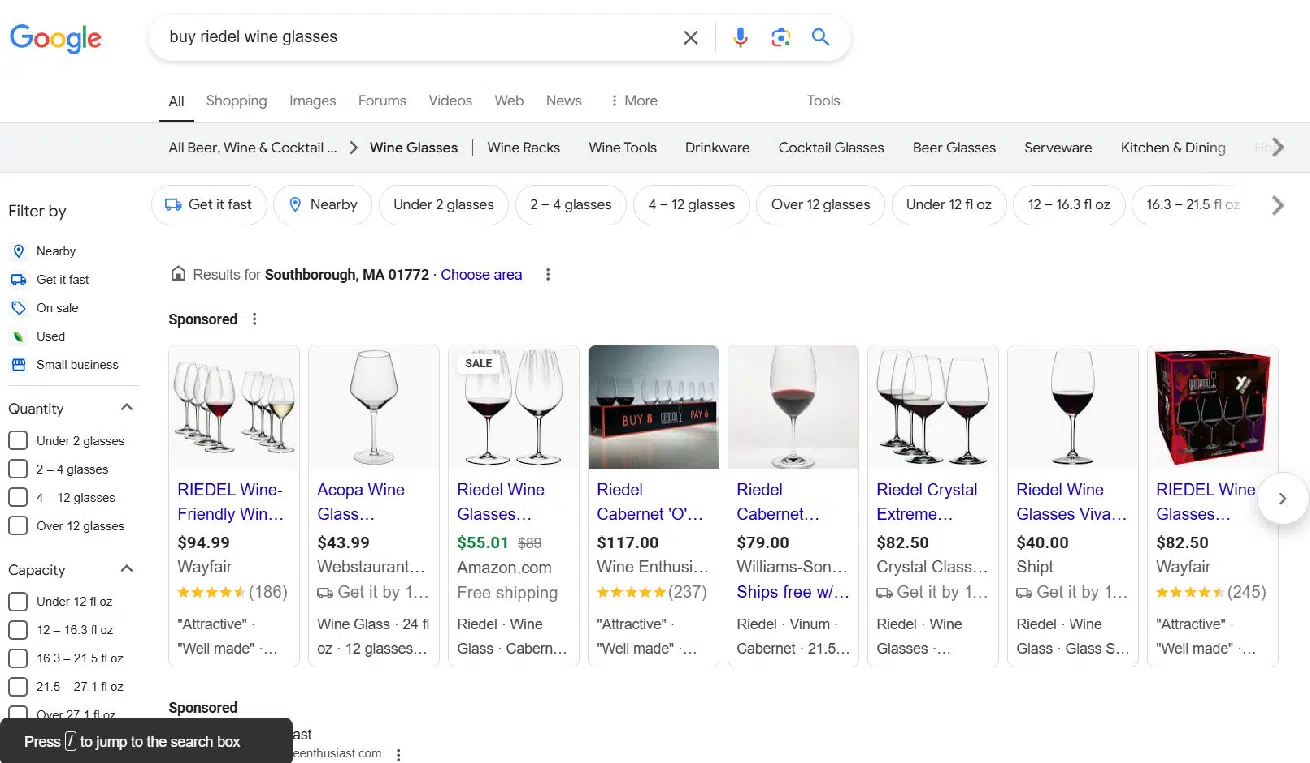
The results are dominated by Google Shopping (as shown above).
Below what I’ve shown, Google has two ads offering online buying opportunities and two pages from the Riedl website.
This result will leave a user who needs the glasses today and therefore wants to shop locally without an answer to their question.
ChatGPT search did a better job with this query as it listed both local retailers and online shopping sites.
Scores for this query:
- ChatGPT search: 6.00.
- Google: 4.00.
Problem type: Insufficient follow-on resources
As discussed in “How query sessions work” earlier in this article, it’s quite common that users will try a series of queries to get all the information they’re looking for.
As a result, a great search experience will facilitate that process.
This means providing a diverse set of resources that makes it easy for users to research and find what they want/need. When these aren’t easily accessed it offers them a poor experience.
As an example, let’s look at how ChatGPT search responds to the query “hotels in San Diego”:
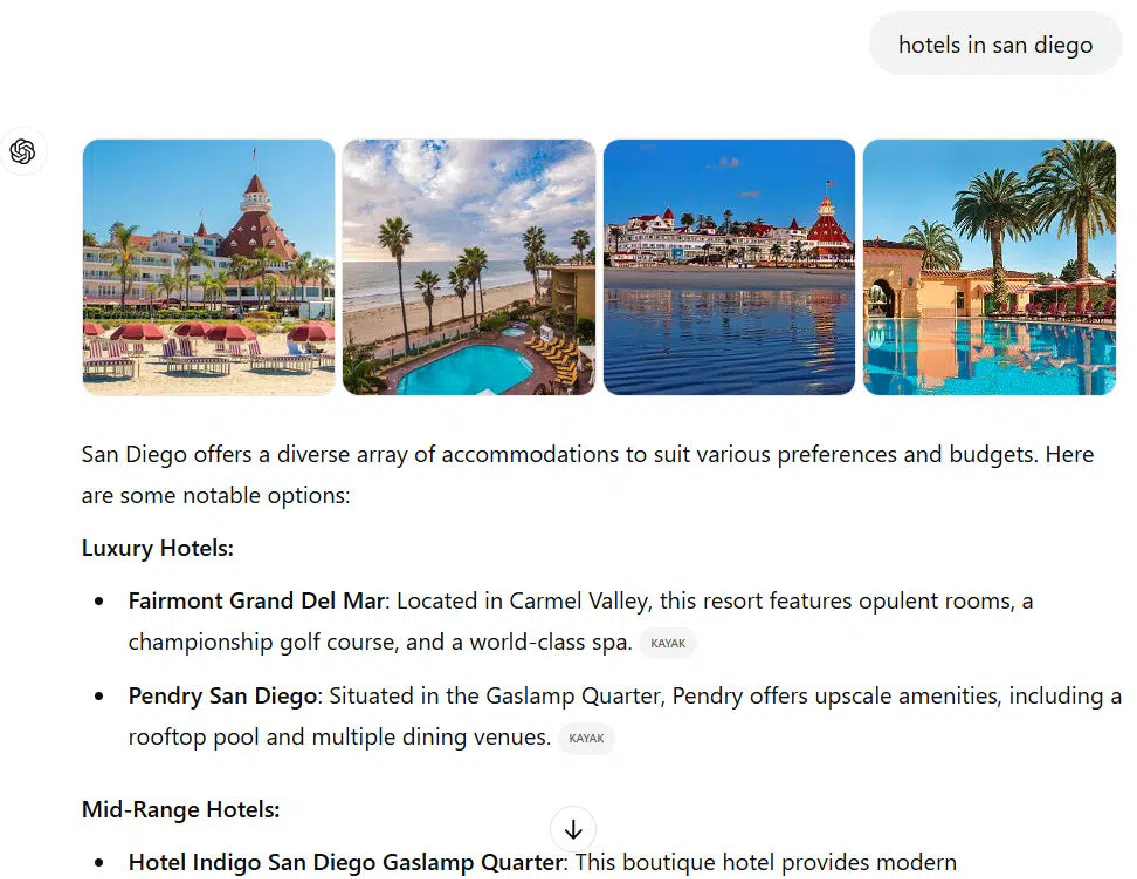
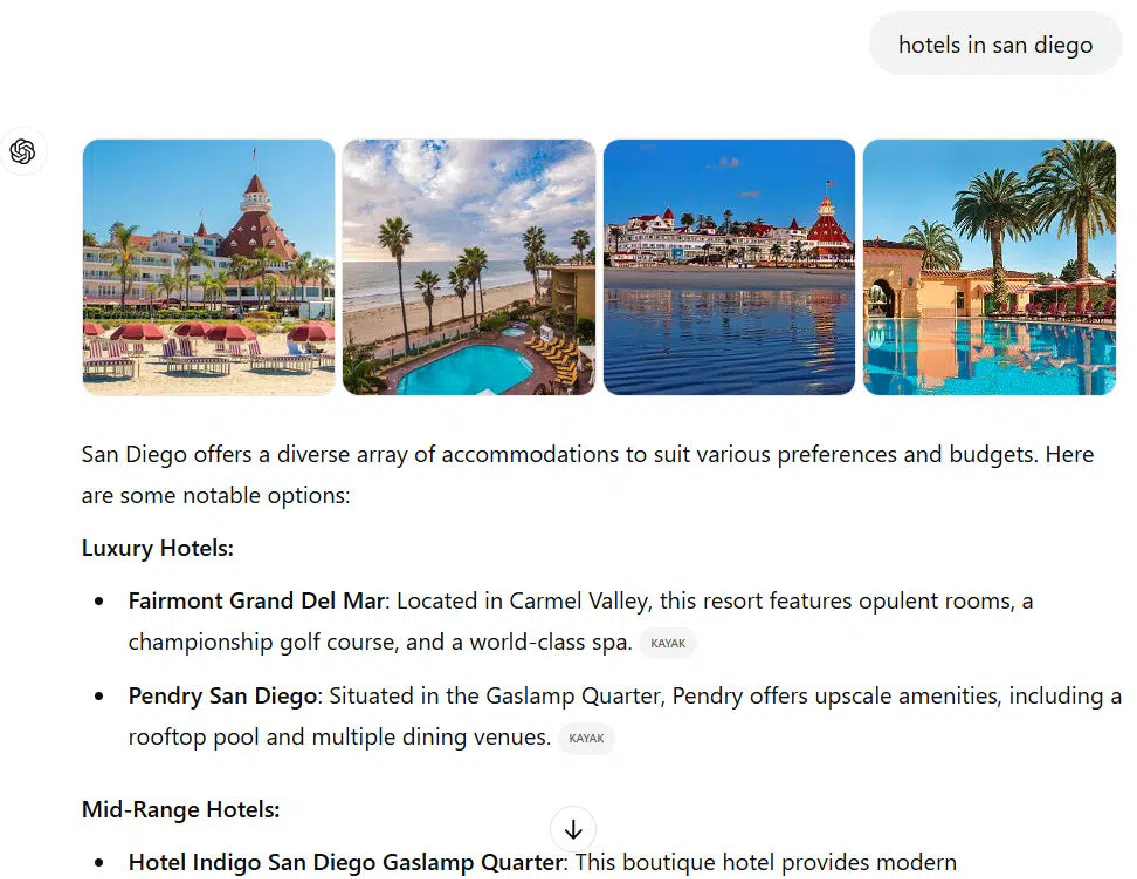
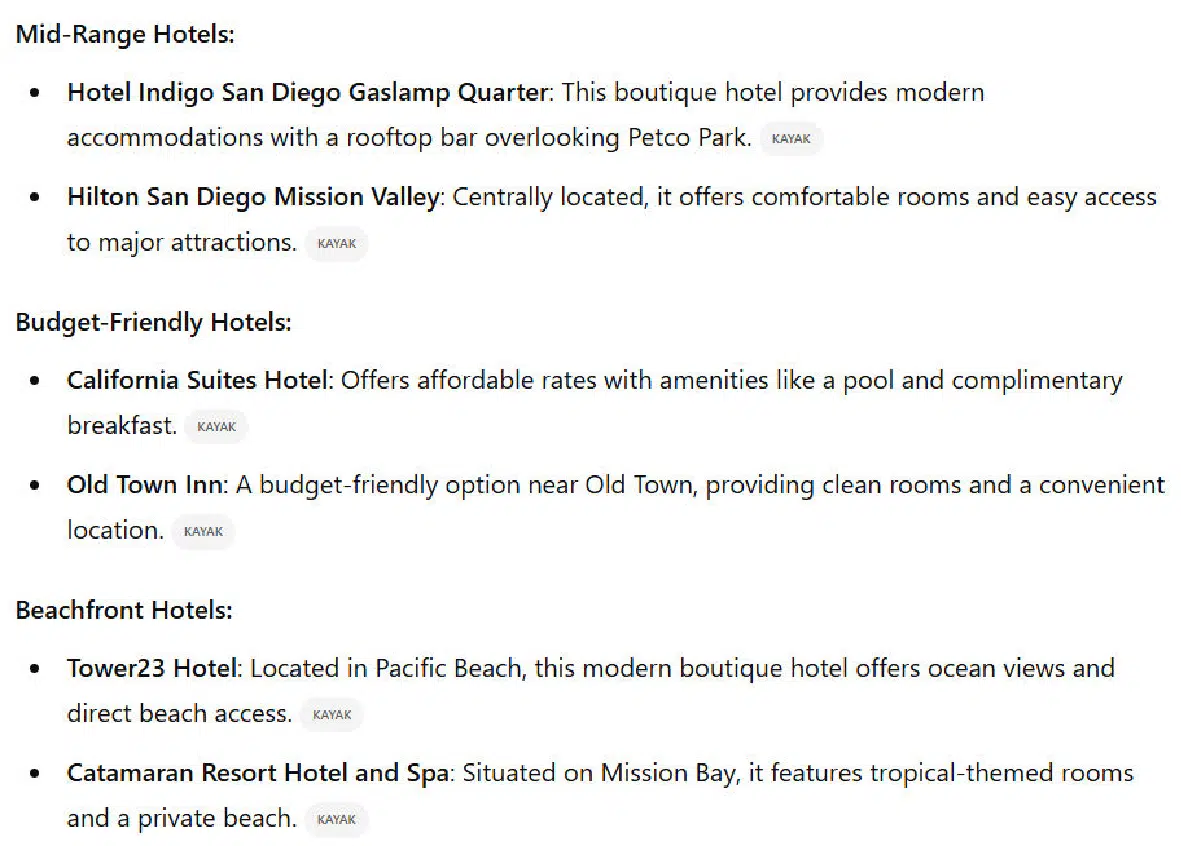
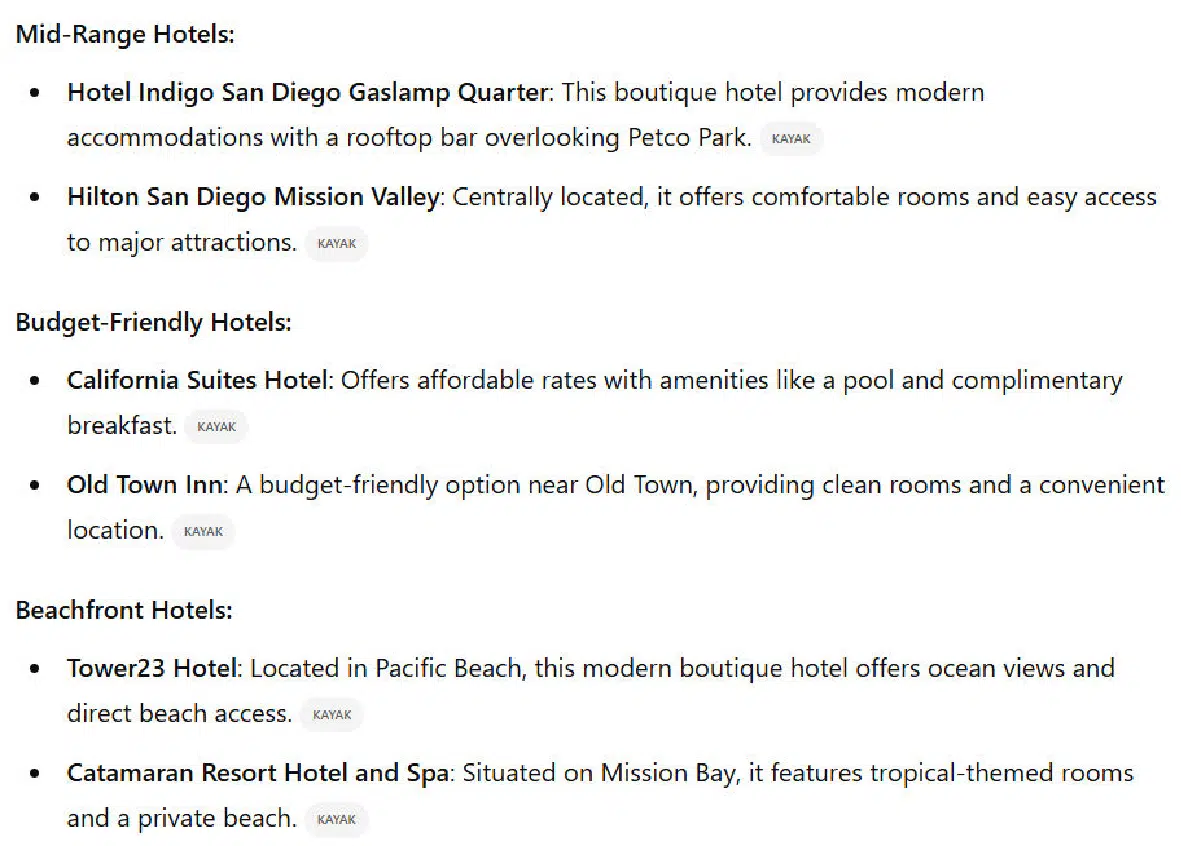
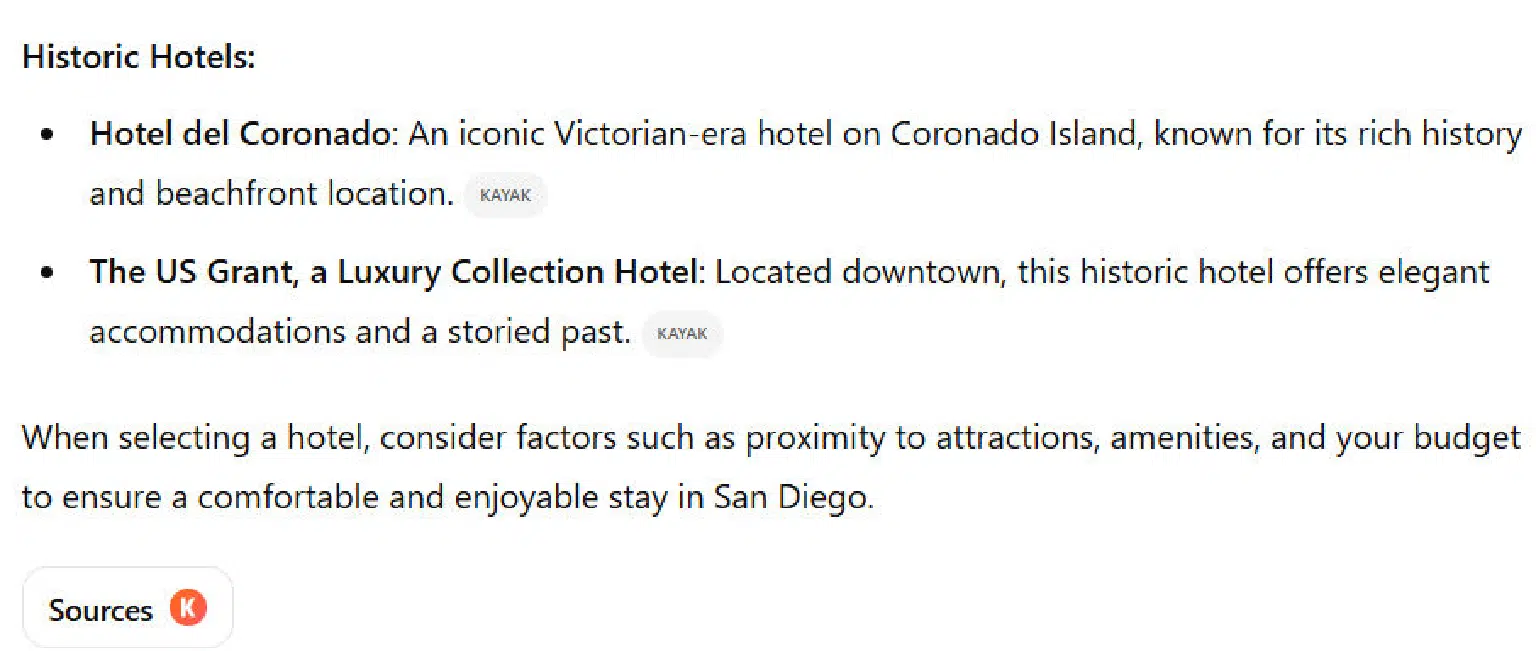
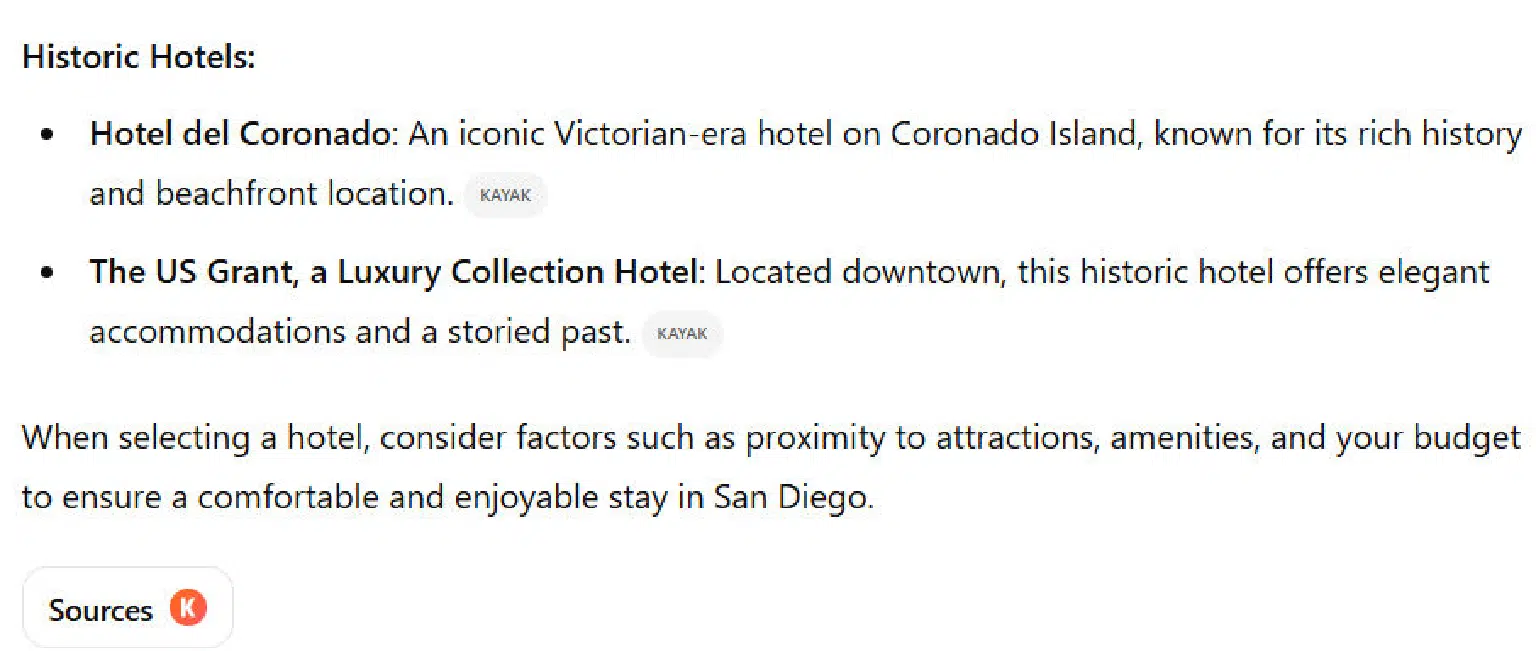
While this provides 11 hotels as options, there are far more than this throughout the San Diego area.
It’s also based on a single source: Kayak.
The user can click through to the Kayak site to get a complete list, but other resources aren’t made available to the user.
In contrast, Google’s results show many different sites that can be used to find what they want. The scores I assigned to the competitors for this one were:
- ChatGPT search: 3.00.
- Google: 6.00.
The winner?
It’s important to note that this analysis is based on a small sample of 62 queries, which is far too limited to draw definitive conclusions about all search scenarios.
A broader takeaway can be gained by reviewing the examples above to see where each platform tends to perform better.
Here’s a breakdown of category winners:
1. Informational queries
- Queries: 42
- Winner: Google
- Google’s average score: 5.83
- ChatGPT search’s average score: 5.19
Google’s slight edge aligns with its strong track record for informational searches.
However, ChatGPT Search performed respectably, despite challenges with errors, omissions, and incomplete responses.
2. Content gap analysis
- Winner: ChatGPT Search
- ChatGPT search’s average score: 3.25
- Google’s average score: 1.0
- ChatGPT Search excels in content gap analysis and related tasks, making it particularly useful for content creators. Winning use cases include:
- Content gap analysis
- Standalone content analysis
- Comparing direct or indirect SERP competitors
- Suggesting article topics and outlines
- Identifying facts/statistics with sources
- Recommending FAQs for articles
While ChatGPT search outperformed Google in this category, its lower overall score highlights areas where improvements are needed, such as accuracy.
3. Navigational queries
Navigational queries were excluded from the test since they typically don’t require detailed text responses.
Google’s dominance in this category is assumed based on its straightforward, website-focused results.
4. Local search queries
- Winner: Google
- Google’s average score: 6.25
- ChatGPT search’s average score: 2.0
Google’s extensive local business data, combined with tools like Google Maps and Waze, ensures its superiority in this category.
5. Commercial queries
- Winner: Google
- Google’s average score: 6.44
- ChatGPT search’s average score: 3.81
This category, comprising 16 queries, favored Google due to its stronger capabilities in showcasing product and service-related results.
6. Disambiguation queries
- Winner: ChatGPT search
- ChatGPT search’s average score: 6.0
- Google’s average score: 5.29
ChatGPT Search edged out Google by more effectively presenting multiple definitions or interpretations for ambiguous terms, providing users with greater clarity.
These scores are summarized in the following table:
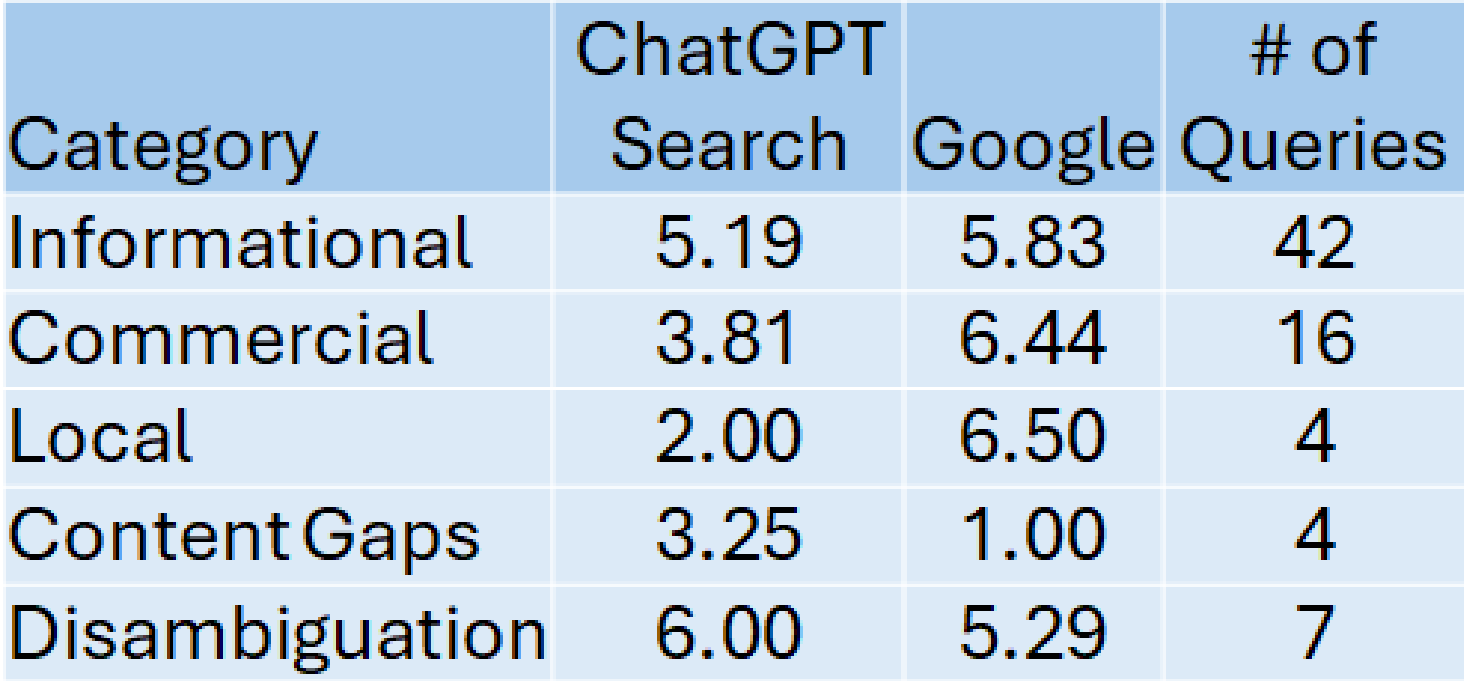
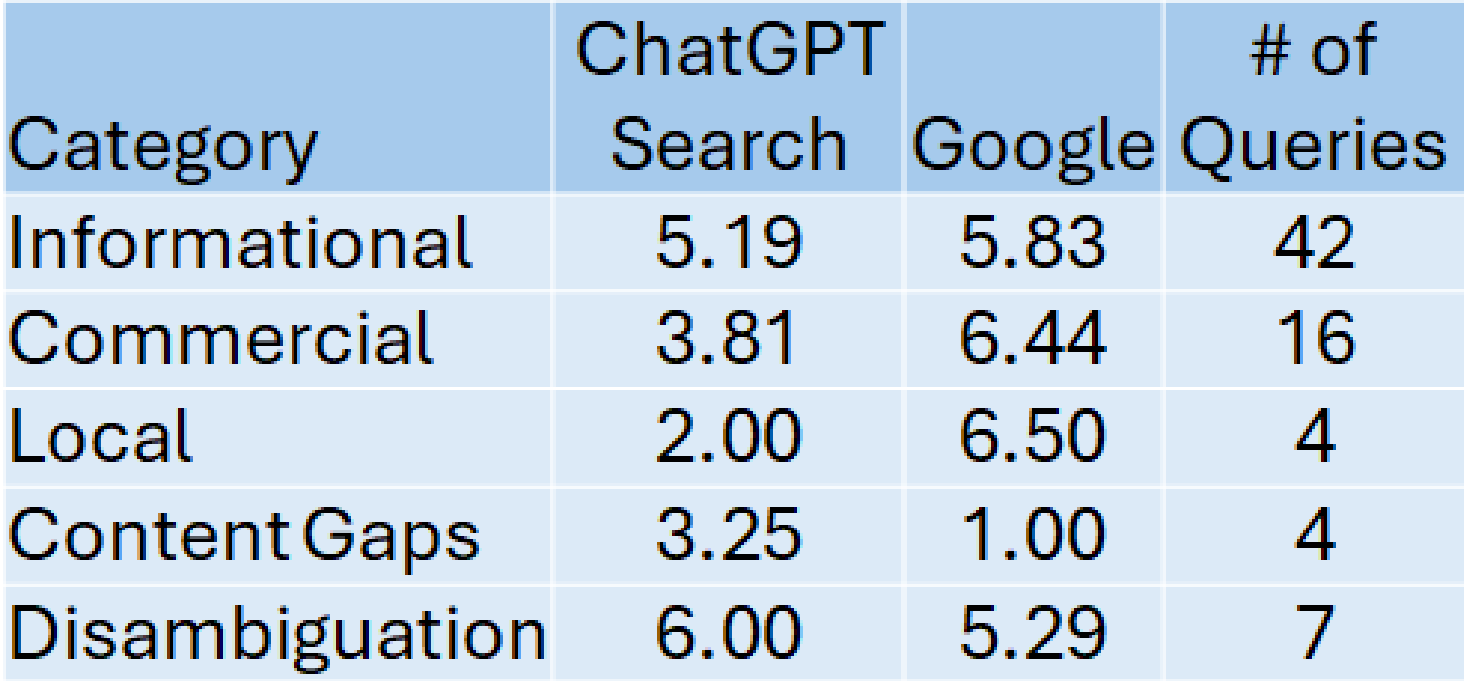
Summary
After a detailed review of 62 queries, I still see Google as the better solution for most searches.
ChatGPT search is surprisingly competitive when it comes to informational queries, but Google edged ChatGPT search out here too.
Note that 62 queries are a tiny sample when considered against the scope of all search.
Nonetheless, as you consider your search plans going forward, I’d advise you to do a segmented analysis like what I did before deciding which platform is the better choice for your projects.
Contributing authors are invited to create content for Search Engine Land and are chosen for their expertise and contribution to the search community. Our contributors work under the oversight of the editorial staff and contributions are checked for quality and relevance to our readers. The opinions they express are their own.
Noticias
Un marco de descubrimiento de arquitectura neuronal de parámetros múltiples automatizados utilizando chatgpt en el backend
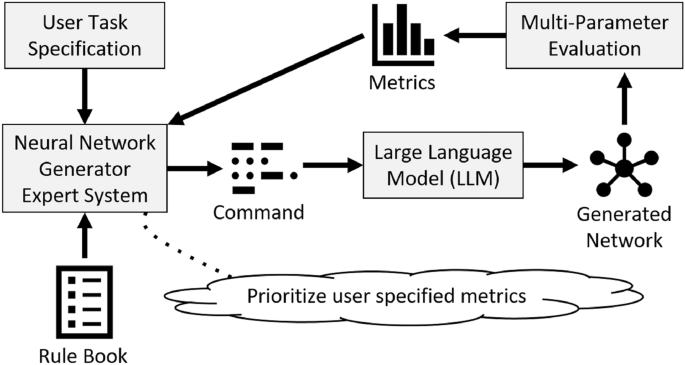
Chua, M. et al. Abordar la incertidumbre de predicción en el aprendizaje automático para la atención médica. Nat. Biomed. Ing. 7711–718 (2023).
Bhardwaj, R. y Tripathi, I. Un algoritmo de ocultación de datos reversibles mejorados que utiliza una red neuronal profunda para E-Healthcare. J. Amb. Intell. Humaniz. Computación. 1410567–10585 (2023).
Nandy, S. et al. Un sistema inteligente de predicción de enfermedades cardíacas basado en la red neuronal artificial enjambre. Computación neuronal. Aplicación 3514723–14737 (2023).
Jaafar, N. y Lachiri, Z. Métodos de fusión multimodal con redes neuronales profundas y metainformación para la detección de agresión en vigilancia. Sistema de expertos. Aplicación 211118523 (2023).
Mahum, R. et al. Un marco robusto para generar resúmenes de video de vigilancia utilizando la combinación de momentos de Zernike y una transformación R y una red neuronal profunda. Multimed. Herramientas apl. 8213811–13835 (2023).
Jan, Z. et al. Inteligencia artificial para la industria 4.0: Revisión sistemática de aplicaciones, desafíos y oportunidades. Sistema de expertos. Aplicación 216119456 (2023).
Raja Santhi, A. y Muthuswamy, P. Industry 5.0 o Industry 4.0 s? Introducción a la industria 4.0 y un vistazo a las posibles tecnologías de la industria 5.0. Int. J. Interact. Des. Manuf. (Ijidem) 17947–979 (2023).
Shafiq, M. et al. Evaluación continua de control de calidad durante la fabricación utilizando algoritmo de aprendizaje supervisado para la industria 4.0. Int. J. Adv. Manuf. Technol. (2023).
Rajput, DS, Meena, G., Acharya, M. y Mohbey, KK Predicción de fallas utilizando red neuronal de convolución difusa en entorno IoT con fusión de datos de detección heterogénea. Medición Sensación 26100701 (2023).
Liyakat, KK S. Enfoque de aprendizaje automático utilizando redes neuronales artificiales para detectar nodos maliciosos en redes IoT. En Conferencia internacional sobre aprendizaje automático, IoT y Big Data 123–134 (Springer, 2023).
Thakkar, A. y Lohiya, R. Clasificación de ataque de datos de intrusión desequilibrados para la red IoT utilizando una red neuronal profunda basada en el aprendizaje. IEEE Internet Things J. 1011888–11895 (2023).
Openai, R. GPT-4 Informe técnico. Preprint en ARXIV: 2303.08774. Ver en el artículo213 (2023).
Wang, J. et al. EL-NAS: Eficiente búsqueda de arquitectura de dominio de atención cruzada para la clasificación de imágenes hiperespectrales. Sensación remota. 154688 (2023).
Yang, T., He, Q. y Huang, L. OM-NAS: Clasificación de imagen de lesión de piel pigmentada basada en una búsqueda de arquitectura neural. Biomed. Optar. Expresar 142153–2165 (2023).
Yang, Y., Wei, J., Yu, Z. y Zhang, R. Un marco de búsqueda de arquitectura neuronal confiable para la clasificación de imágenes de neumonía utilizando tecnología blockchain. J. Supercomput. 801694-1727 (2024).
Hassan, E. et al. Enmascarar modelos R-CNN. Nilo J. Commun. Computación. Sci. 317–27 (2022).
Dong, P. et al. RD-NAS: Mejora de la capacidad de clasificación SuperNet de un solo disparo a través de la destilación de clasificación de proxies de costo cero. En ICASSP 2023-2023 Conferencia internacional IEEE sobre acústica, procesamiento de habla y señales (ICASSP) 1–5 (IEEE, 2023).
Wang, J. et al. NAS-DYMC: red neuronal de convolucional múltiple dinámica basada en NAS para la detección de eventos de sonido. En ICASSP 2023-2023 Conferencia internacional IEEE sobre acústica, procesamiento de habla y señales (ICASSP) 1–5 (IEEE, 2023).
Li, J. et al. Graph Neural Network Architecture Busque para el diagnóstico de fallas de maquinaria giratoria basado en el aprendizaje de refuerzo. Mech. Syst. Proceso de señal. 202110701 (2023).
Yuan, W., Fu, C., Liu, R. y Fan, X. Ssob: Buscando una arquitectura orientada a la escena para la detección de objetos submarinos. VIS. Computación. 395199–5208 (2023).
Jia, X. et al. Detector de objetos rápido y preciso para la conducción autónoma basada en yolov5 mejorado. Sci. Reps. 131–13 (2023).
Mehta, R., Jurečková, O. y Stamp, M. Un enfoque de procesamiento del lenguaje natural para la clasificación de malware. J. Comput. Virol. Tech de piratería. 20173-184 (2024).
Girdhar, N., Coustaty, M. y Doucet, A. Benchmarking Nas para la separación de artículos en periódicos históricos. En Conferencia internacional sobre bibliotecas digitales asiáticas76–88 (Springer, 2023).
Real, E., Aggarwal, A., Huang, Y. y LE, QV Evolución regularizada para la búsqueda de arquitectura del clasificador de imágenes. En Actas de la Conferencia AAAI sobre inteligencia artificial volumen 33, 4780–4789 (2019).
Liu, C. et al. Búsqueda de arquitectura neuronal progresiva. En Actas de la Conferencia Europea sobre Visión Computadora (ECCV) 19–34 (2018).
Cai, H., Chen, T., Zhang, W., Yu, Y. y Wang, J. Búsqueda de arquitectura eficiente por transformación de red. En Actas de la Conferencia AAAI sobre inteligencia artificialvol. 32 (2018).
Pham, H., Guan, M., Zoph, B., Le, Q. y Dean, J. Búsqueda eficiente de arquitectura neuronal a través de parámetros compartiendo. En Conferencia internacional sobre aprendizaje automático 4095–4104 (PMLR, 2018).
Liu, H., Simonyan, K. y Yang, Y. Darts: búsqueda de arquitectura diferenciable. Preimpresión en ARXIV: 1806.09055 (2018).
Ying, C. et al. NAS-Bench-101: Hacia la búsqueda reproducible de arquitectura neuronal. En Conferencia internacional sobre aprendizaje automático 7105–7114 (PMLR, 2019).
Dong, X. y Yang, Y. Nas Bench-201-201: Extendiendo el alcance de la búsqueda de arquitectura neuronal reproducible. Preprint en ARXIV: 2001.00326 (2020).
Krizhevsky, A. y Hinton, G. Aprender múltiples capas de características de pequeñas imágenes (Tech. Rep, Toronto, ON, Canadá, 2009).
Chrabaszcz, P., Loshchilov, I. y Hutter, F. Una variante a la baja de Imagenet como alternativa a los conjuntos de datos CIFAR. Preprint en ARXIV: 1707.08819 (2017).
Ye, P. et al. \(\beta\)-Darts: regularización de beta para la búsqueda de arquitectura diferenciable. En 2022 Conferencia IEEE/CVF sobre visión por computadora y reconocimiento de patrones (CVPR) 10864–10873 (IEEE, 2022).
Movahedi, S. et al. \ (\ lambda \) -Darts: mitigar el colapso del rendimiento al armonizar la selección de operaciones entre las células. Preprint en ARXIV: 2210.07998 (2022).
Zheng, M. et al. ¿Puede GPT-4 realizar la búsqueda de arquitectura neural? Preimpresión en ARXIV: 2304.10970 (2023).
Achiam, J. et al. Informe técnico GPT-4. Preimpresión en ARXIV: 2303.08774 (2023).
Wang, H. et al. Búsqueda de arquitectura neuronal gráfica con GPT-4. Preimpresión en ARXIV: 2310.01436 (2023).
Hassan, E., Bhatnagar, R. y Shams, M. Y. Avance de la investigación científica en ciencias de la computación por Chatgpt y Llama-A Review. En Conferencia internacional sobre fabricación inteligente y sostenibilidad energética 23–37 (Springer, 2023).
Helber, P., Bischke, B., Dengel, A. y Borth, D. Eurosat: un nuevo conjunto de datos y un punto de referencia de aprendizaje profundo para el uso de la tierra y la clasificación de la cobertura de la tierra. IEEE J. Sel. Arriba. Aplicación Tierra obs. Sensación remota. 12(7), 2217–2226 (2019).
Rajaraman, S. et al. Redes neuronales convolucionales previamente entrenadas como extractores de características hacia la detección de parásitos de malaria mejorados en imágenes de frotis de sangre delgada. Peerj 6E4568 (2018).
Maas, A. et al. Vectores de palabras de aprendizaje para el análisis de sentimientos. En Actas de la 49ª Reunión Anual de la Asociación de Lingüística Computacional: Tecnologías de lenguaje humano 142–150 (2011).
Powerapi. Pyjoules: Biblioteca de medición de energía basada en Python para varios dominios, incluidas las GPU NVIDIA. https://github.com/powerapi-ng/pyjoulles (2024). Consultado: 2024-05-31.
Loni, M., Sinaei, S., Zoljodi, A., Daneshtalab, M. y Sjödin, M. Deepmaker: un marco de optimización de objetivos múltiples para redes neuronales profundas en sistemas integrados. Microprocesos. Microsyst. 73102989 (2020).
Suganuma, M., Kobayashi, M., Shirakawa, S. y Nagao, T. Evolución de redes neuronales convolucionales profundas utilizando programación genética cartesiana. Evol. Computación. 28141–163 (2020).
Ren, J. et al. Eigen: enfoque genético de inspiración ecológica para la búsqueda de estructuras de redes neuronales desde cero. En Actas de la conferencia IEEE/CVF sobre visión por computadora y reconocimiento de patrones 9059–9068 (2019).
Xie, L. y Yuille, A. Genetic CNN. En Actas de la Conferencia Internacional IEEE sobre visión por computadora 1379–1388 (2017).
Lu, Z. et al. Diseño evolutivo de criterio múltiple de redes neuronales convolucionales profundas. Preprint en ARXIV: 1912.01369 (2019).
Kandasamy, K., Neiswanger, W., Schneider, J., Poczos, B. y Xing, EP Búsqueda de arquitectura neural con optimización bayesiana y transporte óptimo. Adv. Inf. Neural. Proceso. Syst. 31 (2018).
Elsken, T., Metzen, J.-H. & Hutter, F. Búsqueda de arquitectura simple y eficiente para redes neuronales convolucionales. Preimpresión en ARXIV: 1711.04528 (2017).
Dong, X. y Yang, Y. Buscando una arquitectura neuronal robusta en cuatro horas de GPU. En Actas de la conferencia IEEE/CVF sobre visión por computadora y reconocimiento de patrones 1761–1770 (2019).
Chu, X. et al. DARTS-: Salir de manera robusta del colapso de rendimiento sin indicadores. Preprint en ARXIV: 2009.01027 (2020).
Chen, X., Wang, R., Cheng, M., Tang, X. y Hsieh, C.-J. DRNAS: búsqueda de arquitectura neural de Dirichlet. Preprint en ARXIV: 2006.10355 (2020).
Hu, Y., Wang, X., Li, L. y Gu, Q. Mejora de NAS de un solo disparo con Supernet reducida y expansiva. Reconocimiento de patrones. 118108025 (2021).
Chu, X., Zhang, B. y Xu, R. Fairnas: Repensar la equidad de evaluación de la búsqueda de arquitectura neuronal compartiendo peso. En Actas de la conferencia internacional IEEE/CVF sobre visión por computadora 12239–12248 (2021).
Xiao, H., Wang, Z., Zhu, Z., Zhou, J. y Lu, J. Shapley-NAS: Descubrimiento de la contribución de la operación para la búsqueda de arquitectura neural. En Actas de la conferencia IEEE/CVF sobre visión por computadora y reconocimiento de patrones 11892–11901 (2022).
Yu, K., Ranftl, R. y Salzmann, M. Regularización histórica: clasificación de entrenamiento guiado de Super Net en la búsqueda de arquitectura neural. En Actas de la conferencia IEEE/CVF sobre visión por computadora y reconocimiento de patrones 13723–13732 (2021).
Cavagnero, N., Robbiano, L., Caputo, B. y Avera, G. Freerea: Búsqueda de arquitectura basada en la evolución libre de capacitación. En Actas de la conferencia de invierno IEEE/CVF sobre aplicaciones de visión por computadora 1493–1502 (2023).
Zheng, X. et al. Búsqueda de arquitectura neuronal con representación de información mutua. En Actas de la conferencia IEEE/CVF sobre visión por computadora y reconocimiento de patrones 11912–11921 (2022).
Strubell, E., Ganesh, A. y McCallum, A. Consideraciones de energía y política para el aprendizaje profundo en la PNL. Preprint en ARXIV: 1906.02243 (2019).
Zoph, B., Vasudevan, V., Shlens, J. y Le, Q. V. Aprender arquitecturas transferibles para el reconocimiento de imágenes escalables. En Actas de la conferencia IEEE sobre visión por computadora y reconocimiento de patrones 8697–8710 (2018).
Noticias
El ex ejecutivo de Operai se une a la IA, el sector público y los líderes de ciberseguridad que encabezan Info-Tech Live 2025 en Las Vegas
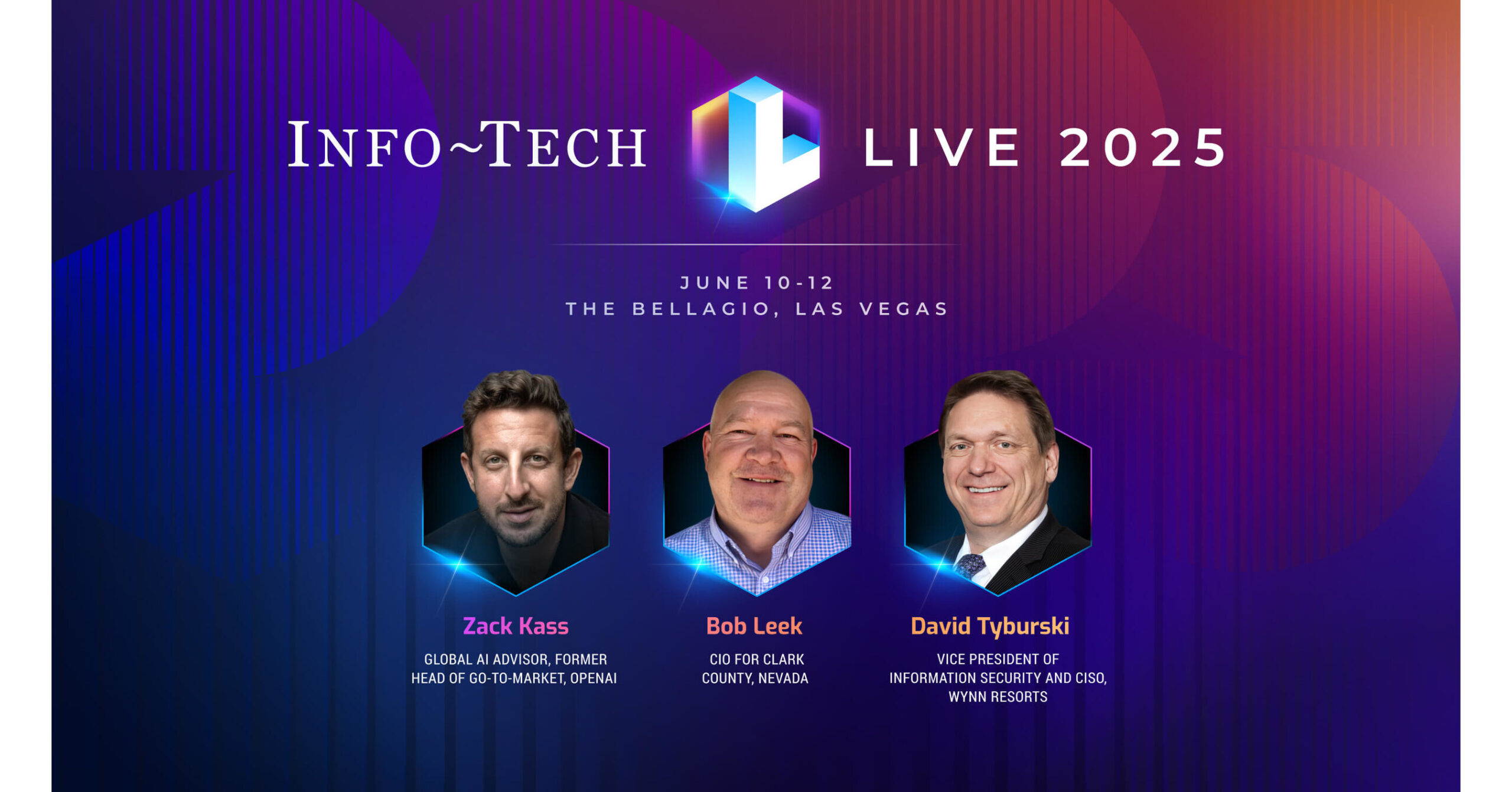
A medida que Momentum continúa construyendo en las semanas previas a la muy esperada conferencia anual de la industria para CIO y líderes de TI, Info-Tech Research Group ha anunciado tres nuevos oradores destacados para Info-Tech Live 2025 en Las Vegas en junio. Los altavoces recién revelados incluyen Zack Kassex jefe del mercado de ir al mercado en Openai; Bob LeeCIO para Condado de Clark, Nevada; y David TyburskiVicepresidente de Seguridad de la Información y CISO en Wynn Resorts – Voces líderes en IA, innovación del sector público y ciberseguridad. Sus notas clave Ofrezca claridad, estrategia y ideas prácticas sobre los desafíos de TI más urgentes de hoy al proporcionar diversas perspectivas sobre cómo la tecnología está remodelando las industrias, las instituciones y el liderazgo en sí.
Toronto, 14 de mayo de 2025 / PRNewswire/-Info-Tech Research Group, una firma líder mundial de investigación y asesoramiento de TI, ha anunciado tres oradores destacados adicionales para su próximo Info-Tech Live 2025 en Las Vegas Conferencia de TI. Los altavoces son Zack Kassex jefe del mercado de ir al mercado en Openai; Bob LeeCIO para Condado de Clark, Nevada; y David TyburskiVicepresidente de Seguridad de la Información y CISO en Wynn Resorts. Estos oradores compartirán su experiencia en innovación de IA, liderazgo del sector público y ciberseguridad empresarial en el escenario principal del evento insignia de la firma, que tiene lugar. 10-12 de junio, 2025en Bellagio en Las Vegas.
Info-Tech Live 2025 reunirá a miles de CIO, CDO, CISO y líderes de TI durante tres días de notas clave, Insights de analistas y compromiso entre pares. La urgencia y la oportunidad que enfrentan los líderes tecnológicos hoy mientras navegan por la interrupción y la innovación se refleja en el tema de este año “Transformarlo. Transformar todo”.
“Estos altavoces destacados para Info-Tech Live 2025 en Las Vegas Refleja las prioridades y presiones en evolución que enfrentan los líderes de TI hoy, en todas las industrias y mercados “, dice el director de investigación del grupo de investigación de información de información, Gord Harrison. “Desde redefinir cómo las organizaciones se involucran con la IA, hasta la transformación de la prestación de servicios públicos, hasta la defensa de la infraestructura digital en las industrias de alto riesgo, estos líderes aportan información crítica del futuro. Juntos, sus perspectivas ayudarán a los asistentes a ir más allá de la conciencia y tomar una acción estratégica y confidencial”.
Recientemente anunciados oradores destacados para información-tech en vivo 2025 en Las Vegas:
Las últimas incorporaciones a la lista de oradores 2025 de Info-Tech ofrecen a los asistentes una gran cantidad de experiencia en décadas de liderazgo práctico, consultoría e innovación. Sus sesiones proporcionarán nuevas perspectivas sobre los desafíos empresariales actuales, desde la navegación de tecnologías emergentes y las demandas de cumplimiento hasta las estrategias de transformación de escala y alinear las inversiones de TI con el crecimiento empresarial. Los oradores recién anunciados incluyen:
- Zack Kass, Asesor global de IA, ex jefe de Go To-Mercado, OpenAI
Zack Kass es un asesor futurista y global que ayuda a Fortune 1000 empresas y gobiernos a adaptarse al panorama de IA que cambia rápidamente. Como ex jefe del mercado de ir a OpenAI, ayudó a construir y liderar a los equipos responsables de traducir la investigación en aplicaciones del mundo real. Kass ahora trabaja para desmitificar la IA y dar forma a un futuro donde la tecnología sirve a las personas y la sociedad.
- Bob LeeCIO para Condado de Clark, Nevada
Bob Lee sirve como CIO para Condado de Clark, Nevadaapoyando a más de 2.4 millones de residentes, 90,000 empresas y más de 50 millones de visitantes anualmente. Con más de 25 años de experiencia en los sectores público y privado, Leek se centra en el cambio transformador, el liderazgo inclusivo y el uso de la tecnología para mejorar los resultados para las comunidades a las que sirve.
- David TyburskiVP de seguridad de la información y director de seguridad de la información para Wynn Resorts
David Tyburski Lidera la estrategia global de ciberseguridad de Wynn Resorts, supervisando la identidad y el acceso, la gestión de riesgos y la respuesta a los incidentes. Con más de 30 años en TI y seguridad, Tyburski también asesora sobre múltiples juntas de la industria y sirve en la Junta Asesora de Tecnología de la Información del Estado de Nevada.
Info-tech en vivo 2025 en Las Vegas Proporcionará estrategias procesables e información de investigación en profundidad a los líderes y ejecutivos de TI en todas las industrias. Los asistentes tendrán la oportunidad de interactuar con los analistas expertos de Info-Tech, participar en sesiones interactivas y mesas redondas, y obtener un conocimiento crítico sobre el panorama de TI en rápida evolución. La conferencia también contará con una impresionante línea de oradores principales, talleres y eventos de redes diseñados para equipar a los asistentes con las herramientas para impulsar la transformación de TI exponencial. Se publicarán anuncios adicionales en las semanas previas a la conferencia.
Para obtener los últimos detalles, visite el Info-Tech Live 2025 en Las Vegas página, y siga el grupo de investigación de información de información sobre LinkedIn y incógnita.
Media pasa por información-Tech Live 2025 en Las Vegas
Los profesionales de los medios, incluidos periodistas, podcasters e influencers, están invitados a asistir a Info-Tech Live 2025 para obtener acceso exclusivo a la investigación, el contenido y las entrevistas con los líderes de la industria. Para aquellos que no pueden asistir en persona, Info-Tech ofrece una opción de pase digital, proporcionando acceso a notas clave en vivo, sesiones seleccionadas y entrevistas virtuales exclusivas con oradores y analistas.
Los profesionales de los medios que buscan solicitar pases en persona o digitales pueden contactar pr@infotech.com Para asegurar su lugar y cubrir los últimos avances en él para su público.
Oportunidades de expositor
Los expositores también están invitados a formar parte de Info-Tech Live y mostrar sus productos y servicios a un público altamente comprometido de tomadores de decisiones de TI. Para obtener más información sobre cómo convertirse en un expositor de información en vivo, comuníquese con events@infotech.com.
Acerca del grupo de investigación de tecnología de información
Info-Tech Research Group es una de las principales empresas de investigación y asesoramiento del mundo, que atiende con orgullo a más de 30,000 profesionales. La compañía produce una investigación imparcial y altamente relevante y brinda servicios de asesoramiento para ayudar a los líderes a tomar decisiones estratégicas, oportunas y bien informadas. Durante casi 30 años, Info-Tech se ha asociado estrechamente con los equipos para proporcionarles todo lo que necesitan, desde herramientas procesables hasta orientación de analistas, asegurando que brinden resultados medibles para sus organizaciones.
Para obtener más información sobre las divisiones de Info-Tech, visite McLean & Company para obtener servicios de investigación y asesoramiento de recursos humanos y SoftWarReviews para obtener información sobre la compra de software.
Los profesionales de los medios pueden registrarse para un acceso sin restricciones a la investigación a través de TI, recursos humanos y software y cientos de analistas de la industria a través del Programa de Insiders de Medios de la empresa. Para obtener acceso, contactar pr@infotech.com.
Grupo de investigación de tecnología de información de origen
Noticias
Operai trae GPT-4.1 y 4.1 mini a Chatgpt-Lo que las empresas deben saber
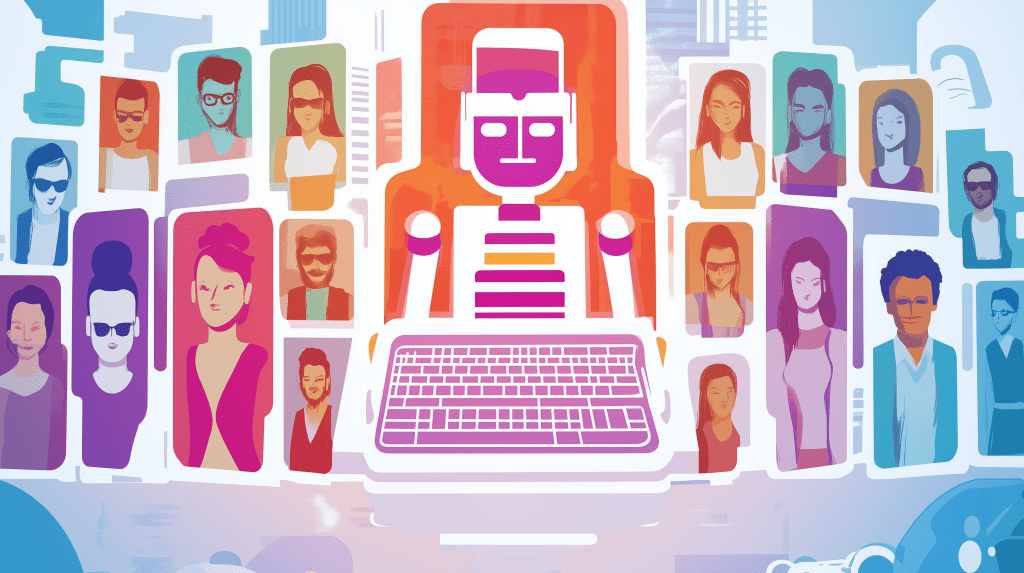
Únase a nuestros boletines diarios y semanales para obtener las últimas actualizaciones y contenido exclusivo sobre la cobertura de IA líder de la industria. Obtenga más información
Operai está implementando GPT-4.1, su nuevo modelo de lenguaje grande (LLM) no inicial que equilibra el alto rendimiento con menor costo, para los usuarios de ChatGPT. La compañía está comenzando con sus suscriptores que pagan en ChatGPT Plus, Pro y Equipo, con el acceso a los usuarios de la empresa y la educación esperada en las próximas semanas.
También está agregando GPT-4.1 Mini, que reemplaza a GPT-4O Mini como el valor predeterminado para todos los usuarios de ChatGPT, incluidos los de nivel gratuito. La versión “Mini” proporciona un parámetro a menor escala y, por lo tanto, una versión menos potente con estándares de seguridad similares.
Ambos modelos están disponibles a través de la selección desplegable “Más modelos” en la esquina superior de la ventana de chat dentro de ChatGPT, dando a los usuarios flexibilidad para elegir entre modelos GPT-4.1, GPT-4.1 mini y razonamiento como O3, O4-Mini y O4-Mini-High.
Inicialmente destinado a usar solo por el software de terceros y los desarrolladores de IA a través de la interfaz de programación de aplicaciones (API) de OpenAI, se agregó GPT-4.1 a ChatGPT siguiendo fuertes comentarios de los usuarios.
El líder de investigación de la capacitación posterior de Operai, Michelle Pokrass, confirmó en X, el cambio fue impulsado por la demanda, escribiendo: “Inicialmente estábamos planeando mantener esta API de modelo solo, pero todos lo querían en Chatgpt ¡feliz codificación!”
El director de productos de Operai, Kevin Weil, publicó en X diciendo: “Lo construimos para los desarrolladores, por lo que es muy bueno para la codificación e instrucciones siguientes, ¡hágalo un intento!”
Un modelo centrado en la empresa
GPT-4.1 fue diseñado desde cero para la practicidad de grado empresarial.
Lanzado en abril de 2025 junto con GPT-4.1 Mini y Nano, esta familia modelo priorizó las necesidades de los desarrolladores y los casos de uso de producción.
GPT-4.1 ofrece una mejora de 21.4 puntos sobre GPT-4O en el punto de referencia de ingeniería de software verificado SWE-Bench, y una ganancia de 10.5 puntos en tareas de seguimiento de instrucciones en el punto de referencia MultiChallenge de Scale. También reduce la verbosidad en un 50% en comparación con otros modelos, un rasgo de los usuarios de la empresa elogió durante las pruebas tempranas.
Contexto, velocidad y acceso al modelo
GPT-4.1 admite el contexto estándar Windows para ChatGPT: 8,000 tokens para usuarios gratuitos, 32,000 tokens para usuarios más y 128,000 tokens para usuarios de Pro.
Según el desarrollador Angel Bogado Publicing en X, estos límites coinciden con los utilizados por los modelos de CHATGPT anteriores, aunque los planes están en marcha para aumentar aún más el tamaño del contexto.
Si bien las versiones API de GPT-4.1 pueden procesar hasta un millón de tokens, esta capacidad ampliada aún no está disponible en ChatGPT, aunque el soporte futuro se ha insinuado.
Esta capacidad de contexto extendida permite a los usuarios de la API alimentar las bases de código enteras o grandes documentos legales y financieros en el modelo, útil para revisar contratos de documentos múltiples o analizar grandes archivos de registro.
Operai ha reconocido cierta degradación del rendimiento con entradas extremadamente grandes, pero los casos de prueba empresarial sugieren un rendimiento sólido de hasta varios cientos de miles de tokens.
Evaluaciones y seguridad
Operai también ha lanzado un sitio web de Safety Evaluations Hub para brindar a los usuarios acceso a métricas clave de rendimiento en todos los modelos.
GPT-4.1 muestra resultados sólidos en estas evaluaciones. En las pruebas de precisión de hecho, obtuvo 0.40 en el punto de referencia SimpleQA y 0.63 en Personqa, superando a varios predecesores.
También obtuvo 0.99 en la medida “no insegura” de OpenAI en las pruebas de rechazo estándar, y 0.86 en indicaciones más desafiantes.
Sin embargo, en la prueba de jailbreak Strongject, un punto de referencia académico para la seguridad en condiciones adversas, GPT-4.1 obtuvo 0.23, detrás de modelos como GPT-4O-Mini y O3.
Dicho esto, obtuvo un fuerte 0.96 en indicaciones de jailbreak de origen humano, lo que indica una seguridad más robusta del mundo real bajo el uso típico.
En la adhesión de instrucciones, GPT-4.1 sigue la jerarquía definida de OpenAI (sistema sobre desarrollador, desarrollador sobre mensajes de usuario) con una puntuación de 0.71 para resolver conflictos de mensajes del sistema frente a usuario. También funciona bien para proteger frases protegidas y evitar regalos de soluciones en escenarios de tutoría.
Contextualización de GPT-4.1 contra predecesores
El lanzamiento de GPT-4.1 se produce después del escrutinio alrededor de GPT-4.5, que debutó en febrero de 2025 como una vista previa de investigación. Ese modelo enfatizó un mejor aprendizaje sin supervisión, una base de conocimiento más rica y alucinaciones reducidas, que caían del 61.8% en GPT-4O al 37.1%. También mostró mejoras en los matices emocionales y la escritura de forma larga, pero muchos usuarios encontraron las mejoras sutiles.
A pesar de estas ganancias, GPT-4.5 generó críticas por su alto precio, hasta $ 180 por millón de tokens de producción a través de API, y por un rendimiento decepcionante en matemáticas y puntos de referencia de codificación en relación con los modelos O-Series O de OpenAi. Las cifras de la industria señalaron que si bien GPT-4.5 era más fuerte en la conversación general y la generación de contenido, tuvo un rendimiento inferior en aplicaciones específicas del desarrollador.
Por el contrario, GPT-4.1 se pretende como una alternativa más rápida y más enfocada. Si bien carece de la amplitud de conocimiento de GPT-4.5 y un modelado emocional extenso, está mejor sintonizado para la asistencia de codificación práctica y se adhiere de manera más confiable a las instrucciones del usuario.
En la API de OpenAI, GPT-4.1 tiene un precio de $ 2.00 por millón de tokens de entrada, $ 0.50 por millón de tokens de entrada en caché y tokens de salida de $ 8.00 por millón.
Para aquellos que buscan un saldo entre velocidad e inteligencia a un costo más bajo, GPT-4.1 Mini está disponible en $ 0.40 por millón de tokens de entrada, $ 0.10 por millón de tokens de entrada en caché y tokens de salida de $ 1.60 por millón.
Los modelos Flash-Lite y Flash de Google están disponibles a partir de $ 0.075– $ 0.10 por millón de tokens de entrada y $ 0.30– $ 0.40 por millón de tokens de salida, menos de una décima parte del costo de las tasas base de GPT-4.1.
Pero si bien GPT-4.1 tiene un precio más alto, ofrece puntos de referencia de ingeniería de software más fuertes y una instrucción más precisa después, lo que puede ser crítico para los escenarios de implementación empresarial que requieren confiabilidad sobre el costo. En última instancia, el GPT-4.1 de OpenAI ofrece una experiencia premium para el rendimiento de precisión y desarrollo, mientras que los modelos Gemini de Google atraen a empresas conscientes de costos que necesitan niveles de modelos flexibles y capacidades multimodales.
Lo que significa para los tomadores de decisiones empresariales
La introducción de GPT-4.1 aporta beneficios específicos a los equipos empresariales que administran la implementación de LLM, la orquestación y las operaciones de datos:
- Ingenieros de IA Supervisando la implementación de LLM puede esperar una velocidad mejorada e instrucción de adherencia. Para los equipos que administran el ciclo de vida LLM completo, desde el modelo de ajuste hasta la resolución de problemas, GPT-4.1 ofrece un conjunto de herramientas más receptivo y eficiente. Es particularmente adecuado para equipos Lean bajo presión para enviar modelos de alto rendimiento rápidamente sin comprometer la seguridad o el cumplimiento.
- La orquestación de IA conduce Centrado en el diseño de tuberías escalable apreciará la robustez de GPT-4.1 contra la mayoría de las fallas inducidas por el usuario y su fuerte rendimiento en las pruebas de jerarquía de mensajes. Esto facilita la integración en los sistemas de orquestación que priorizan la consistencia, la validación del modelo y la confiabilidad operativa.
- Ingenieros de datos Responsable de mantener una alta calidad de datos e integrar nuevas herramientas se beneficiará de la tasa de alucinación más baja de GPT-4.1 y una mayor precisión objetiva. Su comportamiento de salida más predecible ayuda a construir flujos de trabajo de datos confiables, incluso cuando los recursos del equipo están limitados.
- Profesionales de seguridad de TI La tarea de integrar la seguridad en las tuberías de DevOps puede encontrar valor en la resistencia de GPT-4.1 a jailbreaks comunes y su comportamiento de salida controlado. Si bien su puntaje académico de resistencia de jailbreak deja espacio para mejorar, el alto rendimiento del modelo contra las exploits de origen humano ayuda a apoyar la integración segura en herramientas internas.
En estos roles, el posicionamiento de GPT-4.1 como un modelo optimizado para mayor claridad, cumplimiento y eficiencia de implementación lo convierte en una opción convincente para empresas medianas que buscan equilibrar el rendimiento con las demandas operativas.
Un nuevo paso adelante
Mientras que GPT-4.5 representaba un hito de escala en el desarrollo del modelo, GPT-4.1 se centra en la utilidad. No es el más caro o el más multimodal, pero ofrece ganancias significativas en áreas que importan para las empresas: precisión, eficiencia de implementación y costo.
Este reposicionamiento refleja una tendencia de la industria más amplia, alejada de la construcción de los modelos más grandes a cualquier costo y hacia los modelos capaces más accesibles y adaptables. GPT-4.1 cumple con esa necesidad, ofreciendo una herramienta flexible y lista para la producción para equipos que intentan integrar la IA más profundamente en sus operaciones comerciales.
A medida que OpenAI continúa evolucionando sus ofertas de modelos, GPT-4.1 representa un paso adelante en la democratización de IA avanzada para entornos empresariales. Para la capacidad de equilibrio de los tomadores de decisiones con el ROI, ofrece un camino más claro hacia el despliegue sin sacrificar el rendimiento o la seguridad.
-
Startups12 meses ago
Remove.bg: La Revolución en la Edición de Imágenes que Debes Conocer
-
Tutoriales1 año ago
Cómo Comenzar a Utilizar ChatGPT: Una Guía Completa para Principiantes
-
Recursos1 año ago
Cómo Empezar con Popai.pro: Tu Espacio Personal de IA – Guía Completa, Instalación, Versiones y Precios
-
Startups10 meses ago
Startups de IA en EE.UU. que han recaudado más de $100M en 2024
-
Startups1 año ago
Deepgram: Revolucionando el Reconocimiento de Voz con IA
-
Recursos12 meses ago
Perplexity aplicado al Marketing Digital y Estrategias SEO
-
Recursos1 año ago
Suno.com: La Revolución en la Creación Musical con Inteligencia Artificial
-
Noticias10 meses ago
Dos periodistas octogenarios deman a ChatGPT por robar su trabajo