Noticias
A deep dive analysis of 62 queries
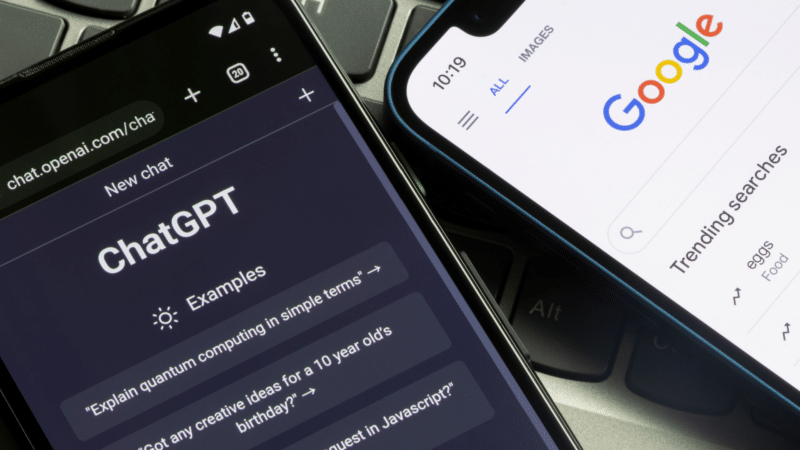
The emergence of ChatGPT search has led to many questions about the quality of the overall results compared to Google.
This is a difficult question to answer, and in today’s article, I will provide some insights into how to do just that.
Note that our understanding is that the technology that makes it possible for OpenAI to offer a search capability is called SearchGPT, but the actual product name is ChatGPT search.
In this article, we will use the name ChatGPT search.
What’s in this report
This report presents an analysis of 62 queries to assess the strengths and weaknesses of each platform.
Each response was meticulously fact-checked and evaluated for alignment with potential user intents.
The process, requiring about an hour per query, highlighted that “seemingly good” and “actually good” answers often differ.
Additionally, when Google provided an AI Overview, it was scored against ChatGPT search.
A combined score for the AI Overviews and the rest of Google’s SERP was also included.
Of the queries tested – two-thirds of which were informational – Google returned an AI Overview in 25 instances (40% of the time).
The queries analyzed fell into multiple categories:
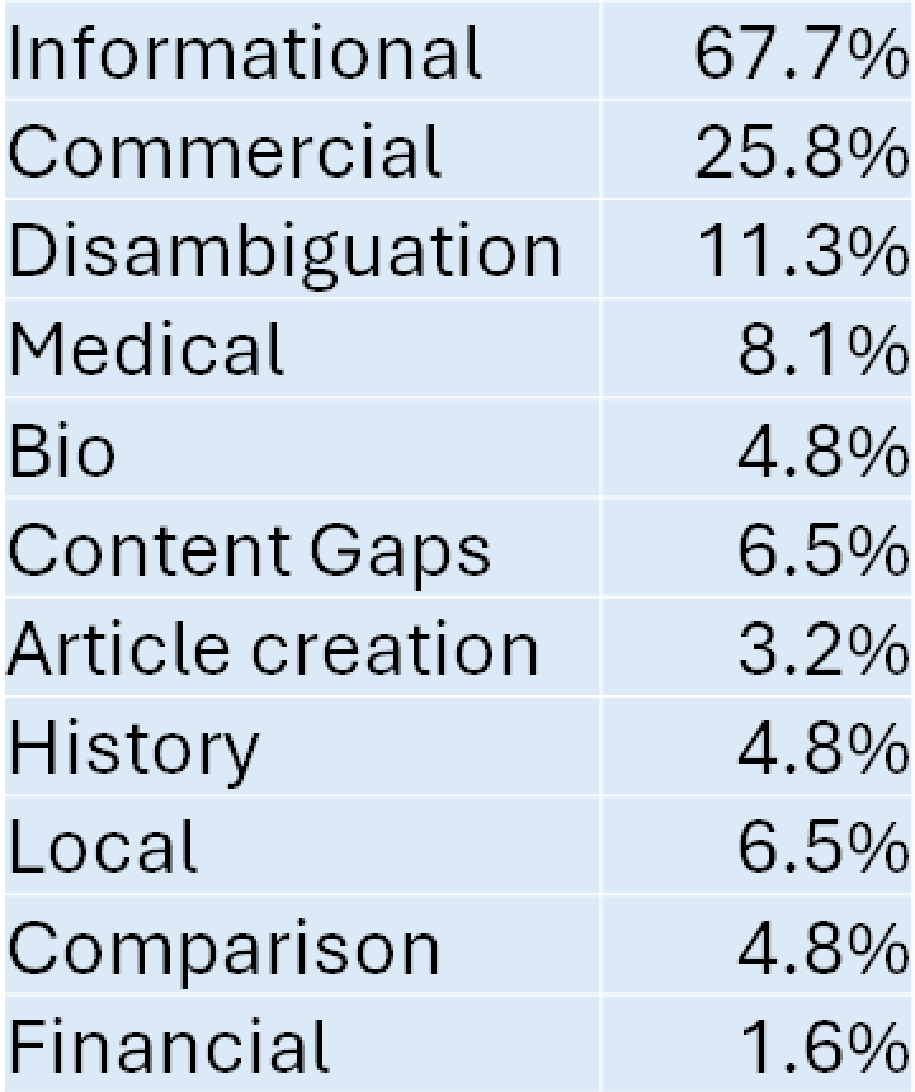
The total number of the above is greater than 100%, and that’s because some queries could fall into more than one classification.
For example, about 13% of the queries were considered informational and commercial.
Detailed information from SparkToro on the makeup of queries suggests a natural distribution of search queries as follows:
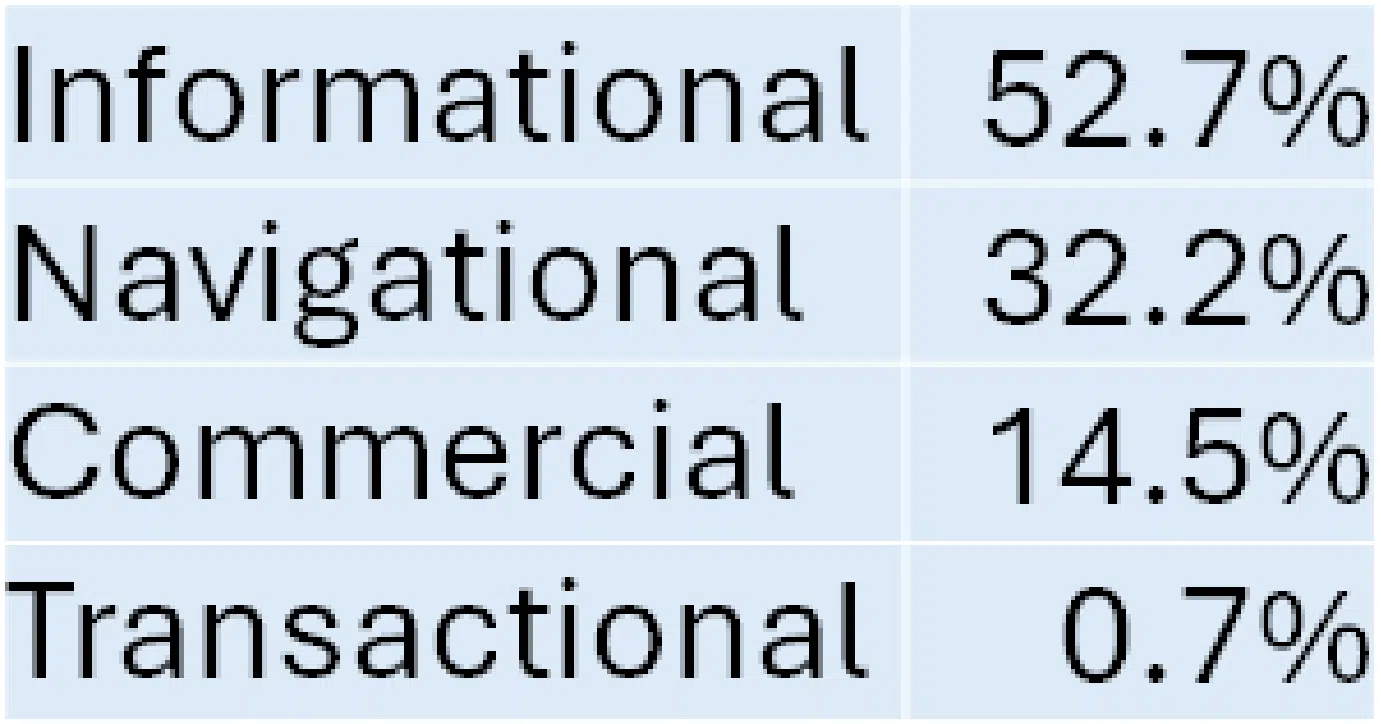
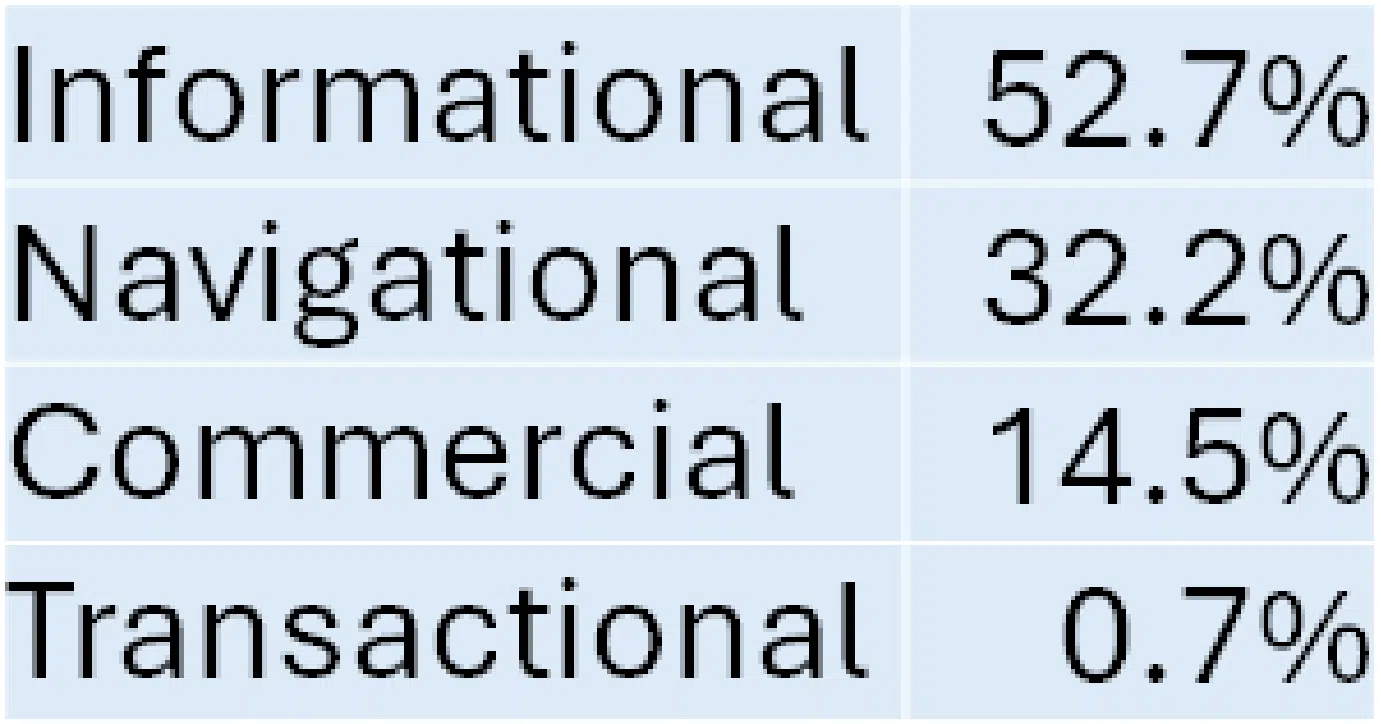
Navigational queries, which comprise nearly a third of all queries, were excluded from this test.
These queries typically demand a straightforward response like, “just give me the website,” and are a category where Google excels.
However, I included queries likely to favor one platform, such as:
- Content gap analysis queries (4): Representing a broader class of content-related queries, which Google doesn’t handle but ChatGPT search attempts (though not always successfully).
- Locally oriented queries (4): These leverage Google’s extensive local business database, Google Maps, and Waze, areas where ChatGPT search struggles to compete.
Metrics used in this study
I designed 62 queries to reflect diverse query intents, aiming to highlight each platform’s strengths and weaknesses.
Each response was scored across specific metrics to evaluate performance effectively.
- Errors: Did the response include incorrect information?
- Omissions: Was important information not in the response?
- Weaknesses: Were other aspects of the response considered weak but not scored as an error or omission?
- Fully addresses: Was the user’s query intent substantially addressed?
- Follow-up resources: Did the response provide suitable resources for follow-up research?
- Quality: An assessment by me of the overall quality of the response. This was done by weighing the other factors contained in this list.
At the end of this article are the total scores for each platform across the 62 queries.
Competitive observations
When considering how different search platforms provide value, it’s important to understand the many aspects of the search experience. Here are some of those areas:
Advertising
Multiple reviewers note that ChatGPT search is ad-free and tout how much better this makes it than Google. That is certainly the case now, but it won’t stay that way.
Microsoft has $13 billion committed to OpenAI so far, and they want to make that money back (and then some).
In short, don’t expect ChatGPT search to remain ad-free. That will change significantly at some point.
An important note is that advertising works best on commercial queries.
As you will see later in this article, I scored Google’s performance on commercial queries significantly higher than ChatGPT search.
Understanding user intent
Google has been working on understanding user intent across nearly infinite scenarios since 2004 or earlier.
They’ve been collecting data based on all the user interactions within search and leveraging what they have seen with the Chrome browser since its launch in 2008.
This data has most likely been used to help train Google algorithms to understand user intent and brand authority on a per query basis.
For reference, as of November 2024, Statcounter pegs Chrome’s market share at 67.5%, Safari at 18.2%, and Edge at 4.8%
This is a critical advantage for Google because understanding the user intent of a query is what it’s all about.
You can’t possibly answer the user’s need without understanding their need. As I’ll illustrate in the next section, this is complex!
How query sessions work
Part of the problem with understanding user intent is that the user may not have fully worked out what they’re looking for until they start the process.
Consider the following example of a query sequence that was given to me via Microsoft many years ago:
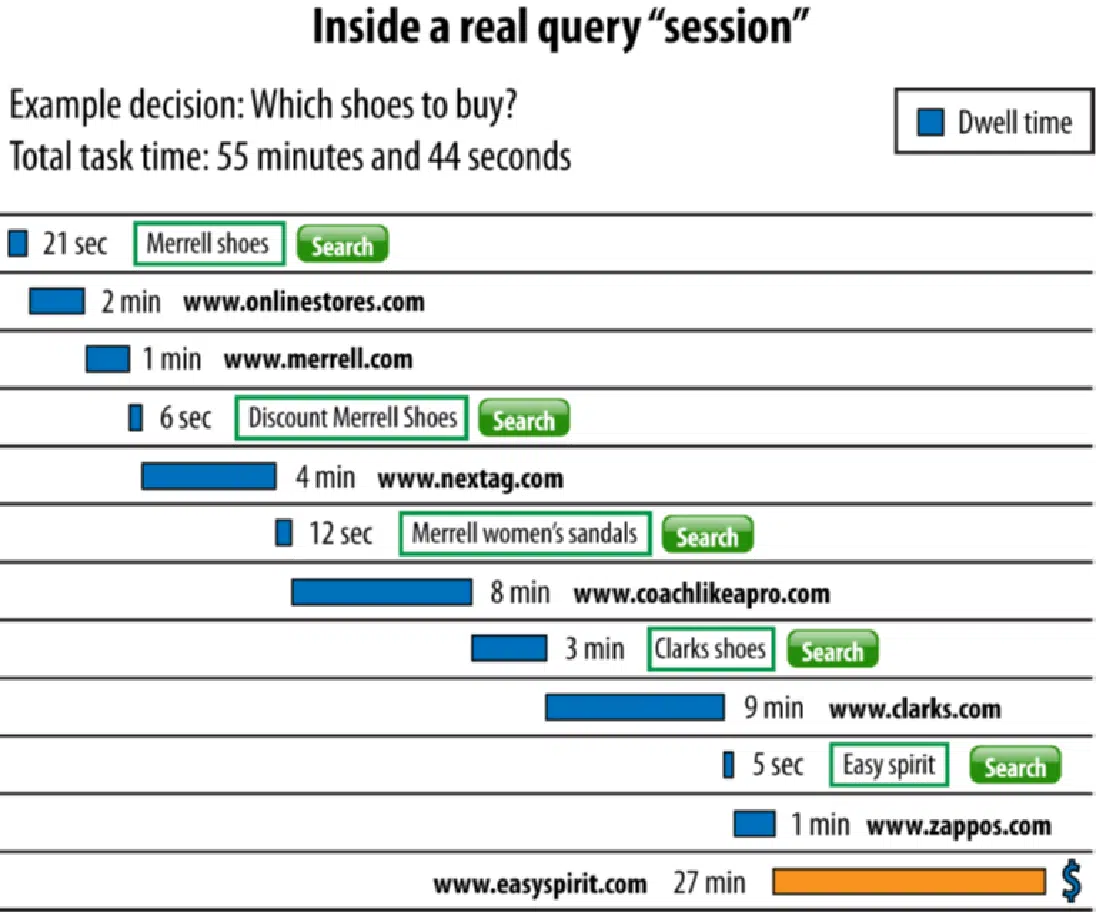
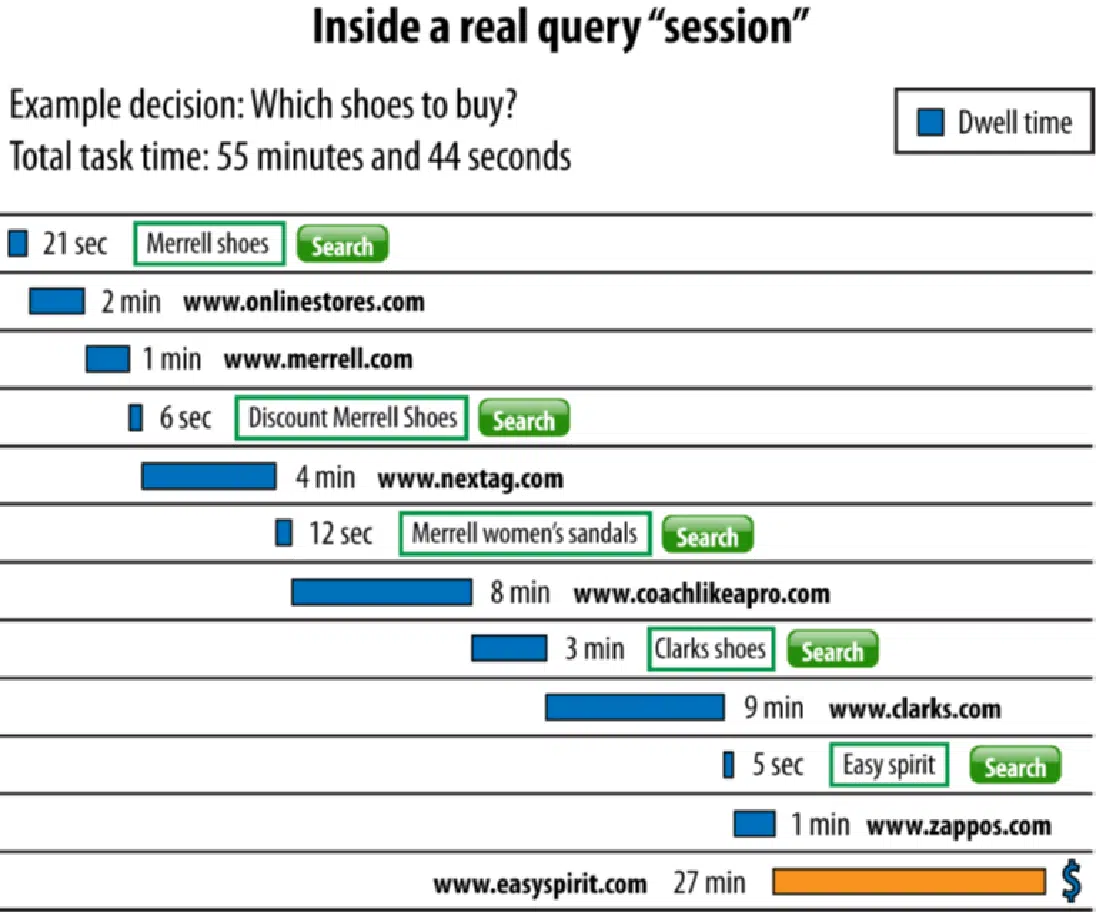
The initial query seems quite simple: “Merrell Shoes.”
You can imagine that the user entering that query often has a specific Merrell shoe in mind, or at least a shoe type, that they want to buy.
However, we see this user’s path has many twists and turns.
For example, the second site they visit is www.merrell.com, a website you might suspect has authoritative information about Merrell shoes.
However, this site doesn’t appear to satisfy the user’s needs.
The user ends up trying four more different queries and visiting six different websites before they finally execute a transaction on www.zappos.com.
This degree of uncertainty in search query journeys is quite common.
Some of the reasons why users have this lack of clarity include is that they:
- Don’t fully understand the need that they’re feeling.
- Don’t know how to ask the right questions to address their need.
- Need more information on a topic before deciding what they need.
- Are in general exploration mode.
Addressing this is an essential aspect of providing a great search experience. This is why the Follow-Up Resources score is part of my analysis.
Understanding categories of queries
Queries can be broadly categorized into several distinct groups, as outlined below:
- Informational: Queries where the user wants information (e.g., “what is diabetes?”).
- Navigational: Queries where the user wants to go to a specific website or page (e.g., “United Mileage Club”).
- Commercial: Queries where the user wants to learn about a product or service (e.g., “Teak dining table”).
- Transactional: Queries where the user is ready to conduct a transaction (e.g., “pizza near me”).
Recent data from SparkToro’s Rand Fishkin provides some insight into the percentage of search queries that fall into each of these categories:
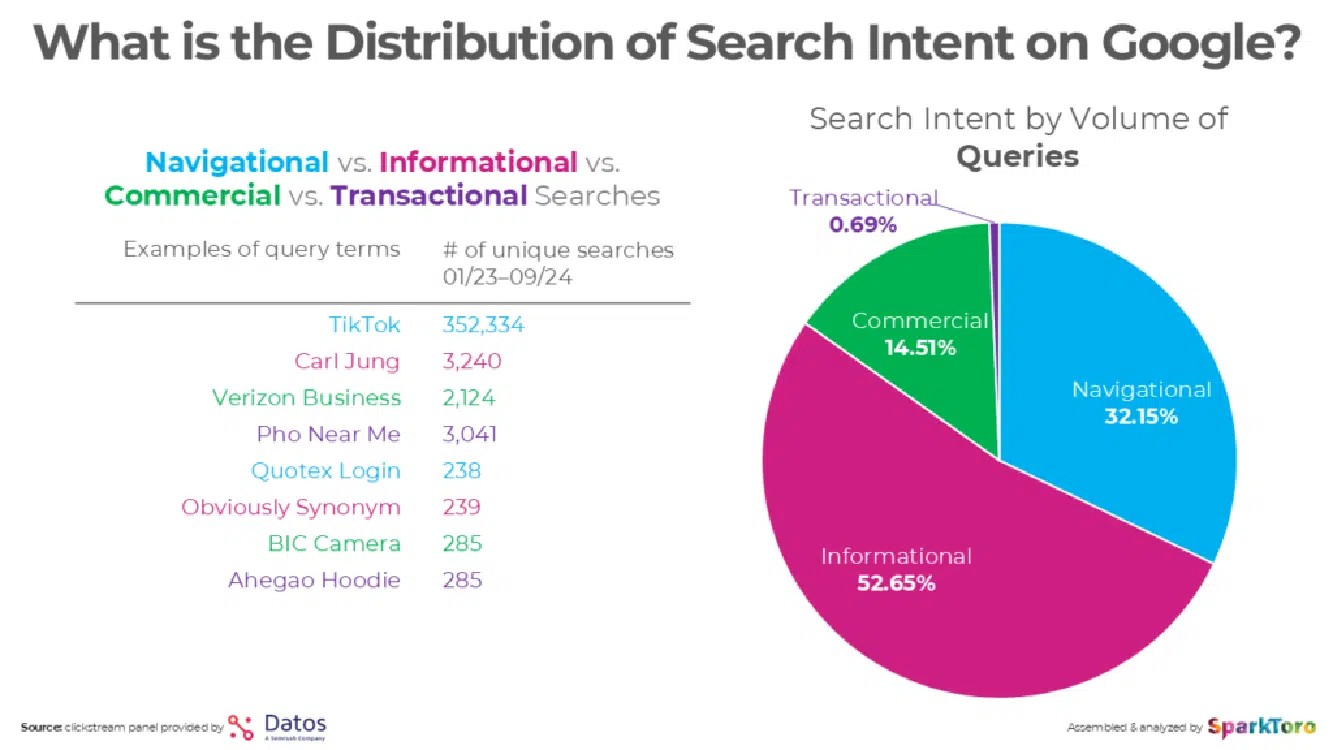
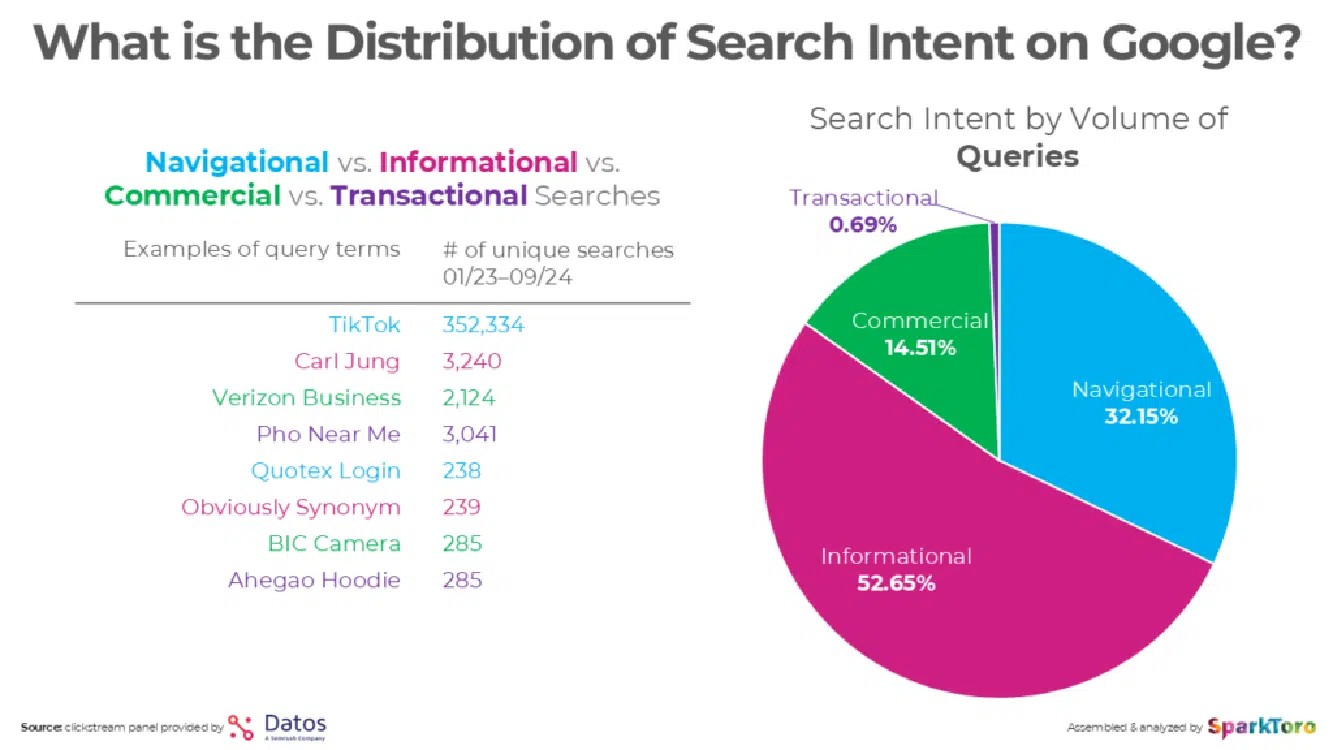
Be advised that the above is a broad view of the categories of queries.
The real work in search relates to handling searches on a query-by-query basis. Each query has many unique aspects that affect how it can be interpreted.
Next, we’ll examine several examples to illustrate this. Then, we’ll compare how ChatGPT search and Google performed on these queries.
Query type: Directions
This query type is a natural strength for Google (as is any locally oriented query). We can see ChatGPT search’s weaknesses in this area in its response:
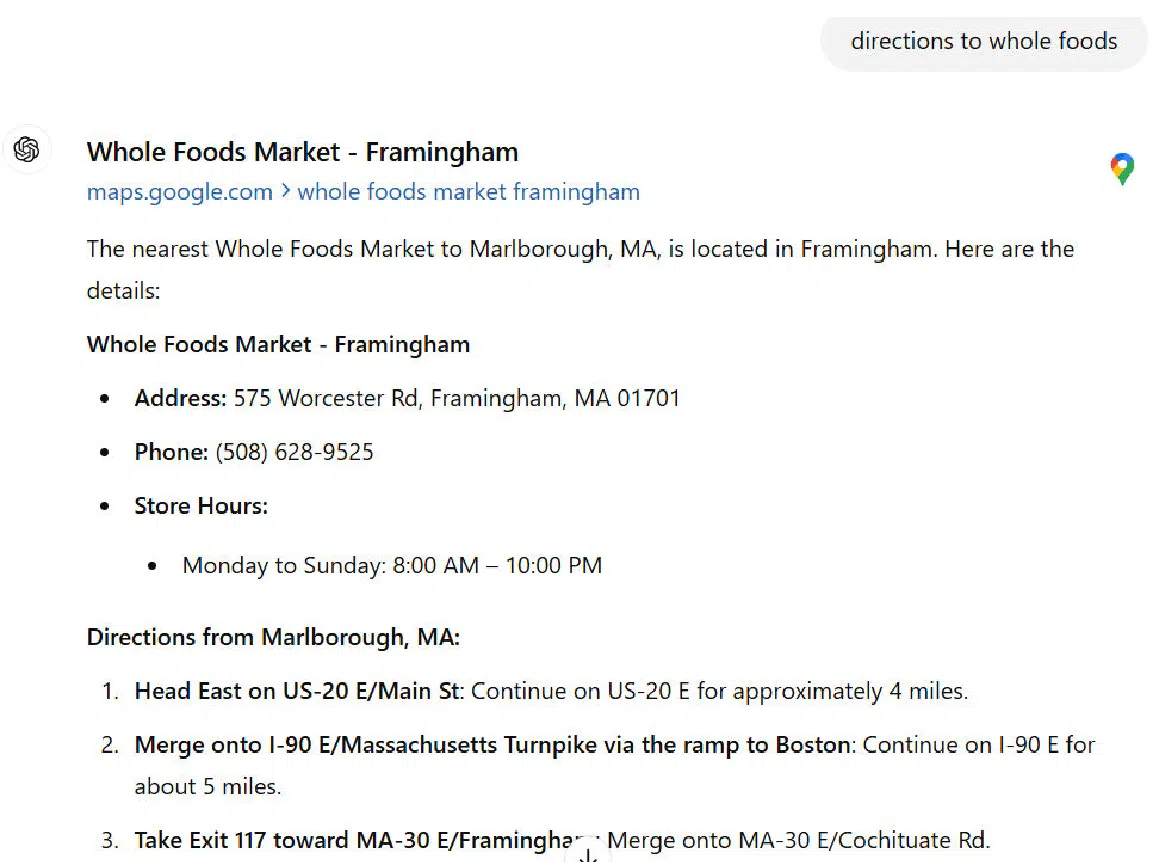
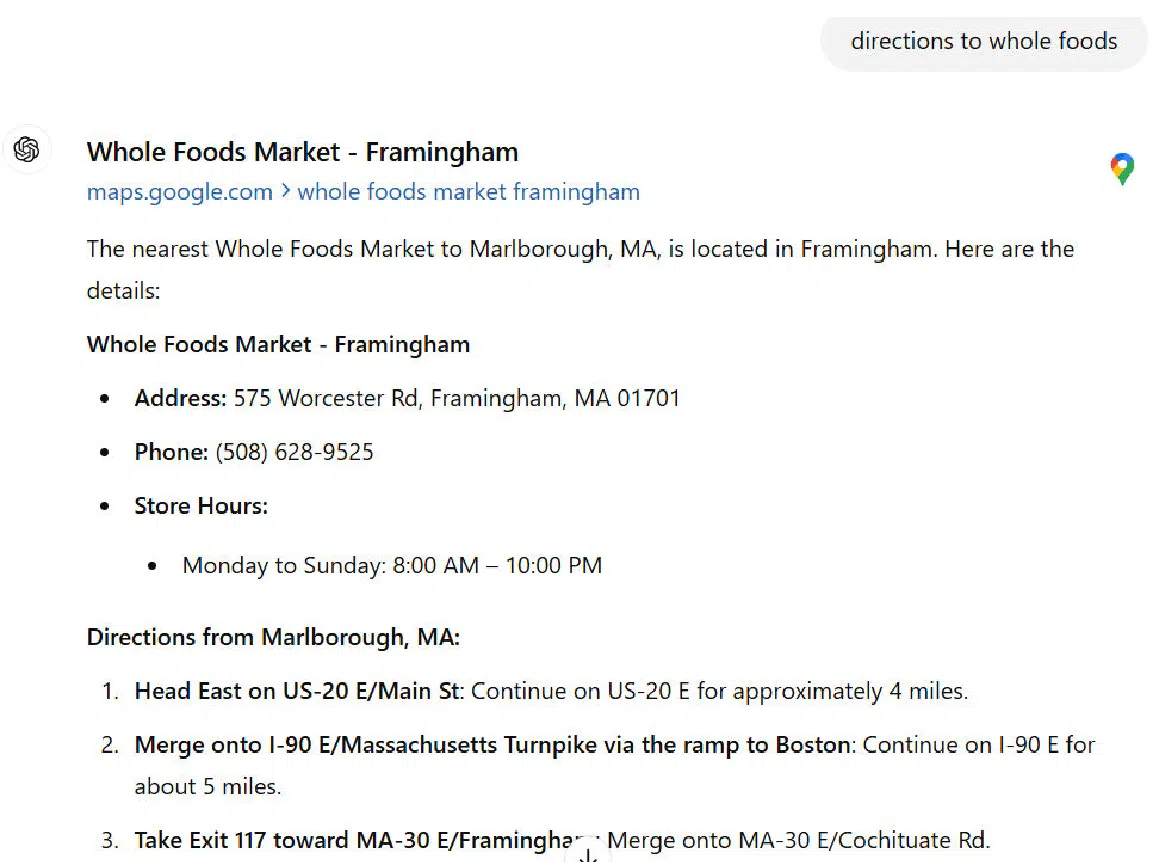
The problems with this response are numerous.
For example, I wasn’t in Marlborough, Massachusetts, when I did the query (I was in the neighboring town of Southborough).
In addition, steps 1 and 2 in the directions are unclear. Anyone following them and heading east on Route 20 would end up at Kenmore Square in Boston without ever crossing I-90 East.
In contrast, Google nails it:
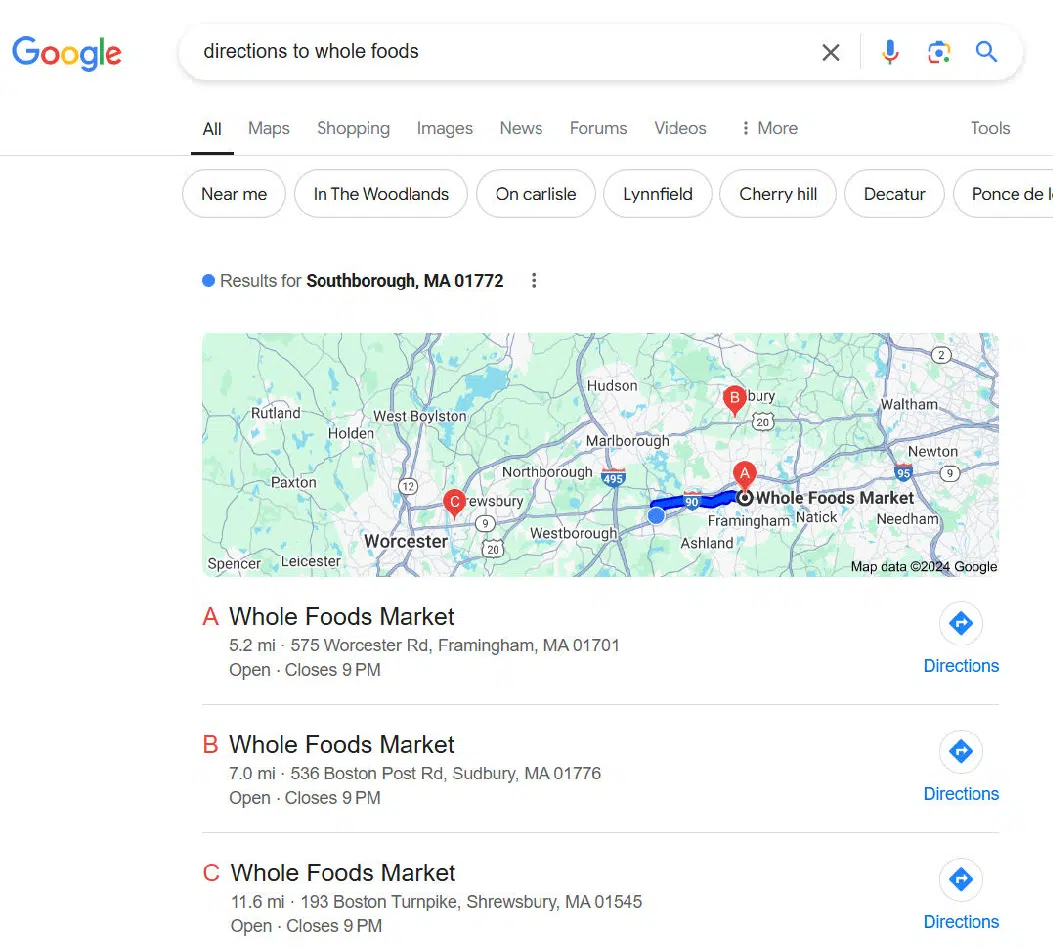
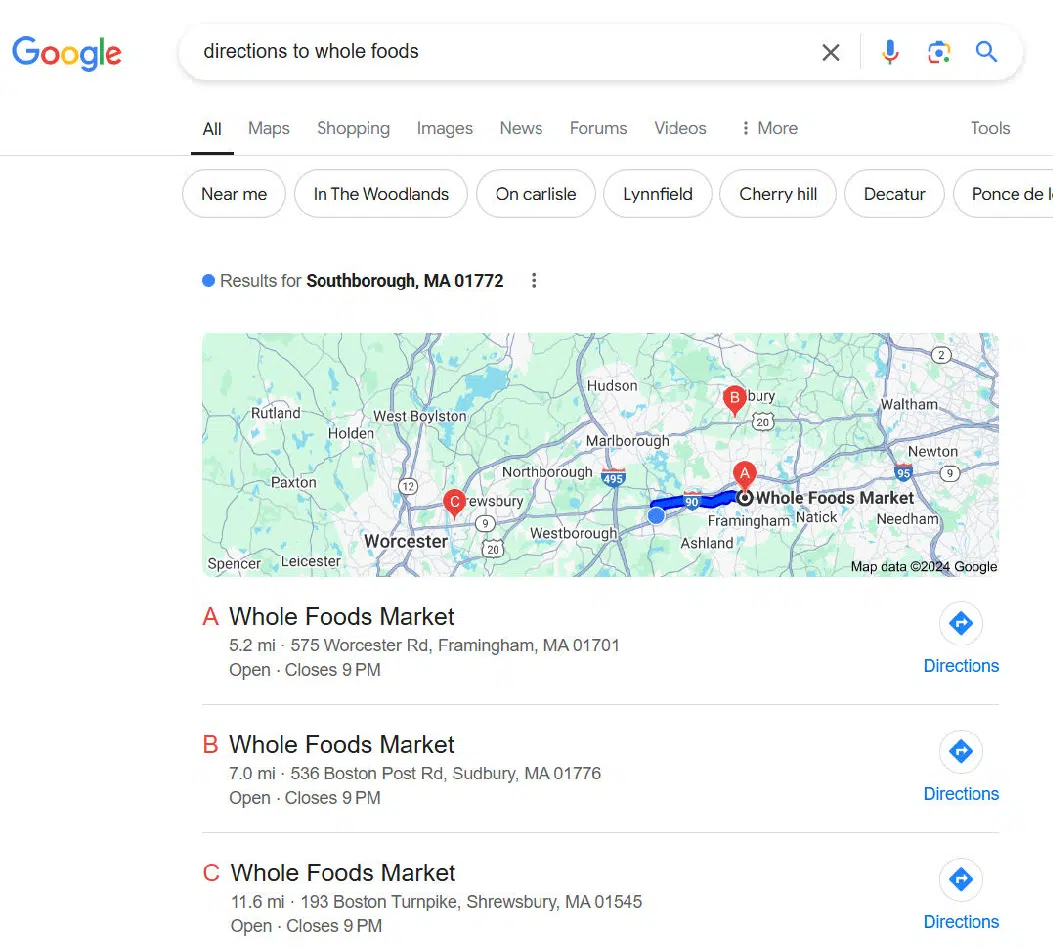
The reason why Google handles this better is simple.
Google Maps has an estimated 118 million users in the U.S., and Waze adds another 30 million users.
I wasn’t able to find a reasonable estimate for Bing Maps, but suffice it to say that it’s far lower than Google’s.
The reason Google is so much better than Bing here is simple – I use Google Maps, and that lets Google know exactly where I am.
This advantage applies to all Google Maps and Waze users in the U.S.
Query type: Local
Other types of local queries present similar issues to those of ChatGPT search. Note that a large percentage of search queries have local intent.
One estimate pegged this at 46% of all queries. This was reportedly shared by a Googler during a Secrets of Local Search conference at GoogleHQ in 2018.
Here is ChatGPT’s response to one example query that I tested:
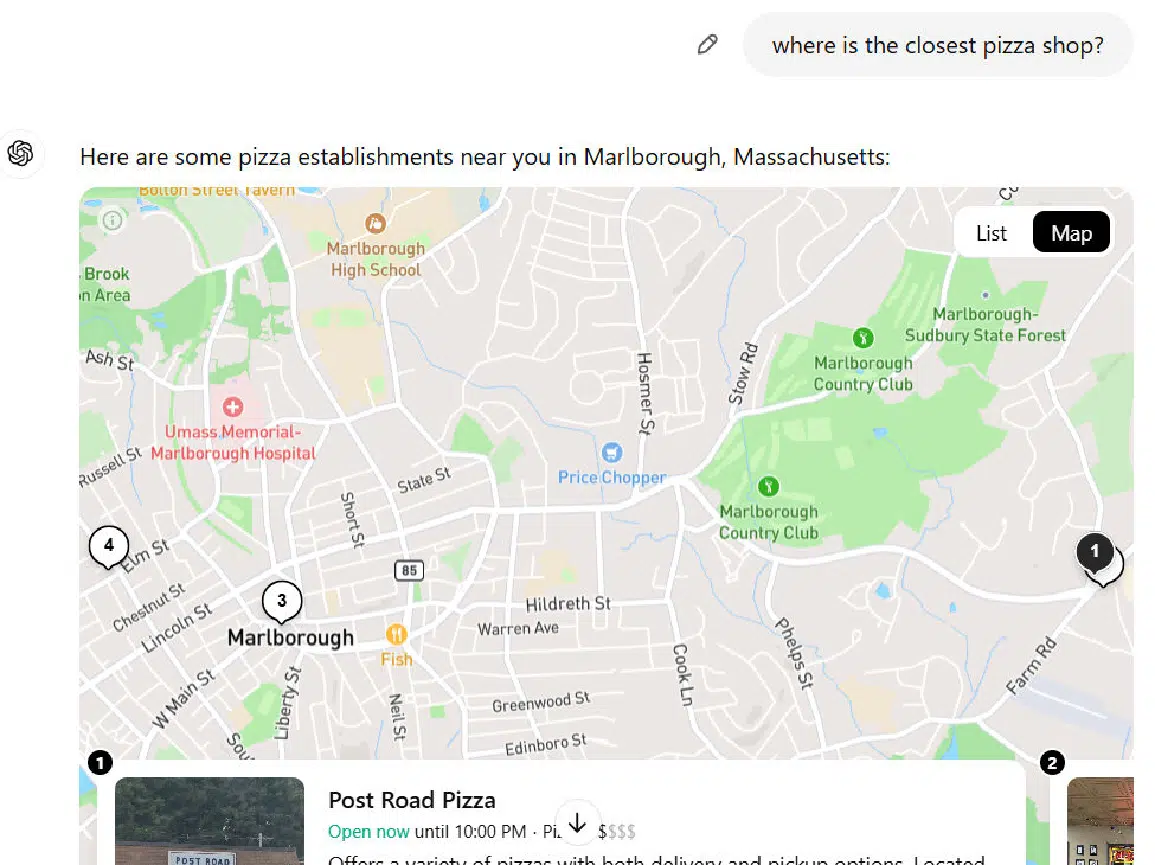
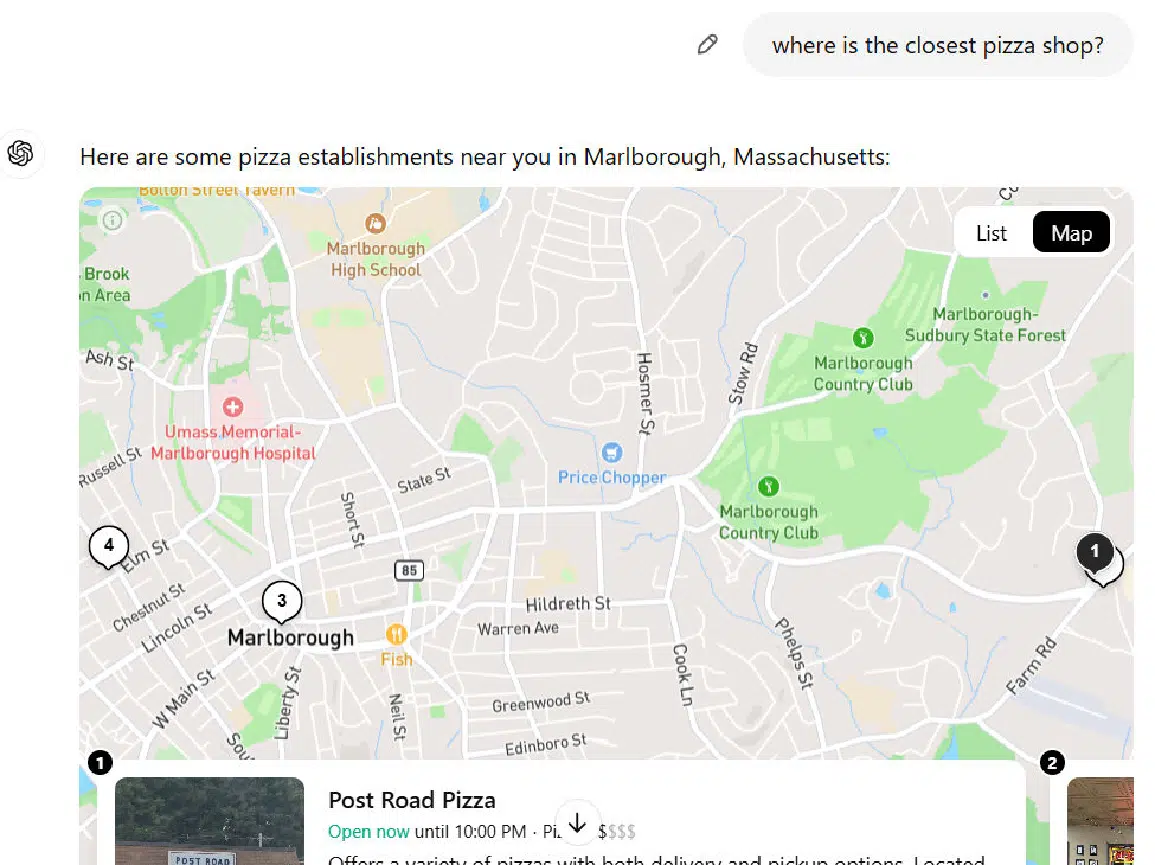
As with the directions example, it thinks that I’m in Marlborough.
In addition, it shows two pizza shops in Marlborough (only one of the two is shown in my screenshot).
Google’s response to this query is much more on point:
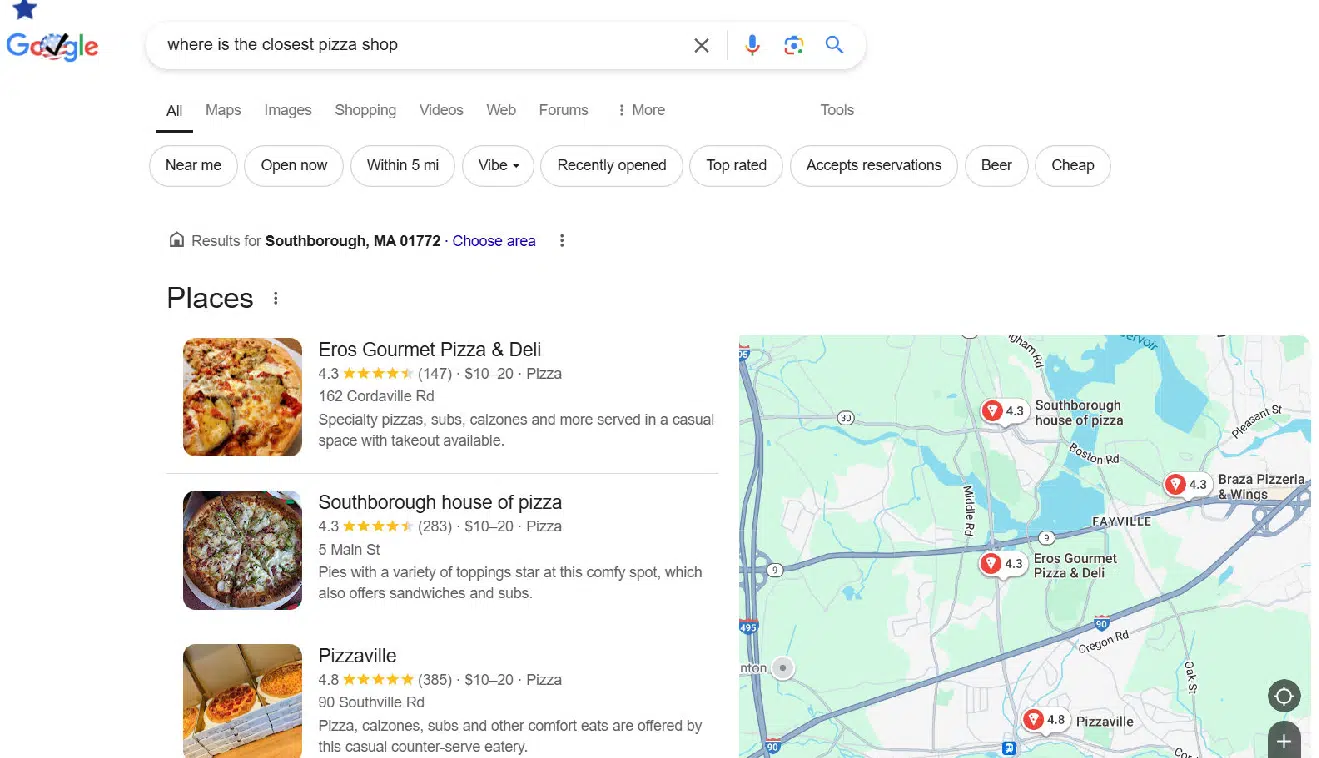
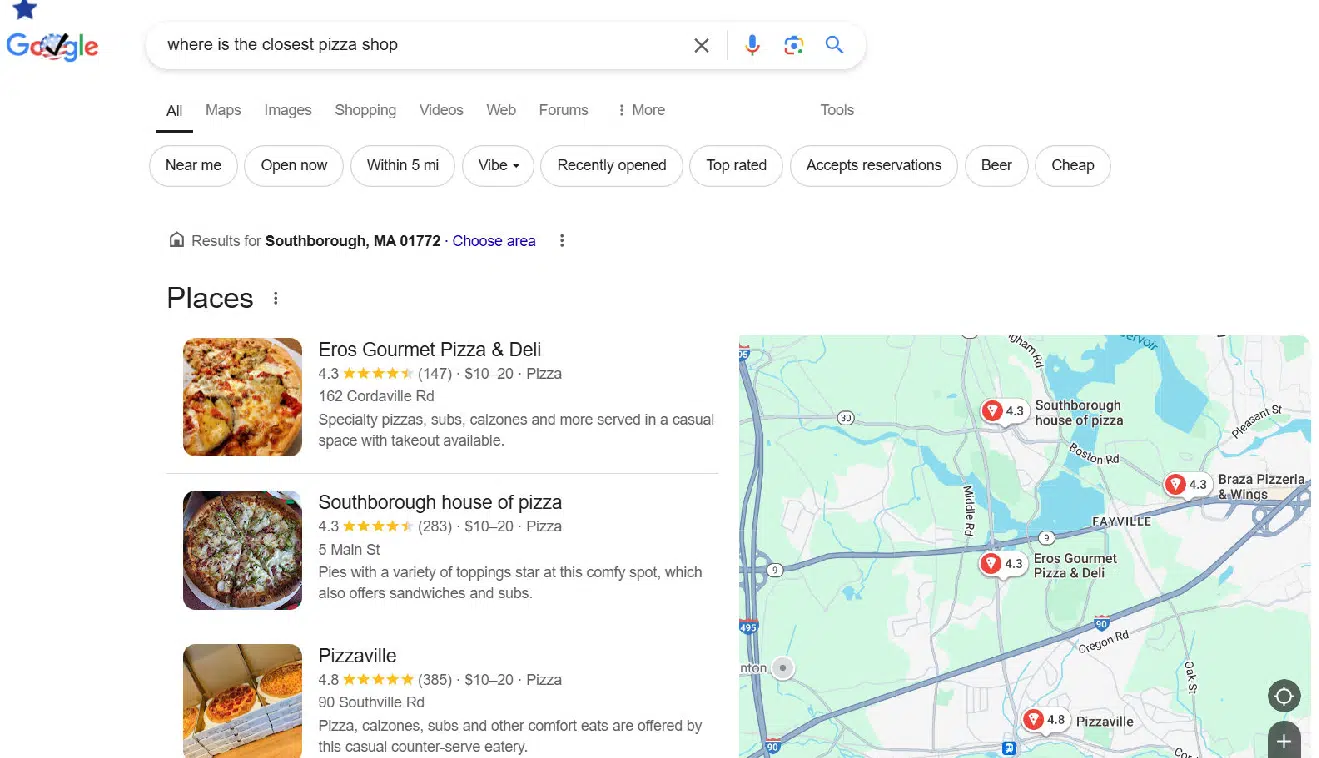
I also gave Google a second version of the query “Pizza shops in Marlborough,” and it returned 11 locations – 9 more than I saw from the ChatGPT search.
This shows us that Google also has far more access to local business data than ChatGPT search.
For this query class (including the Directions discussed previously), I assigned these scores:
- ChatGPT search: 2.00.
- Google: 6.25.
Query type: Content gap analysis
A content gap analysis is one of the most exciting SEO tasks that you can potentially do with generative AI tools.
The concept is simple: provide the tool of your choice a URL from a page on your site that you’d like to improve and ask it to identify weaknesses in the content.
As with most things involving generative AI tools, it’s best to use this type of query as part of a brainstorming process that your subject matter expert writer can use as input to a larger process they go through to update your content.
There are many other different types of content analysis queries that you can do with generative AI that you can’t do with Google (even with AI Overviews) at this point.
For this study, I did four content gap analysis queries to evaluate how well ChatGPT search did with its responses.
Google presented search results related to the page I targeted in the query but did not generate an AI Overview in any of the four cases.
However, ChatGPT search’s responses had significant errors for three of the four queries I tested.
Here is the beginning of ChatGPT search’s response to the one example query where the scope of errors was small:
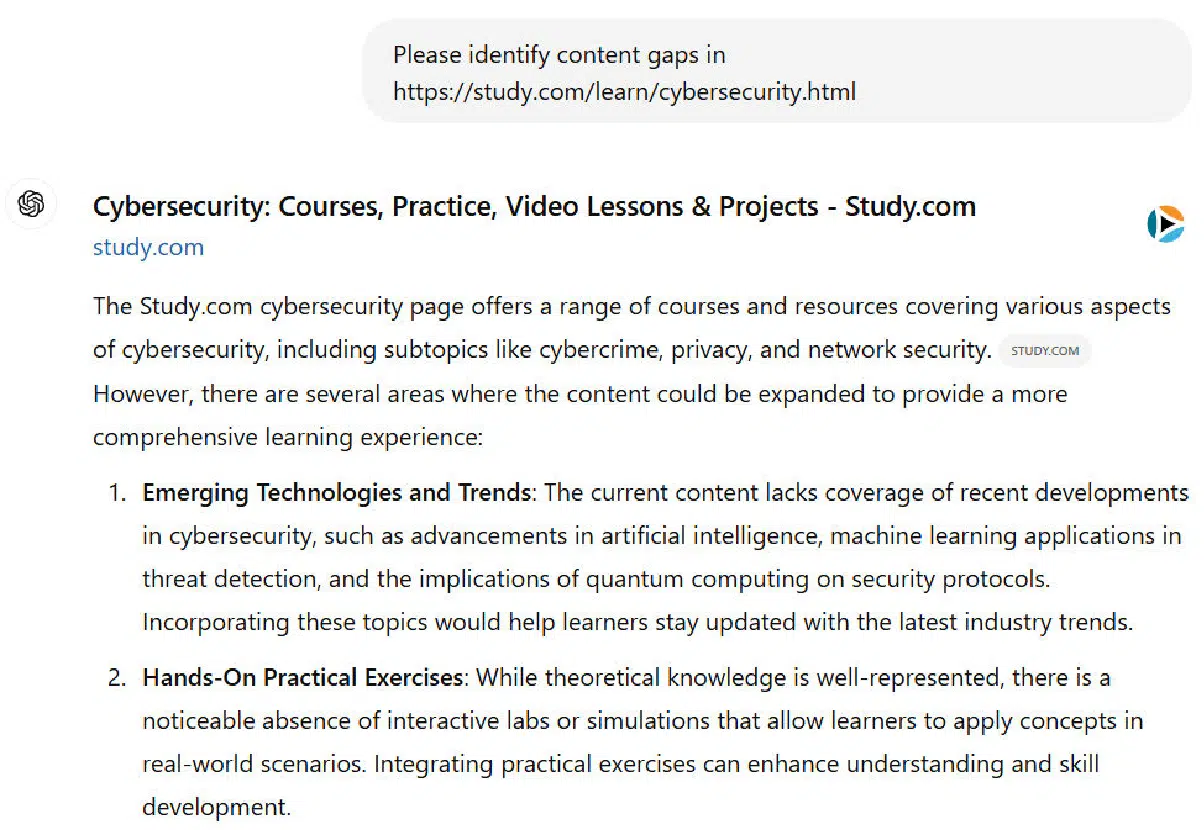
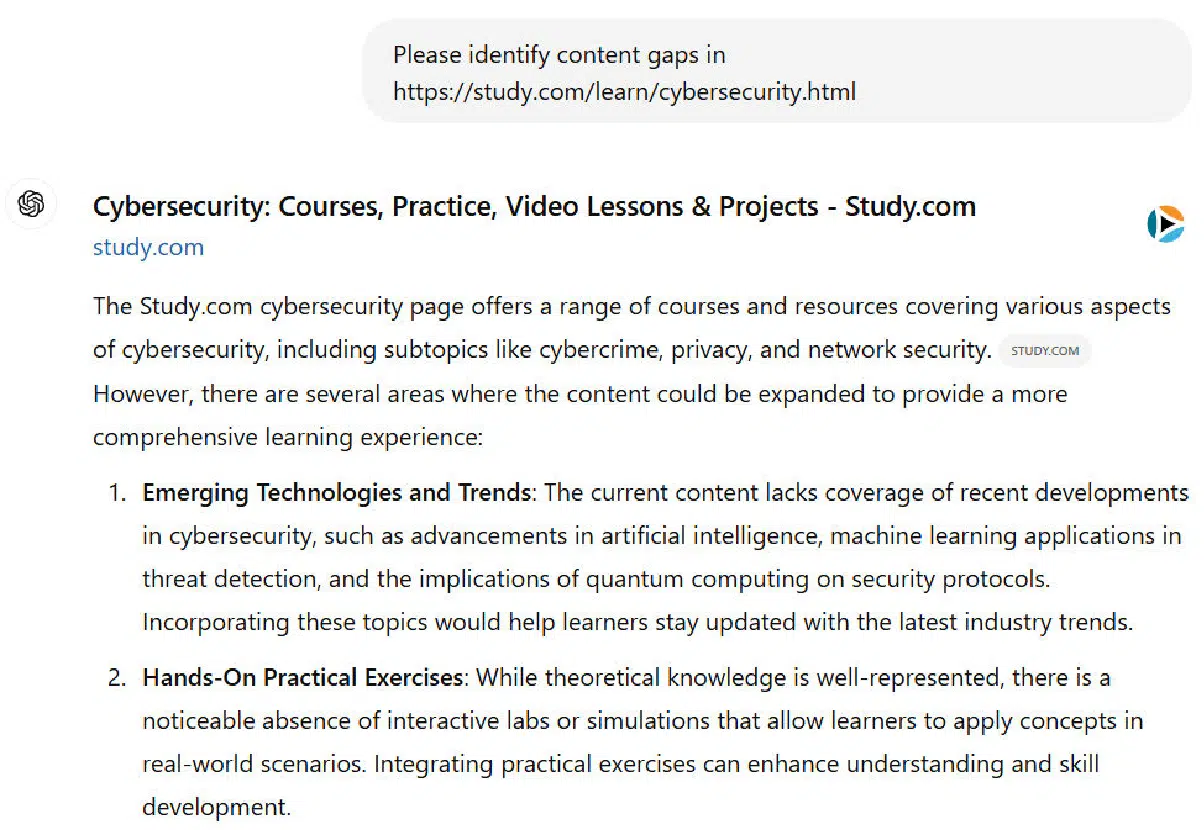
This result from ChatGPT isn’t perfect (there are a few weaknesses, but it’s pretty good. The start of Google’s response to the same query:
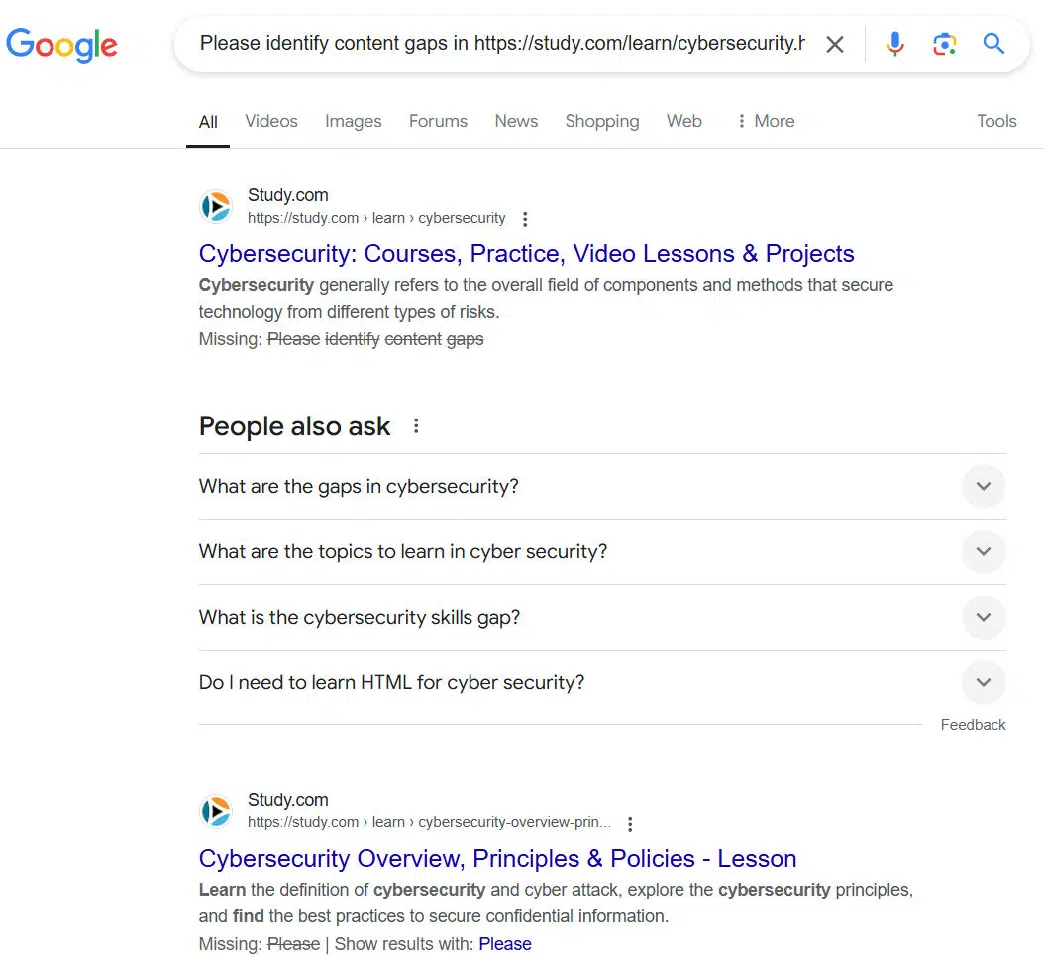
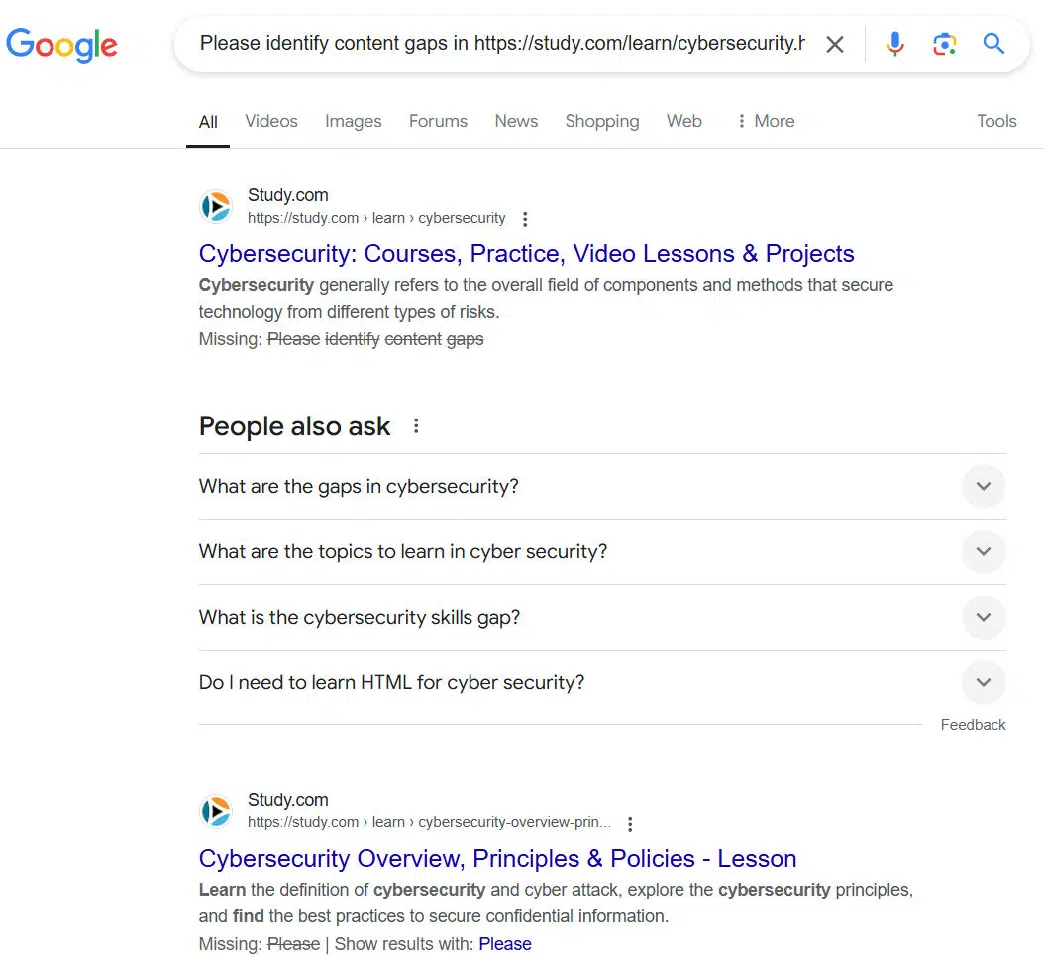
As you can see, Google hasn’t even attempted to perform a content gap analysis. ChatGPT search is better set up to address this type of query.
However, ChatGPT search doesn’t earn a clean sweep for this type of query.
Here is the first part of another example result:
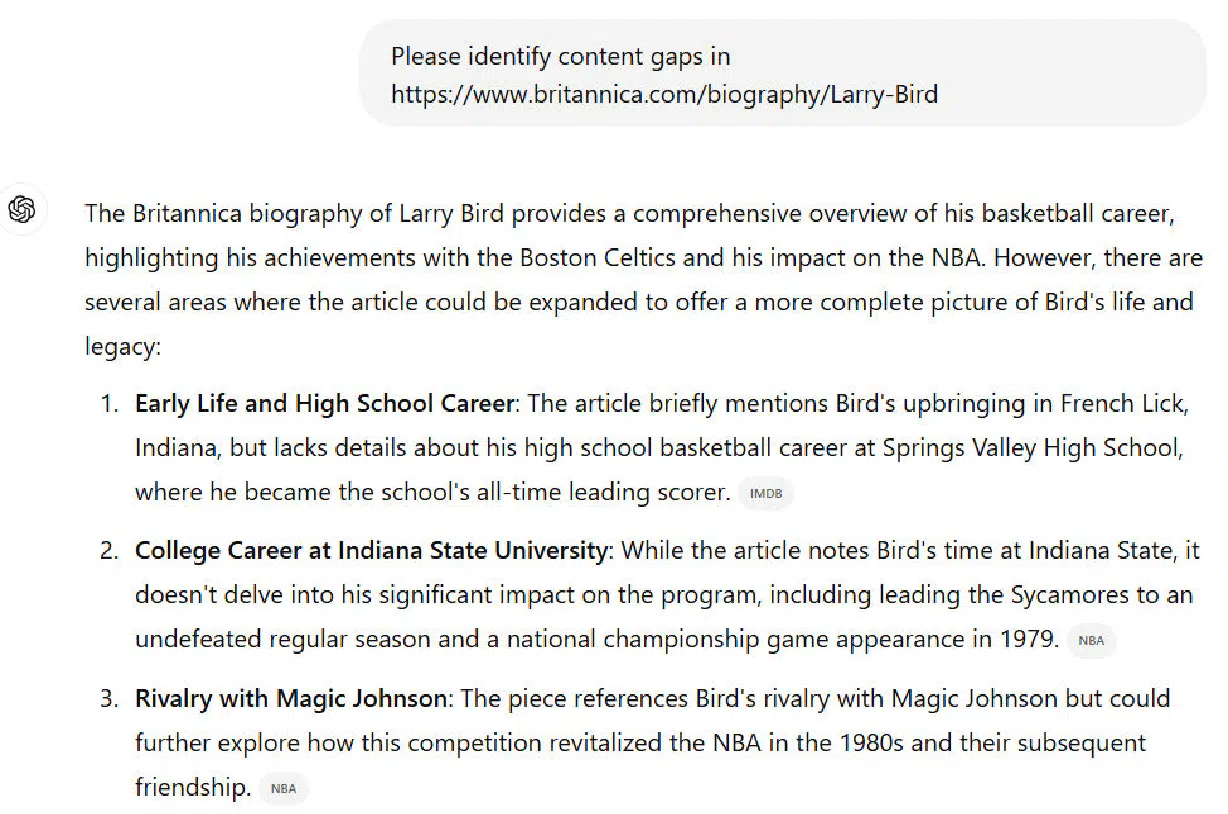
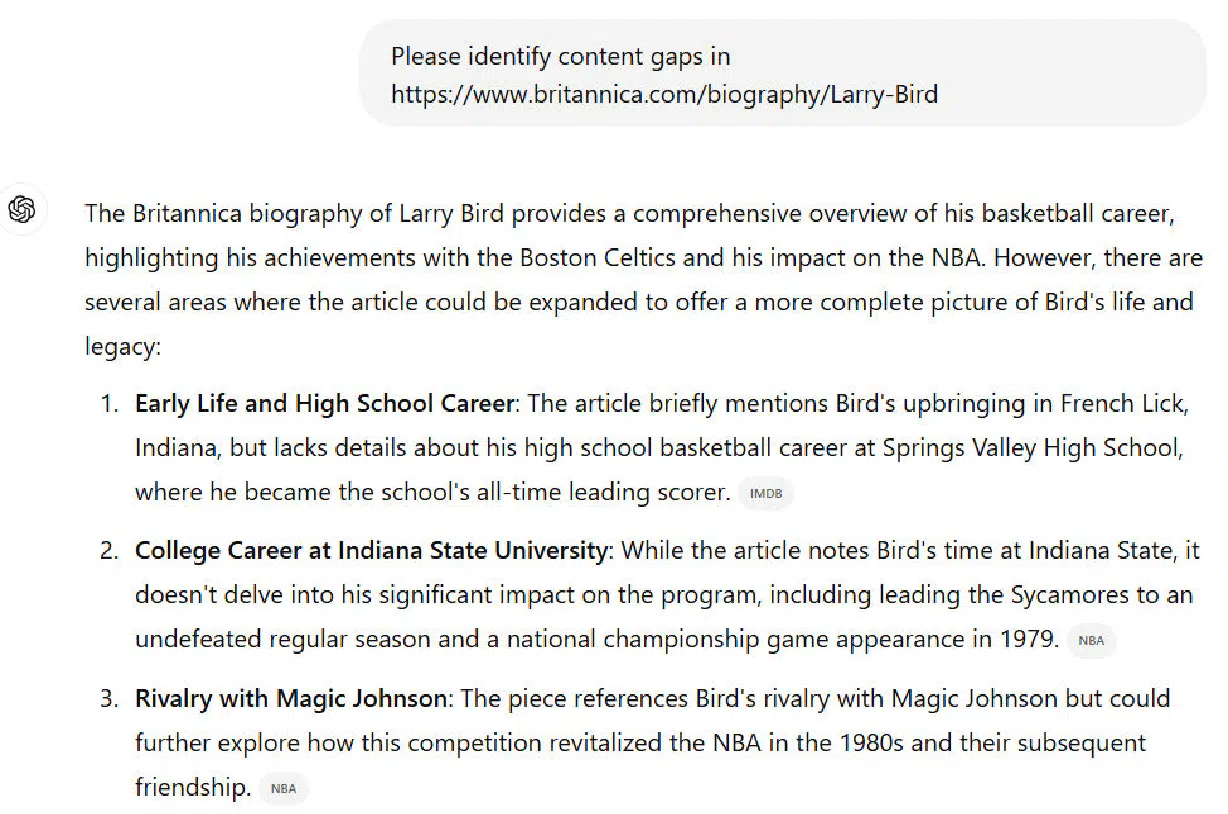
This looks good in principle, but it’s filled with errors. Some of these are:
- The Britannica article does discuss the depth of Larry Bird’s impact on Indiana State University.
- The Britannica article does mention the importance of the Larry Bird / Magic Johnson rivalry to the NBA
- The ChatGPT search response is longer than shown here and there are other errors beyond what I mention here.
Overall, I tried four different content gap analysis queries and ChatGPT search made significant errors in three of them. For this query, I assigned these scores:
- ChatGPT search: 3.25.
- Google: 1.00.
Query type: Individual bio
How these queries perform is impacted by how well-known the person is.
If the person is very famous, such as Lionel Messi, there will be large volumes of material written about them.
If the amount of material written about the person is relatively limited, there is a higher probability that the published online information hasn’t been kept up to date or fact-checked.
We see that in the responses to the query from both ChatGPT search and Google.
Here is what we see from ChatGPT search:
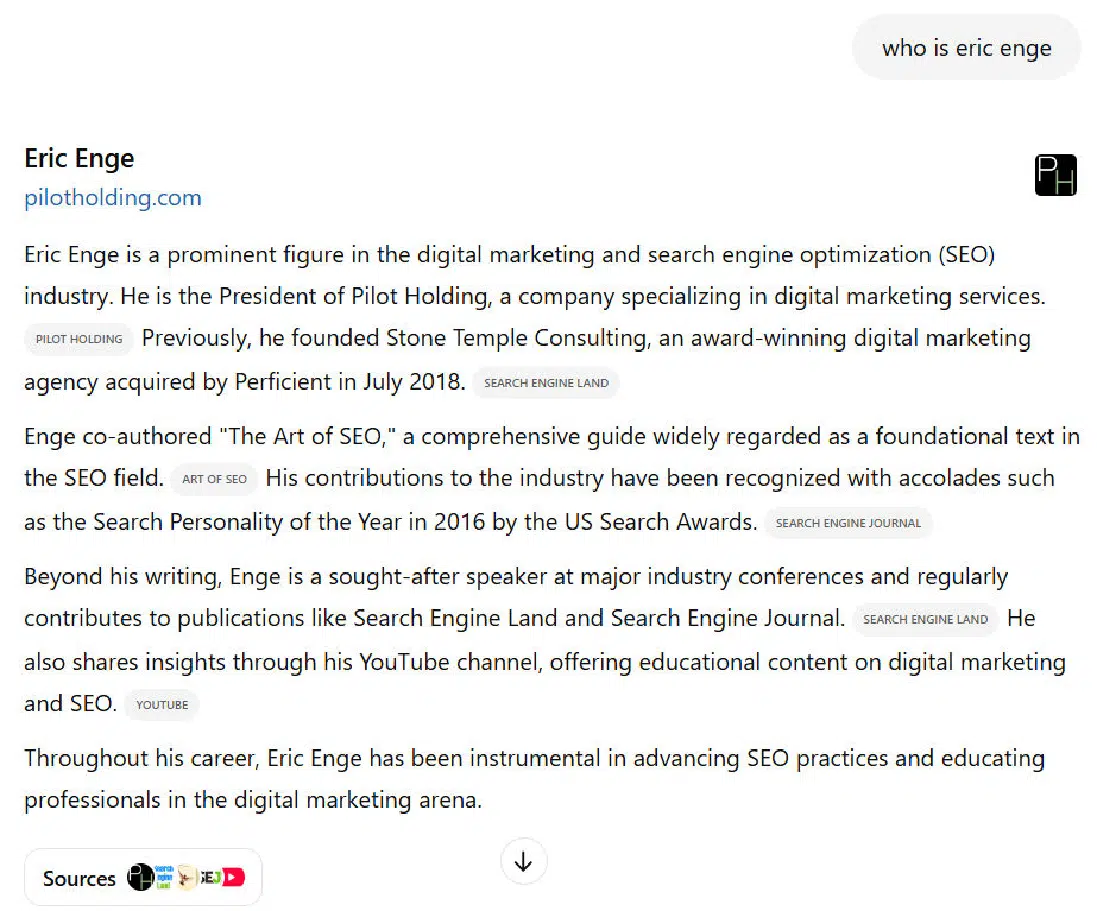
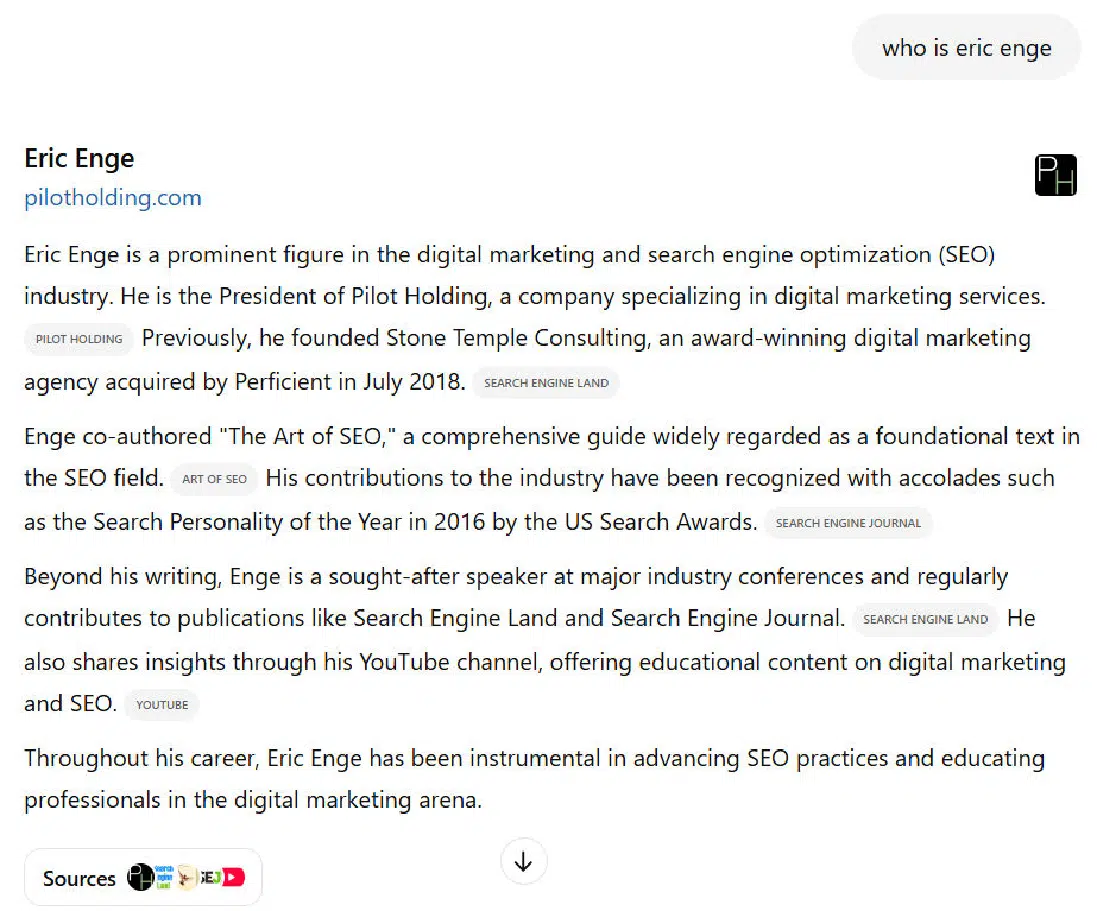
The main issues with this response are in the third paragraph.
I haven’t written for Search Engine Journal in a long time, and it’s also been more than six years since I published a video on my YouTube channel (@stonetemplecons).
Let’s see what Google has to say:
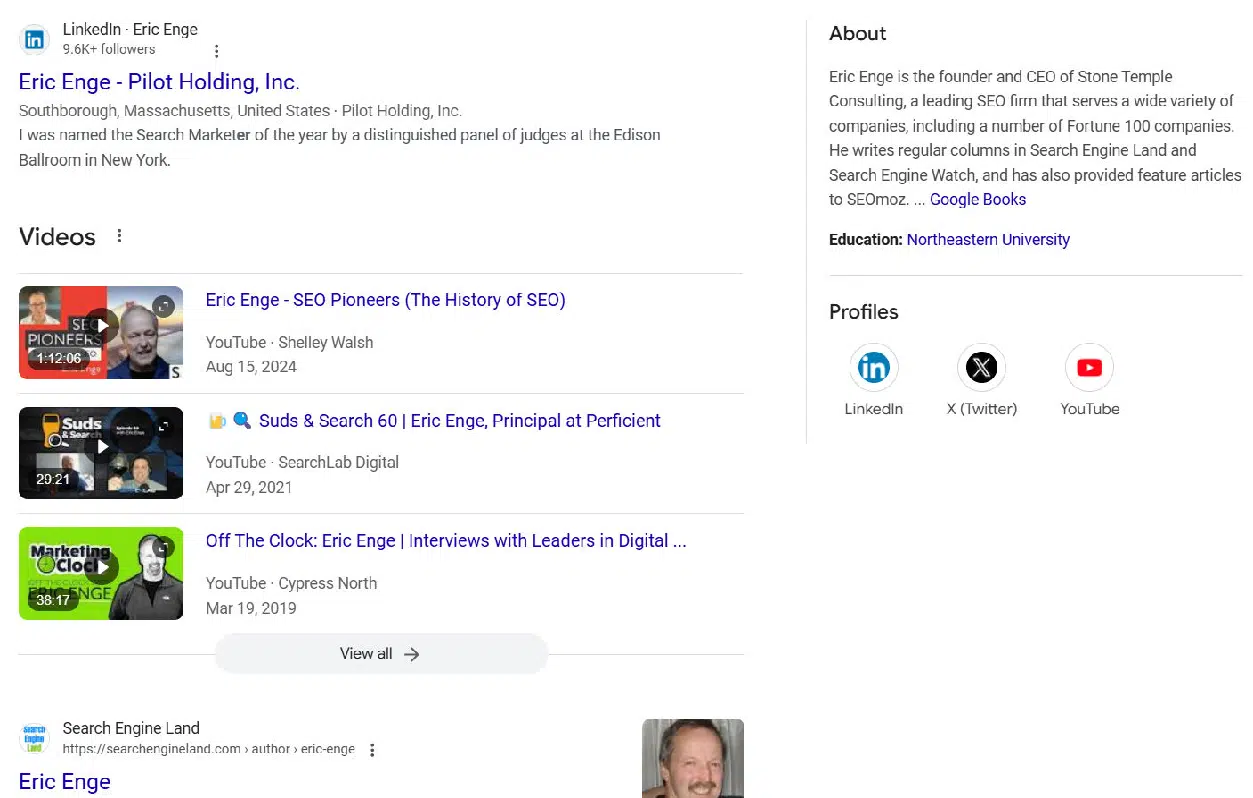
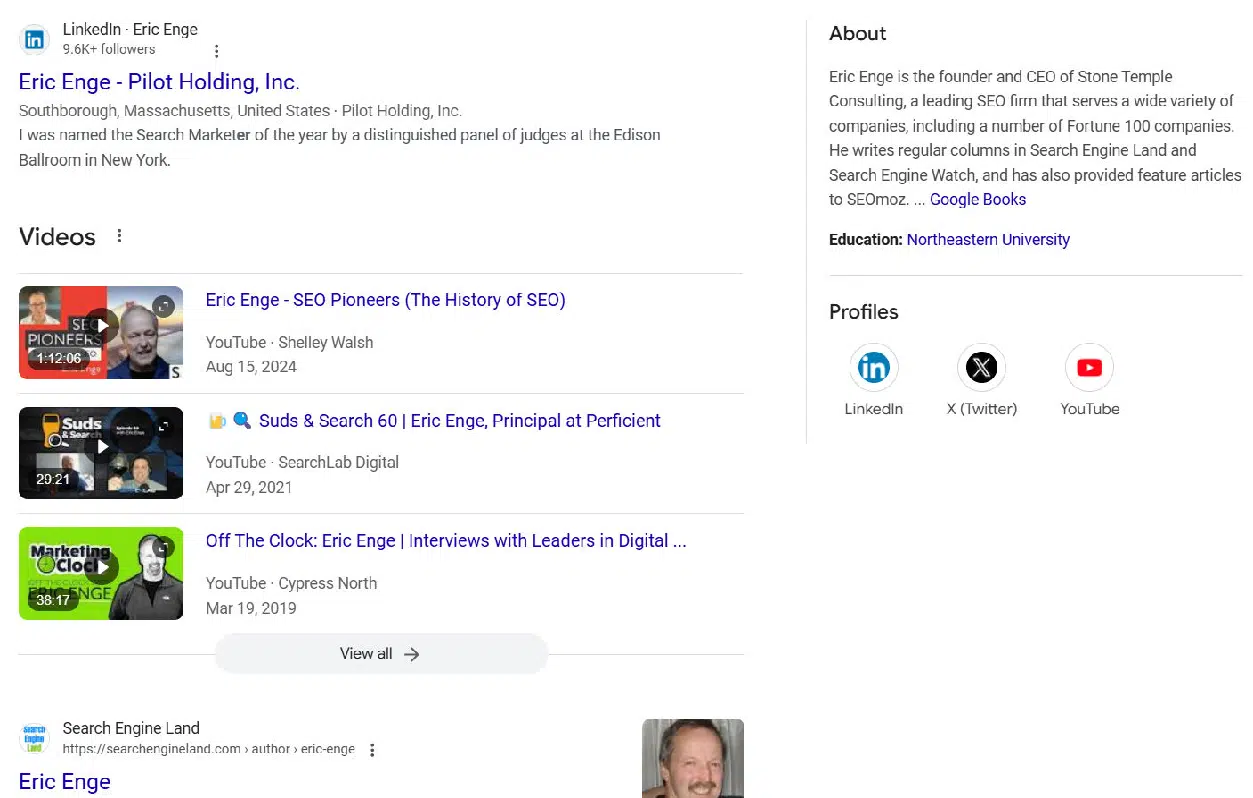
Google also has problems with its response. They lead with quite a few images of me (which are all accurate), and below that, they show my LinkedIn profile and a summary of me drawn from Google Books.
Here, it says that I write for Search Engine Watch (haven’t done that for more than a decade!) and SEOMoz (which rebranded to SEOmoz to Moz in 2013) (also more than a decade!).
These responses are both examples of what I call “Garbage-In-Garbage-Out” queries.
If the web sources aren’t accurate, the tools don’t have the correct information to render.
For bio queries (3 of them), I scored the competitors as follows:
- ChatGPT search: 6.00.
- Google: 5.00.
Query type: Debatable user intent
Arguably, nearly every search query has debatable user intent, but some cases are more extreme than others.
Consider, for example, queries like these:
- Diabetes.
- Washington Commanders.
- Physics.
- Ford Mustang.
Each of these examples represents an extremely broad query that could have many different intents behind it.
In the case of diabetes:
- Does the person just discover that they have (or a loved one has) diabetes, and they want a wide range of general information on the topic?
- Are they focused on treatment options? Long-term outlook? Medications? All of the above?
Or, for a term like physics:
- Do they want a broad definition of what it’s about?
- Or is there some specific aspect of physics that they wish to learn much more about?
Creating the best possible user experience for queries like these is tricky because your response should provide opportunities for each of the most common possible user intents.
For example, here is how ChatGPT responded to the query “physics”:
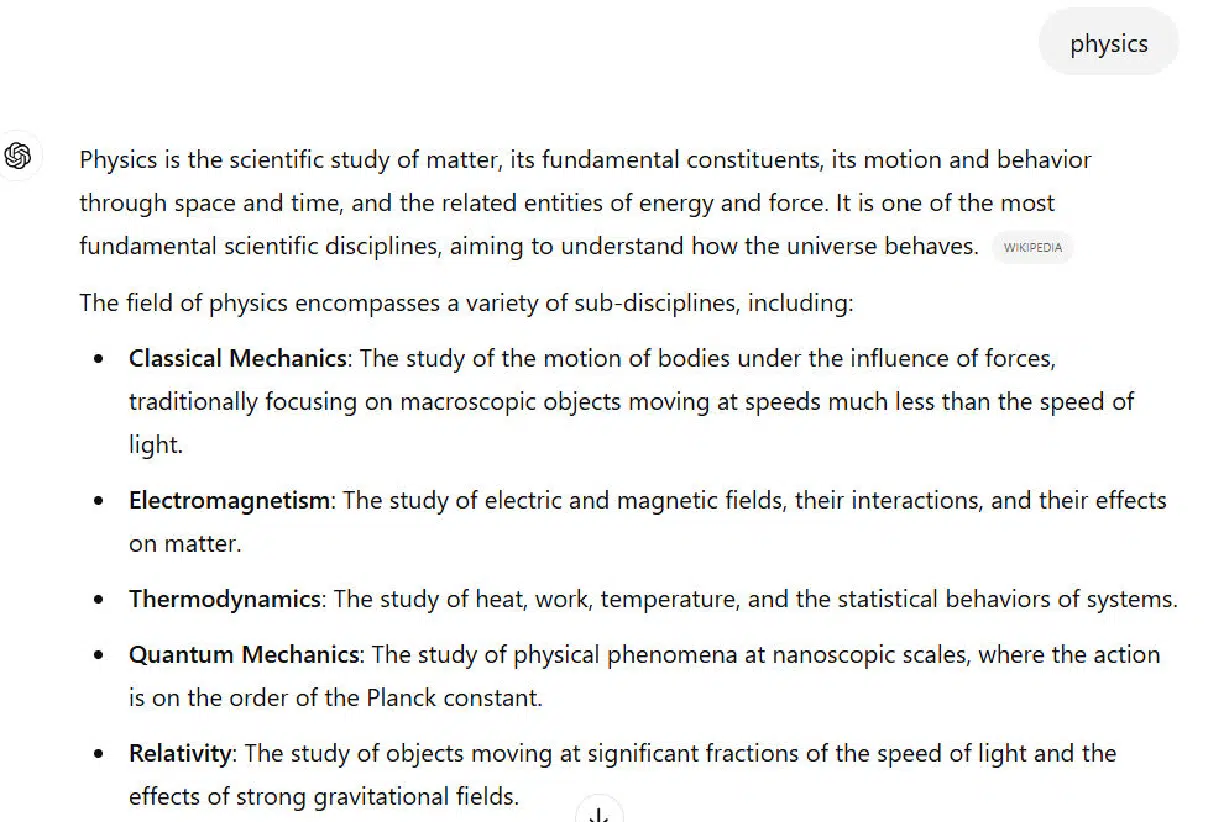
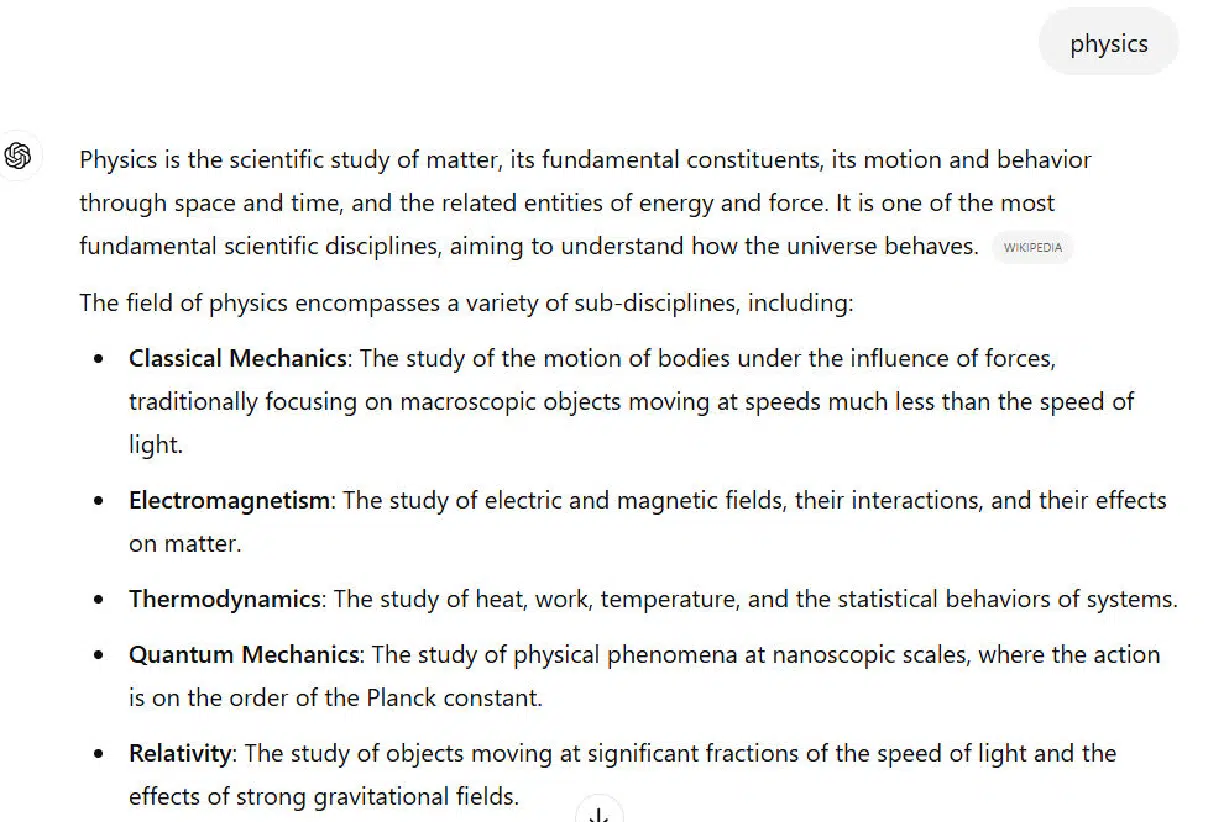
The additional two paragraphs of the response focused on the definition of Physics and kept the response at a very high level.
In contrast, the beginning of Google’s response also focuses on a broad definition of physics, but following that are People Also Ask and Things to Know boxes that address many other potential areas of interest to people who type in this search query:
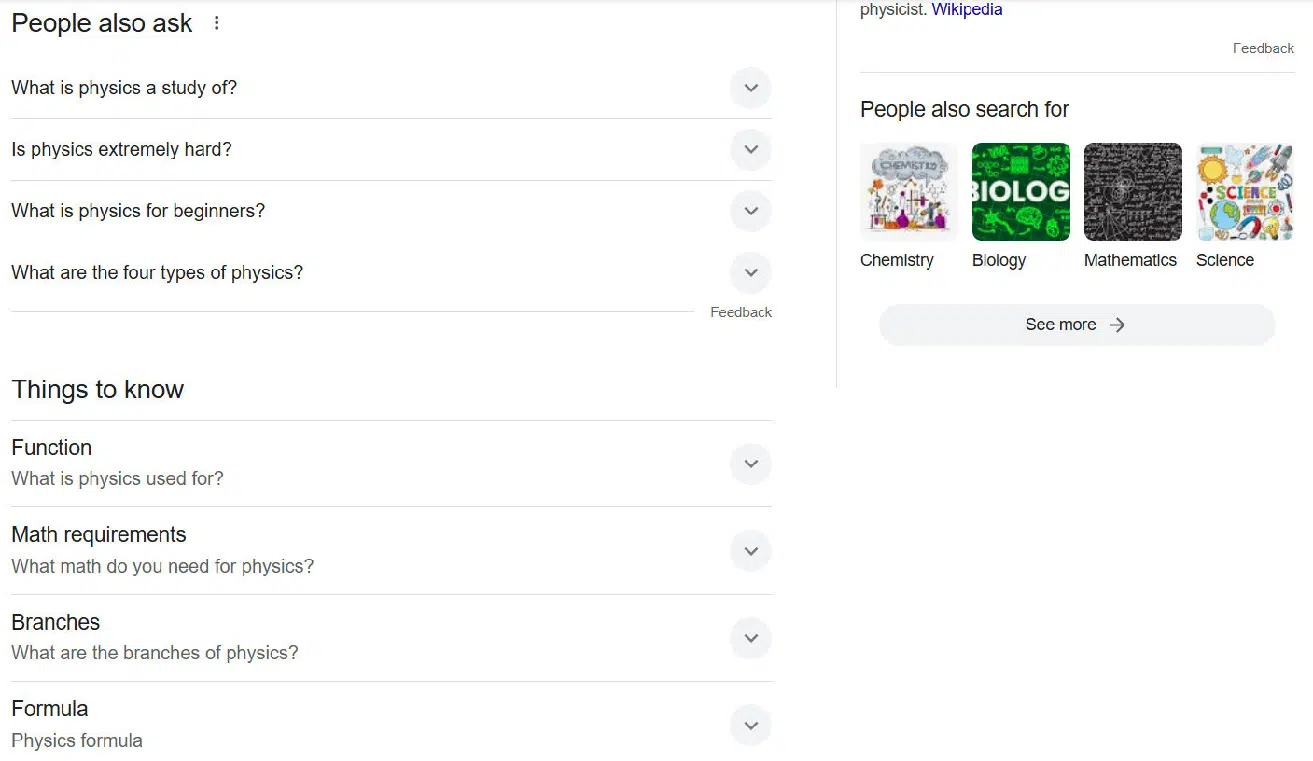
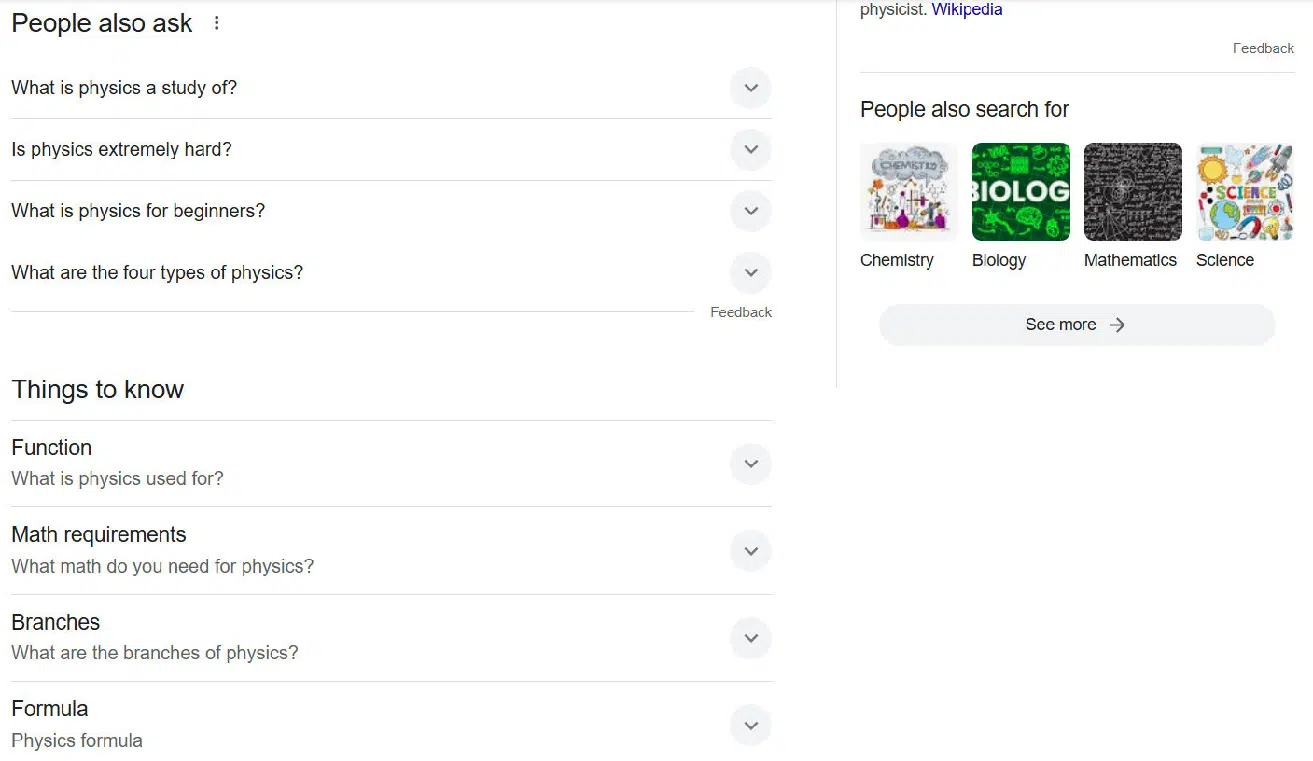
This part of Google’s response shows a recognition of the many possible intents that users who type in the phrase “physics” may have in mind.
For this query, I assigned these scores:
- ChatGPT search: 5.00.
- Google: 7.00.
Query type: Disambiguation
One special class of debatable intents queries is words or phrases that require disambiguation. Here are some example queries that I included in my test set:
- Where is the best place to buy a router?
- What is a jaguar?
- What is mercury?
- What is a joker?
- What is a bat?
- Racket meaning.
For example, here is how ChatGPT search responded to the question, “What is a joker query?”
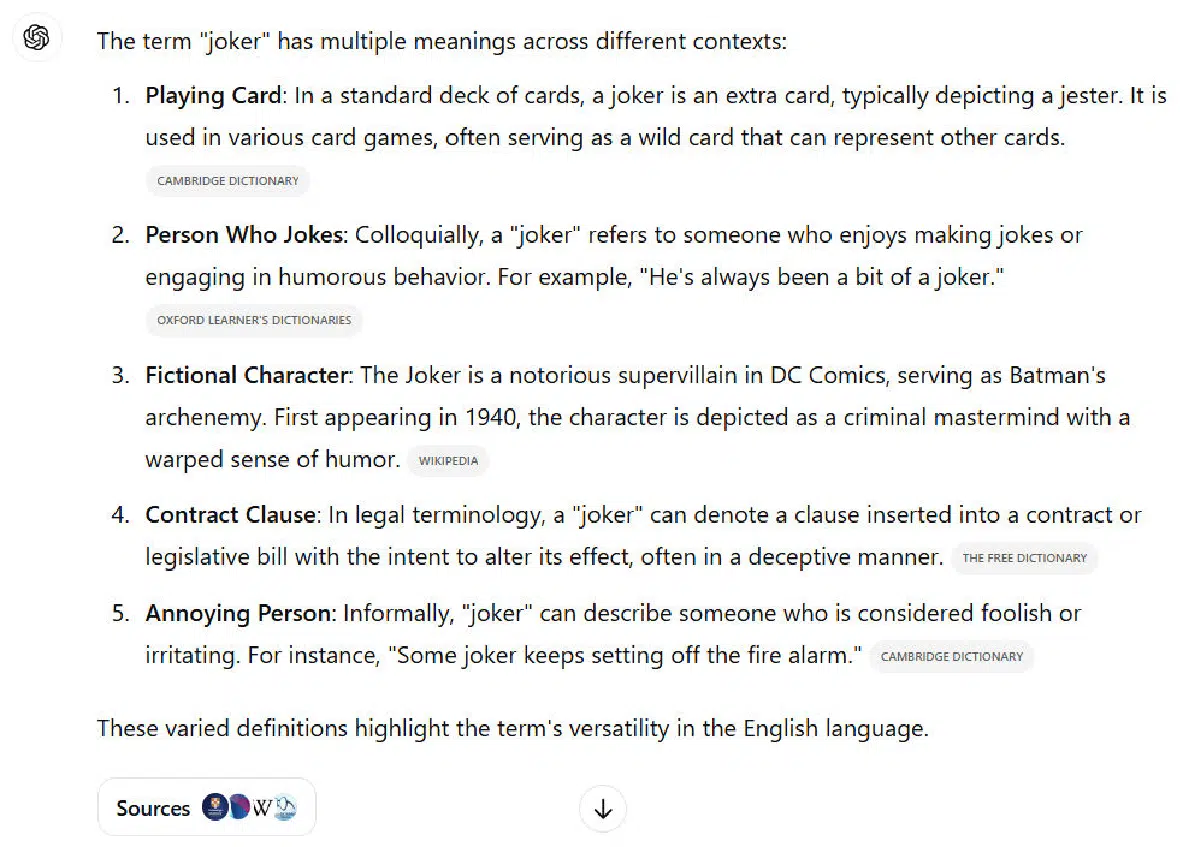
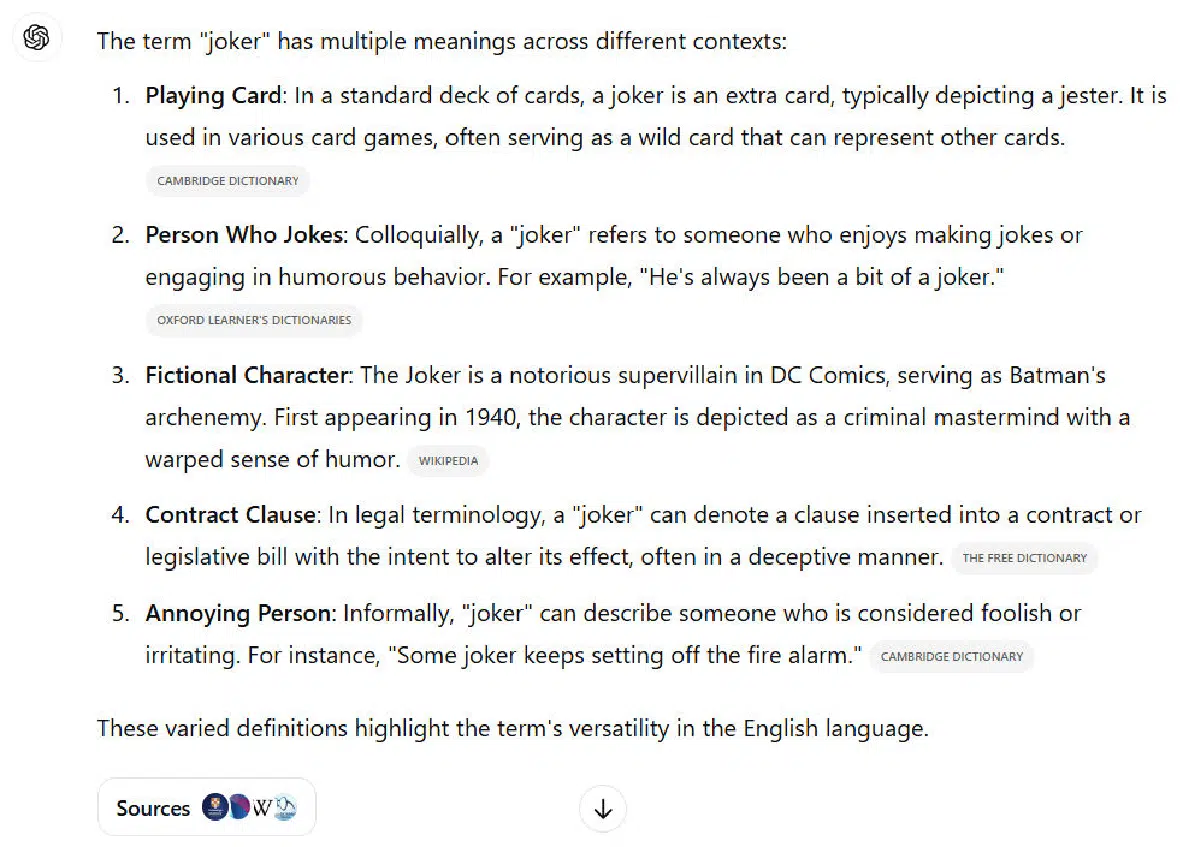
We can see that it offers a nice disambiguation table that provides a brief definition for five different meanings of the term.
It also includes links to pages on the web that users can visit for information related to each meaning.
In contrast, Google focuses on two major intents:
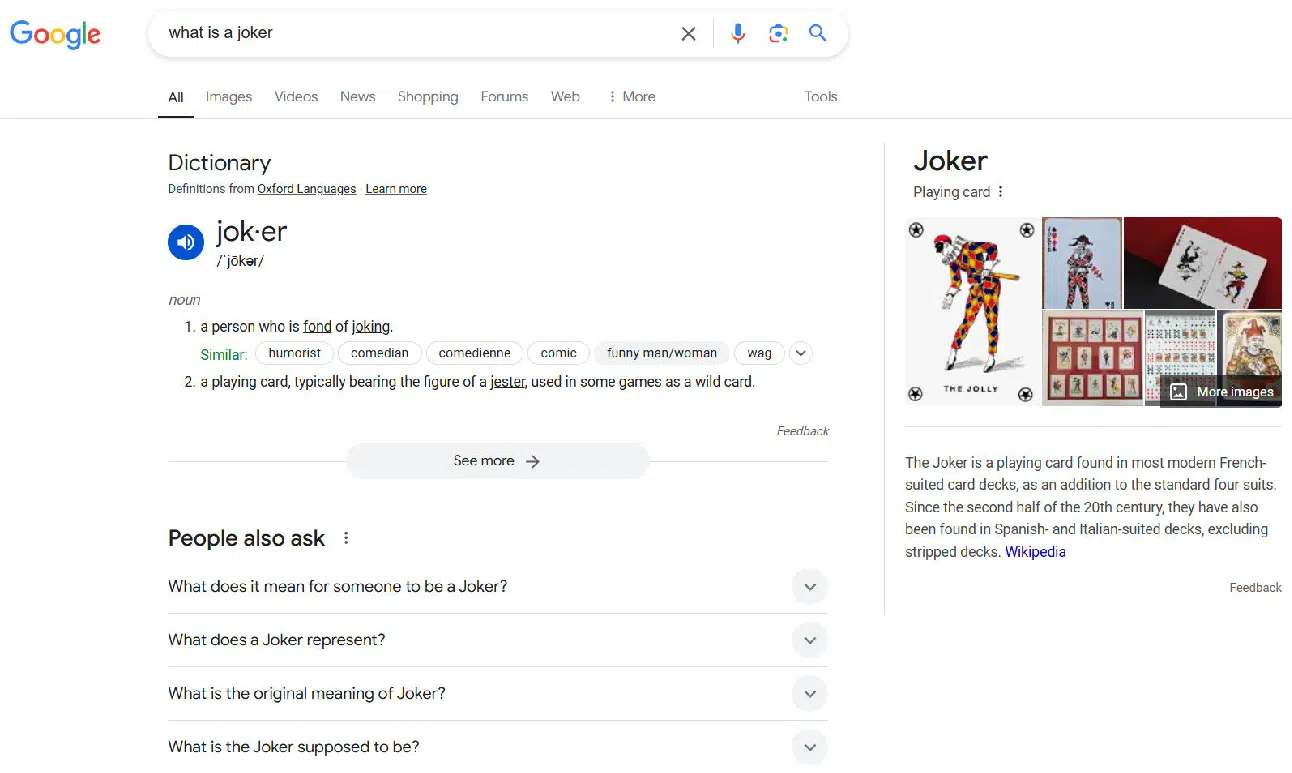
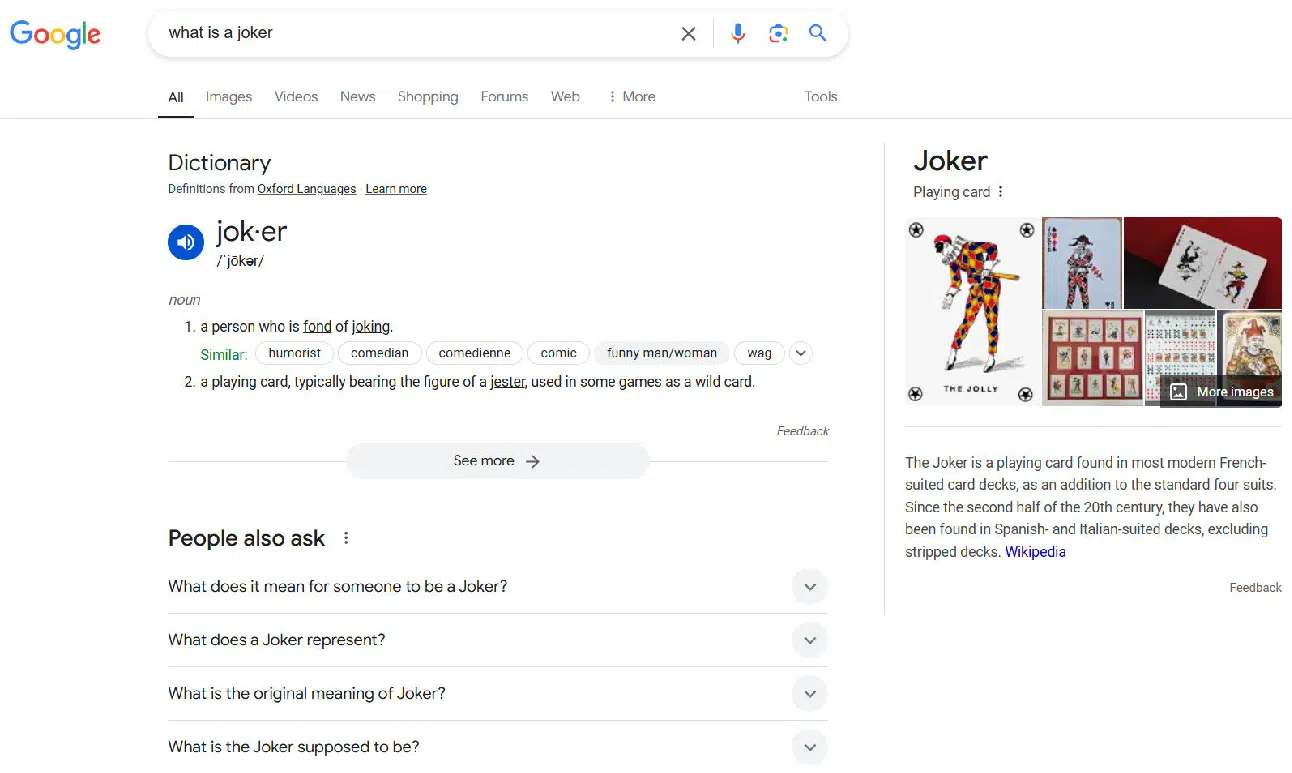
Google’s focus is on the playing card and a person who tells a lot of jokes.
Following this part of the SERP, Google continues this approach with websites focusing on these two definitions.
This means that someone who’s interested in the word “joker” as it applies to contract clauses will have to do an additional search to find what they were looking for (e.g., “meaning of joker when referring to contract clauses”).
Which is better?
Well, it depends.
If the searchers interested in playing cards or people who tell lots of jokes make up more than 90% of the people who enter this search query, then the Google result might be the better of the two.
As it is, I scored the ChatGPT search result a bit higher than Google’s for this query.
Another example of disambiguation failure is simply not addressing it at all. Consider the query example: “where is the best place to buy a router?”
Here is how ChatGPT search addressed it:
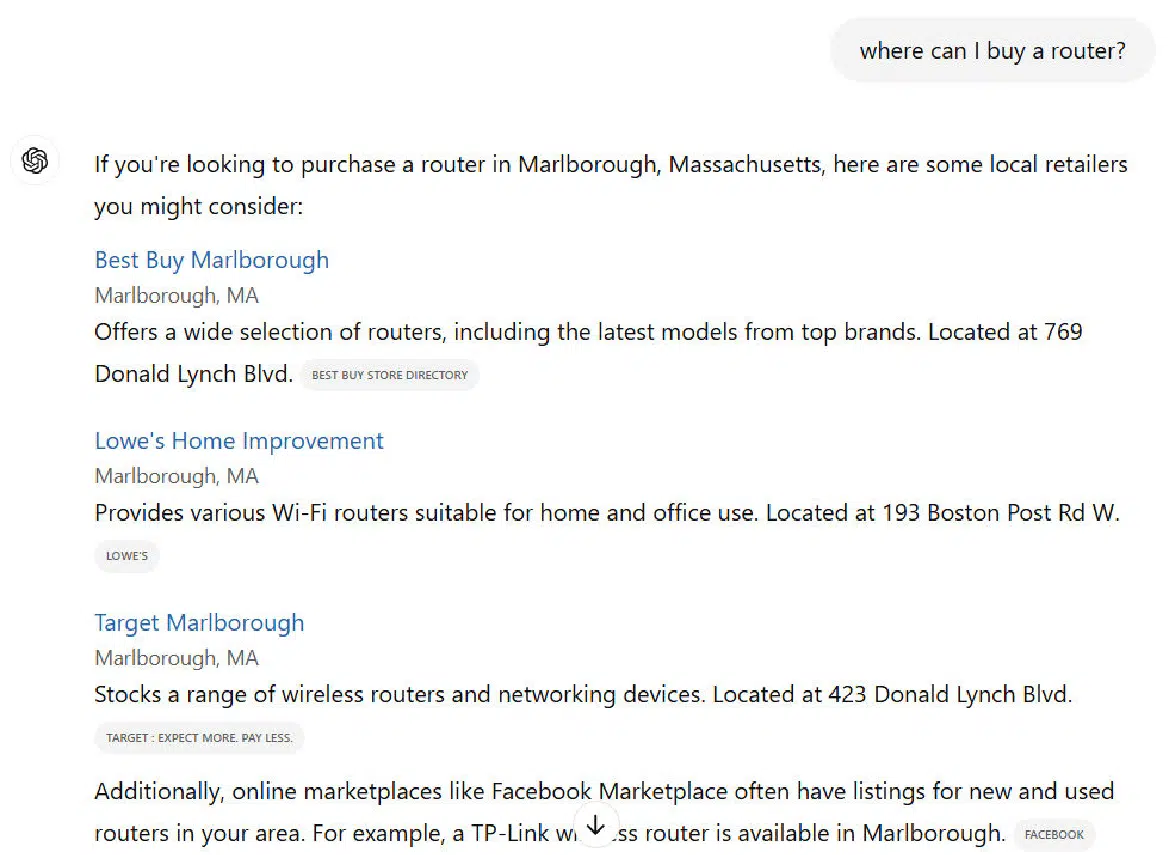
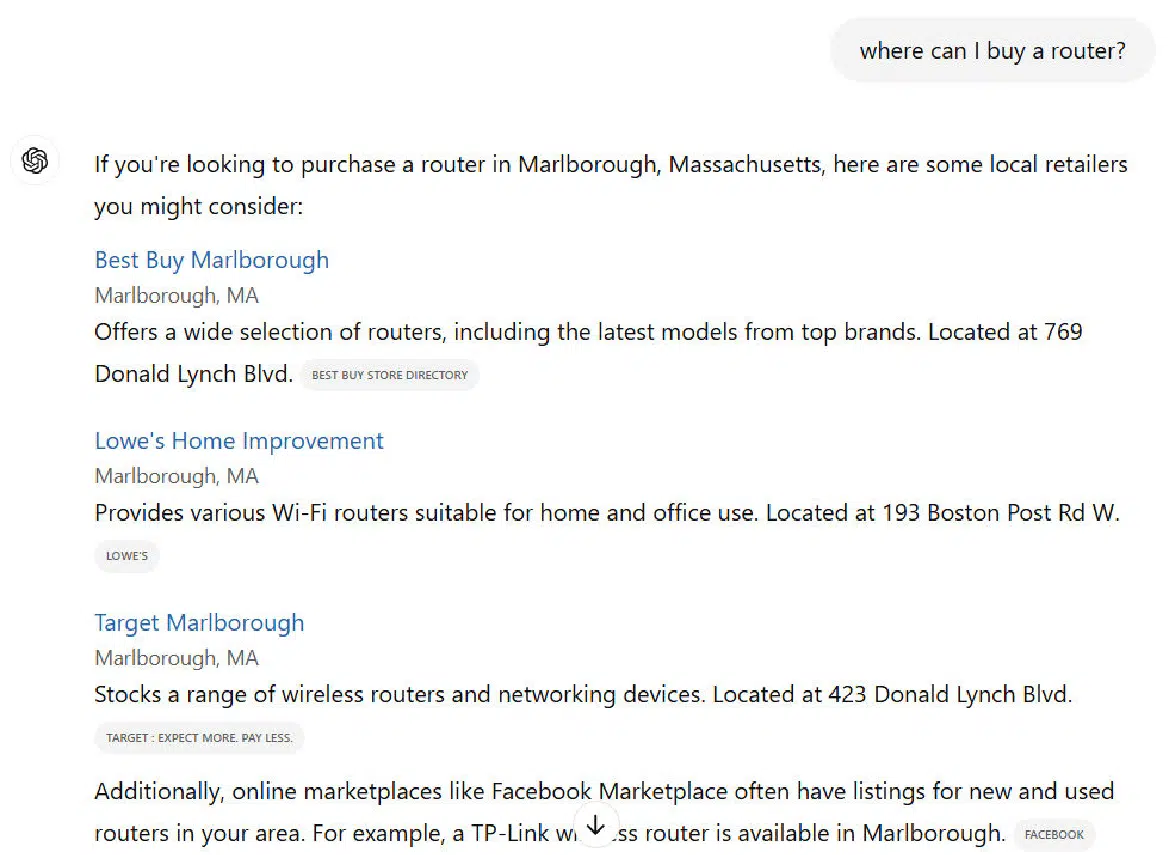
You might think this result is perfect, but routers also refer to a tool used in woodworking projects.
I use one frequently as a part of building furniture from scratch (true story).
There is a large enough audience of people who use these types of routers that I hope to see recognition of this in the SERPs.
Here is Google’s response to the query:
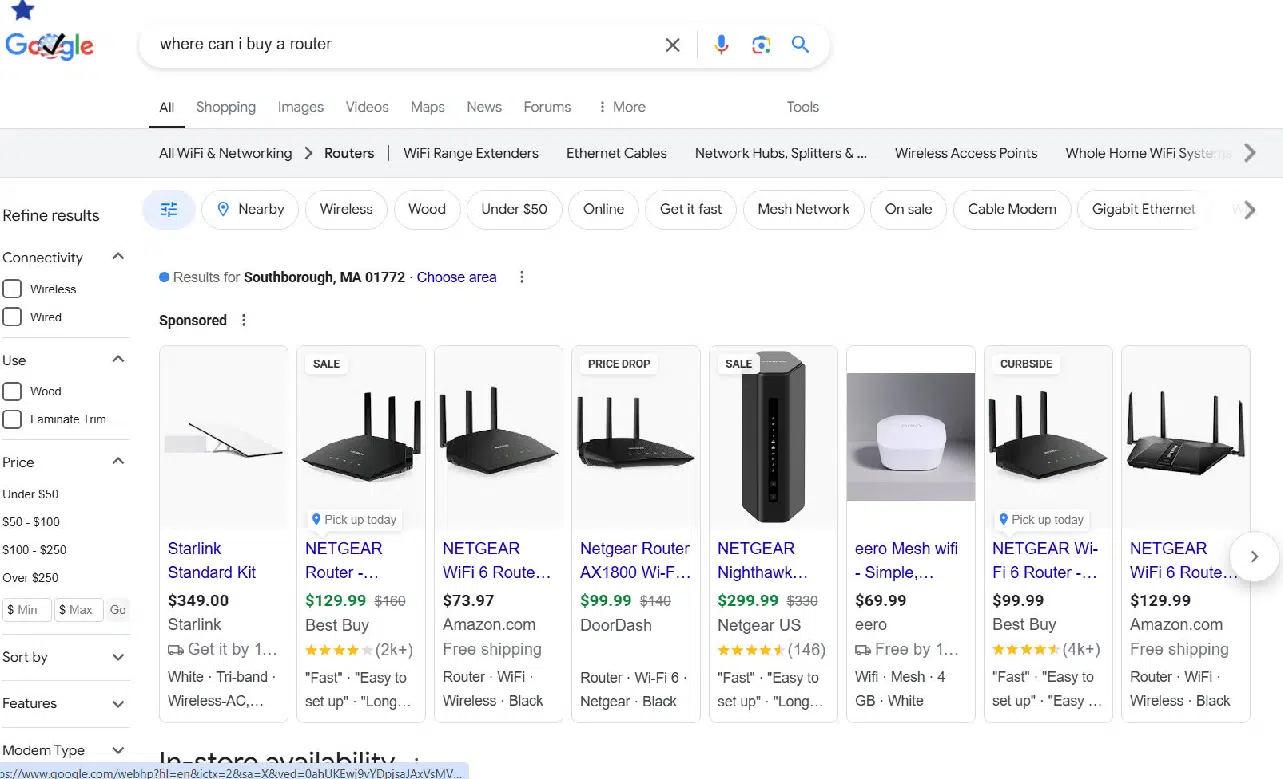
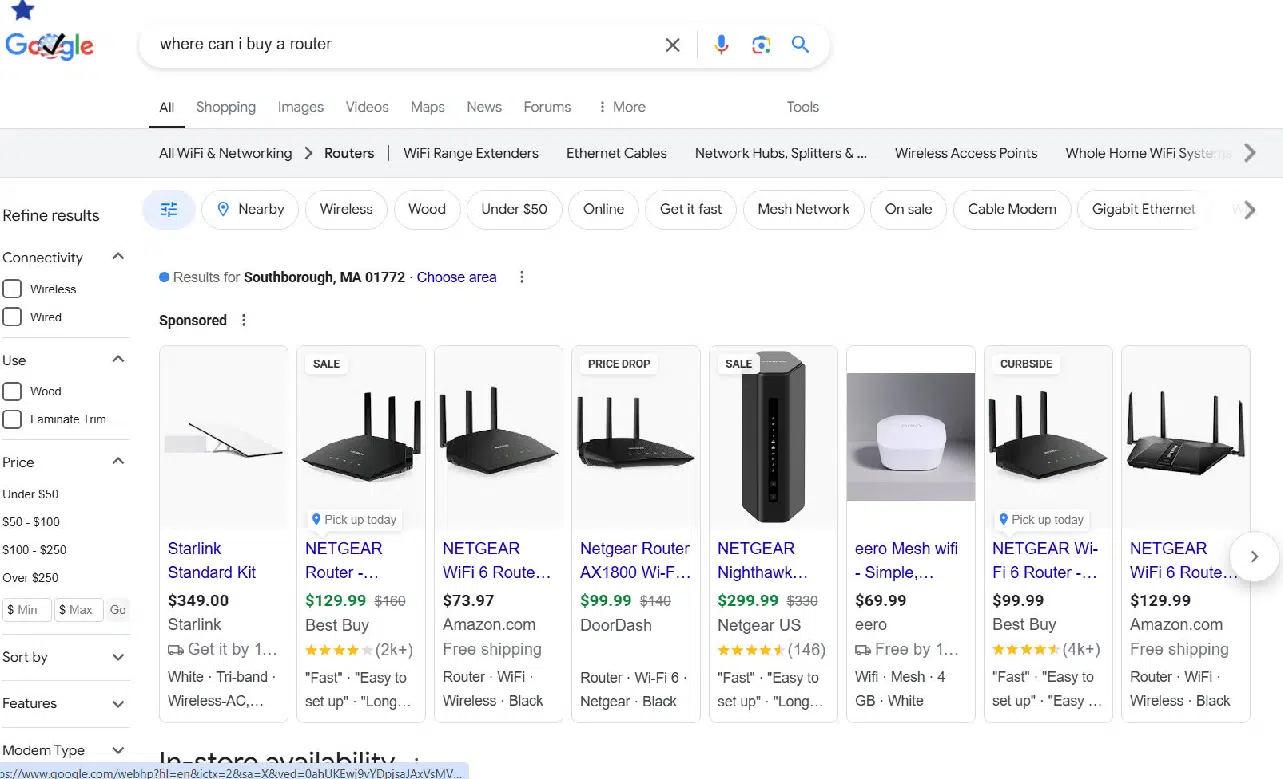
This part of the SERP is followed by:
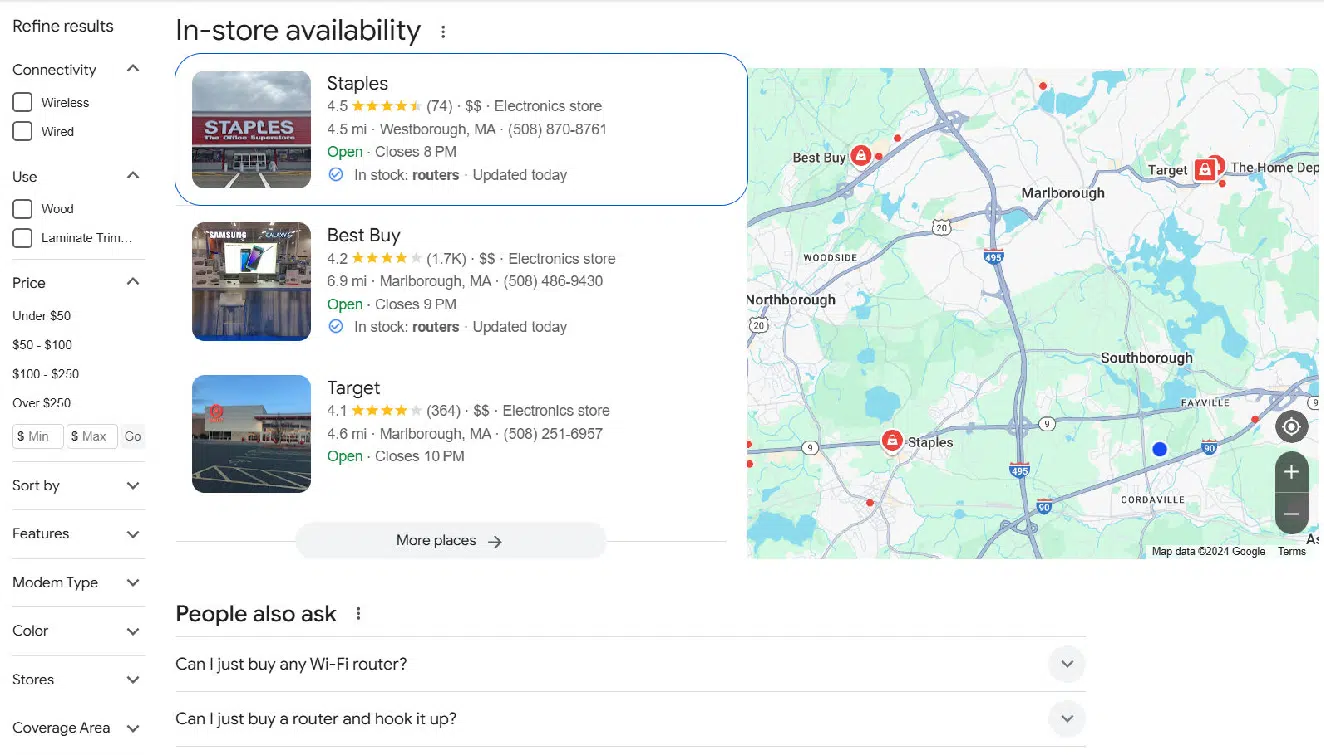
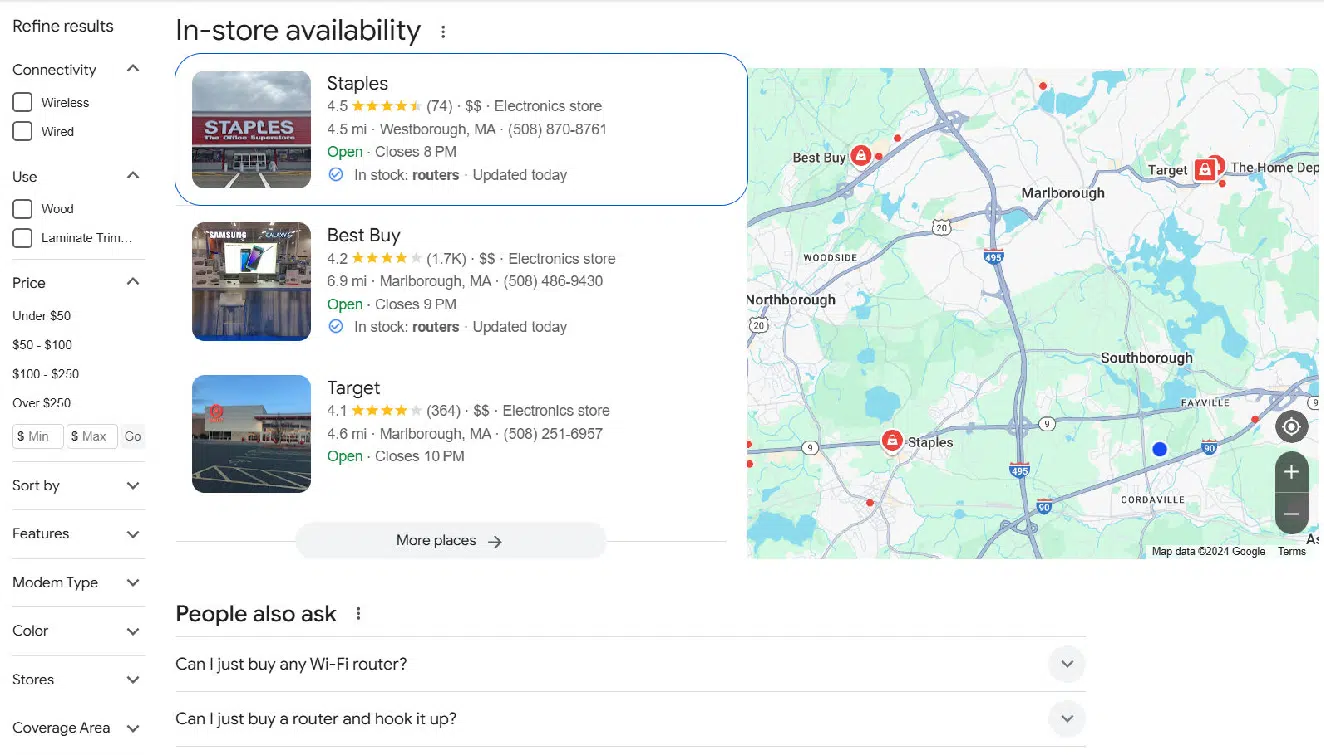
Google focuses on the internet router to the same degree as ChatGPT.
For this class of queries, I assigned these scores:
- ChatGPT search: 6.00.
- Google: 5.29.
Query type: Maintaining context in query sequences
Another interesting aspect of search is that users tend to enter queries in sequences.
Sometimes those query sequences contain much information that helps clarify their query intent.
An example query sequence is as follows:
- What is the best router to use for cutting a circular table top?
- Where can I buy a router?
As we’ve seen, the default assumption when people speak about routers is that they refer to devices for connecting devices to a single Internet source.
However, different types of devices, also called routers, are used in woodworking.
In the query sequence above, the reference to cutting a circular table should make it clear that the user’s interest is in the woodworking type of router.
ChatGPT’s response to the first query was to mention two specific models of routers and the general characteristics of different types of woodworking routers.
Then the response to “where can I buy a router” was a map with directions to Staples and the following content:
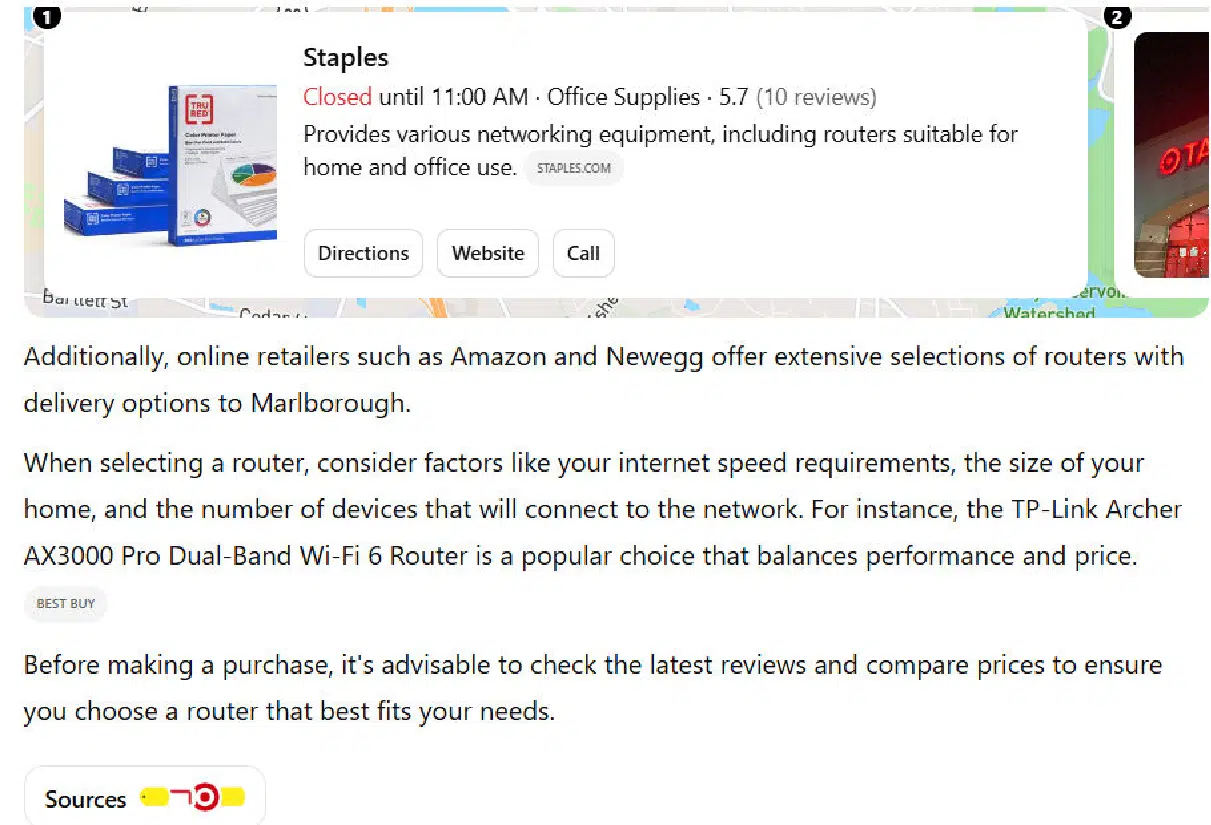
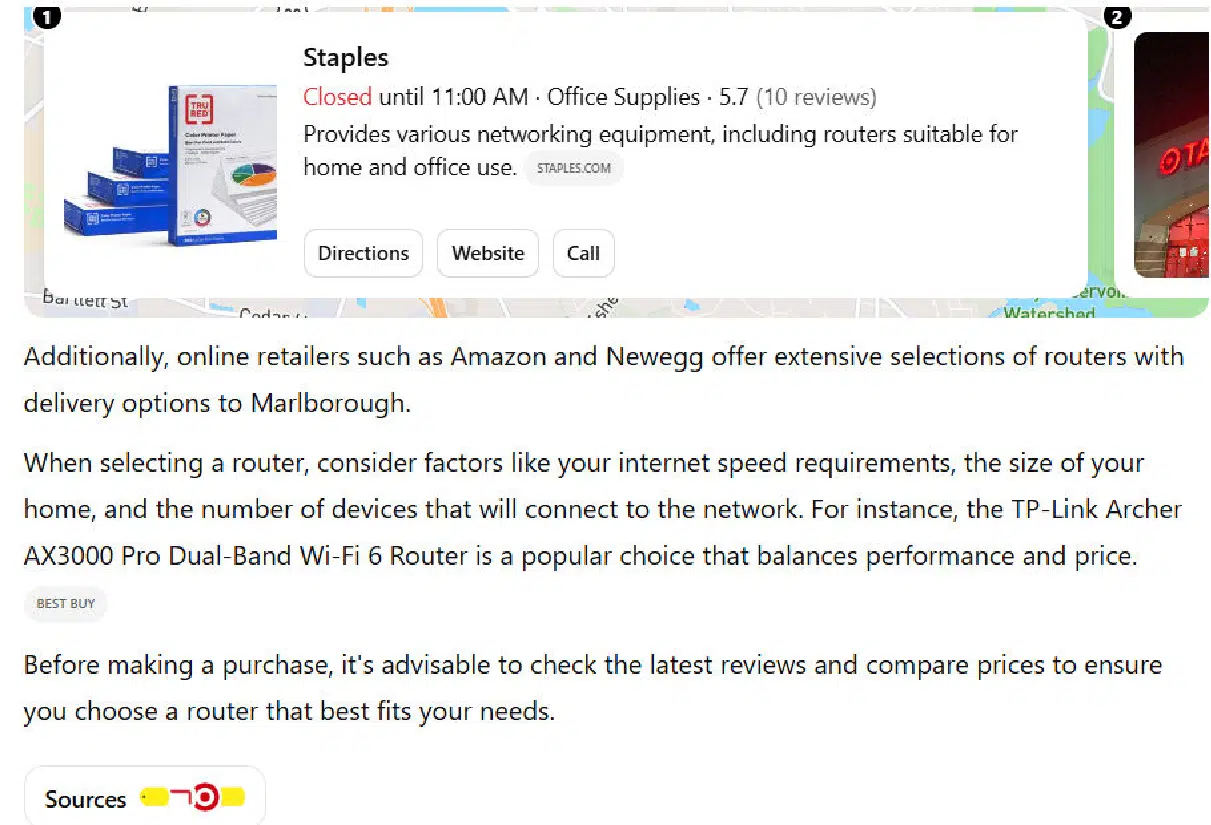
All of the context of the query was 100% lost.
Sadly, Google only performed slightly better.
It identified three locations, two of which were focused on networking routers and one which was focused on woodworking routers (Home Depot):
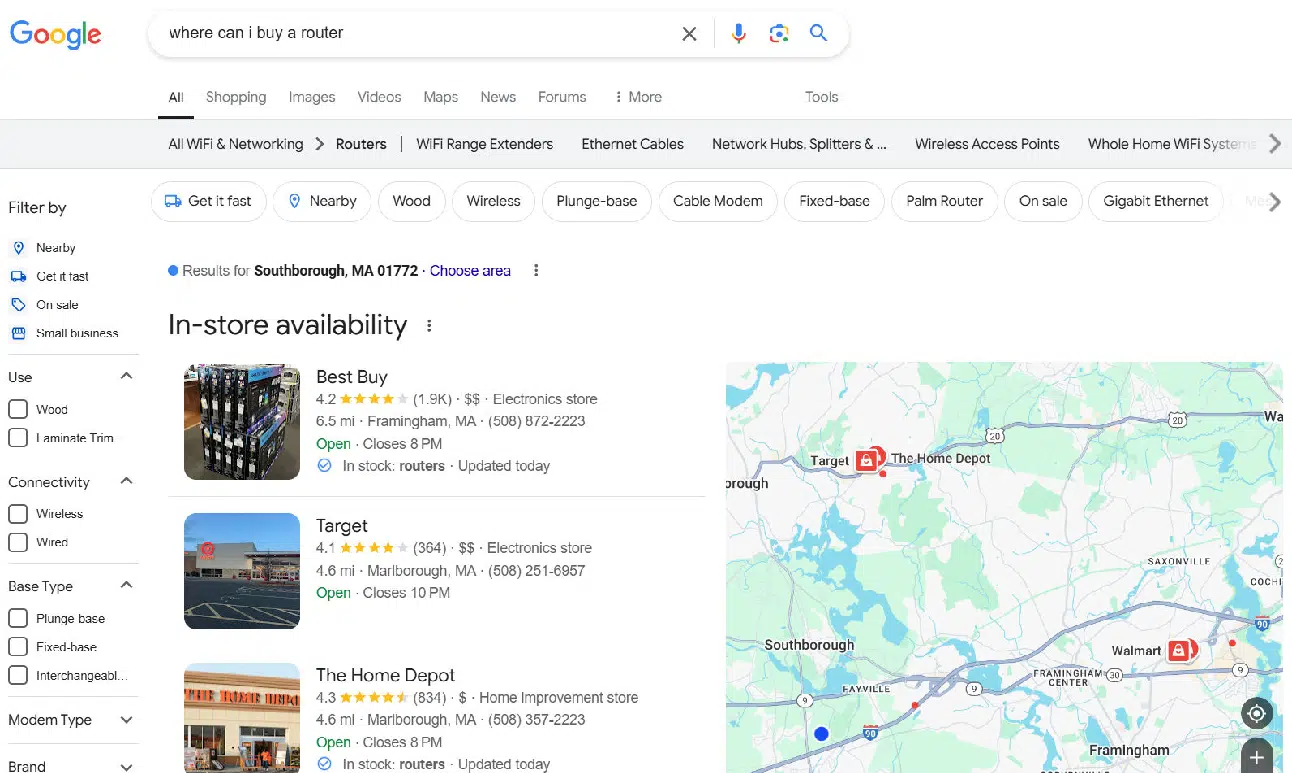
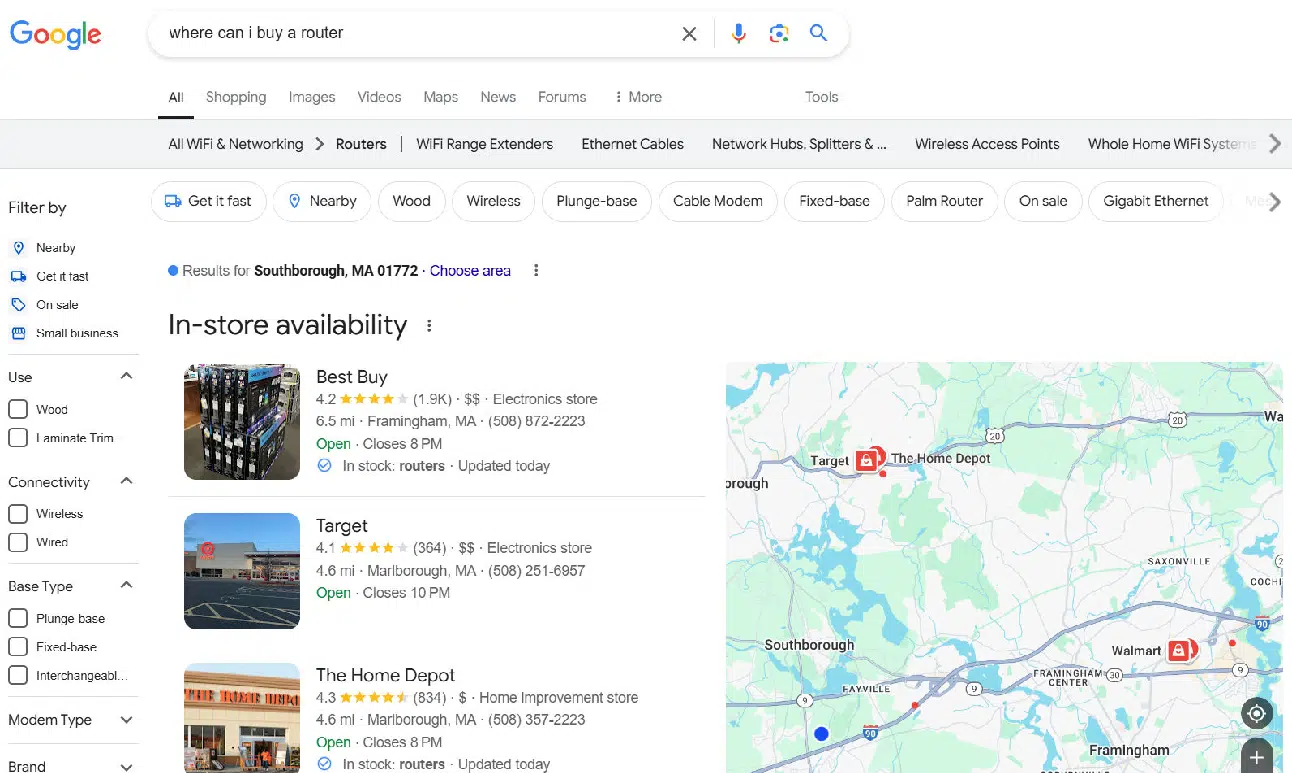
For this query, I scored the tools this way:
- ChatGPT search: 2.00.
- Google: 3.00.
Query type: Assumed typos
Another interesting example is queries where your search is relatively rare, yet it has a spelling that’s similar to another word.
For this issue, my search was: “Please discuss the history of the pinguin.”
The Pinguin was a commerce raider used by the German Navy in World War 2. It just has a spelling very similar to “penguin,” which is an aquatic flightless bird.
Both ChatGPT and Google simply assumed that I meant “penguin” and not “pinguin.”
Here is the result from ChatGPT:
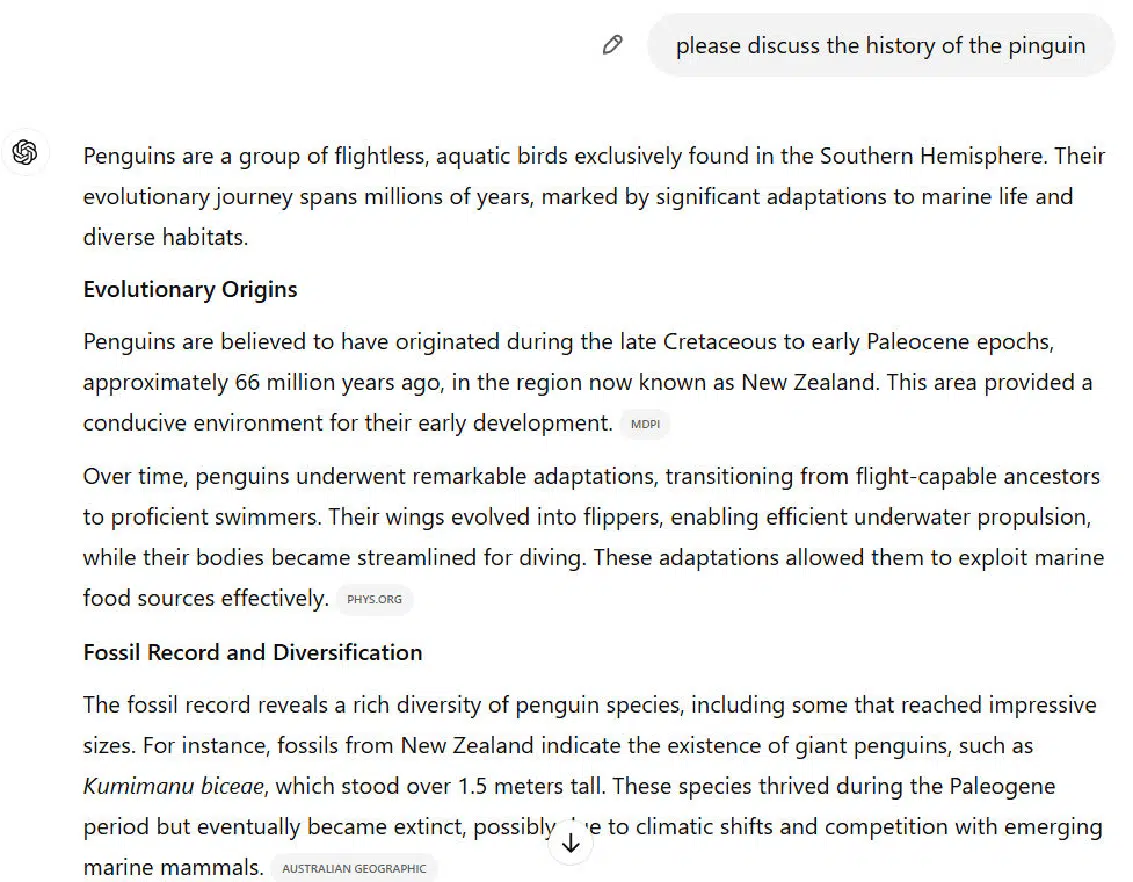
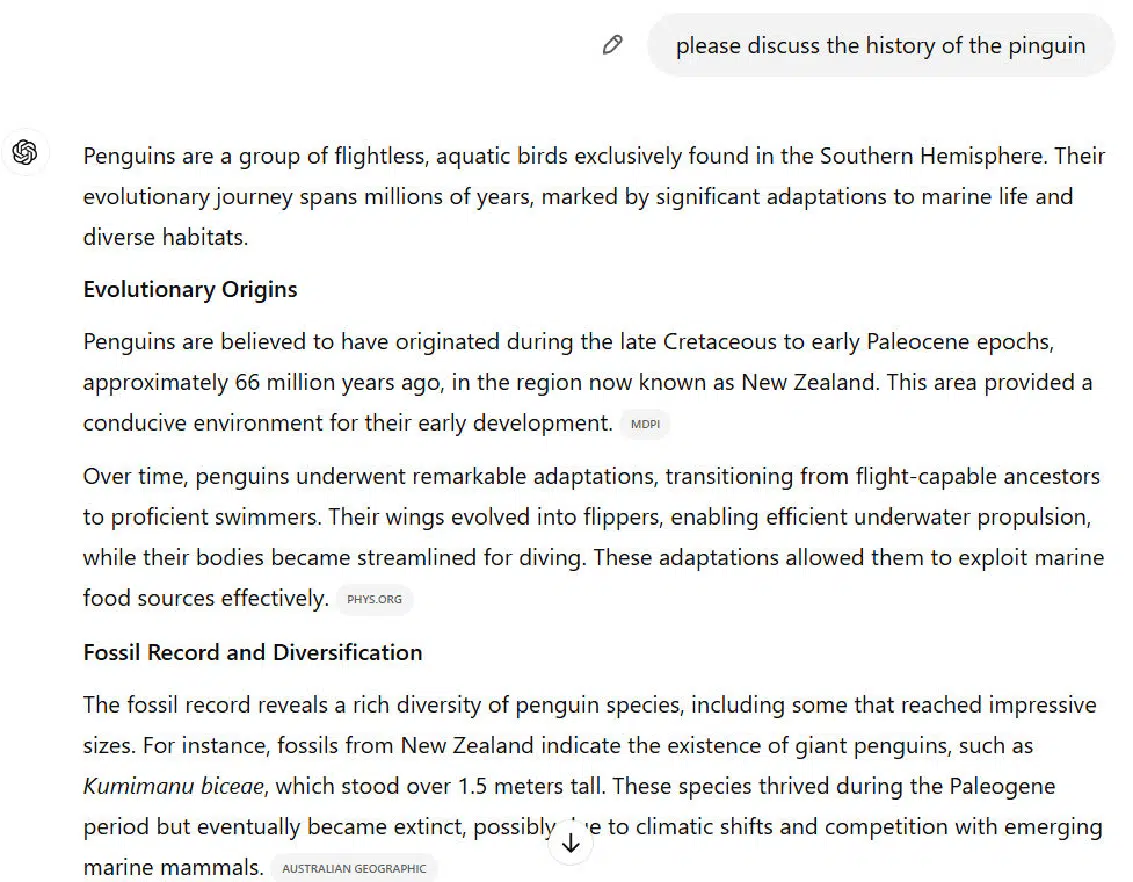
The result continues after what I’ve shown here but continues to focus on the bird, not the boat.
Google makes the same mistake:
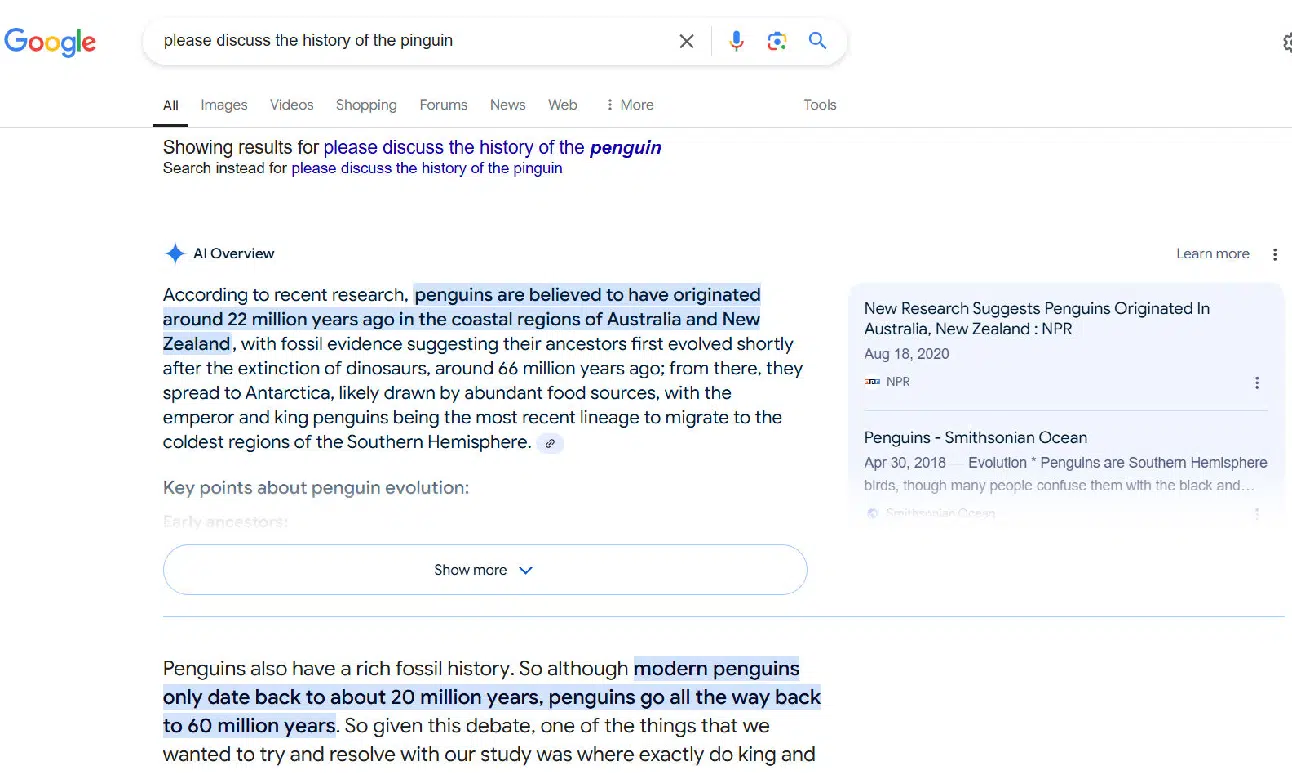
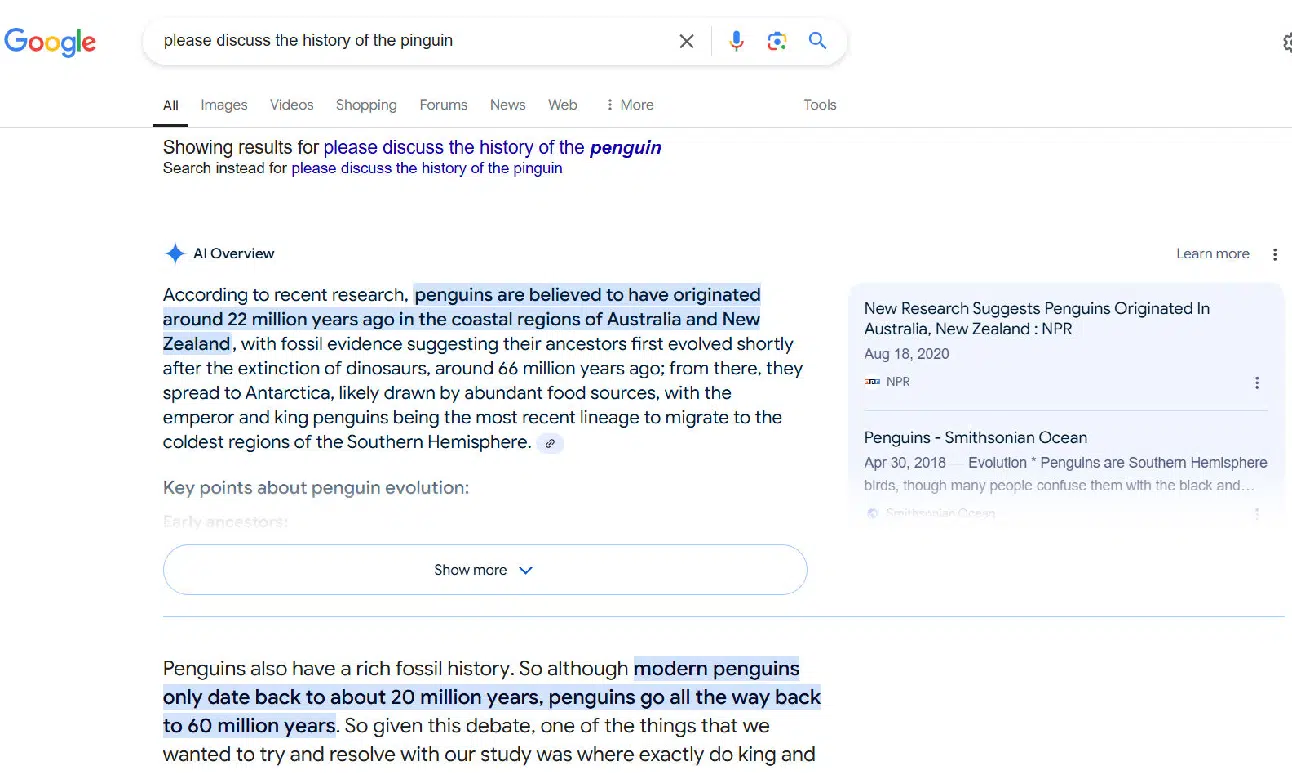
After the AI Overview and the featured snippet I’ve shown here, the SERPs continue to show more results focused on our flightless friends.
To be fair, I’ve referred to this as a mistake, but the reality is that the percentage of people who enter “pinguin” that simply misspelled “penguin” is probably far greater than those who actually mean the German Navy’s WW2 commerce raider.
However, you’ll notice that Google does one thing just a touch better than ChatGPT here.
At the top of the results, it acknowledges that it corrected “pinguin” to “penguin” and allows you to change it back.
The other way I addressed the problem was to do a second query: “Please discuss the history of the pinguin in WW2,” and both ChatGPT and Google gave results on the WW2 commerce raider.
For this query, I assigned these scores:
- ChatGPT search: 2.00.
- Google: 3.00.
Query type: Multiple options are a better experience
There are many queries where a single (even if it is well thought out) response is not what someone is probably looking for.
Consider, for example, a query like: “smoked salmon recipe.”
Even though the query is in the singular, there is little chance that anyone serious about cooking wants to see a single answer.
This type of searcher is looking for ideas and wants to look at several options before deciding what they want to do.
They may want to combine ideas from multiple recipes before they have what they want.
Let’s look at the response from ChatGPT search:
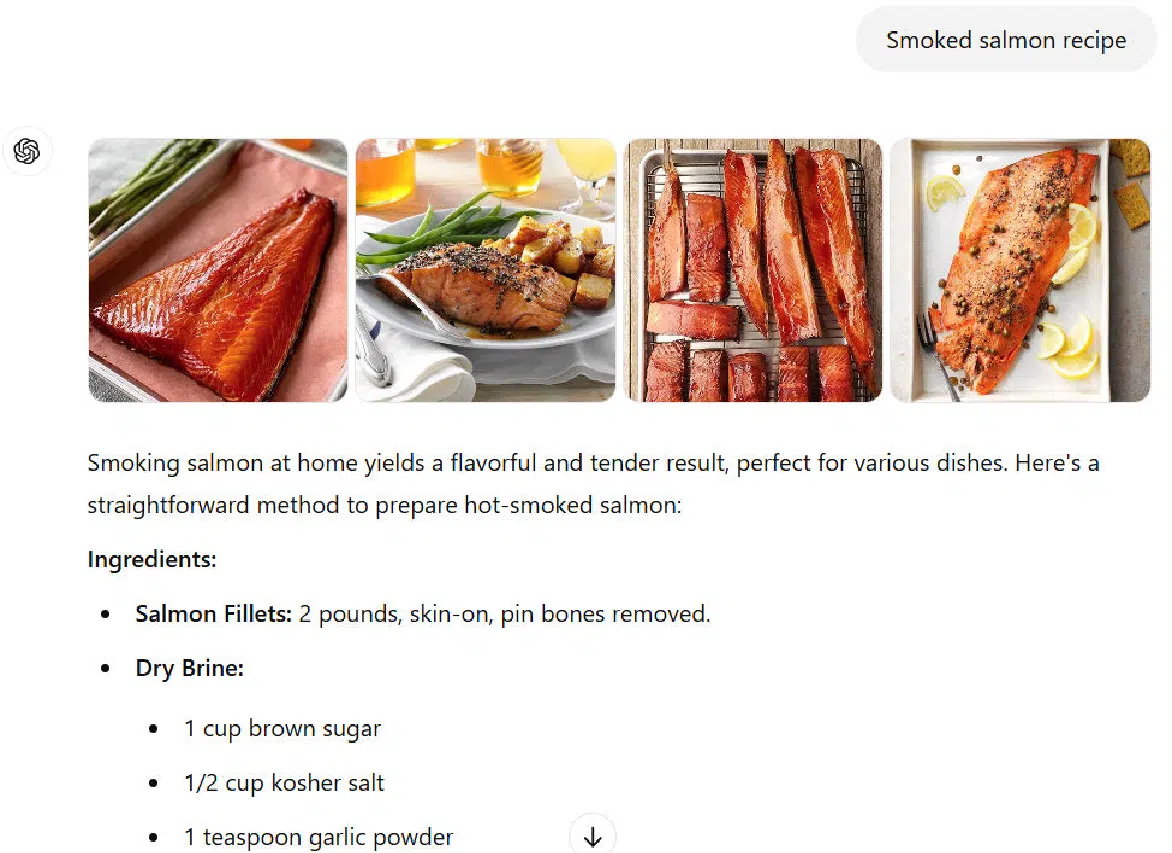
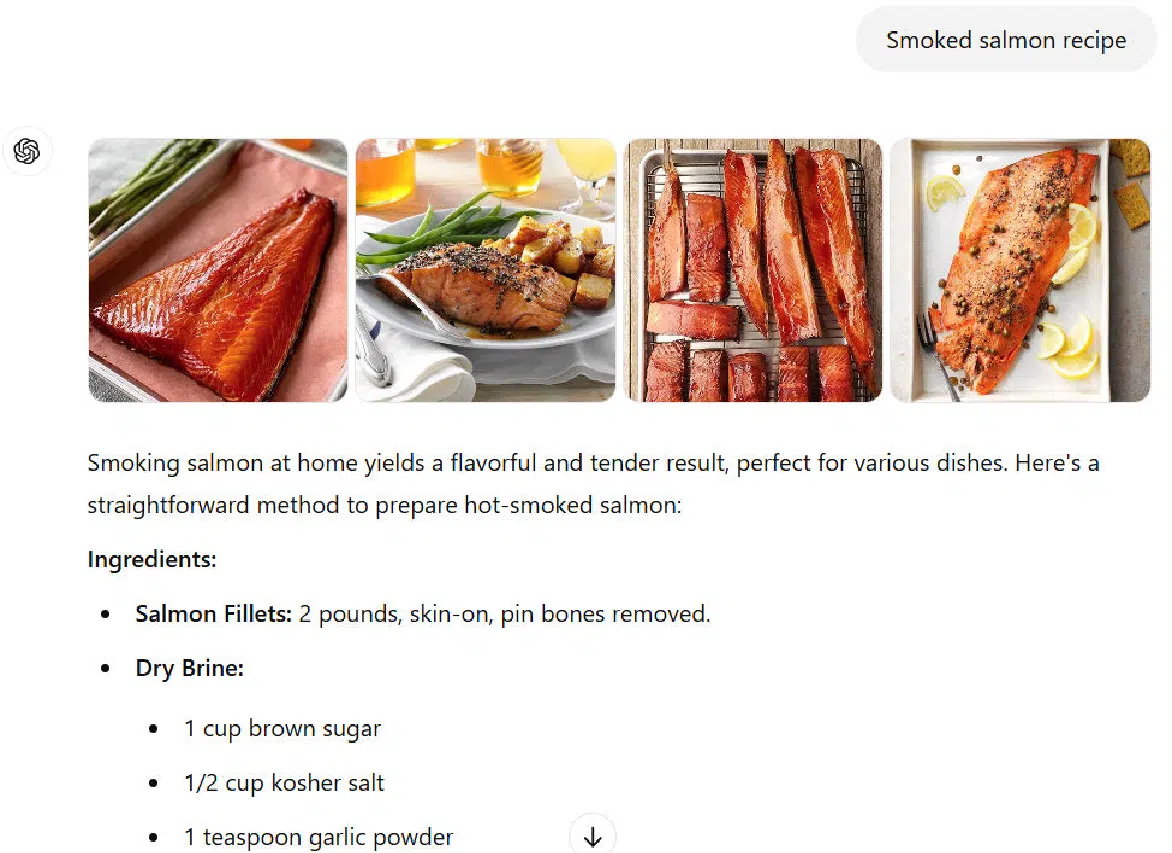
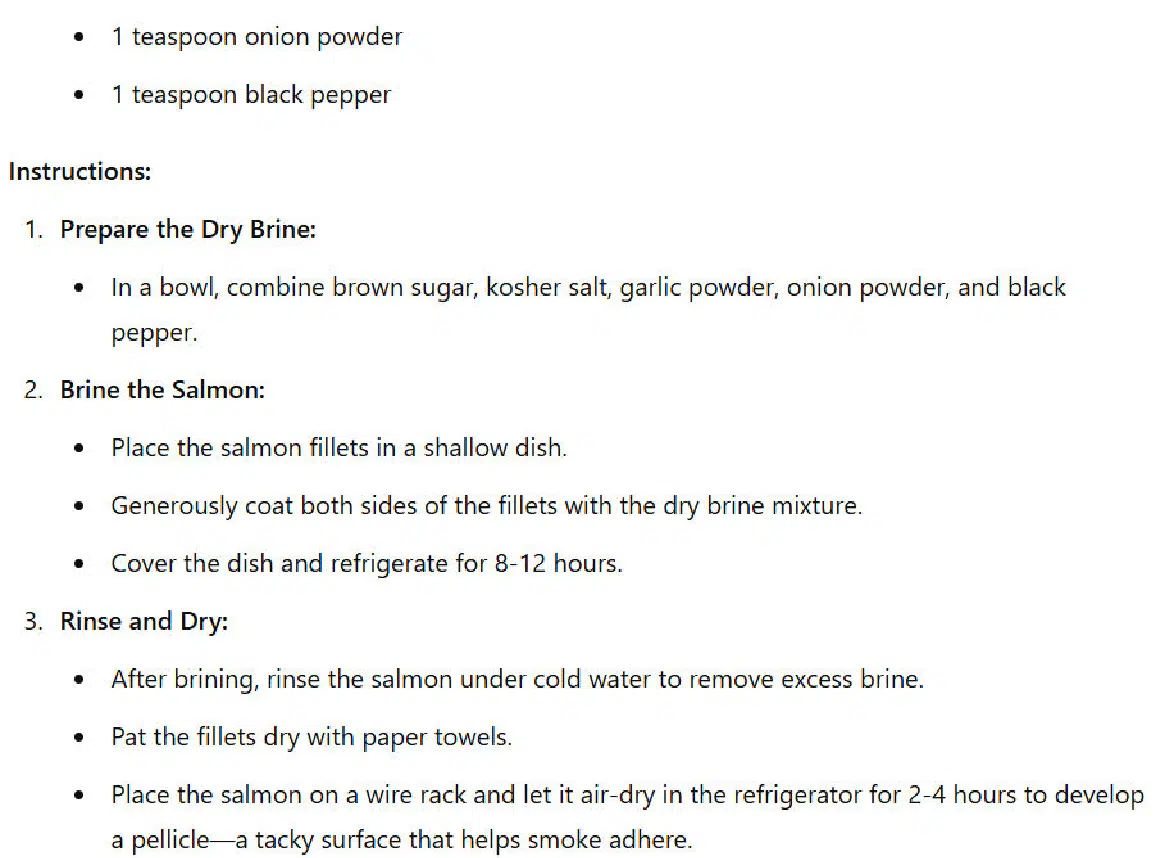
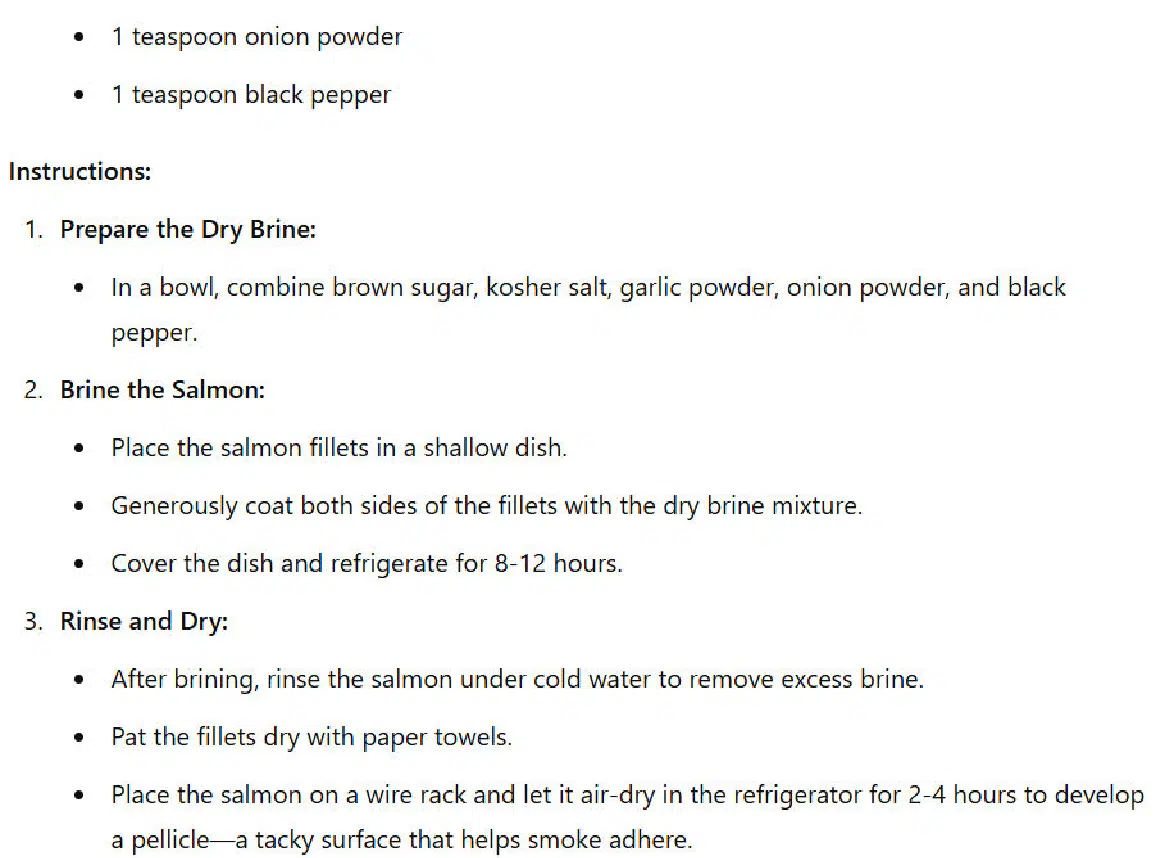
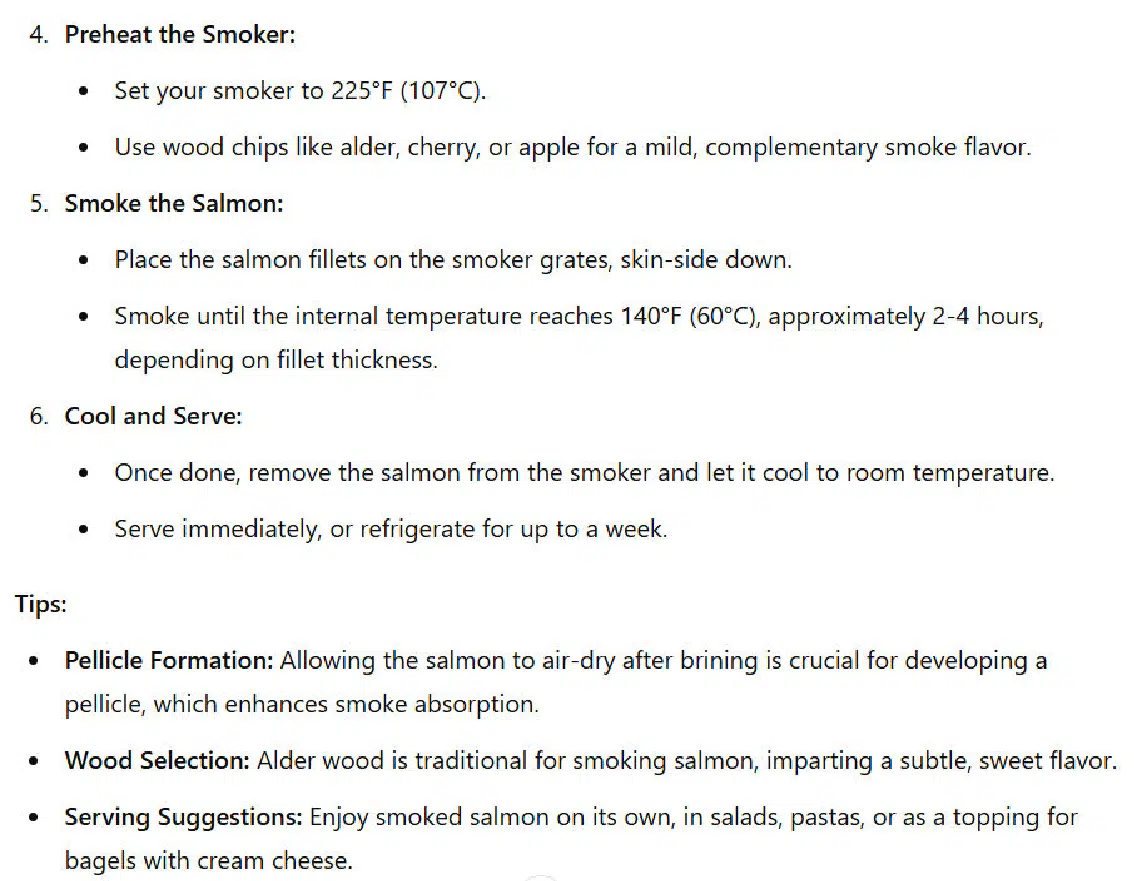
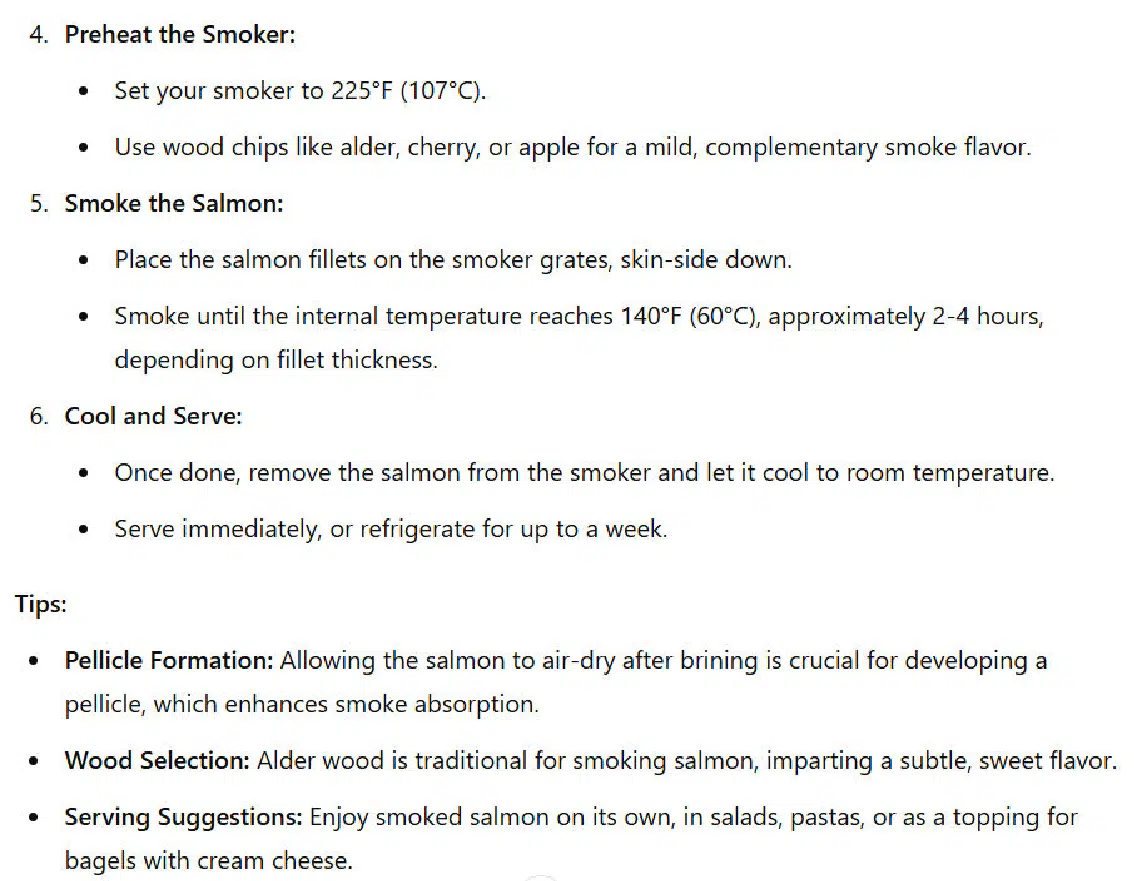
I’ve included the first three screens of the response (out of four), and here you will see that ChatGPT search provides one specific recipe from a site called Honest Food.
In addition, I see some things that don’t align with my experience.
For example, this write-up recommends cooking the salmon to 140 degrees. That’s already beginning to dry the salmon a bit.
From what I see on the Honest Food site, they suggest a range of possible temperatures starting from as low as 125.
In contrast, Google offers multiple recipes that you can access from the SERPs:
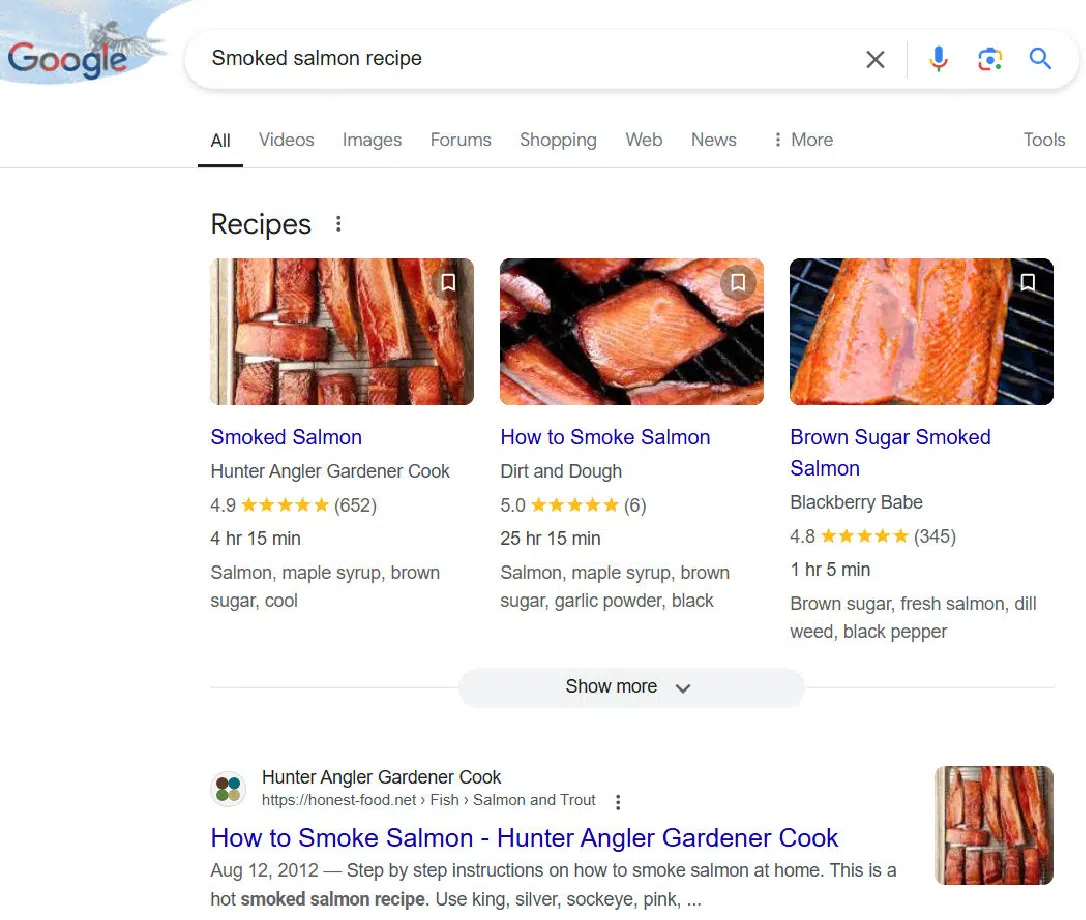
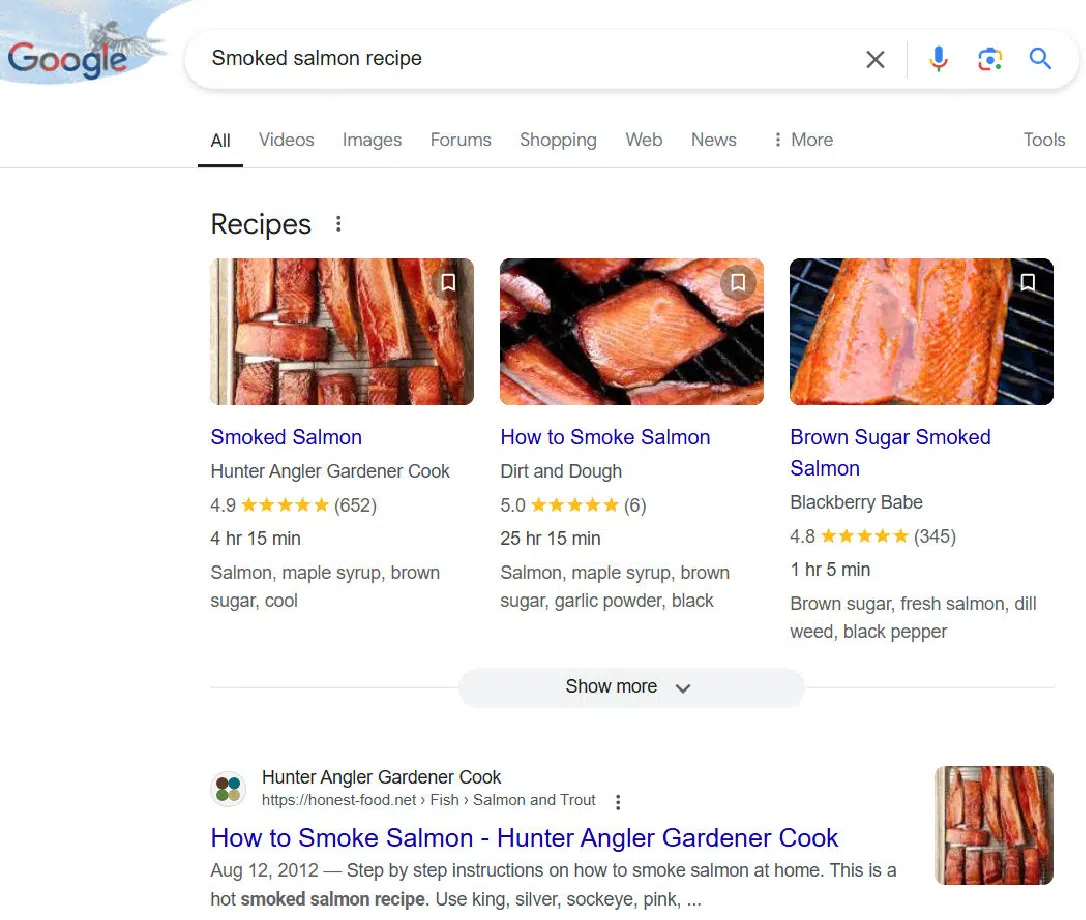
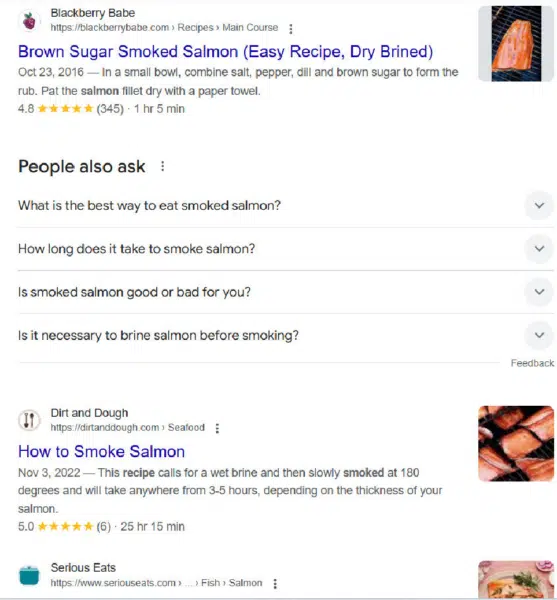
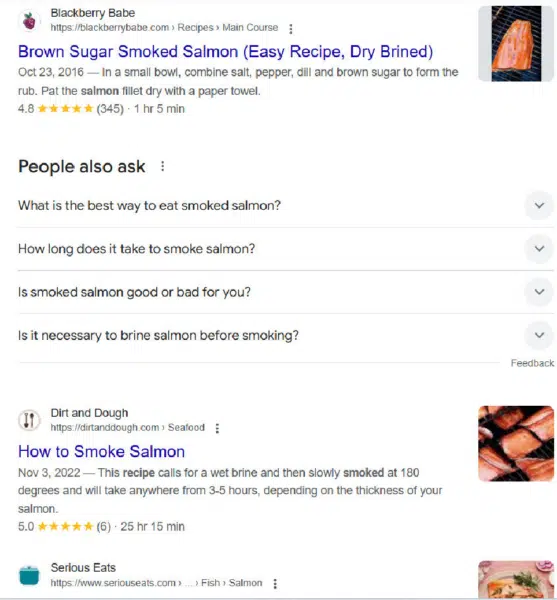
This is an example of a query that I scored in Google’s favor, as having multiple options is what I believe most searchers will want.
The scores I assigned were:
- ChatGPT search: 4.00.
- Google: 8.00.
Get the newsletter search marketers rely on.
Types of problems
Next, we’ll examine the types of things that can go wrong. I looked for these issues while scoring the results.
The analysis noted where problems that generative AI tools are known for were found and potential areas of weakness in Google’s SERPs.
These included:
- Errors.
- Omissions.
- Weaknesses.
- Incomplete coverage.
- Insufficient follow-on resources.
Problem type: Errors
This is what the industry refers to as “hallucinations,” meaning that the information provided is simply wrong.
Sometimes errors aren’t necessarily your money or your life situations, but they still give the user incorrect information.
Consider how ChatGPT search responds to a query asking about the NFL’s overtime rules:
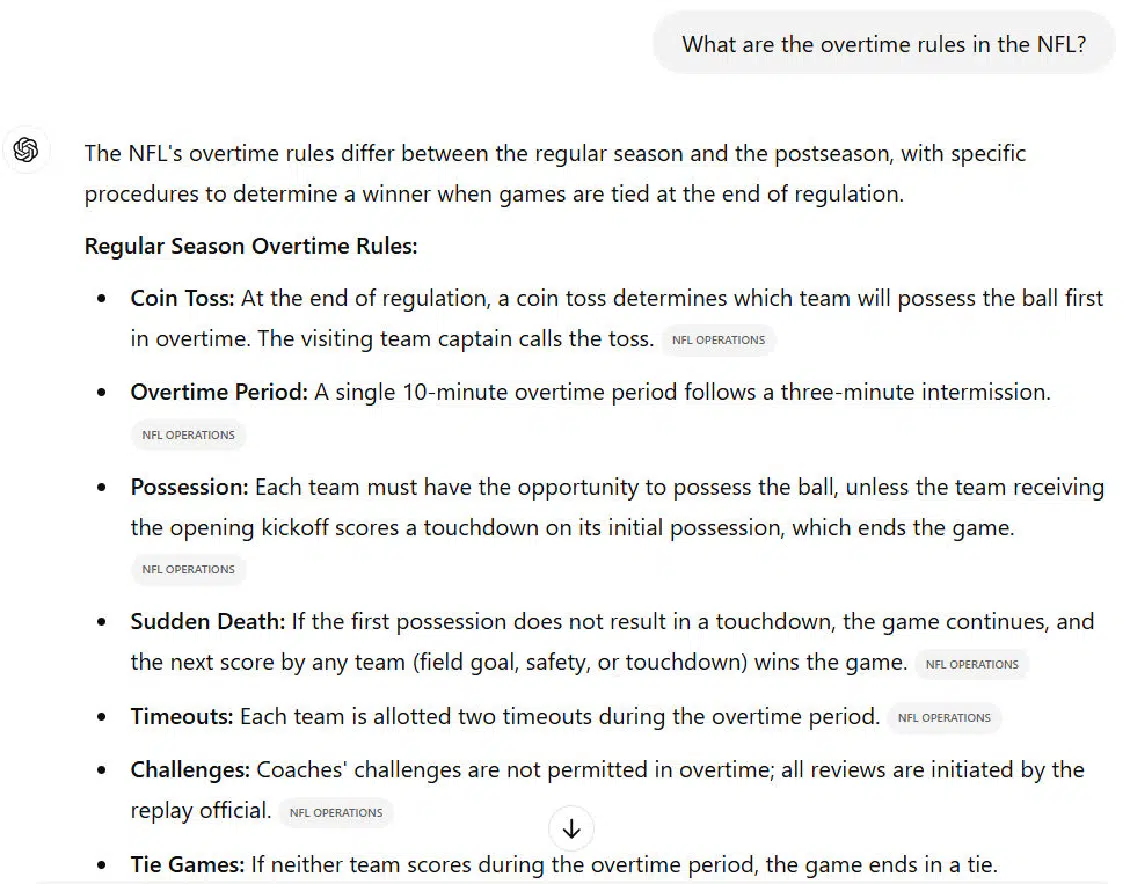
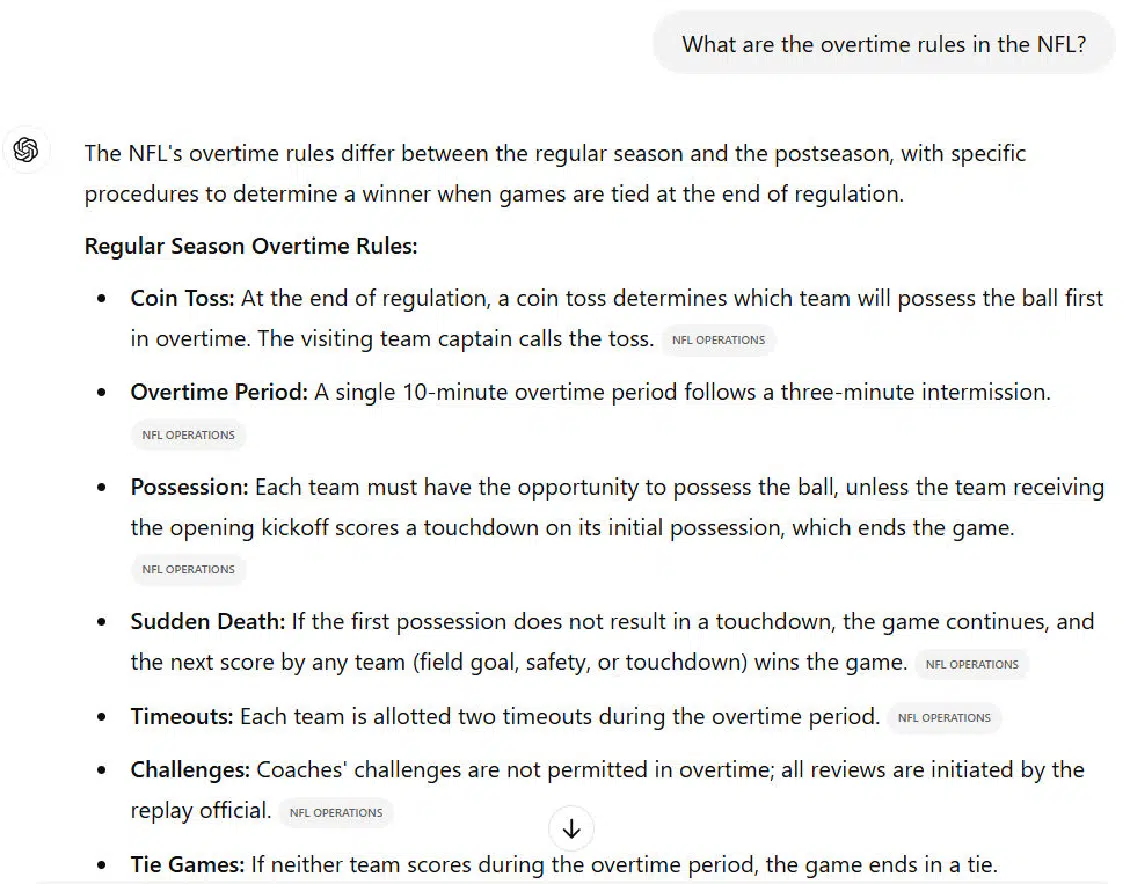
Notice the paragraph discussing how Sudden Death works. Unfortunately, it’s not correct.
It doesn’t account for when the first team that possesses the ball kicks a field goal, in which case they could win the game if the second team doesn’t score a field goal.
If the second team scores a field goal, this will tie the game.
In this event, it’s only after the field goal by the second team that the next score wins the game.
This nuance is missed by ChatGPT search.
Note: The information on the NFL Operations page that ChatGPT search used as a source is correct.
Google’s AI Overview also has an error in it:
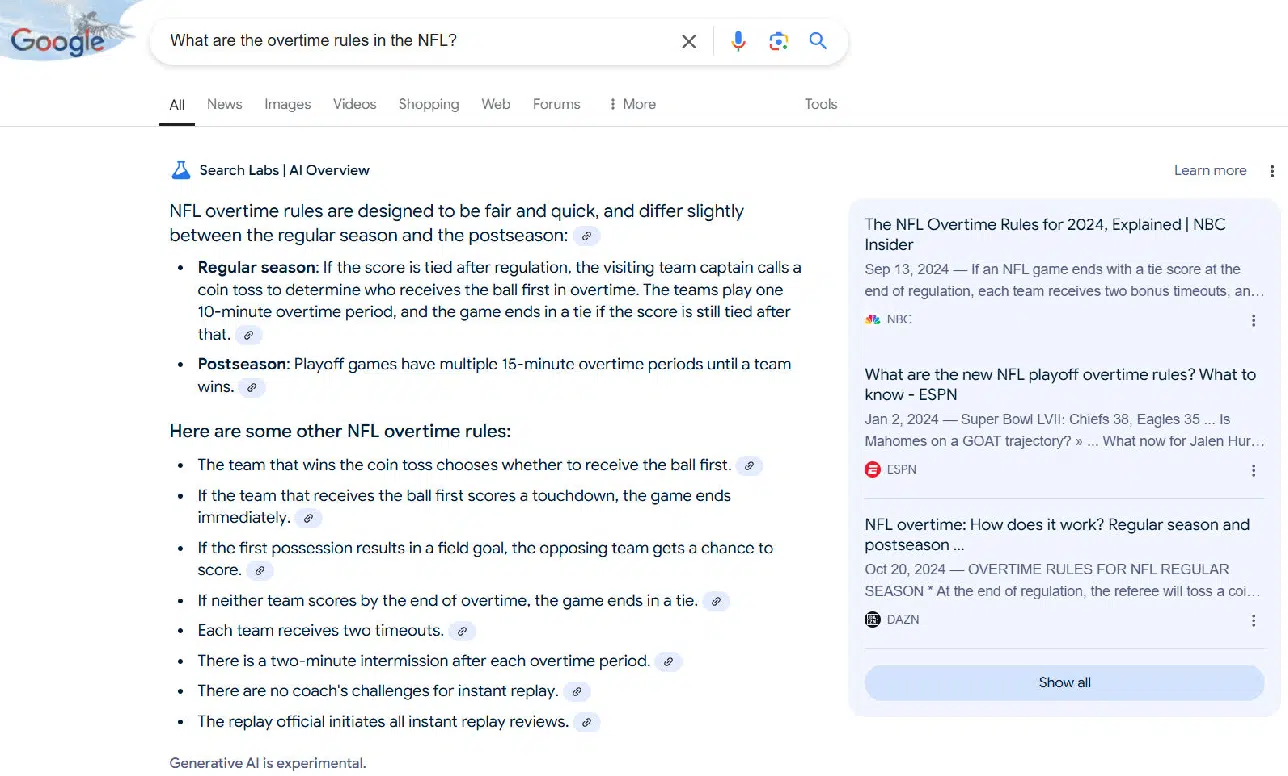
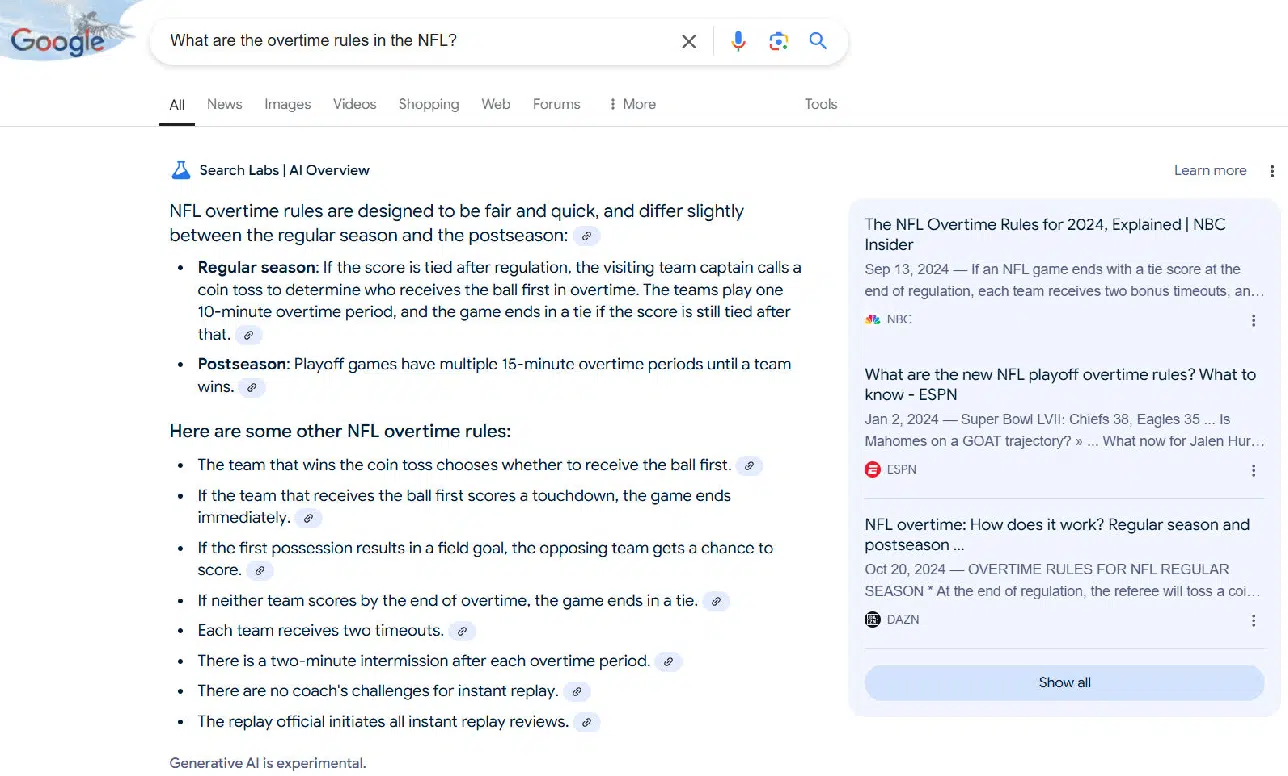
In the second line, where Google outlines “some other NFL overtime rules,” it notes that the same ends if the first team to possess the ball scores a touchdown.
This is true for regular season games but not true in the postseason, where both teams always get an opportunity to possess the ball.
Scores were as follows:
- ChatGPT search: 3.00.
- Google: 4.00.
Problem type: Omissions
This type of issue arises when important information that belongs in the response is left out.
Here is an example where ChatGPT search does this:
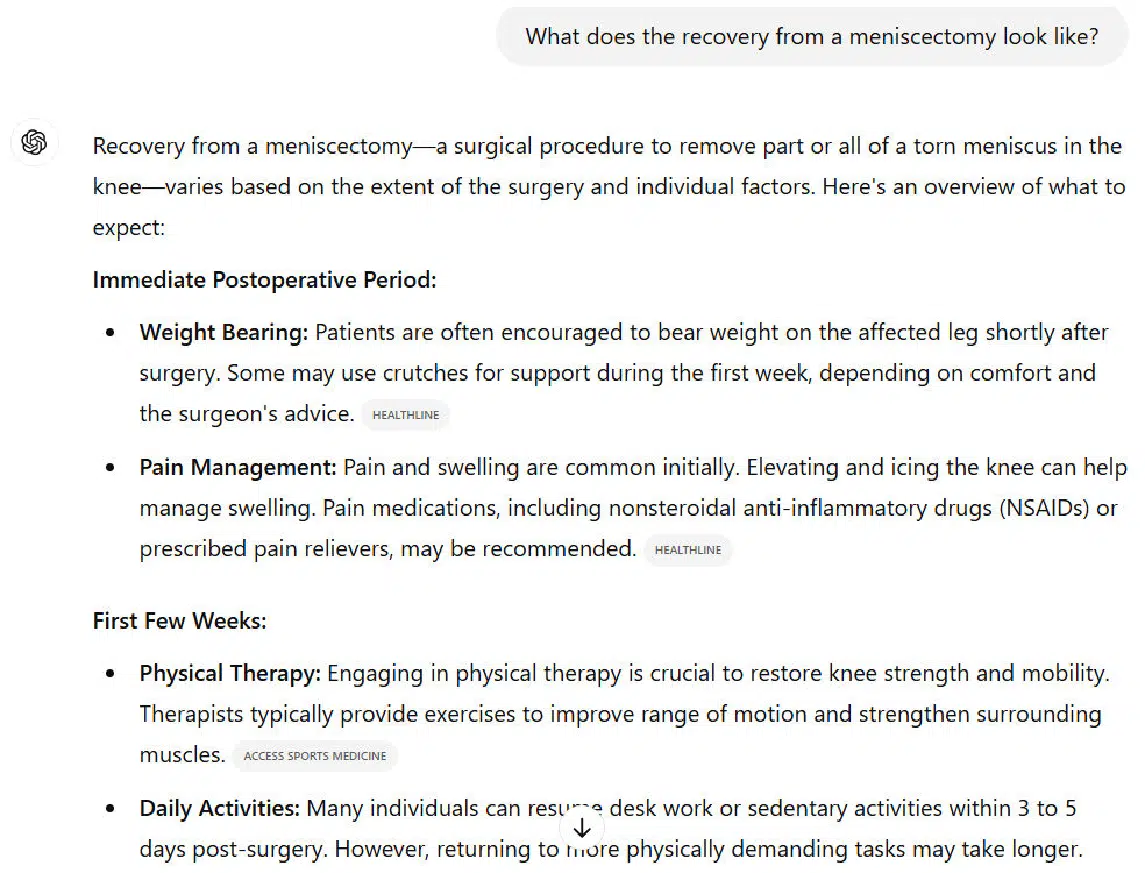
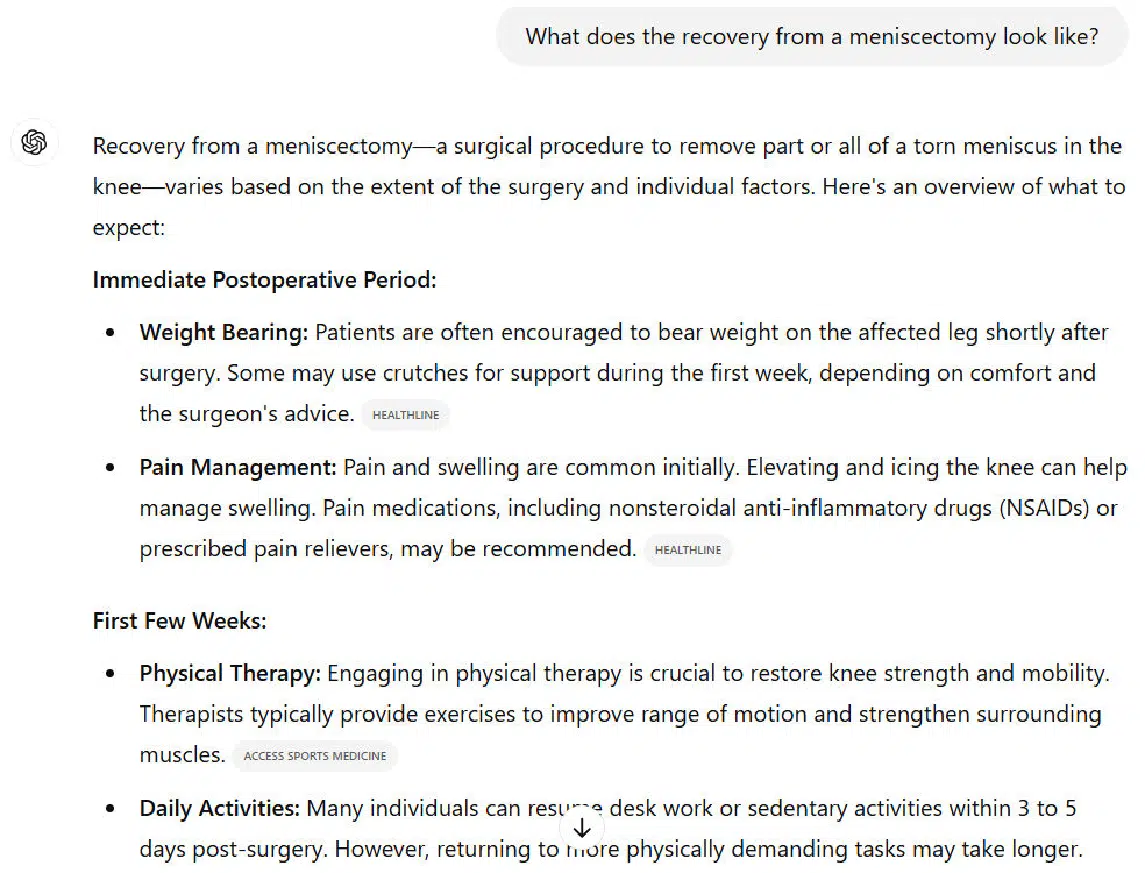
Under Pain Management, there is no mention of Tylenol as a part of a pain management regimen.
This is an unfortunate omission, as many people use only a mix of Tylenol and Ibuprofen to manage the pain after a meniscectomy.
Scores were as follows:
- ChatGPT search: 6.00.
- Google: 5.00.
Problem type: Weaknesses
I used weaknesses to cover cases where aspects of the result could have been more helpful to the searcher but where the identified issue couldn’t properly be called an error or omission.
Here is an example of an AI Overview that illustrates this:
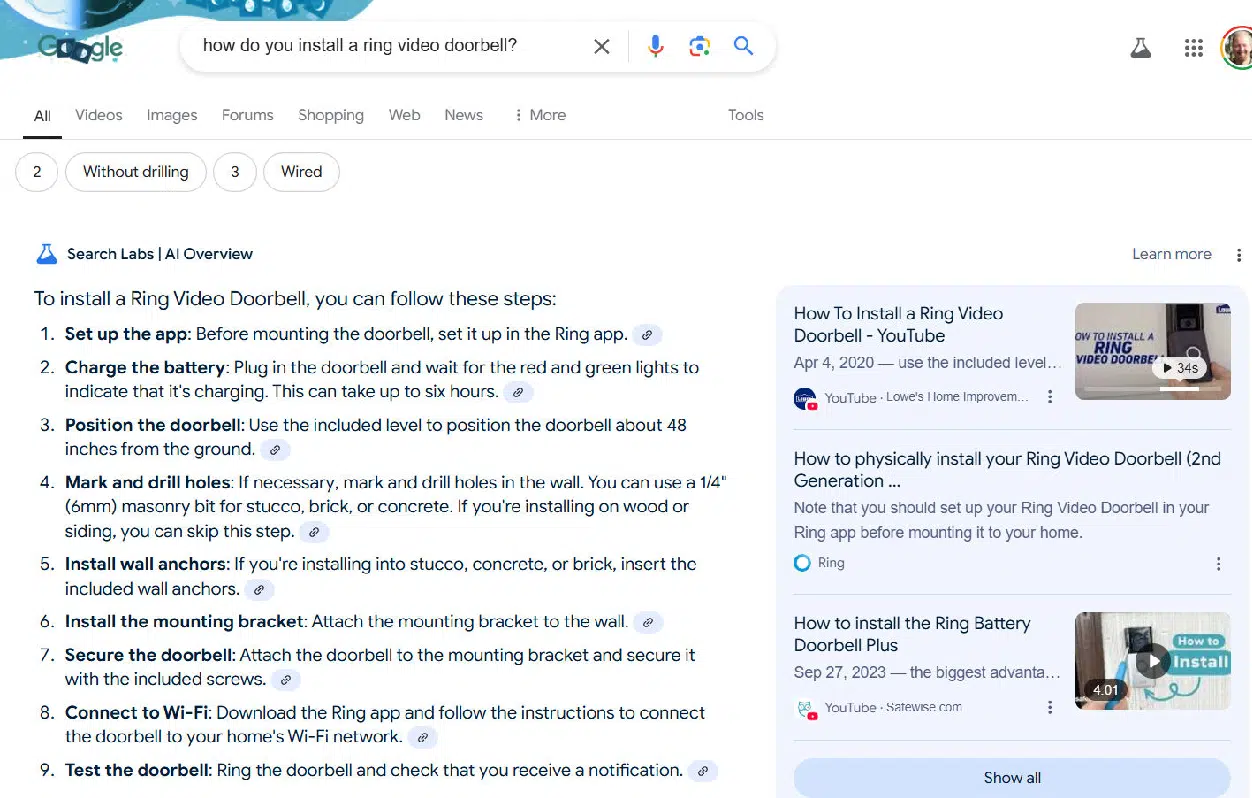
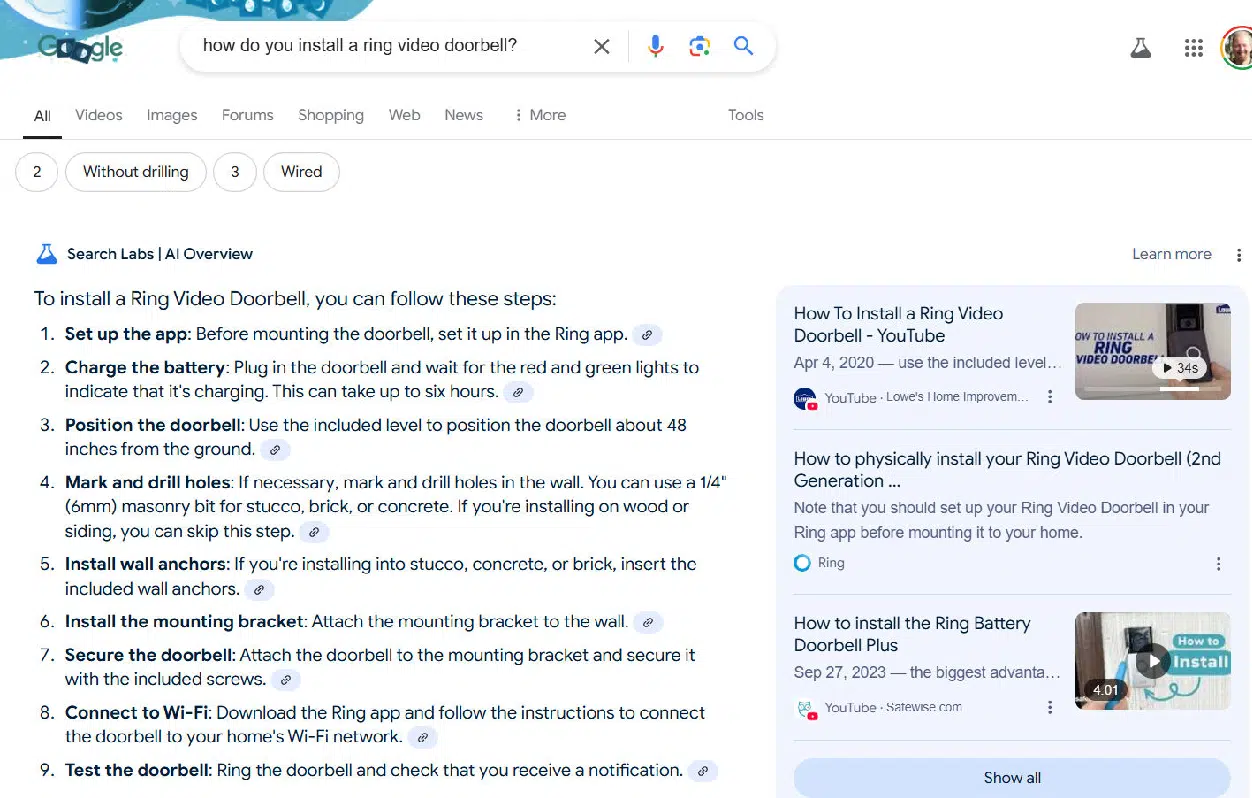
The weakness of this outline is that it makes the most sense to charge the battery as the first step.
Since it takes up to 6 hours to complet,e it’s not that useful to set up the app before completing this step.
Here is how I scored these two responses:
- ChatGPT search: 3.00.
- Google: 5.00.
Problem type: Incomplete coverage
This category is one that I used to identify results that failed to cover a significant user need for a query.
Note that “significant” is subjective, but I tried to use this only when many users would need a second query to get what they were looking for.
Here is an example of this from a Google SERP.
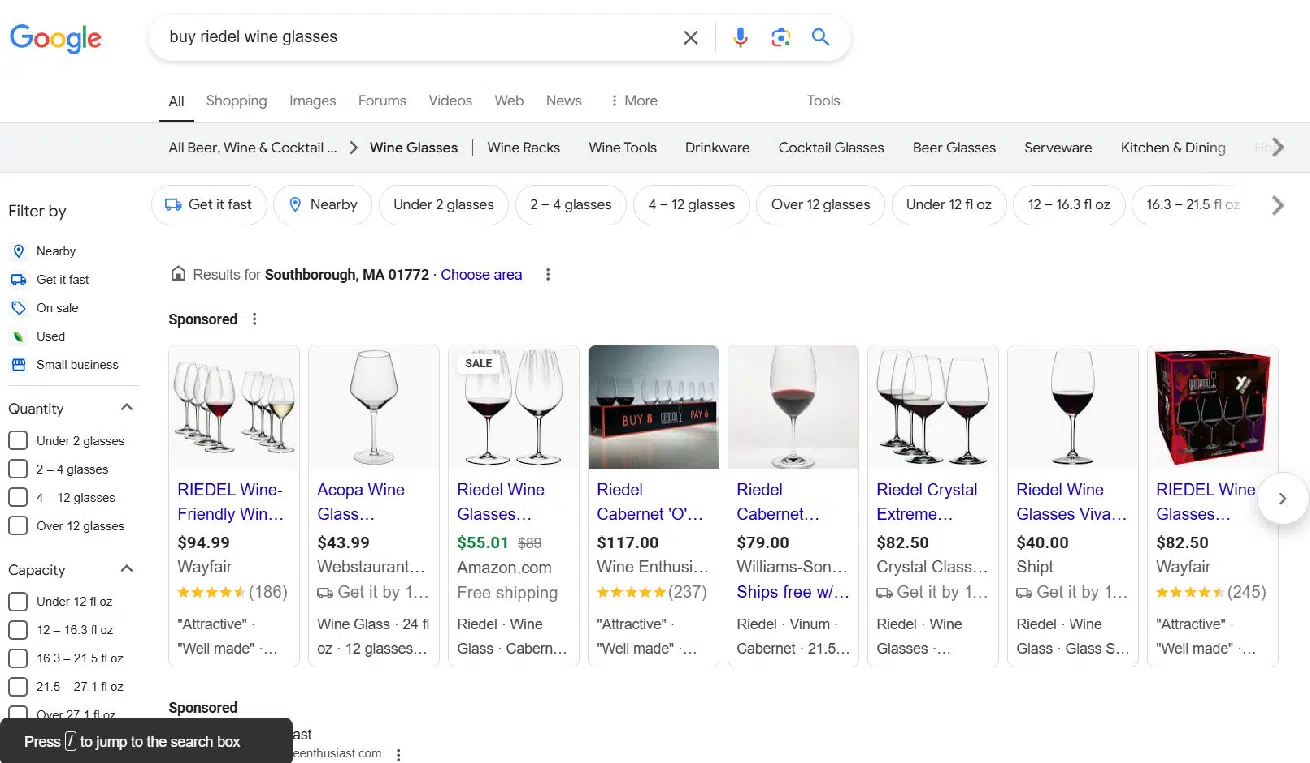
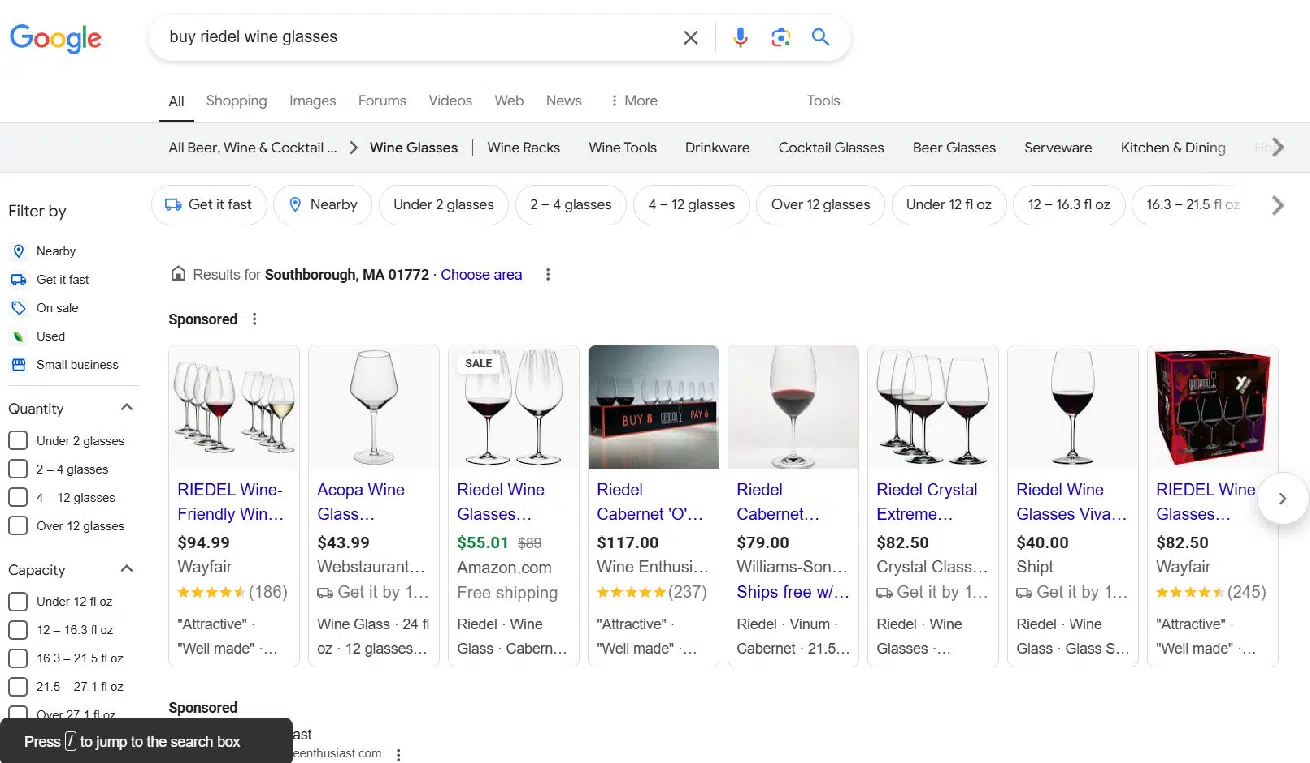
The results are dominated by Google Shopping (as shown above).
Below what I’ve shown, Google has two ads offering online buying opportunities and two pages from the Riedl website.
This result will leave a user who needs the glasses today and therefore wants to shop locally without an answer to their question.
ChatGPT search did a better job with this query as it listed both local retailers and online shopping sites.
Scores for this query:
- ChatGPT search: 6.00.
- Google: 4.00.
Problem type: Insufficient follow-on resources
As discussed in “How query sessions work” earlier in this article, it’s quite common that users will try a series of queries to get all the information they’re looking for.
As a result, a great search experience will facilitate that process.
This means providing a diverse set of resources that makes it easy for users to research and find what they want/need. When these aren’t easily accessed it offers them a poor experience.
As an example, let’s look at how ChatGPT search responds to the query “hotels in San Diego”:
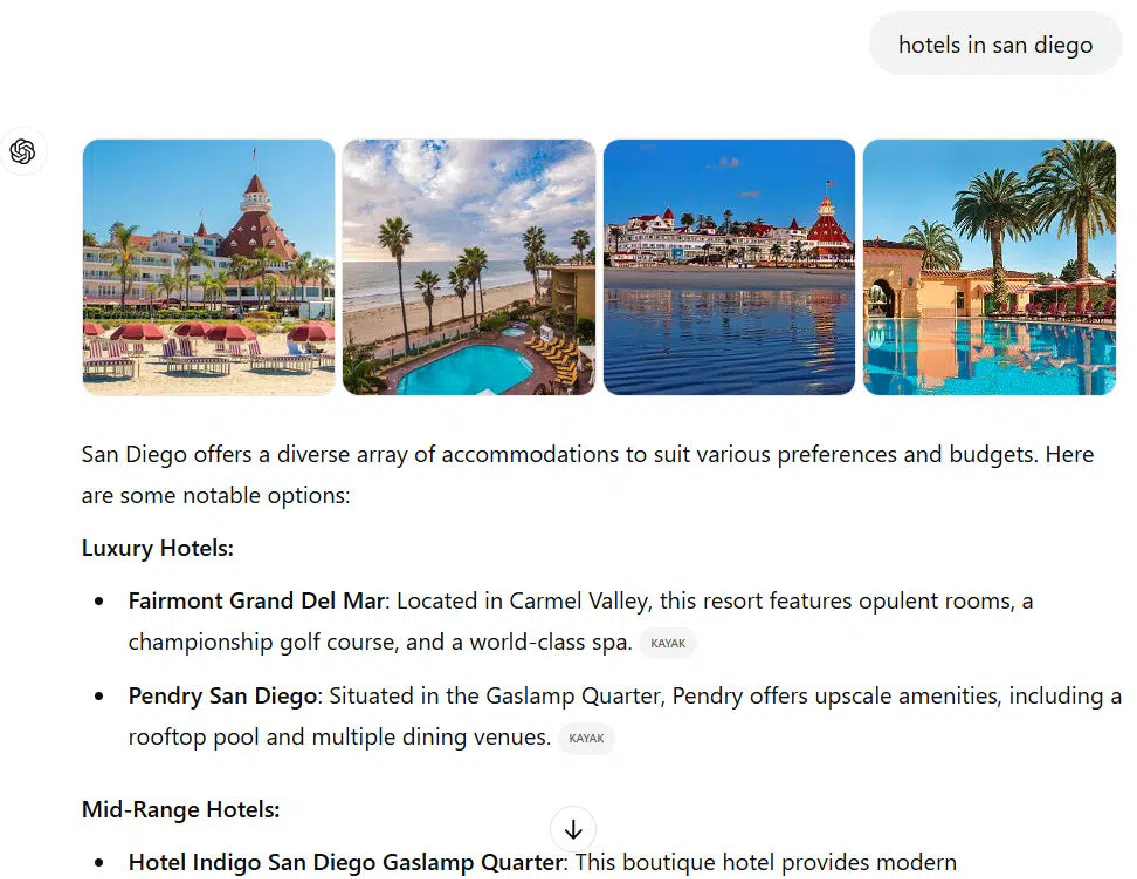
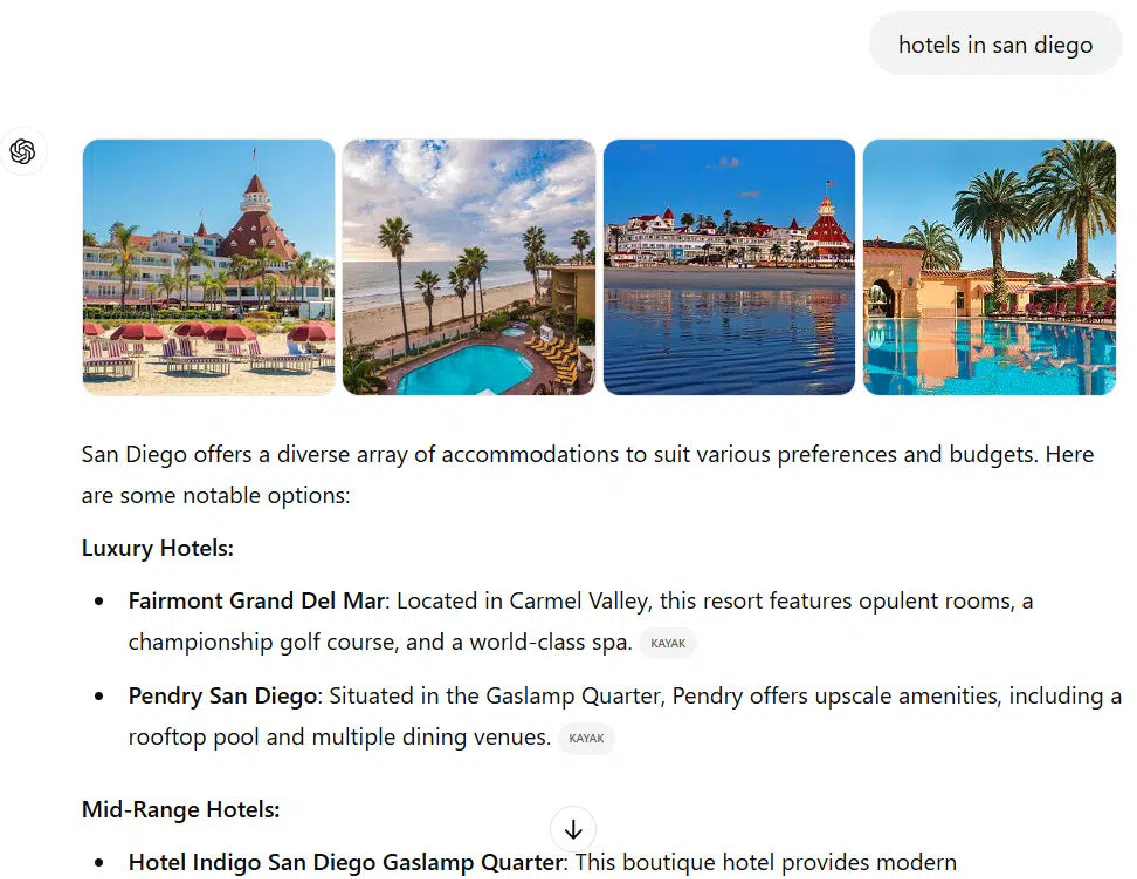
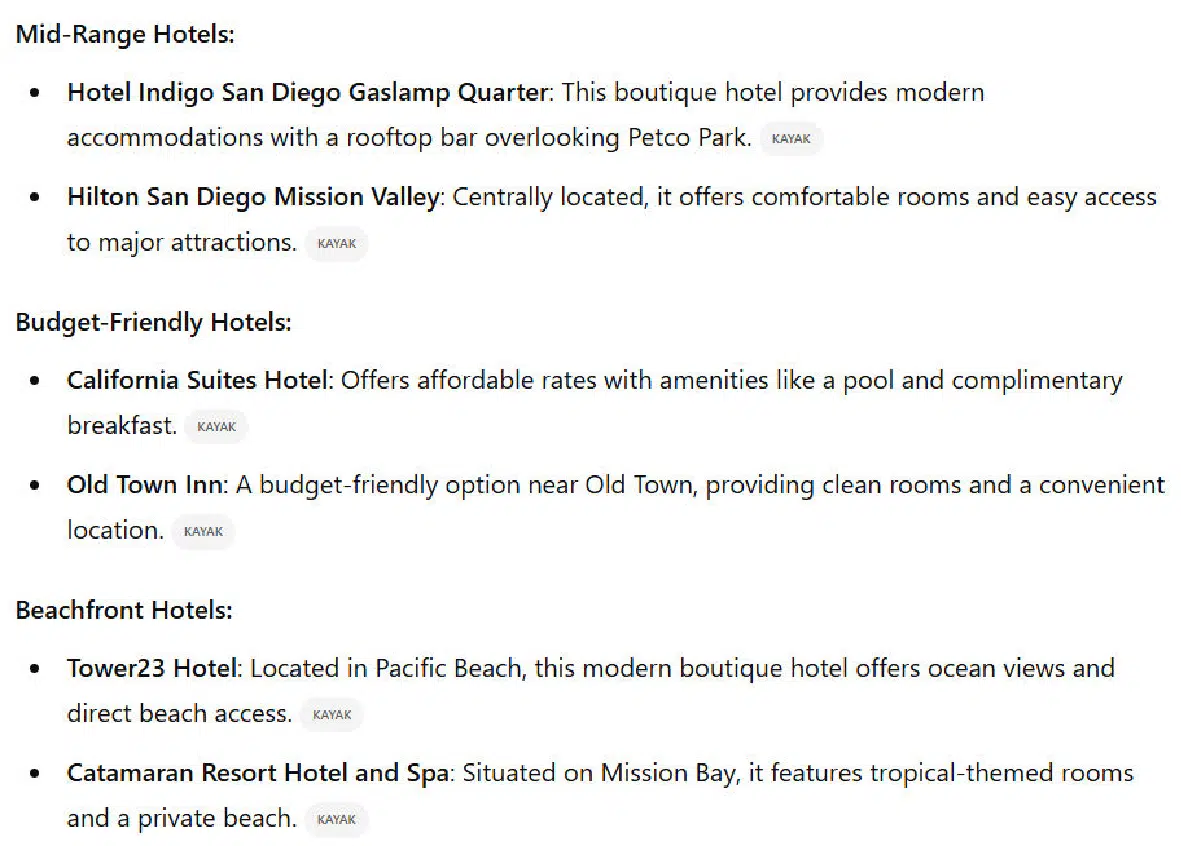
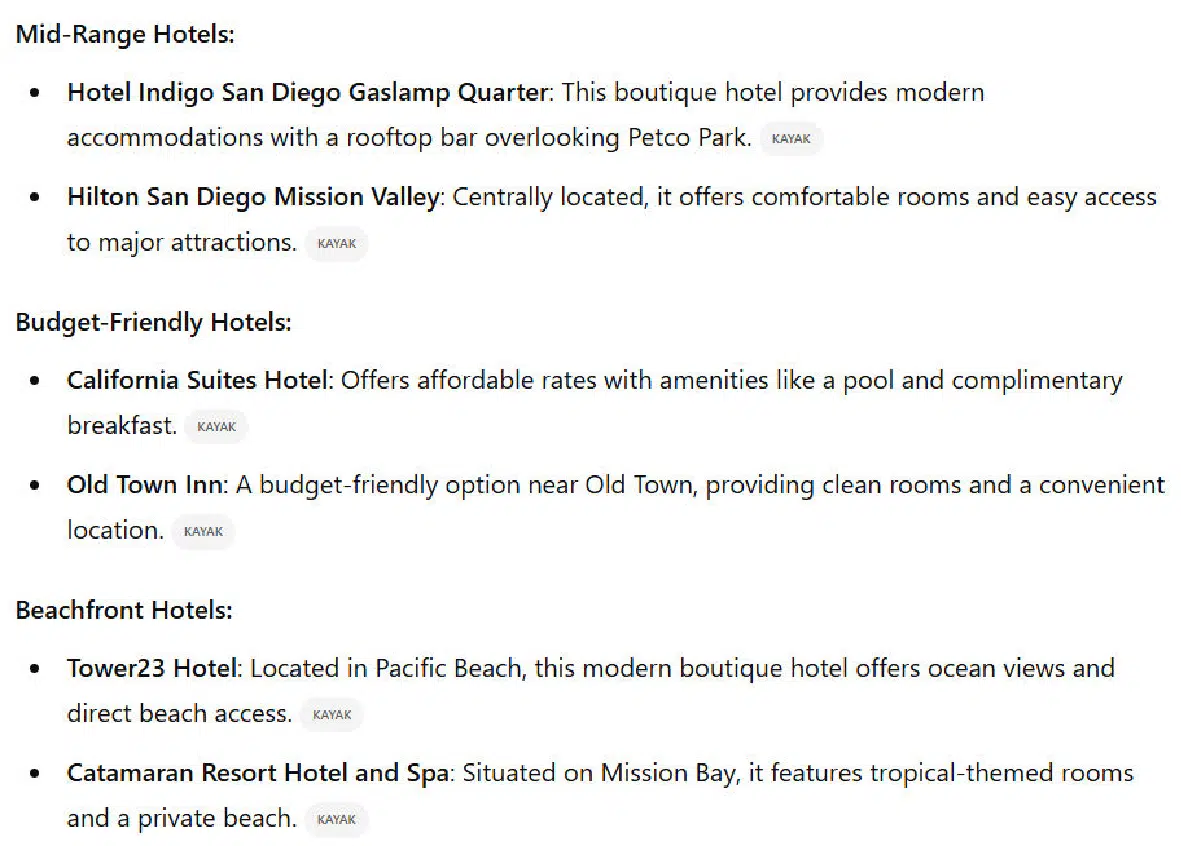
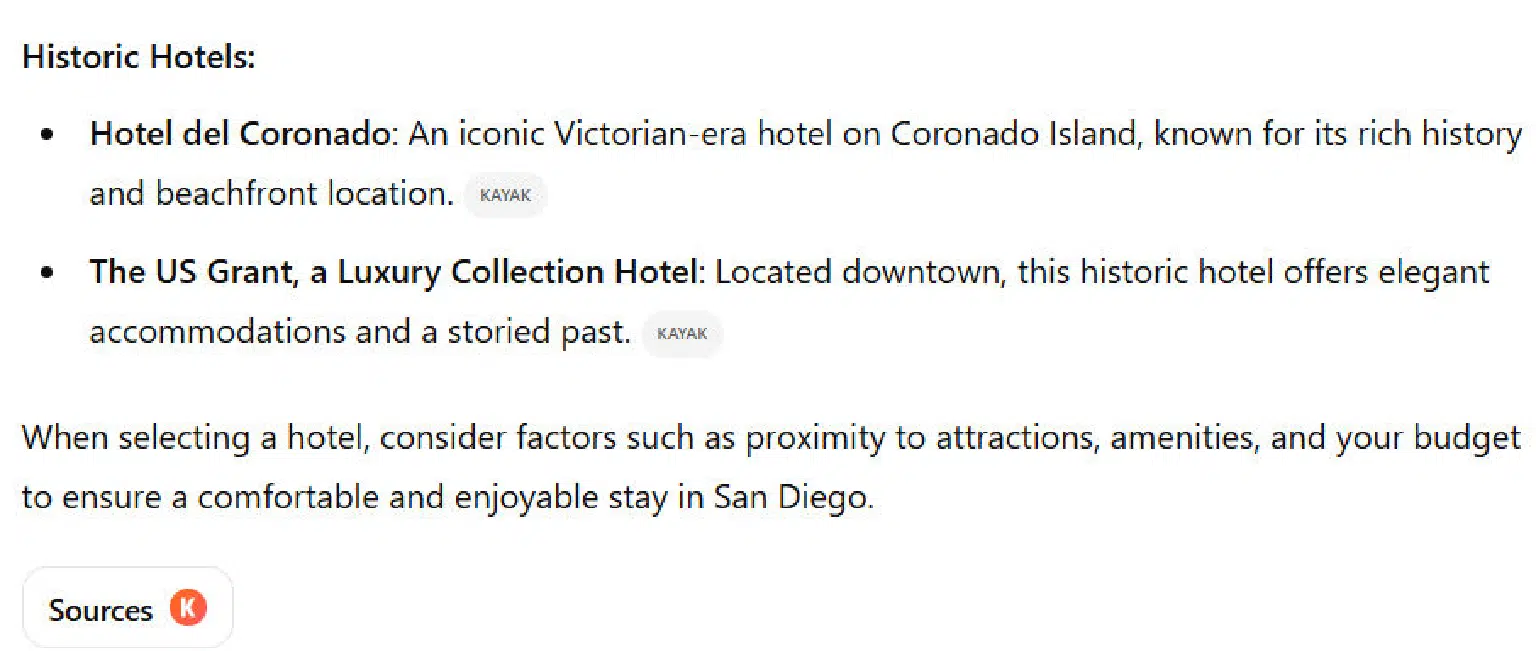
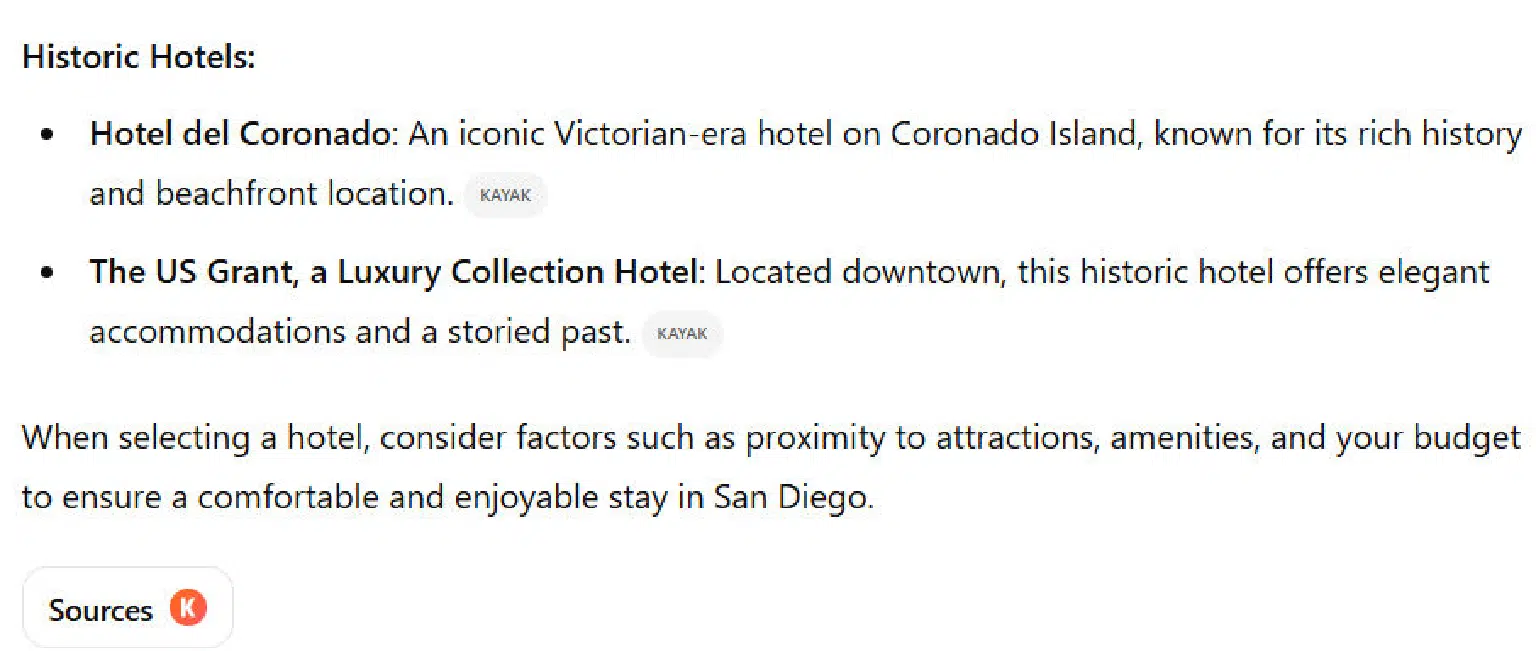
While this provides 11 hotels as options, there are far more than this throughout the San Diego area.
It’s also based on a single source: Kayak.
The user can click through to the Kayak site to get a complete list, but other resources aren’t made available to the user.
In contrast, Google’s results show many different sites that can be used to find what they want. The scores I assigned to the competitors for this one were:
- ChatGPT search: 3.00.
- Google: 6.00.
The winner?
It’s important to note that this analysis is based on a small sample of 62 queries, which is far too limited to draw definitive conclusions about all search scenarios.
A broader takeaway can be gained by reviewing the examples above to see where each platform tends to perform better.
Here’s a breakdown of category winners:
1. Informational queries
- Queries: 42
- Winner: Google
- Google’s average score: 5.83
- ChatGPT search’s average score: 5.19
Google’s slight edge aligns with its strong track record for informational searches.
However, ChatGPT Search performed respectably, despite challenges with errors, omissions, and incomplete responses.
2. Content gap analysis
- Winner: ChatGPT Search
- ChatGPT search’s average score: 3.25
- Google’s average score: 1.0
- ChatGPT Search excels in content gap analysis and related tasks, making it particularly useful for content creators. Winning use cases include:
- Content gap analysis
- Standalone content analysis
- Comparing direct or indirect SERP competitors
- Suggesting article topics and outlines
- Identifying facts/statistics with sources
- Recommending FAQs for articles
While ChatGPT search outperformed Google in this category, its lower overall score highlights areas where improvements are needed, such as accuracy.
3. Navigational queries
Navigational queries were excluded from the test since they typically don’t require detailed text responses.
Google’s dominance in this category is assumed based on its straightforward, website-focused results.
4. Local search queries
- Winner: Google
- Google’s average score: 6.25
- ChatGPT search’s average score: 2.0
Google’s extensive local business data, combined with tools like Google Maps and Waze, ensures its superiority in this category.
5. Commercial queries
- Winner: Google
- Google’s average score: 6.44
- ChatGPT search’s average score: 3.81
This category, comprising 16 queries, favored Google due to its stronger capabilities in showcasing product and service-related results.
6. Disambiguation queries
- Winner: ChatGPT search
- ChatGPT search’s average score: 6.0
- Google’s average score: 5.29
ChatGPT Search edged out Google by more effectively presenting multiple definitions or interpretations for ambiguous terms, providing users with greater clarity.
These scores are summarized in the following table:
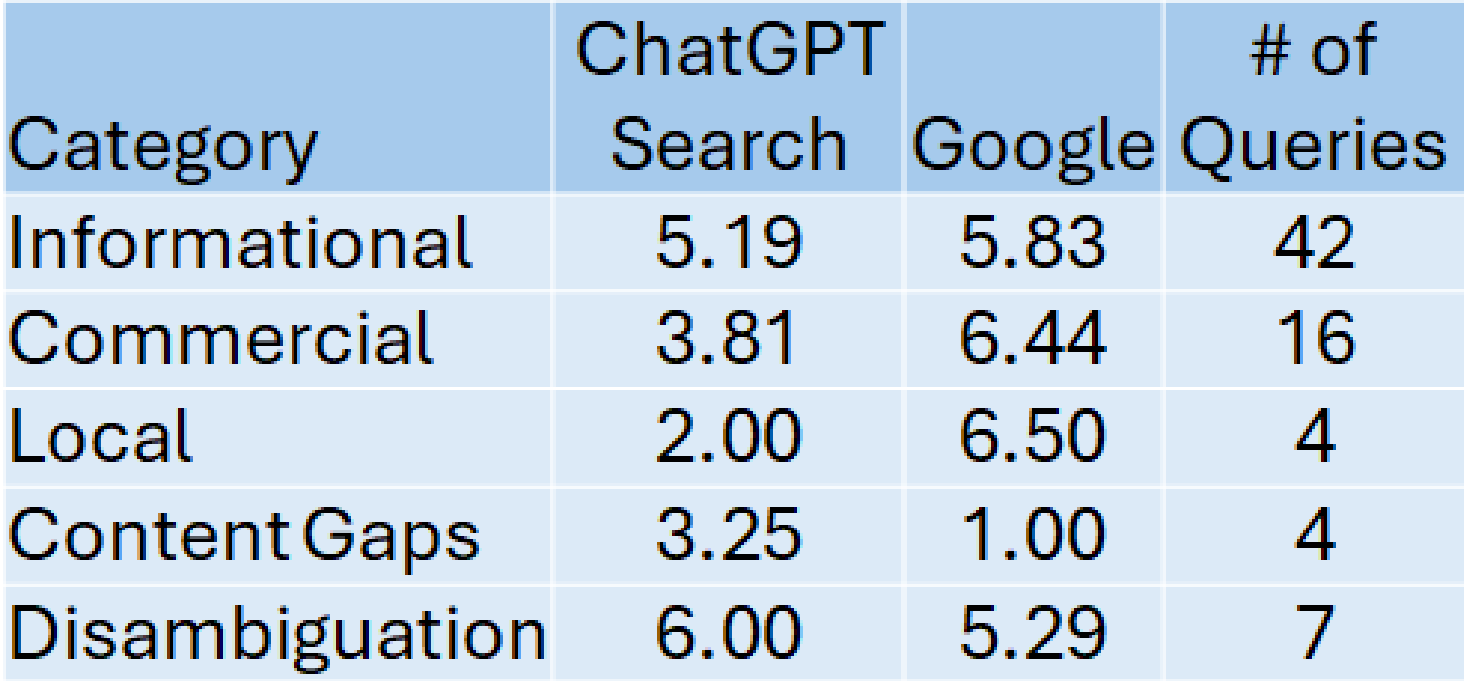
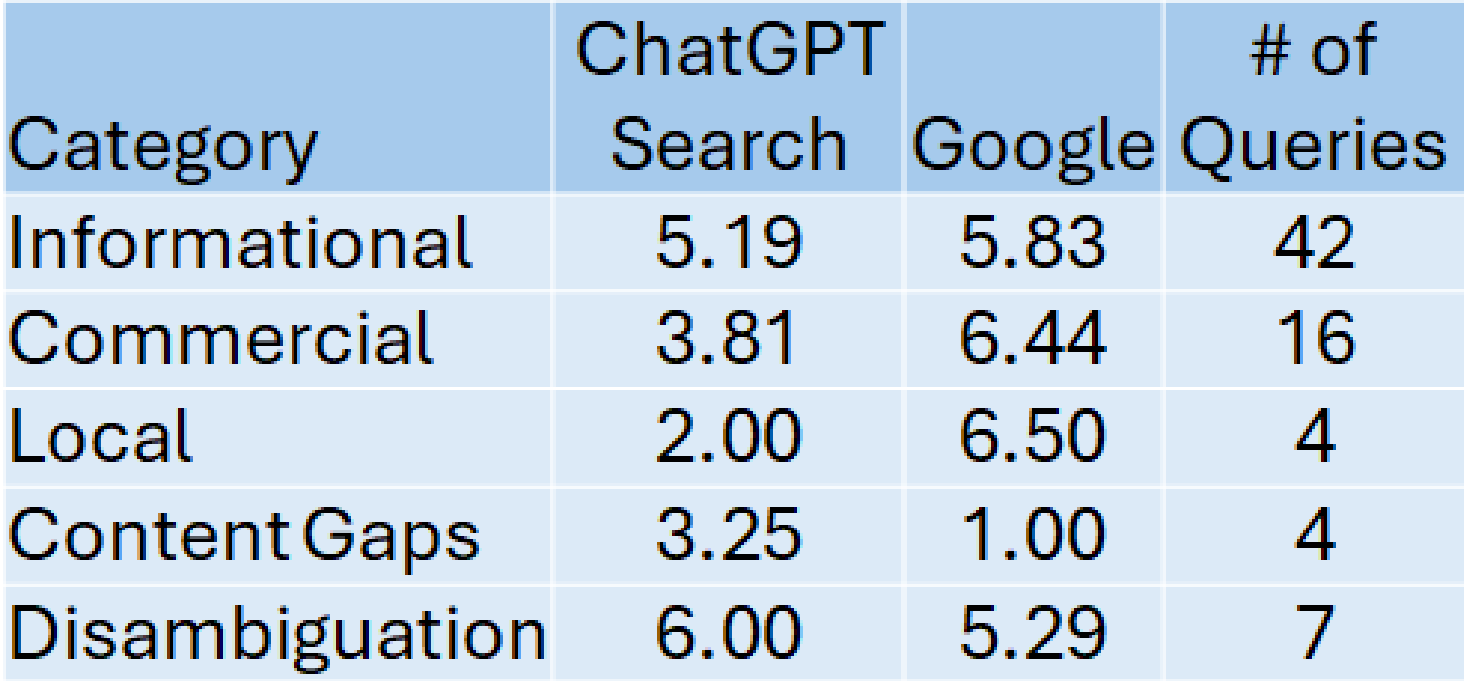
Summary
After a detailed review of 62 queries, I still see Google as the better solution for most searches.
ChatGPT search is surprisingly competitive when it comes to informational queries, but Google edged ChatGPT search out here too.
Note that 62 queries are a tiny sample when considered against the scope of all search.
Nonetheless, as you consider your search plans going forward, I’d advise you to do a segmented analysis like what I did before deciding which platform is the better choice for your projects.
Contributing authors are invited to create content for Search Engine Land and are chosen for their expertise and contribution to the search community. Our contributors work under the oversight of the editorial staff and contributions are checked for quality and relevance to our readers. The opinions they express are their own.
Noticias
Esto es lo que debes saber
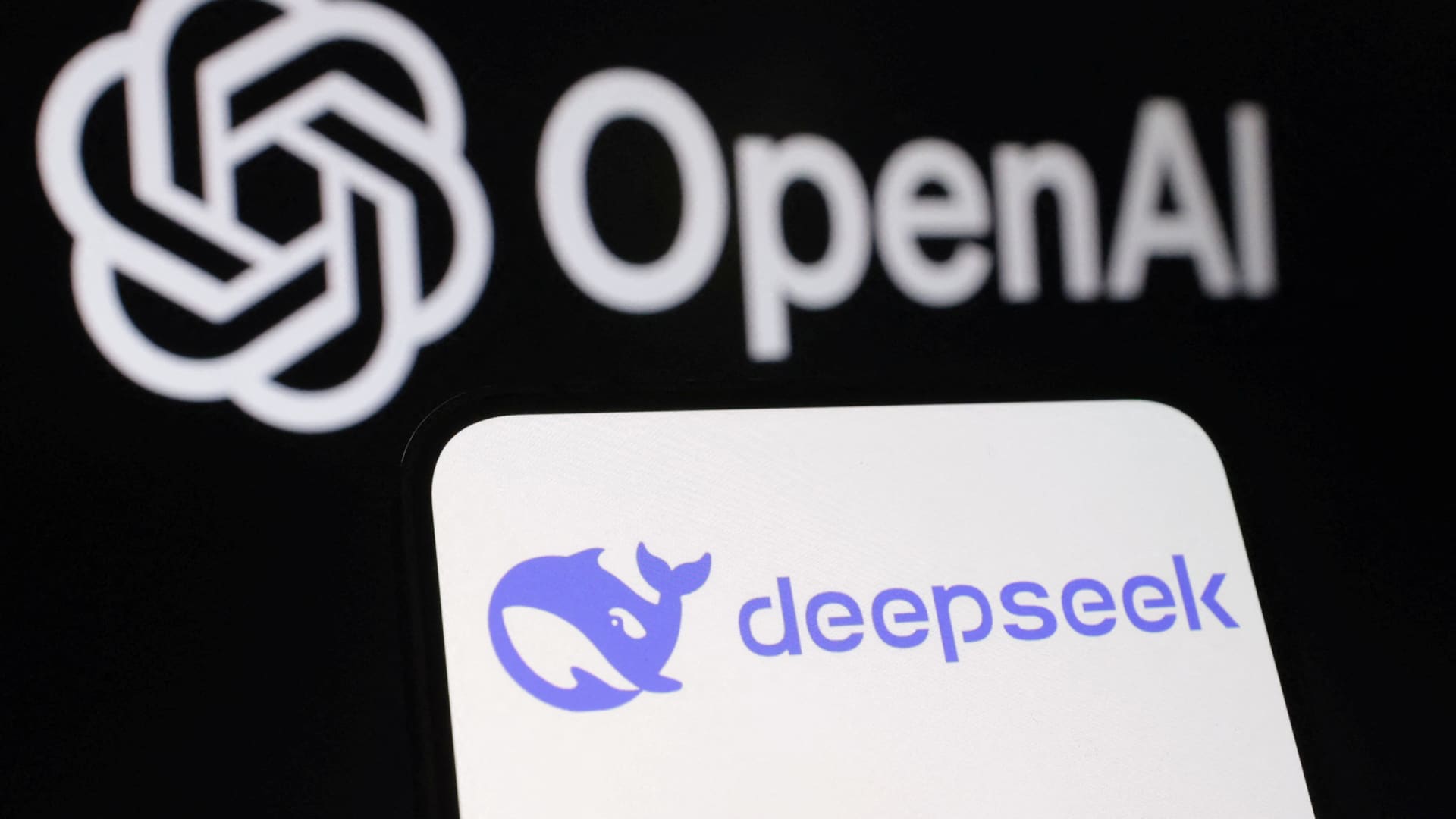
El lunes, la startup china de inteligencia artificial DeepSeek tomó el codiciado lugar de su rival OpenAI como la aplicación gratuita más descargada en los EE. UU. Manzana‘s App Store, destronando a ChatGPT para el asistente de inteligencia artificial de DeepSeek. Las acciones tecnológicas mundiales se vendieron y estaban en camino de eliminar miles de millones en capitalización de mercado.
Líderes tecnológicos, analistas, inversores y desarrolladores dicen que la exageración (y el consiguiente temor de quedarse atrás en el siempre cambiante ciclo exagerado de la IA) puede estar justificada. Especialmente en la era de la carrera armamentista generativa de la IA, donde tanto los gigantes tecnológicos como las nuevas empresas compiten para asegurarse de no quedarse atrás en un mercado que se prevé superará el billón de dólares en ingresos dentro de una década.
¿Qué es DeepSeek?
DeepSeek fue fundada en 2023 por Liang Wenfeng, cofundador de High-Flyer, un fondo de cobertura cuantitativo centrado en la IA. Según se informa, la startup de IA surgió de la unidad de investigación de IA del fondo de cobertura en abril de 2023 para centrarse en grandes modelos de lenguaje y alcanzar la inteligencia artificial general, o AGI, una rama de la IA que iguala o supera al intelecto humano en una amplia gama de tareas, que OpenAI y sus rivales dicen que lo están persiguiendo rápidamente. DeepSeek sigue siendo propiedad total de High-Flyer y financiado por ella, según analistas de Jefferies.
Los rumores en torno a DeepSeek comenzaron a cobrar fuerza a principios de este mes, cuando la startup lanzó R1, su modelo de razonamiento que rivaliza con el o1 de OpenAI. Es de código abierto, lo que significa que cualquier desarrollador de IA puede usarlo, y se ha disparado a la cima de las tiendas de aplicaciones y tablas de clasificación de la industria, y los usuarios elogian su rendimiento y capacidades de razonamiento.
Al igual que otros chatbots chinos, tiene sus limitaciones cuando se le pregunta sobre ciertos temas: cuando se le pregunta sobre algunas de las políticas del líder chino Xi Jinping, por ejemplo, DeepSeek supuestamente aleja al usuario de líneas de preguntas similares.
Otra parte clave de la discusión: el R1 de DeepSeek se construyó a pesar de que Estados Unidos limitó las exportaciones de chips a China tres veces en tres años. Las estimaciones difieren sobre cuánto cuesta exactamente el R1 de DeepSeek o cuántas GPU se incluyen en él. Los analistas de Jefferies estimaron que una versión reciente tenía un “coste de capacitación de sólo 5,6 millones de dólares (suponiendo un costo de alquiler de 2 dólares por hora y 800 horas). Eso es menos del 10% del costo de Meta‘s Llama.” Pero independientemente de las cifras específicas, los informes coinciden en que el modelo fue desarrollado a una fracción del costo de los modelos rivales por OpenAI, Anthropic, Google y otros.
Como resultado, el sector de la IA está inundado de preguntas, entre ellas si el creciente número de rondas de financiación astronómicas y valoraciones de miles de millones de dólares de la industria es necesaria, y si una burbuja está a punto de estallar.
Acciones de NVIDIA cayó un 11%, con el fabricante de chips ASML bajó más del 6%. El Nasdaq cayó más del 2% y cuatro gigantes tecnológicos… Meta, microsoft, Manzana y ASML están listos para informar sus ganancias esta semana.
Los analistas de Raymond James detallaron algunas de las preguntas que afectan a la industria de la IA este mes y escribieron: “¿Cuáles son las implicaciones para la inversión? ¿Qué dice sobre los modelos de código abierto versus los propietarios? ¿Invertir dinero en GPU es realmente una panacea? ¿Existen restricciones a las exportaciones de Estados Unidos? ¿Cuáles son las implicaciones más amplias de [DeepSeek]? Bueno, podrían ser espantosos o no ser un evento, pero tengan la seguridad de que la industria está llena de incredulidad y especulación”.
Los analistas de Bernstein escribieron en una nota el lunes que “según las muchas (ocasionalmente histéricas) tomas calientes que vimos [over the weekend,] las implicaciones van desde ‘Eso es realmente interesante’ hasta ‘Esta es la sentencia de muerte del complejo de infraestructura de IA tal como lo conocemos'”.
Cómo están respondiendo las empresas estadounidenses
Algunos directores ejecutivos de tecnología estadounidenses están luchando por responder antes de que los clientes cambien a ofertas potencialmente más baratas de DeepSeek, y se informa que Meta está iniciando cuatro “salas de guerra” relacionadas con DeepSeek dentro de su departamento de IA generativa.
microsoft El director ejecutivo Satya Nadella escribió en X que el fenómeno DeepSeek era solo un ejemplo de la paradoja de Jevons: “A medida que la IA se vuelva más eficiente y accesible, veremos cómo su uso se dispara, convirtiéndola en un bien del que simplemente no podemos tener suficiente”. “. El director ejecutivo de OpenAI, Sam Altman, tuiteó una cita que atribuyó a Napoleón y escribió: “Una revolución no se puede hacer ni detener. Lo único que se puede hacer es que uno de sus hijos le dé una dirección a fuerza de victorias”.
Yann LeCun, científico jefe de IA de Meta, escribió en LinkedIn que el éxito de DeepSeek es indicativo del cambio de rumbo en el sector de la IA para favorecer la tecnología de código abierto.
LeCun escribió que DeepSeek se ha beneficiado de parte de la tecnología propia de Meta, es decir, sus modelos Llama, y que la startup “ideó nuevas ideas y las construyó sobre el trabajo de otras personas. Debido a que su trabajo está publicado y es de código abierto, todos pueden sacar provecho de ello. Ese es el poder de la investigación abierta y del código abierto”.
Alexandr Wang, director ejecutivo de Scale AI, dijo a CNBC la semana pasada que el último modelo de IA de DeepSeek fue “revolucionario” y que su versión R1 es aún más poderosa.
“Lo que hemos descubierto es que DeepSeek… tiene el mejor rendimiento, o aproximadamente está a la par de los mejores modelos estadounidenses”, dijo Wang, añadiendo que la carrera de IA entre EE.UU. y China es una “guerra de IA”. La empresa de Wang proporciona datos de entrenamiento a actores clave de la IA, incluidos OpenAI, Google y Meta.
A principios de esta semana, el presidente Donald Trump anunció una empresa conjunta con OpenAI, Oracle y SoftBank para invertir miles de millones de dólares en infraestructura de IA en Estados Unidos. El proyecto, Stargate, fue presentado en la Casa Blanca por Trump, el director ejecutivo de SoftBank, Masayoshi Son, el cofundador de Oracle, Larry Ellison, y el director ejecutivo de OpenAI, Sam Altman. Los socios tecnológicos iniciales clave incluirán a Microsoft, Nvidia y Oracle, así como a la empresa de semiconductores Arm. Dijeron que invertirían 100.000 millones de dólares para empezar y hasta 500.000 millones de dólares en los próximos cuatro años.
IA evolucionando
La noticia de la destreza de DeepSeek también llega en medio del creciente revuelo en torno a los agentes de IA (modelos que van más allá de los chatbots para completar tareas complejas de varios pasos para un usuario) que tanto los gigantes tecnológicos como las nuevas empresas están persiguiendo. Meta, Google, Amazon, Microsoft, OpenAI y Anthropic han expresado su objetivo de crear IA agente.
Anthropic, la startup de IA respaldada por Amazon y fundada por ex ejecutivos de investigación de OpenAI, intensificó su desarrollo tecnológico durante el año pasado y, en octubre, la startup dijo que sus agentes de IA podían usar computadoras como humanos para completar tareas complejas. La capacidad de uso de computadoras de Anthropic permite que su tecnología interprete lo que hay en la pantalla de una computadora, seleccione botones, ingrese texto, navegue por sitios web y ejecute tareas a través de cualquier software y navegación por Internet en tiempo real, dijo la startup.
La herramienta puede “usar computadoras básicamente de la misma manera que nosotros”, dijo a CNBC Jared Kaplan, director científico de Anthropic, en una entrevista en ese momento. Dijo que puede realizar tareas con “decenas o incluso cientos de pasos”.
OpenAI lanzó una herramienta similar la semana pasada, introduciendo una función llamada Operador que automatizará tareas como planificar vacaciones, completar formularios, hacer reservas en restaurantes y pedir alimentos.
El microsoft-La startup respaldada lo describe como “un agente que puede ir a la web para realizar tareas por usted” y agregó que está capacitado para interactuar con “los botones, menús y campos de texto que la gente usa a diario” en la web. También puede hacer preguntas de seguimiento para personalizar aún más las tareas que realiza, como información de inicio de sesión para otros sitios web. Los usuarios pueden tomar el control de la pantalla en cualquier momento.
Noticias
Esto es lo que debes saber: NBC 6 South Florida
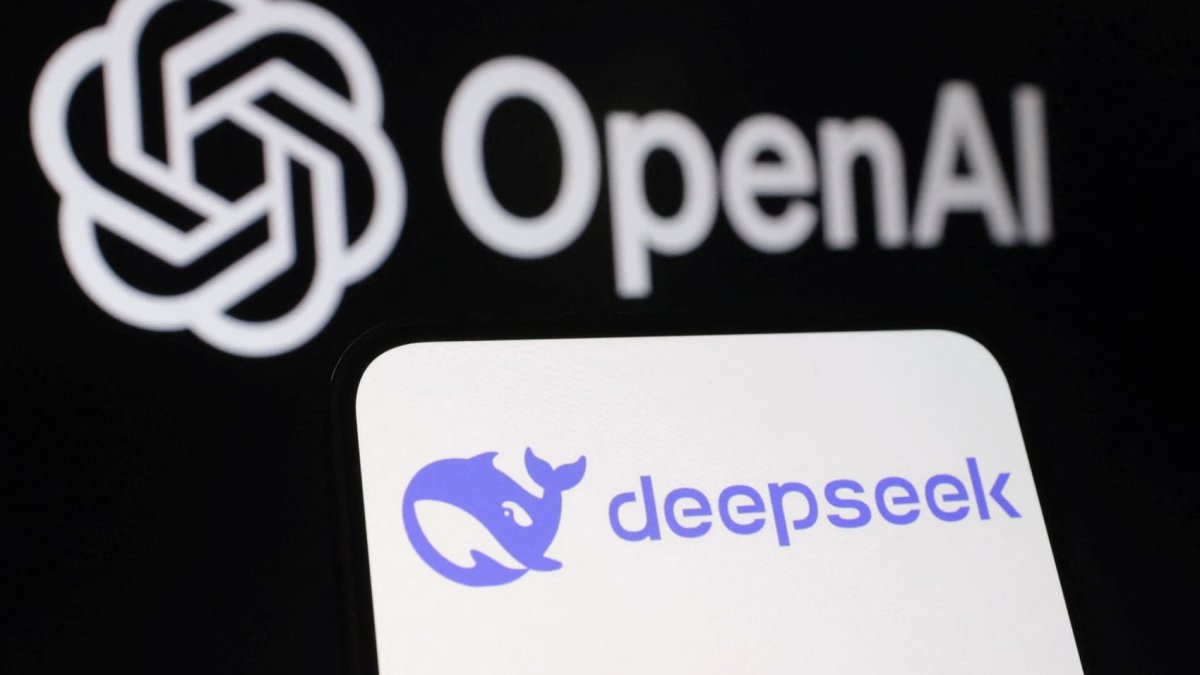
- El zumbido alrededor de la startup de IA China Deepseek comenzó a recoger a Steam a principios de este mes, cuando la startup lanzó R1, su modelo de razonamiento que rivaliza con el O1 de Openai.
- El lunes, Deepseek se hizo cargo del lugar codiciado de su rival Openai para la aplicación gratuita más descargada en los EE. UU. En la App Store de Apple, destronando a Chatgpt para el asistente de IA de Deepseek.
- Global Tech Stocks se vendió, con el gigante de chip de IA Nvidia cayendo un 10%.
El lunes, la startup de inteligencia artificial china Deepseek se hizo cargo del lugar codiciado de su rival Openai como la aplicación gratuita más desactivada en los EE. UU. En la tienda de aplicaciones de Apple, destronando Chatgpt para el asistente de IA de Deepseek. Las acciones de Global Tech se vendieron y estaban en camino de acabar con miles de millones en el límite de mercado.
Los líderes tecnológicos, analistas, inversores y desarrolladores dicen que el bombo, y el consiguiente temor de quedarse atrás en el ciclo de bombo de IA en constante cambio, pueden estar justificados. Especialmente en la era de la carrera armamentista generativa de IA, donde los gigantes tecnológicos y las startups están corriendo para garantizar que no se queden atrás en un mercado previsto para superar los ingresos de $ 1 billón en una década.
¿Qué es Deepseek?
Deepseek fue fundada en 2023 por Liang Wenfeng, cofundador de High-Flyer, un fondo cuantitativo de cobertura centrado en la IA. Según los informes, la startup de IA surgió de la Unidad de Investigación de AI del fondo de cobertura en abril de 2023 para centrarse en modelos de idiomas grandes y alcanzar la inteligencia general artificial, o AGI, una rama de IA que iguala o supere el intelecto humano en una amplia gama de tareas, que se abren. Y sus rivales dicen que están persiguiendo rápidamente. Deepseek sigue siendo propiedad y financiado por High-Flyer, según analistas de Jefferies.
El zumbido alrededor de Deepseek comenzó a recoger a Steam a principios de este mes, cuando la startup lanzó R1, su modelo de razonamiento que rivaliza con el O1 de OpenAI. Es de código abierto, lo que significa que cualquier desarrollador de IA puede usarlo, y se ha disparado a la cima de las tiendas de aplicaciones y las tablas de clasificación de la industria, con los usuarios elogios de su rendimiento y capacidades de razonamiento.
Al igual que otros chatbots chinos, tiene sus limitaciones cuando se les pregunta sobre ciertos temas: cuando se le pregunta sobre algunas de las políticas del líder chino Xi Jinping, por ejemplo, Deepseek aleja al usuario de líneas similares de preguntas.
Otra parte clave de la discusión: R1 de Deepseek se construyó a pesar de las exportaciones de chips de EE. UU. A China tres veces en tres años. Las estimaciones difieren exactamente en la cantidad de R1 de Deepseek, o en cuántas GPU entró. Los analistas de Jefferies estimaron que una versión reciente tenía un “costo de capacitación de solo US $ 5,6 millones (suponiendo un costo de alquiler de US $ 2/h800 horas). Eso es menos del 10% del costo de la LLAMA de Meta”. Pero independientemente de los números específicos, los informes acuerdan que el modelo se desarrolló a una fracción del costo de los modelos rivales por OpenAI, Anthrope, Google y otros.
Como resultado, el sector de la IA está inundado de preguntas, incluido si es necesario el creciente número de rondas de financiación astronómica y las valoraciones de mil millones de dólares, y si una burbuja está a punto de estallar.
Las acciones de NVIDIA cayeron un 11%, con el fabricante de chips ASML más del 6%. El NASDAQ cayó por 2%, y cuatro gigantes tecnológicos: Meta, Microsoft, Apple y ASML están listos para informar las ganancias esta semana.
Los analistas de Raymond James detallaron algunas de las preguntas que afectan a la industria de la IA este mes, escribiendo: “¿Cuáles son las implicaciones de inversión? ¿Qué dice sobre los modelos de origen abierto versus patentado? ¿Está arrojando dinero a las GPU realmente una panacea? trabajando? ¿Cuáles son las implicaciones más amplias de [DeepSeek]? Bueno, podrían ser terribles o un no evento, pero tengan la seguridad de que la industria está llena de incredulidad y especulación “.
Los analistas de Bernstein escribieron en una nota el lunes que “según las muchas tomas (ocasionalmente histéricas) que vimos que vimos [over the weekend,] El rango de implicaciones en cualquier lugar desde ‘eso es realmente interesante’ hasta ‘Esta es la luz de muerte del complejo de infraestructura de IA tal como lo conocemos’ “.
Cómo están respondiendo las empresas estadounidenses
Algunos CEO de tecnología estadounidense están trepando por responder antes de que los clientes cambien a ofertas potencialmente más baratas de Deepseek, y Según los informes, Meta inicia cuatro “salas de guerra” relacionadas con Deepseek dentro de su departamento generativo de IA.
El CEO de Microsoft, Satya Nadella, escribió en X que el fenómeno de Deepseek era solo un ejemplo de la paradoja de Jevons, escribiendo, “A medida que AI se vuelve más eficiente y accesible, veremos su uso Skyroocket, convirtiéndolo en una mercancía, simplemente no podemos obtener suficiente de.” El CEO de Operai, Sam Altman, tuiteó una cita que atribuyó a Napoleón, escribiendo: “Una revolución no se puede hacer ni detener.
Yann Lecun, el jefe científico de AI de Meta, escribió en LinkedIn que el éxito de Deepseek es indicativo de cambiar las mareas en el sector de IA para favorecer la tecnología de código abierto.
Lecun escribió que Deepseek se ha beneficiado de algunas de la propia tecnología de Meta, es decir, sus modelos de llama, y que la startup “se les ocurrió nuevas ideas y las construyó en la parte superior del trabajo de otras personas. Debido a que su trabajo es publicado y de código abierto, todos pueden Se beneficia de él.
Alexandr Wang, CEO de Scale AI, le dijo a CNBC la semana pasada que el último modelo de IA de Deepseek fue “devastador de la tierra” y que su lanzamiento de R1 es aún más poderoso.
“Lo que hemos encontrado es que Deepseek … es el mejor desempeño, o aproximadamente a la par con los mejores modelos estadounidenses”, dijo Wang, y agregó que la carrera de IA entre los Estados Unidos y China es una “guerra de IA”. La compañía de Wang proporciona datos de capacitación a jugadores clave de IA, incluidos OpenAI, Google y Meta.
A principios de esta semana, presidente Donald Trump anunció una empresa conjunta con OpenAI, Oracle y Softbank para invertir miles de millones de dólares en infraestructura de IA de EE. UU. El proyecto, Stargate, fue presentado en la Casa Blanca por Trump, el CEO de SoftBank, Masayoshi,, cofundador Larry Ellison, y el CEO de Operai, Sam Altman. Los socios de tecnología iniciales clave incluirán Microsoft, Nvidia y Oracle, así como el brazo de la compañía de semiconductores. Dijeron que invertirían $ 100 mil millones para comenzar y hasta $ 500 mil millones en los próximos cuatro años.
AI evolucionando
La noticia de la destreza de Deepseek también se produce en medio de la creciente exageración en torno a los agentes de IA, modelos que van más allá de los chatbots para completar tareas complejas de varios pasos para un usuario, que los gigantes tecnológicos y las startups están persiguiendo. Meta, Google, Amazon, Microsoft, Openai y Anthrope han expresado su objetivo de construir IA de agente.
Anthrope, la startup de IA respaldada por Amazon fundada por ex ejecutivos de investigación de Openai, aumentó su desarrollo de tecnología durante el año pasado, y en octubre, la startup dijo que sus agentes de IA pudieron usar computadoras como humanos para completar tareas complejas. La capacidad de uso de la computadora de Anthrope permite que su tecnología interprete lo que está en la pantalla de una computadora, seleccione botones, ingrese texto, navegue por los sitios web y ejecute tareas a través de cualquier software y navegación en Internet en tiempo real, dijo la startup.
La herramienta puede “usar computadoras básicamente de la misma manera que lo hacemos”, dijo Jared Kaplan, director científico de Anthrope, a CNBC en una entrevista en ese momento. Dijo que puede hacer tareas con “decenas o incluso cientos de pasos”.
Openai lanzó una herramienta similar la semana pasada, presentando una característica llamada operador que automatizará tareas como planificar vacaciones, completar formularios, hacer reservas de restaurantes y ordenar comestibles.
La startup respaldada por Microsoft lo describe como “un agente que puede ir a la web para realizar tareas para usted”, y agregó que está capacitado para interactuar con “los botones, los menús y los campos de texto que las personas usan a diario” en la web . También puede hacer preguntas de seguimiento para personalizar aún más las tareas que completa, como la información de inicio de sesión para otros sitios web. Los usuarios pueden tomar el control de la pantalla en cualquier momento.
Noticias
¿Por qué DeepSeek AI de repente es tan popular?
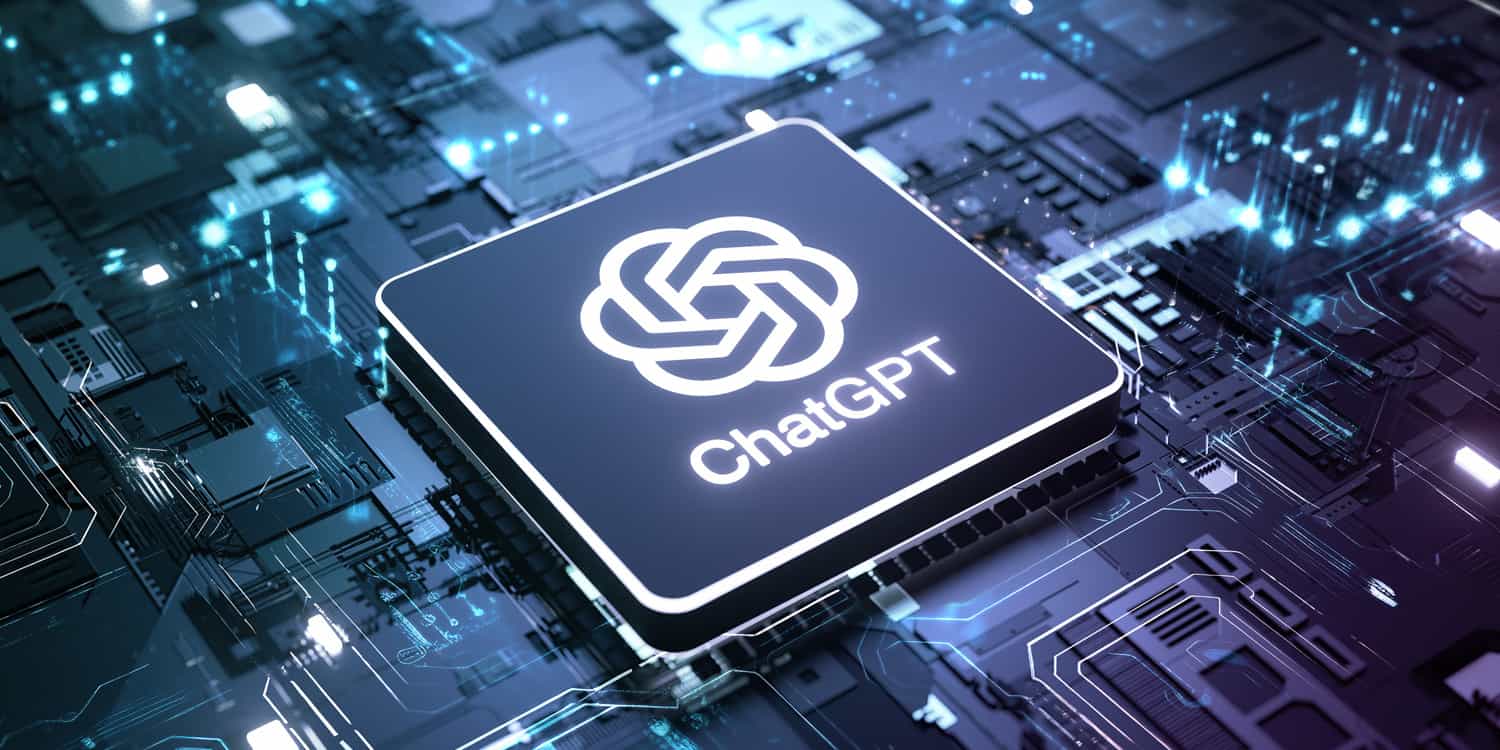
OpenAI lanzó su agente Operador AI para ChatGPT el jueves, lo que debería haber sido un hito importante para la empresa y el desarrollo de la IA en general. Si bien no pagaría $200 al mes para probar esta versión inicial de Operador, lo que vi en las demostraciones de OpenAI me dejó alucinado. El operador está muy por delante de los agentes de inteligencia artificial de Google, al menos en lo que respecta a demostraciones. No puedo esperar a tenerlo en mis manos una vez que OpenAI lo lleve a otros niveles pagos de ChatGPT y, lo que es más importante para mí personalmente, a la UE.
Sin embargo, la verdadera historia de la IA que se está apoderando del mundo no es ChatGPT, Operador o el enorme proyecto Stargate que se anunció la semana pasada. La historia de DeepSeek AI se apoderó del mundo cuando la startup china lanzó su modelo de razonamiento R1 que puede igualar el ChatGPT o1 de OpenAI.
No hay nada sorprendente en eso; Esperamos que otras empresas de IA igualen o1. Después de todo, OpenAI ya presentó o3, que debería anunciarse en unos días o semanas. Lo inusual de DeepSeek es que la empresa china hizo que sus modelos fueran de código abierto, por lo que cualquier empresa o desarrollador puede acceder a ellos e inspeccionarlos. Más interesante es el artículo de investigación sobre R1 que publicó DeepSeek, que afirma que el modelo altamente sofisticado fue entrenado a una fracción del costo del o1 de OpenAI.
La noticia de que el entrenamiento de DeepSeek R1 es posible con solo del 3% al 5% de los recursos que OpenAI necesita para un progreso similar con ChatGPT causó sensación en todo el mundo. Las acciones relacionadas con la IA se desplomaron durante las primeras operaciones del lunes, justo cuando DeepSeek saltó hasta convertirse en el número uno en la App Store, superando a ChatGPT.
Uno de los problemas del software de IA actual tiene que ver con el coste de desarrollo y uso del producto. Desarrollar modelos avanzados como o1 puede costar decenas de millones. El proceso requiere tarjetas gráficas (GPU) de alta gama que proporcionen la potencia informática y el gasto de energía necesarios.
Es por eso que los productos terminados como ChatGPT o1 no pueden estar disponibles de forma gratuita y sin limitaciones. Empresas como OpenAI necesitan cubrir costos y obtener ganancias. Es por eso que el enorme programa Stargate de 500 mil millones de dólares es una decisión tan monumental para el desarrollo de la IA, especialmente considerando la inevitable carrera armamentista de IA entre Estados Unidos y China.
Agregue las sanciones de EE. UU. que impiden que China acceda a los mismos chips y GPU de alta gama que hacen posible el desarrollo de productos ChatGPT o1, y uno pensaría que ChatGPT, Gemini, Meta AI y Claude no pueden obtener una competencia significativa de China.
Ahí es donde DeepSeek sorprendió al mundo. La startup china sabía que no podía competir contra OpenAI basándose en la potencia bruta. No podría tener acceso a la misma cantidad de GPU que acaparan empresas como OpenAI. Entonces, los investigadores de DeepSeek adoptaron otro enfoque para R1 y encontraron formas de entrenar un modelo de razonamiento avanzado sin acceso al mismo hardware.
No es sólo eso, sino que DeepSeek hizo que el acceso a R1 fuera mucho más barato que ChatGPT de OpenAI, lo cual es un avance significativo. Agregue la naturaleza de código abierto de los modelos DeepSeek y podrá ver por qué los desarrolladores acudirían en masa para probar la IA de la empresa china y por qué DeepSeek surgiría en la App Store.
Según la investigación, la startup china reemplazó la tecnología Supervised Fine-Tuning (SFT) que OpenAI utiliza para entrenar ChatGPT con Reinforcement Learning (RL) para producir resultados más rápidos y económicos. SFT se basa en mostrarle a la IA formas de resolver problemas brindando acceso a los datos para que la IA sepa qué tipo de respuestas brindar a varias indicaciones.
RL se basa en el modelo de IA, intenta descubrir las respuestas con un sistema de recompensa implementado y luego proporciona retroalimentación a la IA. RL permitió a DeepSeek mejorar las capacidades de razonamiento de R1 y superar la falta de computación. Sin embargo, como VentureBeat explica, se necesitaba algo de entrenamiento SFT, donde los humanos supervisan la IA, en las primeras fases de R1 antes de cambiar a RL.
Si bien señalé los inconvenientes obvios de depender de un rival de ChatGPT de China en este momento, no hay duda de que DeepSeek merece atención.
Como mínimo, las innovaciones que desarrollaron los investigadores de DeepSeek se pueden copiar en otros lugares para lograr avances similares. Después de todo, las primeras versiones de DeepSeek mostraron que la startup china podría haber copiado el trabajo de desarrollo de ChatGPT. Ya sea IA u otra cosa, las innovaciones tecnológicas siempre serán robadas y adaptadas.
Piénselo: a DeepSeek se le ocurrió una forma más eficiente de entrenar la IA utilizando solo unas 50.000 GPU, 10.000 de las cuales eran GPU NVIDIA compradas antes de las restricciones a las exportaciones de EE. UU. Comparativamente, empresas como OpenAI, Google y Anthropic operan con más de 500.000 GPU cada una, por VentureBeat.
Me imagino que los investigadores de estas empresas ahora están compitiendo para ver cómo y si pueden replicar el éxito de DeepSeek R1. También me imagino que encontrarán formas de hacerlo.
Con tanta computación y recursos a disposición de OpenAI, Google, Meta y Anthropic, pronto serán posibles avances similares a R1 además de lo que ya está disponible en los modelos de IA.
Además, si bien el mercado se vio afectado por las noticias sobre la IA de DeepSeek en China, no creo que el hardware, la potencia informática y la energía no importen en el futuro del desarrollo de la IA. Nuevamente, combine las innovaciones de DeepSeek con, digamos, un fondo de 500 mil millones de dólares y acceso a tarjetas gráficas de alta tecnología NVIDIA, y podría obtener las primeras fases de AGI.
Una vez que se empleen métodos similares a DeepSeek R1 para el desarrollo de ChatGPT y Gemini, los costos del acceso avanzado a la IA probablemente disminuirán para los usuarios premium. Esta sería una victoria clave para los consumidores.
Las empresas occidentales de IA no podrán mantener los costos altos y competir con DeepSeek R1 y sus sucesores. Algunos desarrolladores siempre elegirán los modelos más baratos a pesar del país de origen de la IA y el sesgo de entrenamiento. Como recordatorio, los modelos de DeepSeek mostrarán un sesgo hacia China. Este sigue siendo un software que debe cumplir con las leyes de censura locales.
Señalaré que China no se quedará de brazos cruzados. Estas son victorias tempranas. DeepSeek no está solo, ya que ByteDance también lanzó un chatbot de primer nivel. Se invertirán miles de millones de dólares en el desarrollo de la IA en el país para computación y energía. Recuerde, no todo lo que viene de China puede tomarse al pie de la letra. No está claro si los costos de entrenar DeepSeek son reales. La transparencia sólo funciona hasta cierto punto.
Afortunadamente, debido a que DeepSeek es de código abierto, otros pronto podrán ver si el entrenamiento similar al R1 se puede realizar con éxito en otros lugares.
VentureBeat hace un gran trabajo explicando las complejidades del desarrollo de DeepSeek R1 en este enlace. El documento técnico de DeepSeek que acompaña al lanzamiento de R1 el lunes se puede encontrar en GitHub.
-
Startups8 meses ago
Remove.bg: La Revolución en la Edición de Imágenes que Debes Conocer
-
Recursos9 meses ago
Cómo Empezar con Popai.pro: Tu Espacio Personal de IA – Guía Completa, Instalación, Versiones y Precios
-
Recursos9 meses ago
Suno.com: La Revolución en la Creación Musical con Inteligencia Artificial
-
Recursos8 meses ago
Perplexity aplicado al Marketing Digital y Estrategias SEO
-
Tutoriales9 meses ago
Cómo Comenzar a Utilizar ChatGPT: Una Guía Completa para Principiantes
-
Startups7 meses ago
Startups de IA en EE.UU. que han recaudado más de $100M en 2024
-
Estudiar IA8 meses ago
Curso de Inteligencia Artificial de UC Berkeley estratégico para negocios
-
Noticias7 meses ago
Dos periodistas octogenarios deman a ChatGPT por robar su trabajo