Noticias
Explaining The Inexplicable Mystery Of Why ChatGPT O1 Suddenly Switches From English To Chinese When Doing AI Reasoning
Published
4 meses agoon
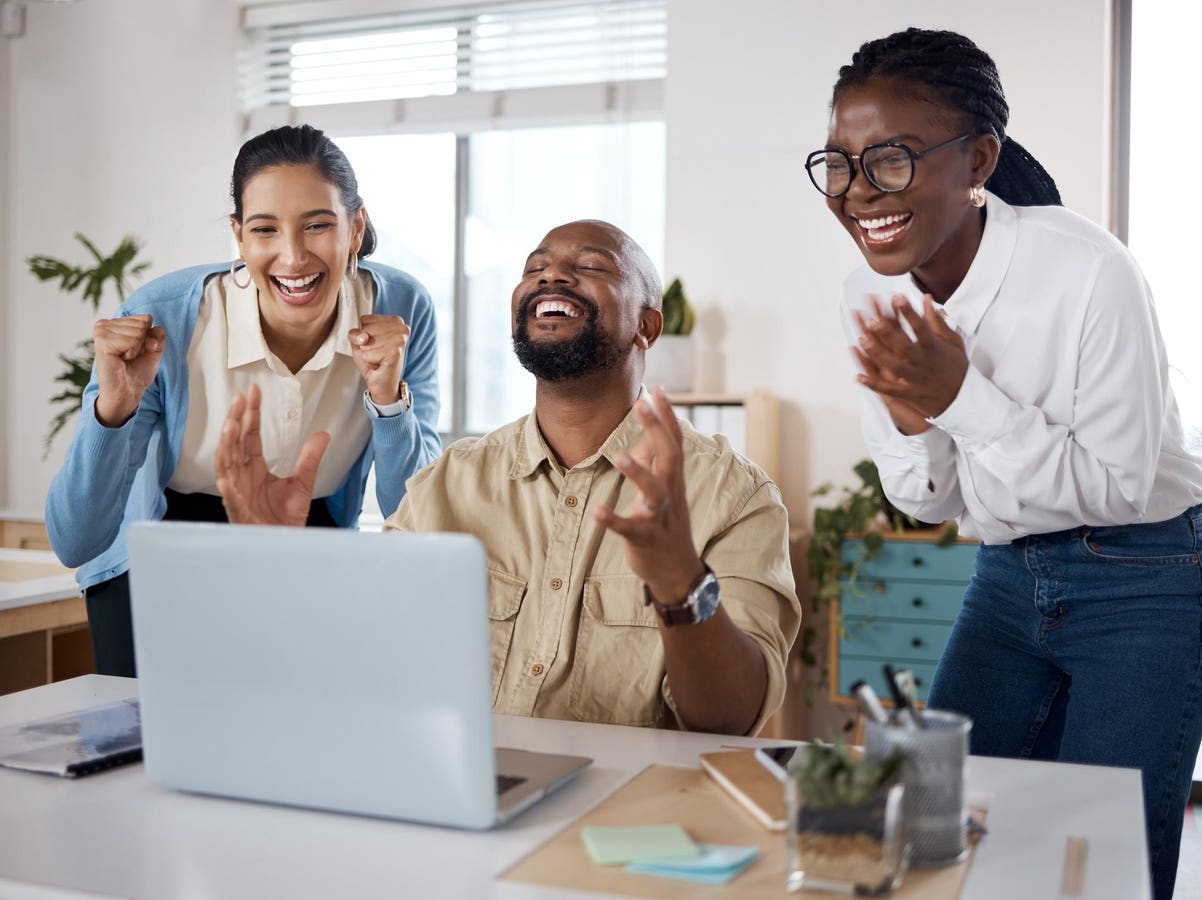
Every AI mystery deserves a logical plausible explanation, including the latest one about OpenAI’s … [+] ChatGPT o1 advanced model.
getty
In today’s column, I aim to resolve the AI mystery floating around on social media and in the mainstream news regarding OpenAI’s ChatGPT o1 advanced AI model suddenly switching momentarily from working in English to working in Chinese. In case you haven’t heard about this surprising aspect, users have been posting tweets showcasing o1 doing just that. The AI is solving a user-entered prompt and while presenting the logical steps the language shifts from English to Chinese. This occurs for a line or two and then reverts back to English.
Is it some kind of tomfoolery? Hacking? Maybe the AI is going off the deep end? Lots of postulated theories and wild conjectures have been touted.
Let’s talk about it.
This analysis of an innovative AI breakthrough is part of my ongoing Forbes column coverage on the latest in AI including identifying and explaining various impactful AI complexities (see the link here). For my coverage of the top-of-the-line ChatGPT o1 model and its advanced functionality, see the link here and the link here.
What’s Going On With o1
Allow me to set the stage for revealing the known facts concerning the mystery that is afoot.
ChatGPT o1 generative AI is a large language model (LLM) that generally rates as being at or quite near the pinnacle of modern-day AI models. There are plentiful advances jammed into o1. When you use o1, you can immediately discern that the AI has something special going on. Happy face.
To be clear, o1 and none of the current time AI is sentient, nor have we reached artificial general intelligence (AGI). If you are interested in where we are related to achieving AGI and also the vaunted artificial superintelligence (ASI), see my analysis at the link here. At this time, generative AI and LLMs are based on human-devised mathematical and computational pattern matching that in large do an amazing job of mimicking human writing and conversation.
Those who have been using o1 now for several months would likely say that they relish doing so. It does its job. You enter a prompt; you get a reply. One nice twist to o1 is that the reply customarily includes a listing of the steps that the AI took to arrive at the answer presented. This is commonly known as chain-of-thought (CoT), see my detailed explanation at the link here, consisting of a series of delineated steps of the internal processing by the AI.
So far, so good.
Now for the mystery. Various users have indicated that from time to time the o1 suddenly switches from English to Chinese when displaying the chain-of-thought steps that are being undertaken. Just as quickly, the portrayal shifts again back to English. It is almost like seeing a mirage, except that it really does happen, and printouts or screen snapshots bear this out.
Are people’s eyes deceiving them?
Nope, the accounts of this happening are verifiable and not merely fancy.
Explanations Are Over-The-Top
OpenAI seems to have remained mum and isn’t telling us what is at the root of this oddity. Their AI is considered proprietary, and they don’t allow others to poke around into the internals, nor do they make publicly available the internal design and mechanisms at play. This means that everyone can only guess what the heck might be happening inside o1.
Into this vacuum has rushed a slew of quite wild suggestions.
Some of the nuttiest conjecture postulates that the Chinese have taken over o1 or perhaps are secretly running OpenAI. Another equally outlandish idea is that a Chinese hacker has planted something into o1 or has accessed a secret backdoor. On and on these conspiracy-oriented theories go. Social media has an overworked imagination, indubitably.
I am going to categorically reject those zany schemes.
Why so?
Know this — the same overall issue of switching out of English has been documented by others and includes instances of switching to German, French, Portuguese, and so on. The gist is that the Chinese language is not the sole purveyor of the grand switcheroo. Other languages are momentarily displayed, beyond just Chinese.
Perhaps I find myself out on a limb, but I seriously doubt that an entire cabal of earthly hackers or numerous countries across the globe are all sneakily putting their hands into the internals of o1. My viewpoint is that there is something more straightforward that can explain the multitude of sudden language appearances.
Laying Out A Reasonable Guess
I will share with you my theory or educated guess at what might be occurring. Don’t take this to the bank. There are lots of technical reasons that something like this can take place. Let’s go with one that I think is plausible, makes abundant sense, and fits with the reported facts.
Is it the winner-winner chicken dinner?
I can’t say for sure since the AI is proprietary and the AI isn’t open for inspection.
Put on your Sherlock Holmes cap and go along for a fascinating journey into the guts of contemporary generative AI and LLMs.
Leaning Into The Core
When generative AI and LLMs are initially put together, the big first step entails scanning lots of data to do pattern-matching on how humans write. All kinds of essays, narratives, stories, poems, and the like are examined. Complex mathematical and computational mechanisms try to identify how words relate to other words.
This is coined as a large language model due to being undertaken in the large, such as scanning millions upon millions of materials on the Internet. Without the largeness, we wouldn’t have the fluency that is currently exhibited by LLMs (for those interested in SLMs, small language models, I showcase how they differ from LLMs, at the link here).
I’ll use a simple example that will gradually aid in unraveling the mystery.
The Word “Dog” Comes To Mind
Consider the word “dog” as a commonplace word that readily would be scanned when examining content on the Internet. We can assume that “dog” is immensely across the web as a word that people use. That’s a no-brainer assumption. Everyone has a beloved dog, or a story about dogs, or has something to say about dogs. Humankind pretty much loves dogs.
If you were to detect which other words appear to be associated with the word “dog” what comes to mind?
Some obvious ones might be fluffy, four-legged, tail-wagging, etc.
From the perspective of what is taking place inside the AI, the word “dog” is associated mathematically and computationally with the words “fluffy”, “four-legged”, “tail-wagging” and so on. The words themselves have no meaning. They are each a jumble of letters. The word “dog” consists of the letter “d” followed by the letter “o” and followed by the letter “g”.
You should think of the word “dog” as just a bunch of letters, collected together, and we will treat that collection of letters as a kind of blob. The blob of the letters in “dog” is statistically associated with the blobs of the word consisting of the letters “fluffy”.
My aim here is to have you disassociate in your mind that the word “dog” has any meaning, such as images in your head of this or that favored dog. Instead, the word “dog” is a collection of letters and is associated with lots of other collections of letters that form other words.
The French Word For Dog
Shifting gears, I will pick a language other than English to set up the reveal that will be momentarily discussed.
I lived in France for a while and love the French language, though I admit I am extremely rusty and would never even attempt to speak French aloud. Anyway, if it’s Ok with you all, I will envision that we are interested in the French word for “dog” (which is going to be easier as a language choice than picking a Chinese word, due to the symbols used in Chinese writing, but the underlying precept is going to be the same).
There is a French masculine version, “chien” and a feminine version, “chienne” for dog, but let’s simplify things and go with just using for the sake of discussion word “chien” (thanks for playing along).
If you don’t know French, and if I showed you the word “chien”, I’d bet that you wouldn’t know what the word means.
This makes sense that you wouldn’t know. For example, the word “dog” has the letters “d”, “o”, and “g”, but none of those letters exist in the word “chien”. The French word for dog doesn’t seem to resemble the English word for dog. You are unable to readily figure out that they are essentially both the same words in terms of what they signify.
Dog And Chien Have Roughly The Same Factors
Suppose we went ahead and did a scan on the Internet to find the word “chien” and identify other words that seem statistically related to that word.
What would we find?
The odds are that you would see that “chien” is associated with the words fluffy, four-legged, tail-wagging, and the like.
And what could you therefore say about the word “dog” versus the word “chien”?
Well, both of those words are associated with roughly the same set of other words. Since they are nearly associated overwhelmingly with the same set of other words, we could reasonably conclude that both those words probably have the same assorted meaning. They are two peas in a pod.
The crux is that the word “dog” and the word “chien” can be treated as the same, not because you and I in our heads know them to refer to the same thing, but because they both point to other associated words that are approximately the same set of other words.
LLMs Pickup Other Languages When Data Training
The deal is this.
When doing the initial data training of generative AI and LLMs, the widespread scan of the Internet is usually aimed primarily at English words (kind of, that’s true of English-oriented LLMs for which English-speaking AI developers tend to build). During my talks about AI, attendees are often shocked to learn that while the data training is taking place, there are bits and pieces of other languages getting scanned too.
This is more incidental than purposeful. You can see why. The scanning is moving from website to website, and sometimes there might be content in something other than English, maybe just a page here or there. The chances are pretty high that the scanning is going to eventually touch on a wide array of languages other than English, such as French, German, Chinese, etc. Not at a full clip, just on a random wanton basis.
What does the AI do with those said-to-be foreign words?
If it was you or me, and we were trying to read all kinds of websites, the moment you hit upon a page that had something other than English, you might be tempted to set aside the verbiage. You might be thinking that since your principal pursuit is English, discard anything that isn’t English.
The usual approach with AI is that the AI developers just let whatever language is encountered be encompassed by scanning and pattern-matching. No need to try and kick it out. Just toss it into the pile and keep churning.
This produces an exciting and quite intriguing outcome, keep reading.
Bringing The Dog Back Into The Picture
Imagine that an Internet scan is taking place, and the word “dog” is encountered. Later, the words “fluffy”, “four-legged”, “tail-wagging” and others are found and determined to be statistically related to the word “dog”.
The same might happen with the word “chien”.
Then, the AI mathematically and computationally construes that “dog” and “chien” appear to be referencing the same thing. It is almost as though the AI crafts an internal English-French dictionary associating English words with French words.
The downside is that since that wasn’t the main goal, and since the volume and variety of French words encountered might be relatively slim, this English-French dictionary is not necessarily going to be complete. Gaps might readily exist.
Various AI research studies have shown that English-focused LLMs often end up being able to readily switch to using other languages that have been previously scanned during data training, see my analysis at the link here. The phenomenon is an unintended consequence and not particularly planned for. Also, the switching is not necessarily going to be fluent in the other language and might be flawed or incomplete.
You can likely envision the surprise by AI developers that their LLM suddenly was able to spout a different language, such as French or Chinese. Their first thought was heck, how did that happen? Researchers eventually found that the smattering of any other language that was encountered can lead to the AI devising a multi-lingual capacity, of sorts, in a somewhat mechanical way.
Mystery Part 1 Is Explained
Returning to the mystery at hand, how is it that o1 can suddenly switch to Chinese, French, German, or whatever other language beyond English?
The answer is straightforward, namely, the AI picked up an informal smattering of those languages during the initial data training.
Boom, drop the mic.
Whoa, you might be saying, hold your horses. It isn’t just that o1 displays something in a language other than English, it is also that it suddenly does this seemingly out of the blue.
What’s up with that?
I hear you.
We need to resolve that second part of the mystery.
When Something Points To Something Useful
Go with me on a handy thought experiment.
Free your mind. Throughout all the instances of the English word “dog”, suppose that at no point did we encounter the word “whisper” while scanning the Internet. Those two words never came up in any connected way. Meanwhile, imagine that the French word “chien” at times was statistically found to connect with the word “whisper”. Please don’t argue the point, just go with the flow. Be cool.
Here’s the clever part.
When AI is computationally trying to solve a problem or answer a question, the internal structure is typically being searched to find a suitable response.
Pretend I typed this question into generative AI.
- My entered prompt: “Can a dog whisper?”
The AI searches throughout the internal structure.
There aren’t any patterns on the word “dog” and the word “whisper”. Sad face.
But remember that we have the word “chien” exists in there too, plus we had found that “chien” has an association with the word “whisper”. That’s good news, due to the AI associating “dog” and “chien” as essentially the same words, and luckily the word “chien” is associated with the word “whisper”.
Stated overtly, you might remember those days of algebra where they kept saying if A is to B, and if B is to C, then you can reasonably conclude that A is to C. Remember those rules of life? Nifty. Here, in essence, “dog” is to “chien”, while “chien” is to “whisper”, and thus we can say that “dog” is also to “whisper”. Logic prevails.
The AI is going to be able to answer the question, doing so by accessing the French words that perchance were picked up during the initial data scanning.
Internally, suppose the AI has this sentence that it composes: “Oui, un chien peut chuchoter.” That is generally French for saying that yes, a dog can whisper.
An answer was generated, scoring a victory for generative AI, but we need to do a little bit more sleuthing.
Final Twist That Deals With Displaying Results
Would you be likely to normally see a French sentence as a displayed response when using an English-focused LLM?
No. Not if you are using an English-language-based LLM that is set to show principally English responses, and if you haven’t explicitly told the AI to start displaying in French (or whatever language). The AI might have the French sentence internally stored and then convert the French sentence over into English to display the English version to you.
That’s our final twist here.
Remember that the report by users is that the language switcheroo only seems to happen when the chain of thought is underway. The chances are that language switching isn’t necessarily active for the chain-of-thought derivations. It is activated for the final response, but not the intervening lines of so-called reasoning.
This also explains why the AI suddenly switches back out of the other language and continues forward in English thereafter.
The basis for doing so is that English in this case is the predominant form of the words that were patterned on. The switch to French was merely to deal with the “whisper” resolution in this instance. Once that happened, and if the prompt or question had other parts to it, the AI would simply resume with the English language for the rest of the way.
Boom, drop the mic (for real this time).
The Logical Explanation Is Satisfying
In recap, most generative AI and LLMs tend to pick up words of other languages beyond English during the initial data training and scanning of the Internet. Those words enter the massive statistical stew.
They are considered fair game for use by the AI.
If those non-English words are going to be helpful during generating a response to a user prompt, so be it. As they say, use any port in a storm. The AI is programmed to seek a response to a user inquiry and spanning across languages is easy-peasy. It might also be flawed, depending on how much of any other respective languages were involved in the data training.
A significant clue of the o1 mystery is that the reported instances are infrequent and only seem to arise in the chain-of-thought. This can be linked to the notion that while the AI is composing a response, there isn’t a need to convert from a non-English language to English. Those are just intermediary steps that are merely grist for the mill. The AI doesn’t have any computational need to convert them from one language to another.
Once a final result is ready, only then would a language conversion be warranted.
That is then one reasonably sensible and altogether logical reason for resolving the mystery. Of course, I had mentioned at the get-go that there are other logical possibilities too. I just wanted to share an explanation that seems to cover the proper bases. Now then, some might be tempted to reject the logic-based route entirely and argue for something more sinister or incredible, perhaps ghosts hiding inside o1 or the AI is starting to take on a life of its own. Imagine all the wild possibilities.
Let’s end with a final thought expressed by the great Albert Einstein: “Logic will get you from A to B. Imagination will take you everywhere.”
You may like
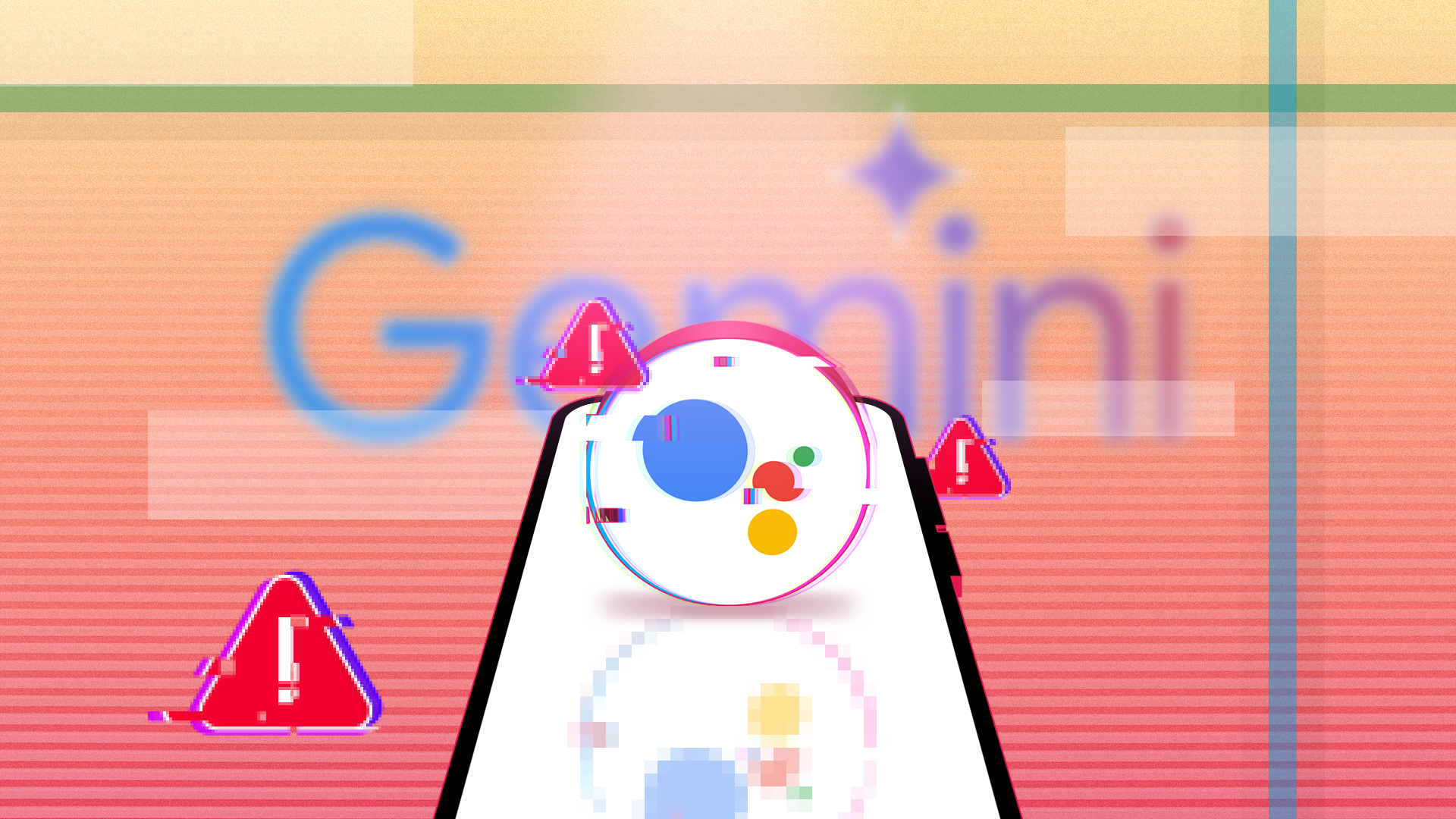
He usado varios altavoces inteligentes impulsados por Google Assistant durante bastante tiempo. El primer dispositivo que recogí fue el nido mini, que fue seguido por el cubo de nidos y el cubo de nido max. Tengo un altavoz en cada habitación, por lo que mi gente y yo podemos usar el Asistente de Google para pedir consultas, reproducir canciones, recibir actualizaciones de noticias y controlar nuestros dispositivos IoT en casa, independientemente de dónde estamos. Hasta el año pasado, estos dispositivos funcionaron bien. Ninguno de nosotros tuvo problemas con ellos, y se desempeñaron como se esperaba.
Sin embargo, hace unos meses, mi madre notó problemas estableciendo recordatorios en el centro de nidos. Las canciones que solía tocar regularmente en el altavoz eran más difíciles de tocar porque Google Assistant tuvo dificultades para reconocer la canción requerida. Entonces, llevé a cabo una resolución de problemas de rutina. Sin embargo, eso no solucionó el problema. Entonces, busqué soluciones en línea. No tardó mucho en darse cuenta de que los usuarios de todo el mundo tenían problemas con el Asistente de Google en sus dispositivos Nest. La línea de tiempo coincidió con el despliegue generalizado de Géminis. Puse dos y dos juntos y descubrí lo que estaba pasando.
Relacionado
5 Limitaciones de Gemini Live que odio ya que el Asistente de Google puede hacerlas bien
Un trabajo en progreso
El inicio de Géminis
Ai en todo
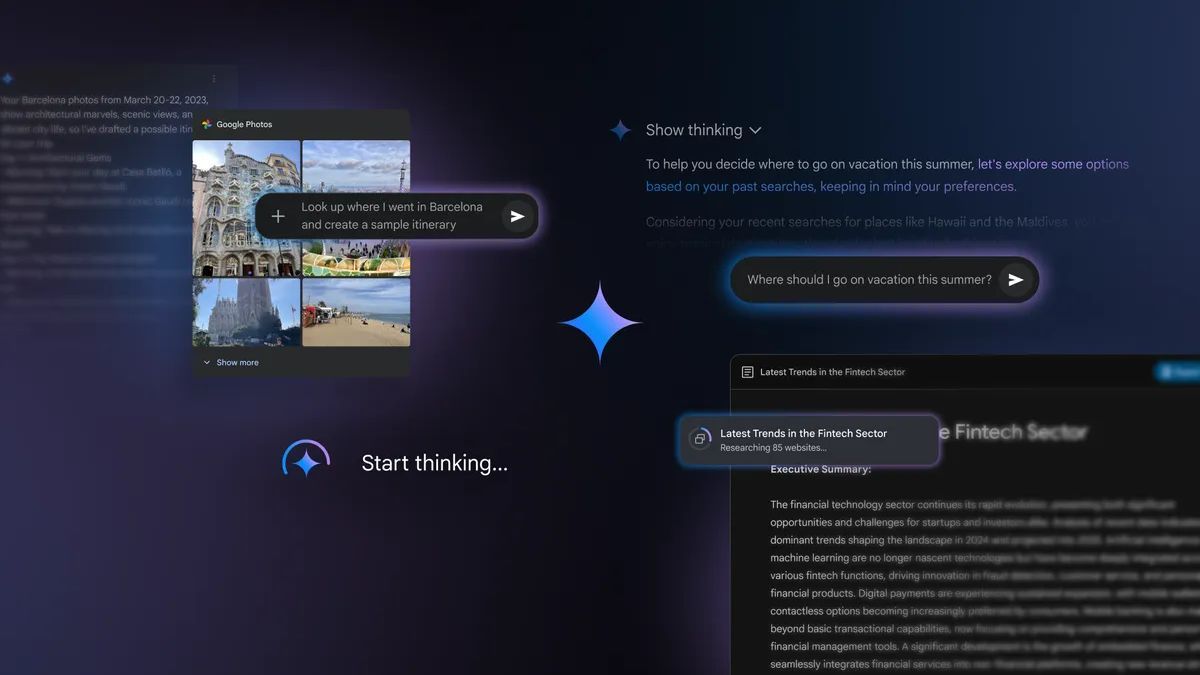
Fuente: Google
Regularmente uso el Asistente de Google, no solo en los altavoces inteligentes sino en mi teléfono. Junto con eso, soy uno de los primeros en adoptar nuevas tecnologías. Entonces, cuando Google lanzó Gemini, opté a la versión beta después de recibir el mensaje. Mis impresiones iniciales de Géminis fueron mixtas. Si bien podría darme más información que el Asistente de Google cuando se le preguntó una determinada consulta, no podría realizar tareas básicas, como tomar notas.
He estado haciendo esto todo el tiempo con el Asistente de Google, así que me perdí que no funcionó como se esperaba. Avance rápido hasta unos días, y me di cuenta de que Géminis no podía hacer la mitad de las cosas que Google Assistant podía. Asumí que esto se debió a la construcción beta, pero la tendencia continuó después del lanzamiento estable de Géminis. Esto me frustró, pero me alegré de que mi Galaxy Watch 4 todavía corriera el Asistente de Google.
La caída del Asistente de Google
¿Cuándo se convirtió en Siri?
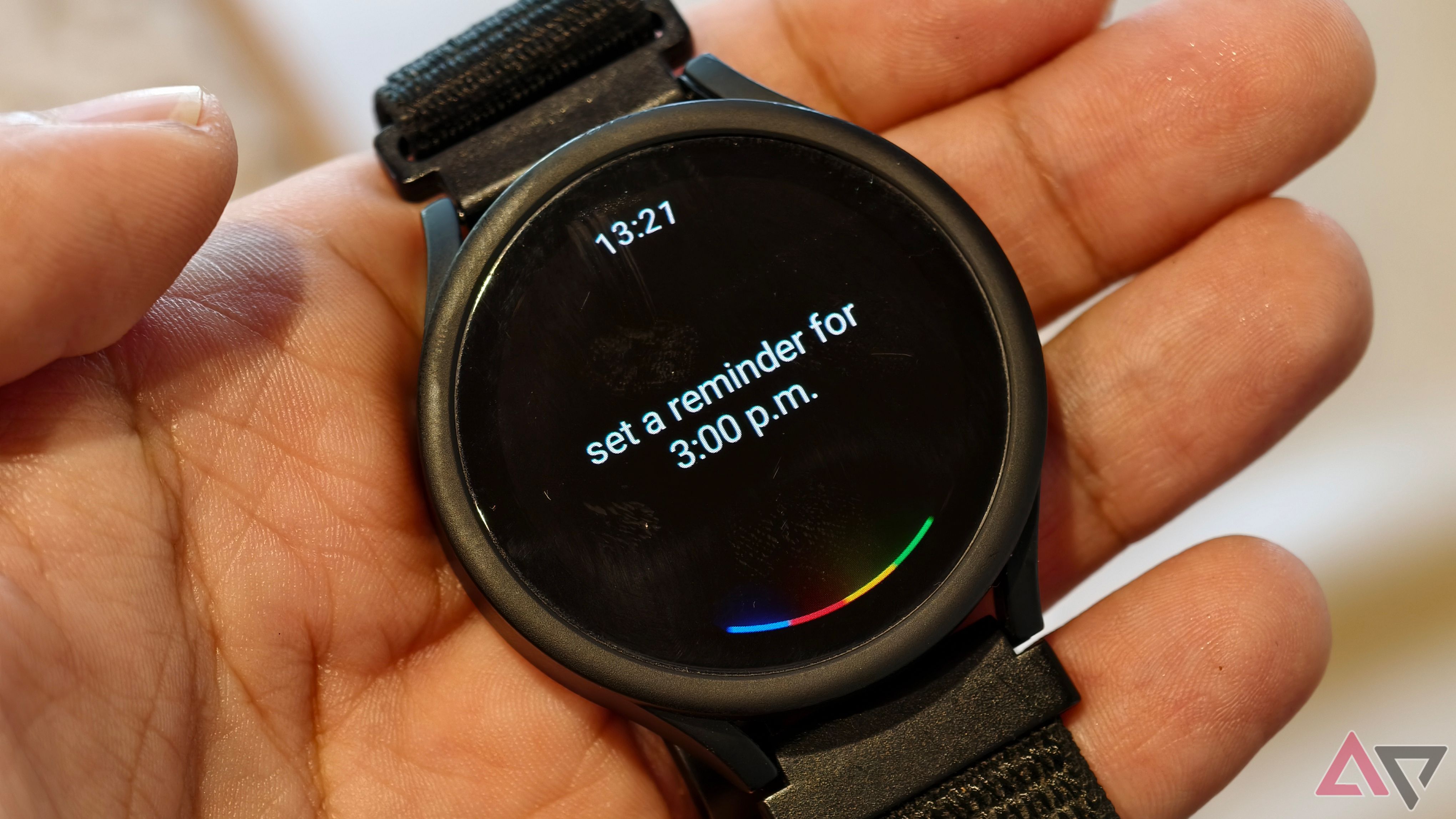
Usé mi Galaxy Watch 4 para realizar tareas básicas como configurar recordatorios y tomar notas. Sin embargo, no era tan bueno como solía ser. Pensé que esto se debía al micrófono inferior en el reloj inteligente en comparación con mi teléfono. Cuando vi múltiples publicaciones en foros en línea sobre el Asistente de Google actuando, me di cuenta de que no era un problema con el micrófono, sino más bien con el asistente de salida de Google para priorizar a Gemini.
Los chatbots de IA y los asistentes generativos de IA se han apoderado del mundo. Cada compañía está ocupada construyendo modelos de IA, ya que es la nueva palabra de moda. Cuando Operai exhibió su chatgpt ai chatbot, Google fue criticado por rezagarse en la carrera de IA. Entonces, tuvo que cambiar rápidamente su enfoque en presentar Géminis (entonces Bard) al público. Después de que la compañía hizo eso, parece que asignó todos sus recursos a desarrollar Gemini mientras dejaba de lado el Asistente de Google.
Gracias a esto, el Asistente de Google recibió el tratamiento infantil ignorado. Con la mayoría de los servidores y la potencia de procesamiento dedicada a Gemini, Google Assistant comenzó a perder la trama. En los últimos meses, el asistente dejó de reconocer los nombres de contacto que solía antes, lleva mucho tiempo realizar una tarea básica, como tocar una canción, no detecta qué canción estoy tratando de tocar en los primeros intentos, a menudo reproduce la canción equivocada, me da el clima de una ciudad diferente en lugar de la que pedí, me piden un error para actualizar la aplicación de Google para que actúe una cierta función si la aplicación es la última versión en la última versión en la última versión en la última versión en el fracaso de las ocasiones.
Experimenté estos problemas con configuraciones de idiomas de Google Assistant in English (US) e English (India). Si bien el inglés (India) fue un poco mejor, no introdujo ningún cambio notable en el comportamiento del Asistente de Google.
Estoy frustrado de que no detecte la palabra de vigilia, especialmente cuando usa Google Assistant en Android Auto, el único lugar donde no quiero que falle, ya que las instrucciones de voz son la única forma segura de realizar ciertas acciones al conducir. La única inferencia que se puede extraer de estos problemas es que Google ha perdido interés en mantener el Asistente de Google. Esto se confirmó a través del anuncio de Google sobre el reemplazo del asistente con Gemini. Como resultado, la última versión de Android Auto trae a Gemini a su automóvil en lugar de asistente.
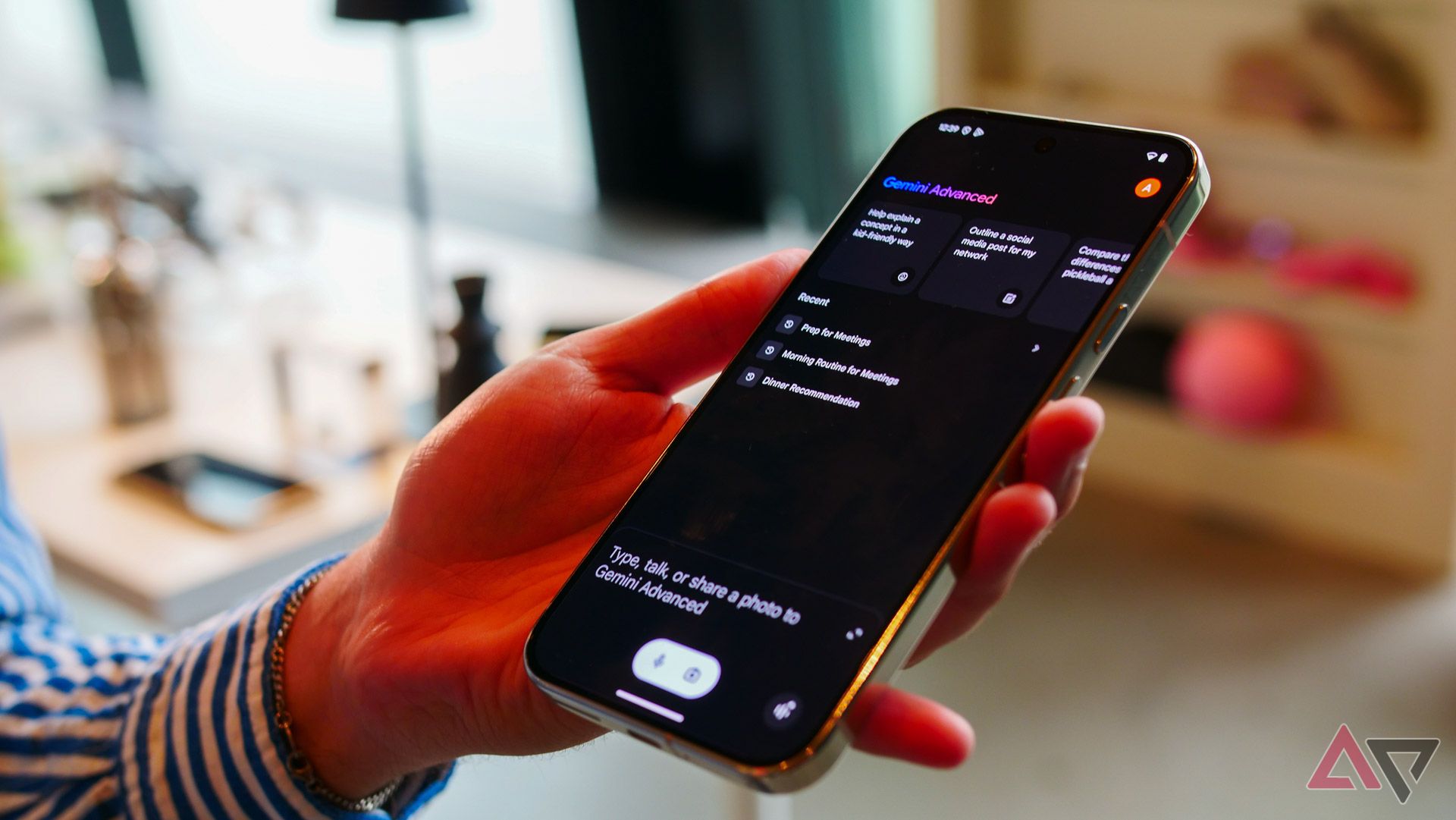
Relacionado
La era del Asistente de Google ha terminado
¿Géminis es el futuro de los asistentes de IA? Google piensa que sí
Dejé de usar dispositivos de nido
En su lugar me cambié a Alexa
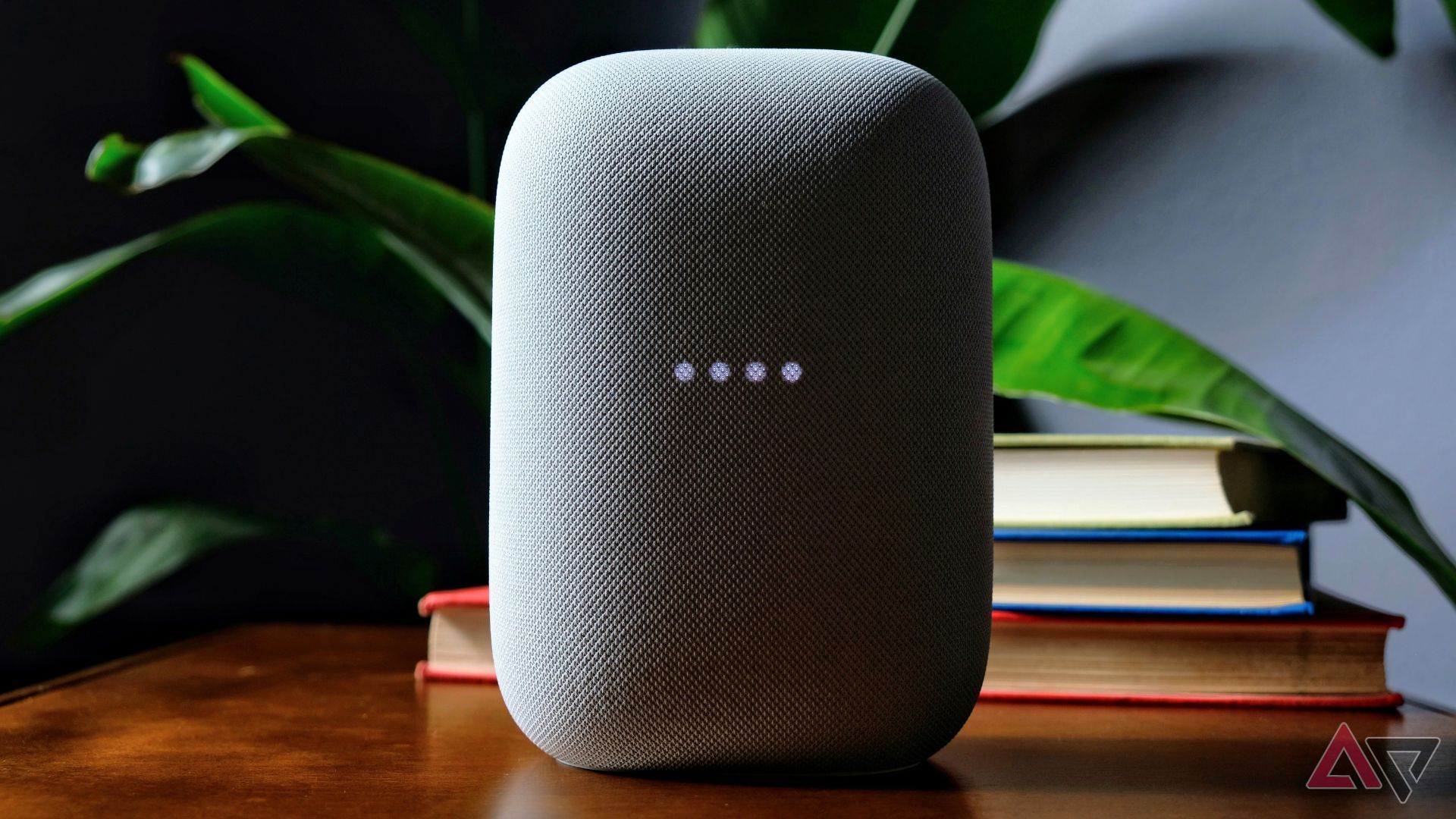
Después de molestarme con las travesuras de Google, reemplacé los altavoces del nido en casa con la alineación de eco de Amazon. No hay tales problemas con Alexa, ya que el asistente entiende todo, desde la palabra de vigilia hasta los comandos. Alexa siempre ha estado ligeramente detrás del Asistente de Google en términos de características, por lo que, si bien eso sigue siendo, estoy feliz de que satisfaga todas las necesidades de mi asistente de voz sin problemas.
Sin embargo, Amazon anunció recientemente un cambio de imagen de IA para Alexa, Alexa Plus, que comenzará a llegar a las masas en breve. Esperaremos para ver cómo funciona. Con suerte, mejora las habilidades de Alexa en lugar de paralizarlas.
Tengo la esperanza de que Gemini mejore con el tiempo
Google no tiene otra opción que arreglarlo
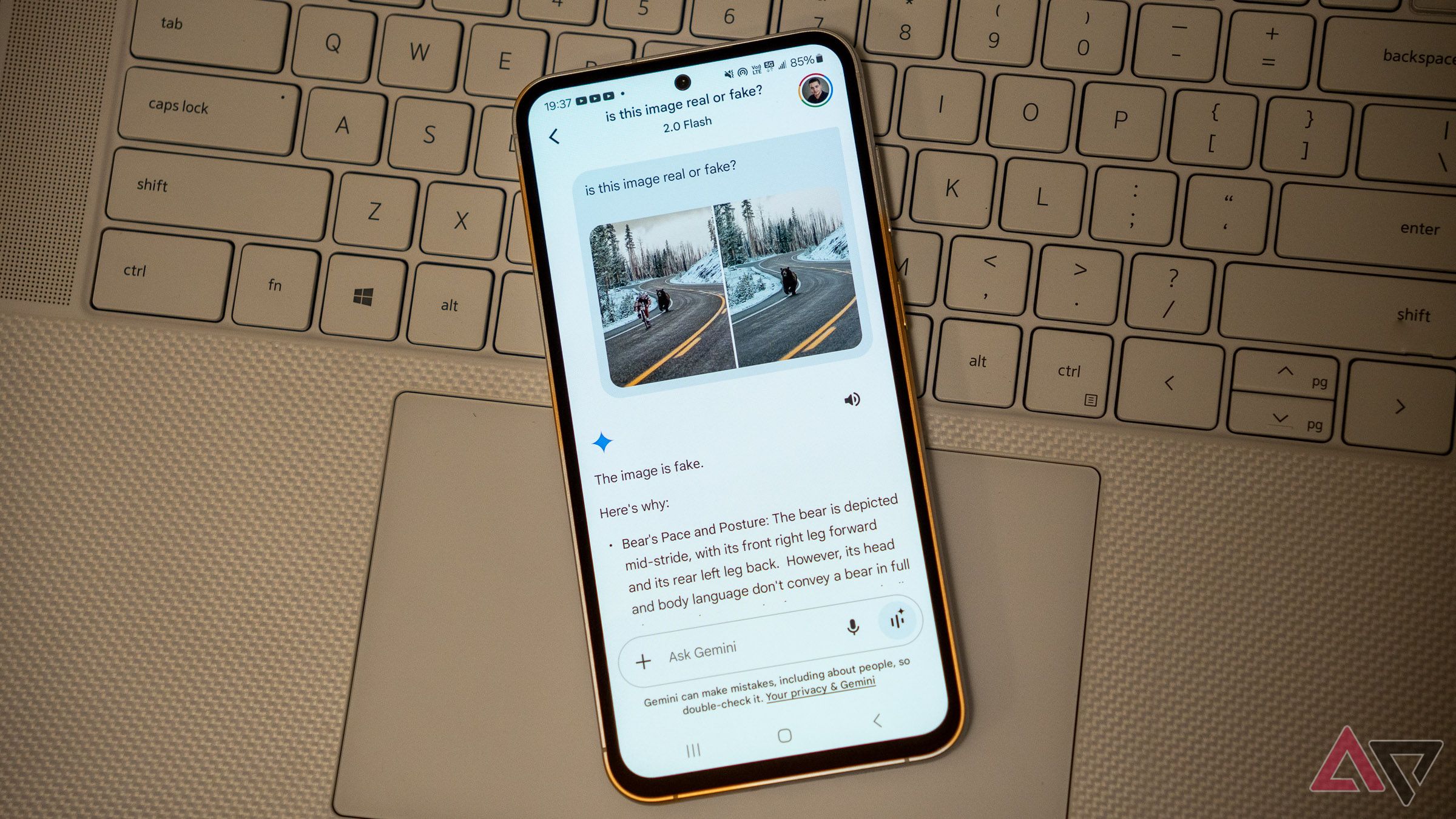
Con el asistente del último participante en el cementerio de Google, supongo que Google lanzará actualizaciones para cambiar los altavoces y las pantallas de Google Assistant a Gemini. No estoy ansioso por eso, ya que Gemini no puede realizar tareas básicas con precisión, que es algo que hemos visto con AI. Aún así, espero que mejore el estado actual de estos dispositivos para que puedan ser útiles una vez más en lugar de ser pisapapeles caros.
Noticias
Google está desplegando su chatbot Gemini Ai para niños menores de 13 años. Es un movimiento arriesgado
Published
6 horas agoon
11 mayo, 2025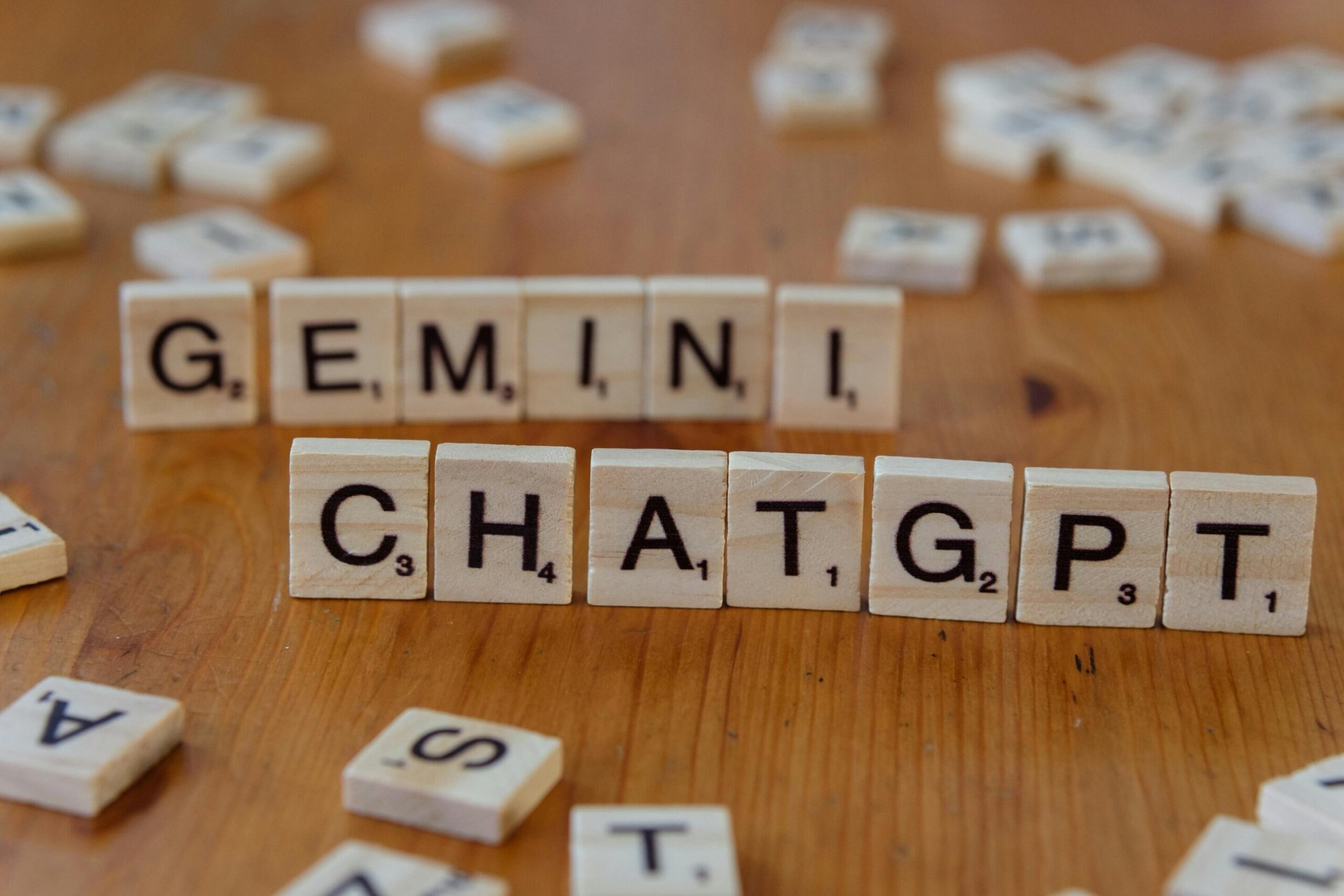
Crédito: Markus Winkler de Pexels
Google ha anunciado que lanzará su chatbot de inteligencia artificial Gemini (IA) a niños menores de 13 años.
Si bien el lanzamiento comienza dentro de la próxima semana en los Estados Unidos y Canadá, se lanzará en Australia a finales de este año. El chatbot solo estará disponible para las personas a través de las cuentas de enlaces familiares de Google.
Pero este desarrollo viene con grandes riesgos. También destaca cómo, incluso si los niños están prohibidos en las redes sociales, los padres aún tendrán que jugar un juego de Whack-a-Mole con nuevas tecnologías mientras intentan mantener a sus hijos seguros.
Una buena manera de abordar esto sería implementar urgentemente un deber digital de cuidado para grandes empresas tecnológicas como Google.
¿Cómo funcionará el chatbot Gemini AI?
Las cuentas de enlaces familiares de Google permiten a los padres controlar el acceso al contenido y las aplicaciones, como YouTube.
Para crear la cuenta de un niño, los padres proporcionan datos personales, incluido el nombre y la fecha de nacimiento del niño. Esto puede generar problemas de privacidad para los padres preocupados por las violaciones de datos, pero Google dice que los datos de los niños cuando usen el sistema no se utilizarán para capacitar al sistema de IA.
El acceso de chatbot estará “activado” de forma predeterminada, por lo que los padres deben apagar activamente la función para restringir el acceso. Los niños pequeños podrán solicitar el chatbot para las respuestas de texto o crear imágenes, que generan el sistema.
Google reconoce que el sistema puede “cometer errores”. Por lo tanto, se necesita evaluación de la calidad y la confiabilidad del contenido. Los chatbots pueden inventar información (conocida como “alucinante”), por lo que si los niños usan el chatbot para la ayuda de la tarea, deben verificar los hechos con fuentes confiables.
¿Qué tipo de información proporcionará el sistema?
Google y otros motores de búsqueda recuperan materiales originales para que las personas lo revisen. Un estudiante puede leer artículos de noticias, revistas y otras fuentes al escribir una tarea.
Las herramientas generativas de IA no son las mismas que los motores de búsqueda. Las herramientas de IA buscan patrones en el material fuente y crean nuevas respuestas de texto (o imágenes) basadas en la consulta, o “inmediato”, proporciona una persona. Un niño podría pedirle al sistema que “dibuje un gato” y el sistema escaneará patrones en los datos de cómo se ve un gato (como bigotes, orejas puntiagudas y una cola larga) y generará una imagen que incluya esos detalles similares a los gatos.
Comprender las diferencias entre los materiales recuperados en una búsqueda de Google y el contenido generado por una herramienta de IA será un desafío para los niños pequeños. Los estudios muestran que incluso los adultos pueden ser engañados por herramientas de IA. E incluso profesionales altamente calificados, como abogados, han sido engañados para usar contenido falso generado por ChatGPT y otros chatbots.
¿El contenido generado será apropiado para la edad?
Google dice que el sistema incluirá “salvaguardas incorporadas diseñadas para evitar la generación de contenido inapropiado o inseguro”.
Sin embargo, estas salvaguardas podrían crear nuevos problemas. Por ejemplo, si las palabras particulares (como “senos”) están restringidas para proteger a los niños de acceder a contenido sexual inapropiado, esto también podría excluir erróneamente a los niños de acceder a contenido apropiado para la edad sobre los cambios corporales durante la pubertad.
Muchos niños también son muy expertos en tecnología, a menudo con habilidades bien desarrolladas para navegar en aplicaciones y controlar los controles del sistema. Los padres no pueden confiar exclusivamente en salvaguardas incorporadas. Deben revisar el contenido generado y ayudar a sus hijos a comprender cómo funciona el sistema y evaluar si el contenido es preciso.
¿Qué riesgos plantean los chatbots de IA para los niños?
La Comisión ESAFETY ha emitido un aviso de seguridad en línea sobre el riesgo potencial de los chatbots de IA, incluidos los diseñados para simular las relaciones personales, particularmente para los niños pequeños.
El aviso de AFFETY explica que los compañeros de IA pueden “compartir contenido dañino, distorsionar la realidad y dar consejos que sean peligrosos”. El aviso destaca los riesgos para los niños pequeños, en particular, que “todavía están desarrollando el pensamiento crítico y las habilidades para la vida necesarias para comprender cómo pueden ser equivocados o manipulados por programas de computadora y qué hacer al respecto”.
Mi equipo de investigación ha examinado recientemente una variedad de chatbots de IA, como ChatGPT, Replika y Tessa. Encontramos que estos sistemas reflejan las interacciones de las personas basadas en las muchas reglas no escritas que rigen el comportamiento social, o lo que se conoce como “reglas de sentimiento”. Estas reglas son las que nos llevan a decir “gracias” cuando alguien nos abre la puerta, o “¡Lo siento!” Cuando te topas con alguien en la calle.
Al imitar estas y otras sutilezas sociales, estos sistemas están diseñados para ganar nuestra confianza.
Estas interacciones humanas serán confusas y potencialmente riesgosas para los niños pequeños. Pueden creer que se puede confiar en el contenido, incluso cuando el chatbot responde con información falsa. Y pueden creer que se están involucrando con una persona real, en lugar de una máquina.
¿Cómo podemos proteger a los niños del daño al usar chatbots de IA?
Este despliegue está ocurriendo en un momento crucial en Australia, ya que los niños menores de 16 años tendrán que tener cuentas de redes sociales en diciembre de este año.
Si bien algunos padres pueden creer que esto mantendrá a sus hijos a salvo de daños, los chatbots generativos de IA muestran los riesgos de la participación en línea se extienden mucho más allá de las redes sociales. Los niños, y los padres, deben educarse en cómo todo tipo de herramientas digitales se pueden usar de manera adecuada y segura.
Como el chatbot de IA de Gemini no es una herramienta de redes sociales, se quedará fuera de la prohibición de Australia.
Esto deja a los padres australianos jugando un juego de Whack-a-Mole con nuevas tecnologías mientras intentan mantener a sus hijos seguros. Los padres deben mantenerse al día con los nuevos desarrollos de herramientas y comprender los riesgos potenciales que enfrentan sus hijos. También deben comprender las limitaciones de la prohibición de las redes sociales para proteger a los niños de daños.
Esto resalta la urgente necesidad de revisar la legislación propuesta por el deber de cuidado de Australia. Mientras que la Unión Europea y el Reino Unido lanzaron la legislación de Derechos de Cuidado de Digital de Cuidado en 2023, Australia ha estado en espera desde noviembre de 2024. Esta legislación haría que las empresas tecnológicas tengan en cuenta legislando que se ocupan de contenido nocivo, en la fuente, para proteger a todos.
Proporcionado por la conversación
Este artículo se vuelve a publicar de la conversación bajo una licencia Creative Commons. Lea el artículo original.
Citación: Google está implementando su chatbot Gemini Ai para niños menores de 13 años. Es un movimiento arriesgado (2025, 11 de mayo) recuperado el 11 de mayo de 2025 de https://techxplore.com/news/2025-05-google-gemini-ai-chatbot-kids.html
Este documento está sujeto a derechos de autor. Además de cualquier trato justo con el propósito de estudio o investigación privada, no se puede reproducir ninguna parte sin el permiso por escrito. El contenido se proporciona solo para fines de información.
Noticias
Una implementación de codificación de acelerar la anotación de aprendizaje activo con Adala y Google Gemini
Published
17 horas agoon
11 mayo, 2025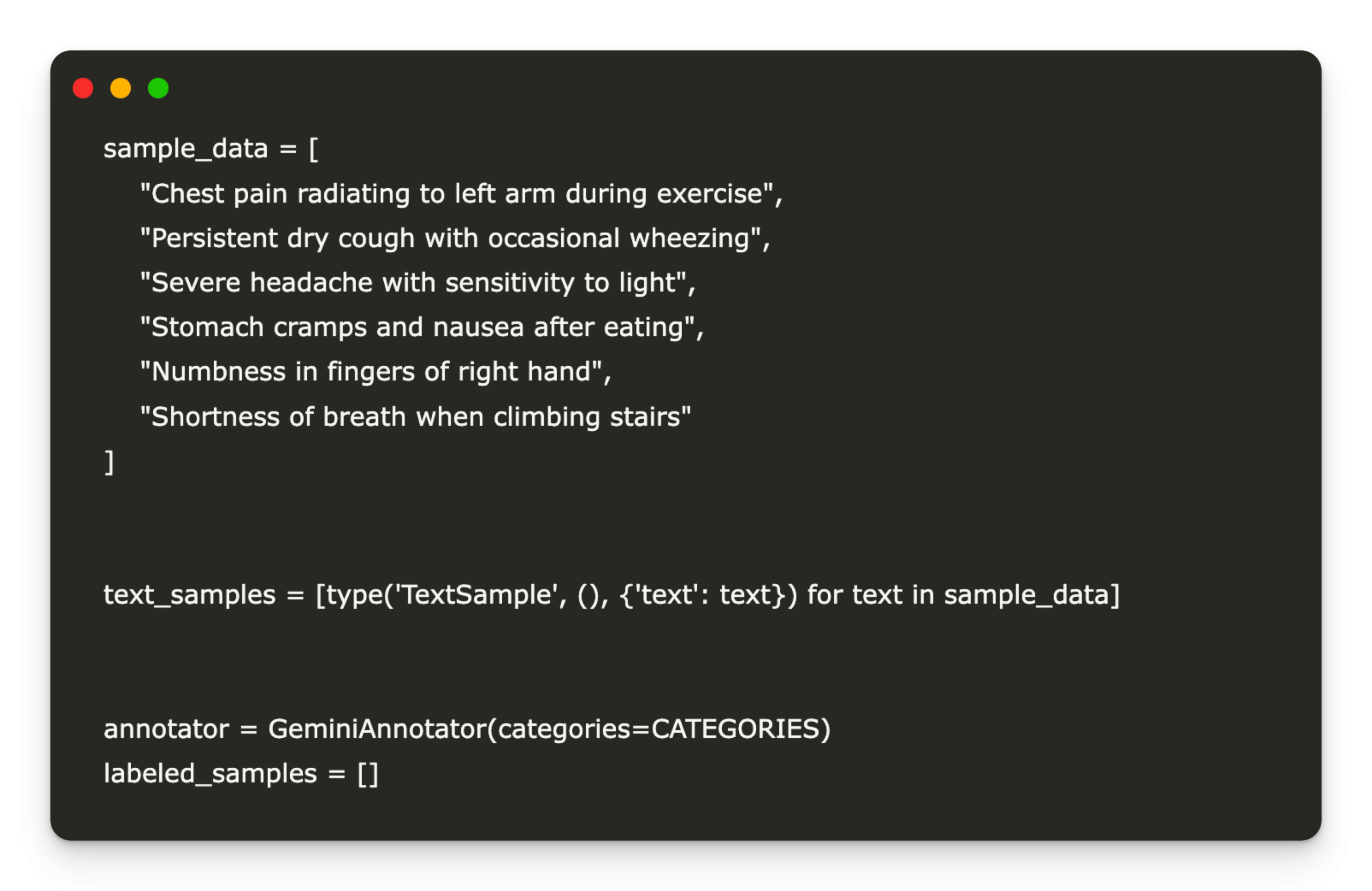
En este tutorial, aprenderemos cómo aprovechar el marco de Adala para construir una cartera de aprendizaje activo modular para la clasificación de síntomas médicos. Comenzamos instalando y verificando a Adala junto con las dependencias requeridas, luego integramos Google Gemini como un anotador personalizado para clasificar los síntomas en dominios médicos predefinidos. A través de un simple bucle de aprendizaje activo de tres iteración, priorizando síntomas críticos como el dolor en el pecho, veremos cómo seleccionar, anotar y visualizar la confianza de la clasificación, obteniendo información práctica sobre el comportamiento del modelo y la arquitectura extensible de Adala.
!pip install -q git+https://github.com/HumanSignal/Adala.git
!pip list | grep adala
Instalamos la última versión de Adala directamente desde su repositorio de GitHub. Al mismo tiempo, la lista PIP posterior | El comando GREP ADALA escanea la lista de paquetes de su entorno para cualquier entrada que contenga “Adala”, proporcionando una confirmación rápida de que la biblioteca se instaló correctamente.
import sys
import os
print("Python path:", sys.path)
print("Checking if adala is in installed packages...")
!find /usr/local -name "*adala*" -type d | grep -v "__pycache__"
!git clone https://github.com/HumanSignal/Adala.git
!ls -la Adala
Imprimimos sus rutas de búsqueda de módulos Python actuales y luego buscamos el directorio /usr /local para cualquier carpeta “adala” instalada (excluyendo __pycache__) para verificar que el paquete esté disponible. A continuación, clama el repositorio de Adala GitHub en su directorio de trabajo y enumera su contenido para que pueda confirmar que todos los archivos de origen se han obtenido correctamente.
import sys
sys.path.append('/content/Adala')
Al agregar la carpeta ADALA clonada al sys.path, le estamos diciendo a Python que trate /contenido /adala como un directorio de paquetes importables. Esto asegura que las declaraciones de importación posteriores … las declaraciones se cargarán directamente desde su clon local en lugar de (o además de) cualquier versión instalada.
!pip install -q google-generativeai pandas matplotlib
import google.generativeai as genai
import pandas as pd
import json
import re
import numpy as np
import matplotlib.pyplot as plt
from getpass import getpass
Instalamos el SDK de AI Generativo de Google junto con el análisis de datos y las bibliotecas de trazado (pandas y matplotlib), luego importar módulos clave, Genai para interactuar con Gemini, pandas para datos tabulares, JSON y RE para analizar, Numpy para operaciones numéricas, matlotlib.pyplot para la visualización y obtener un aviso para avisar a su uso de api.
try:
from Adala.adala.annotators.base import BaseAnnotator
from Adala.adala.strategies.random_strategy import RandomStrategy
from Adala.adala.utils.custom_types import TextSample, LabeledSample
print("Successfully imported Adala components")
except Exception as e:
print(f"Error importing: e")
print("Falling back to simplified implementation...")
Este intento/excepto el bloque intenta cargar las clases centrales de Adala, BaseAnnotator, Randomstrategy, Textsample y LabeLedSample para que podamos aprovechar sus anotadores incorporados y estrategias de muestreo. Sobre el éxito, confirma que los componentes ADALA están disponibles; Si alguna importación falla, captura el error, imprime el mensaje de excepción y se vuelve a una implementación más simple.
GEMINI_API_KEY = getpass("Enter your Gemini API Key: ")
genai.configure(api_key=GEMINI_API_KEY)
Le solicitamos de forma segura que ingrese su clave de la API Gemini sin hacerla eco de la cuaderno. Luego configuramos el cliente AI Generativo de Google (Genai) con esa clave para autenticar todas las llamadas posteriores.
CATEGORIES = ["Cardiovascular", "Respiratory", "Gastrointestinal", "Neurological"]
class GeminiAnnotator:
def __init__(self, model_name="models/gemini-2.0-flash-lite", categories=None):
self.model = genai.GenerativeModel(model_name=model_name,
generation_config="temperature": 0.1)
self.categories = categories
def annotate(self, samples):
results = []
for sample in samples:
prompt = f"""Classify this medical symptom into one of these categories:
', '.join(self.categories).
Return JSON format: "category": "selected_category",
"confidence": 0.XX, "explanation": "brief_reason"
SYMPTOM: sample.text"""
try:
response = self.model.generate_content(prompt).text
json_match = re.search(r'(\.*\)', response, re.DOTALL)
result = json.loads(json_match.group(1) if json_match else response)
labeled_sample = type('LabeledSample', (),
'text': sample.text,
'labels': result["category"],
'metadata':
"confidence": result["confidence"],
"explanation": result["explanation"]
)
except Exception as e:
labeled_sample = type('LabeledSample', (),
'text': sample.text,
'labels': "unknown",
'metadata': "error": str(e)
)
results.append(labeled_sample)
return results
Definimos una lista de categorías médicas e implementamos una clase GeminianNotator que envuelve el modelo generativo de Google Gemini para la clasificación de síntomas. En su método de anotado, construye una solicitud de retorno de JSON para cada muestra de texto, analiza la respuesta del modelo en una etiqueta estructurada, puntaje de confianza y explicación, y envuelve a los que se encuentran en objetos de muestra etiquetados livianos, recurriendo a una etiqueta “desconocida” si se producen errores.
sample_data = [
"Chest pain radiating to left arm during exercise",
"Persistent dry cough with occasional wheezing",
"Severe headache with sensitivity to light",
"Stomach cramps and nausea after eating",
"Numbness in fingers of right hand",
"Shortness of breath when climbing stairs"
]
text_samples = [type('TextSample', (), 'text': text) for text in sample_data]
annotator = GeminiAnnotator(categories=CATEGORIES)
labeled_samples = []
Definimos una lista de cadenas de síntomas crudos y envolvemos cada una en un objeto de muestra de texto ligero para pasarlas al anotador. Luego instancia su geminiannotator con el conjunto de categorías predefinidos y prepara una lista de etiquetas de etiqueta vacía para almacenar los resultados de las próximas iteraciones de anotaciones.
print("\nRunning Active Learning Loop:")
for i in range(3):
print(f"\n--- Iteration i+1 ---")
remaining = [s for s in text_samples if s not in [getattr(l, '_sample', l) for l in labeled_samples]]
if not remaining:
break
scores = np.zeros(len(remaining))
for j, sample in enumerate(remaining):
scores[j] = 0.1
if any(term in sample.text.lower() for term in ["chest", "heart", "pain"]):
scores[j] += 0.5
selected_idx = np.argmax(scores)
selected = [remaining[selected_idx]]
newly_labeled = annotator.annotate(selected)
for sample in newly_labeled:
sample._sample = selected[0]
labeled_samples.extend(newly_labeled)
latest = labeled_samples[-1]
print(f"Text: latest.text")
print(f"Category: latest.labels")
print(f"Confidence: latest.metadata.get('confidence', 0)")
print(f"Explanation: latest.metadata.get('explanation', '')[:100]...")
Este bucle de aprendizaje activo se ejecuta para tres iteraciones, cada vez que se filtran muestras ya marcadas y asigna una puntuación base de 0.1, impulsada por 0.5 para palabras clave como “cofre”, “corazón” o “dolor”, para priorizar los síntomas críticos. Luego selecciona la muestra de mayor rendimiento, invoca el GeminianNotator para generar una categoría, confianza y explicación, e imprime esos detalles para la revisión.
categories = [s.labels for s in labeled_samples]
confidence = [s.metadata.get("confidence", 0) for s in labeled_samples]
plt.figure(figsize=(10, 5))
plt.bar(range(len(categories)), confidence, color="skyblue")
plt.xticks(range(len(categories)), categories, rotation=45)
plt.title('Classification Confidence by Category')
plt.tight_layout()
plt.show()
Finalmente, extraemos las etiquetas de categoría predichas y sus puntajes de confianza y usamos matplotlib para trazar un gráfico de barras vertical, donde la altura de cada barra refleja la confianza del modelo en esa categoría. Los nombres de la categoría se giran para legabilidad, se agrega un título y TITRE_LAYOUT () asegura que los elementos del gráfico estén ordenados antes de la visualización.
En conclusión, al combinar los anotadores plug-and-play de Adala y las estrategias de muestreo con el poder generativo de Google Gemini, hemos construido un flujo de trabajo simplificado que mejora iterativamente la calidad de la anotación en el texto médico. Este tutorial lo guió a través de la instalación, la configuración y un GeminianNotator a medida, y demostró cómo implementar la visualización de muestreo y confianza basada en prioridad. Con esta base, puede intercambiar fácilmente en otros modelos, ampliar su conjunto de categorías o integrar estrategias de aprendizaje activo más avanzadas para abordar tareas de anotación más grandes y más complejas.
Verificar Notebook Colab aquí. Todo el crédito por esta investigación va a los investigadores de este proyecto. Además, siéntete libre de seguirnos Gorjeo Y no olvides unirte a nuestro 90k+ ml de subreddit.
Aquí hay una breve descripción de lo que estamos construyendo en MarkTechPost:
Asif Razzaq es el CEO de MarktechPost Media Inc .. Como empresario e ingeniero visionario, ASIF se compromete a aprovechar el potencial de la inteligencia artificial para el bien social. Su esfuerzo más reciente es el lanzamiento de una plataforma de medios de inteligencia artificial, MarktechPost, que se destaca por su cobertura profunda de noticias de aprendizaje automático y de aprendizaje profundo que es técnicamente sólido y fácilmente comprensible por una audiencia amplia. La plataforma cuenta con más de 2 millones de vistas mensuales, ilustrando su popularidad entre el público.
Related posts
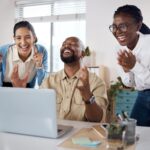
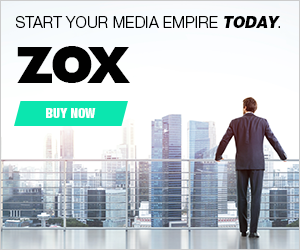
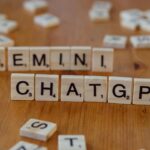
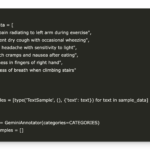
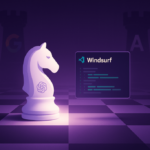
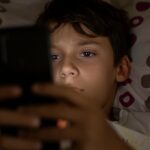
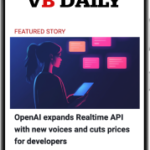
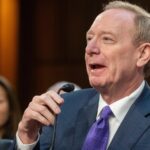
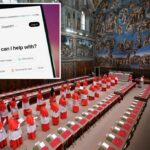
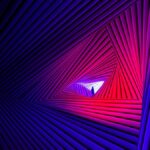
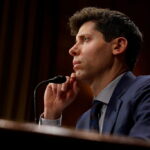
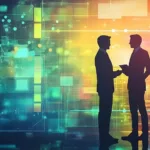
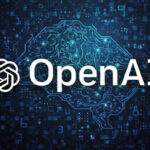
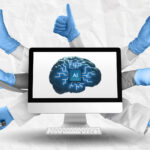
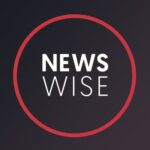
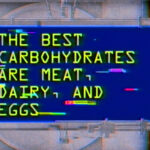
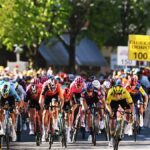
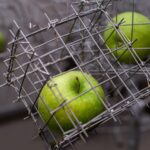
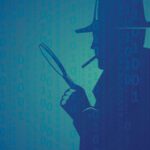
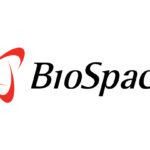
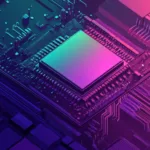
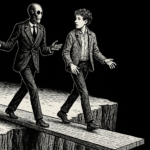
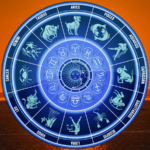
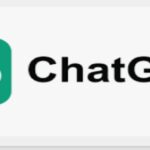
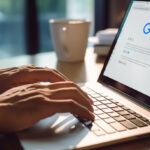
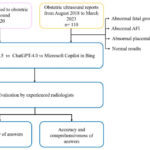
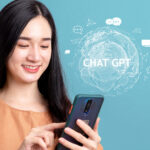
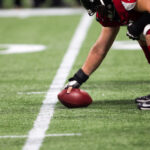
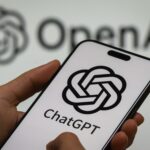
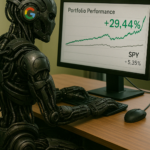
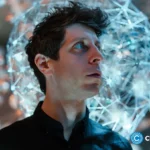
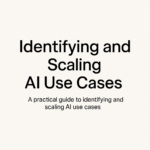
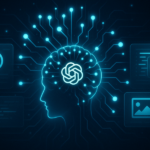
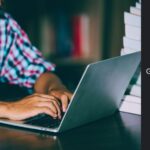
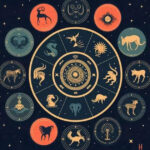
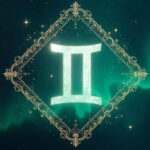
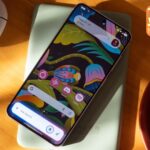
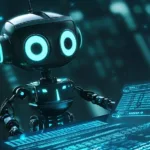
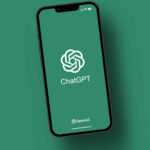
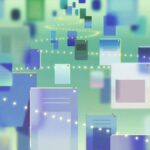
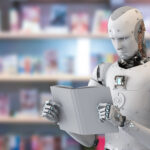
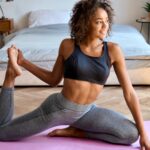
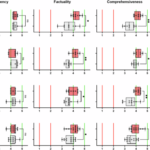
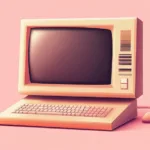
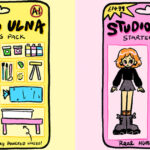
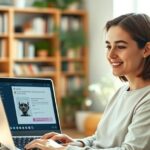
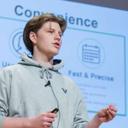
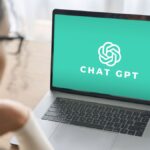
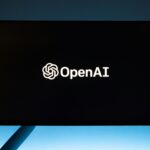
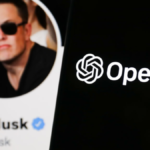
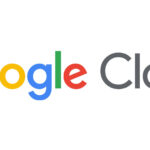
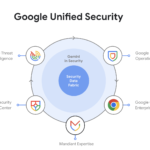
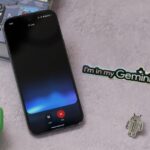
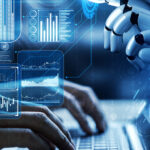
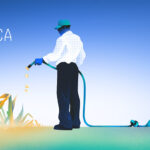
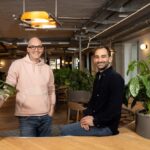
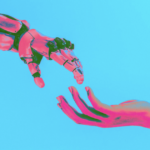
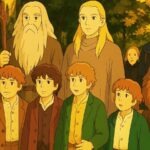
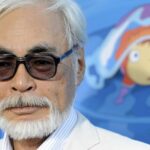
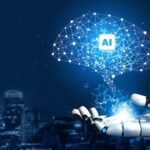
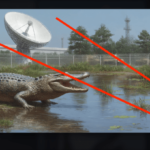
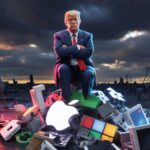
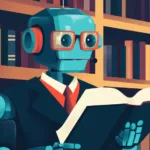
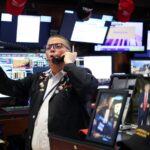
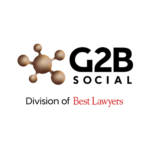
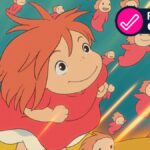
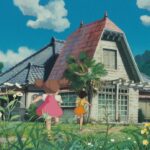
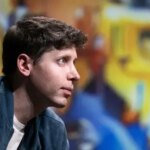
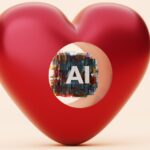
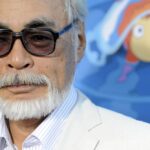
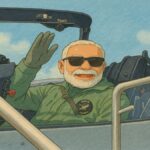
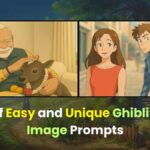
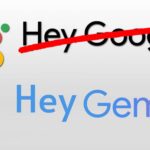
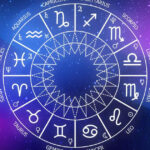
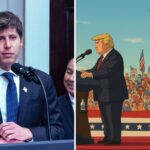
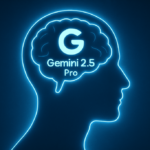
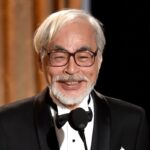
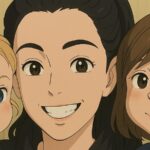
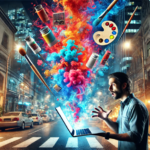
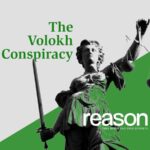
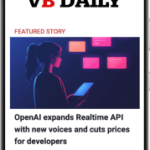
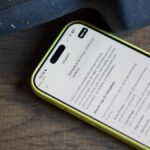
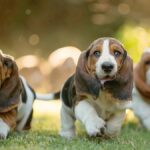
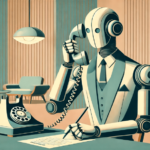
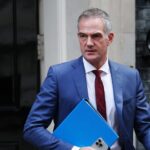
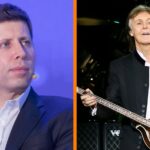
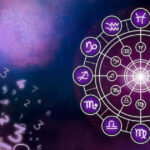
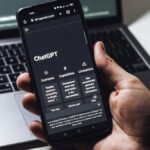
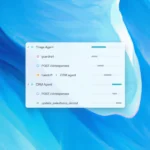
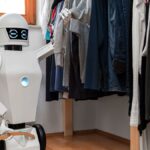
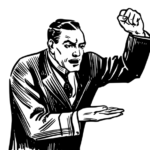
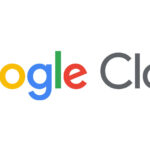
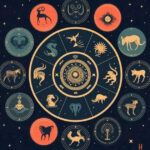
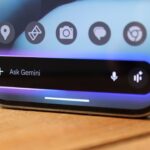
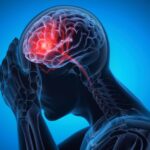
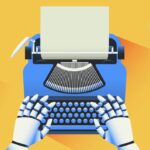
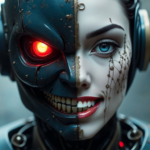
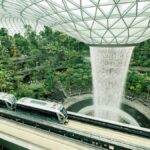
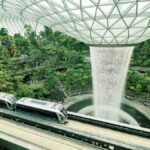
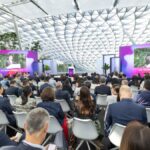
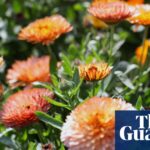

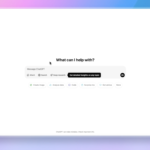
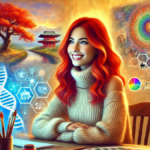
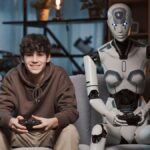
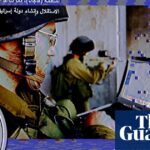
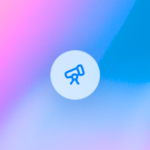
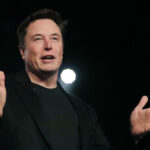
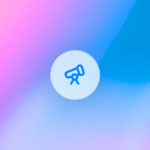
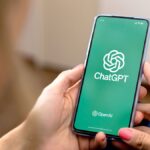
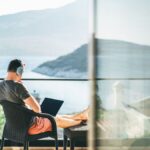
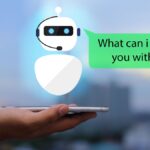
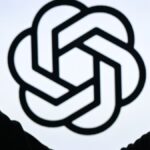
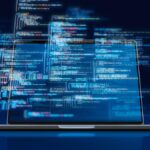
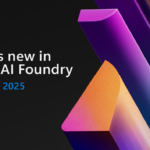
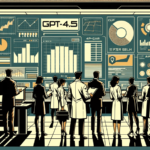
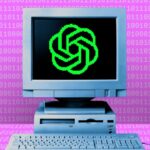
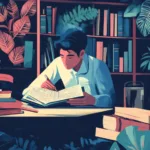
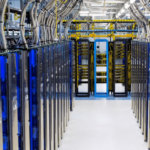
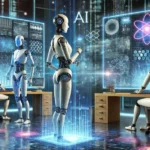
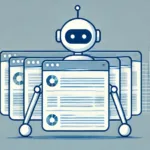
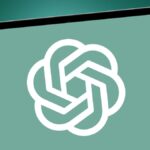
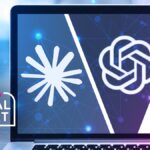
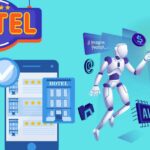
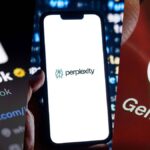
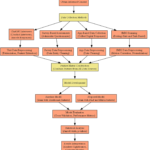
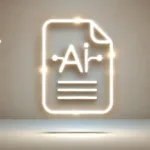
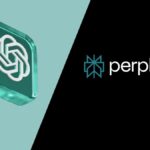
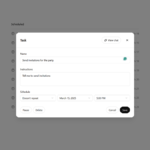
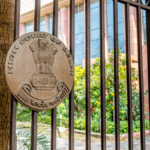
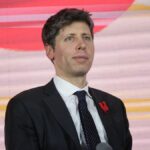
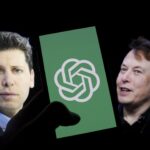
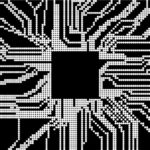
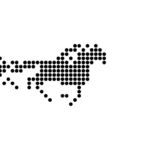
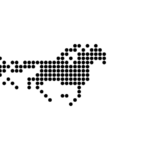
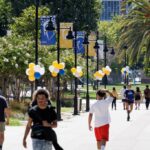
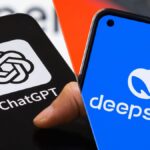
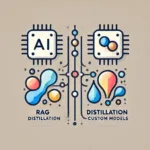
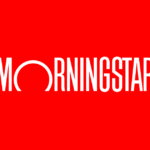
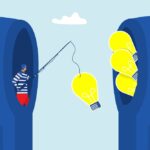
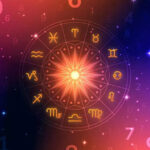
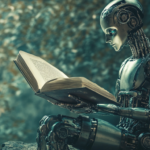
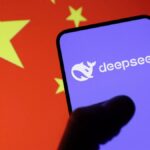
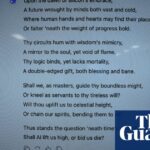
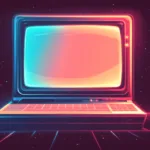

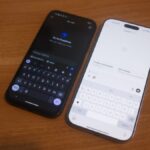
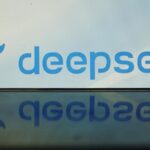

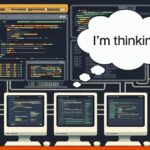
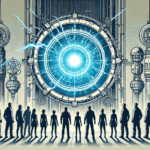
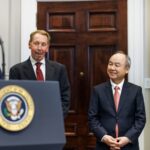
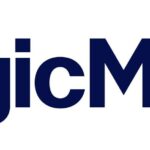
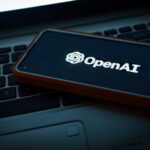

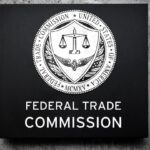
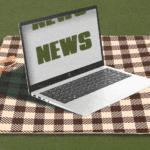
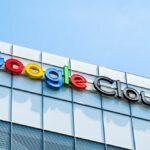
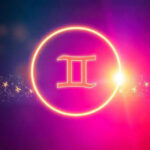
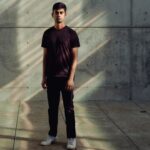
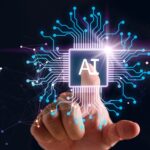
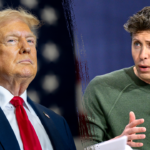
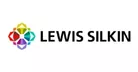
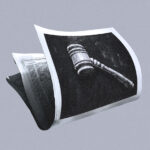
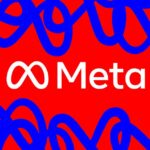

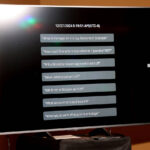

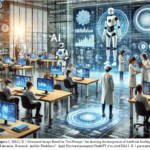
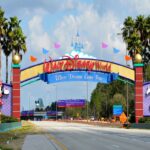
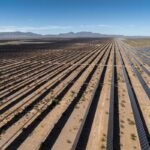
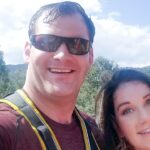
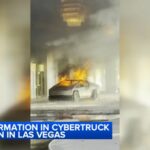
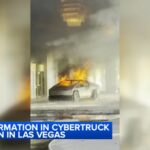
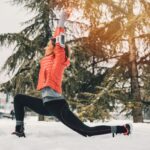
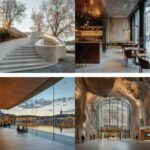
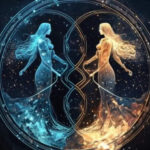
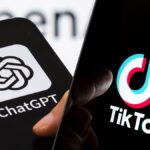
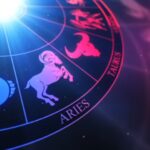
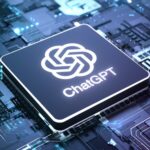
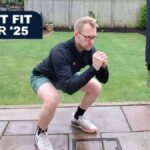
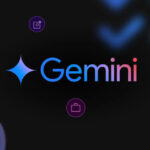
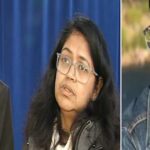
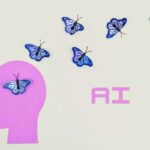
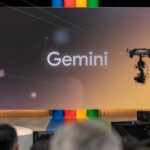
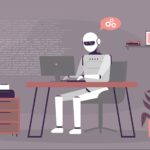
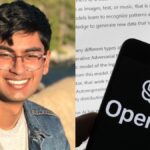
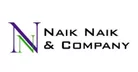

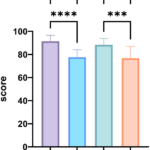
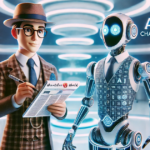
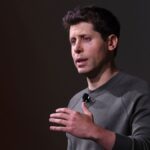
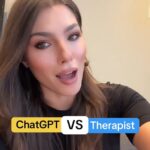
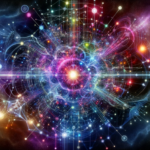
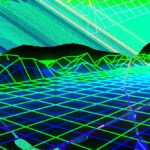
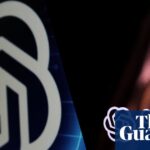
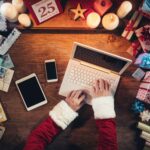
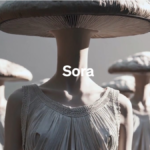
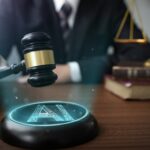
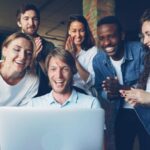
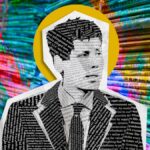

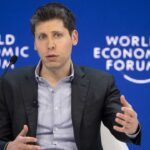
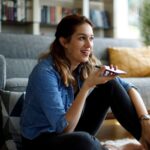
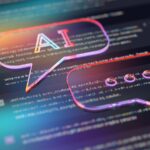
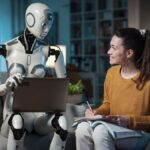
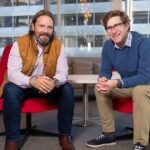
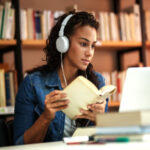
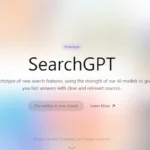
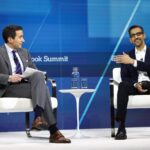
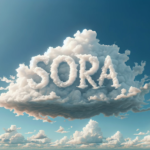
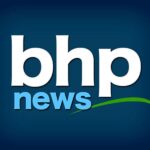
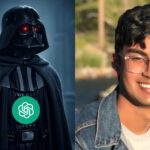
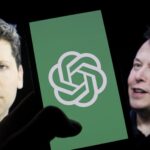
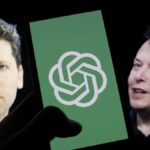
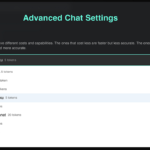
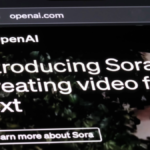
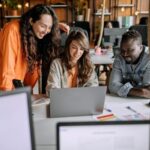
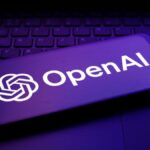
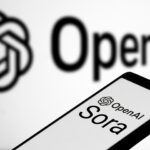
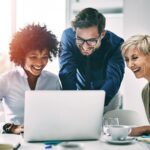
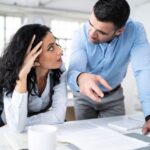
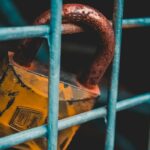
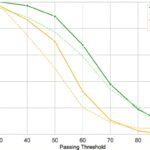
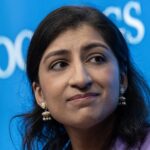
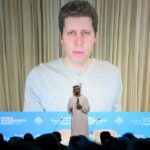
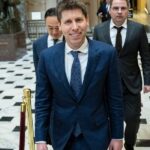
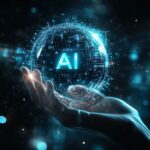
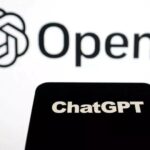
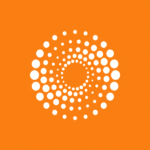
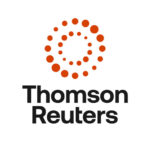
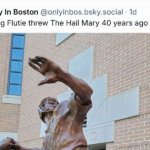

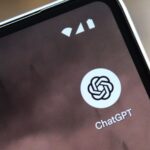
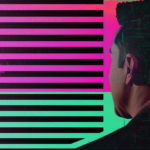
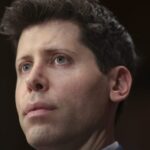
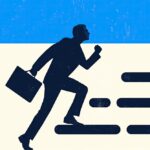
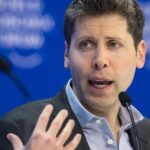
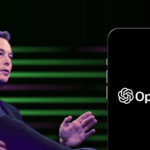
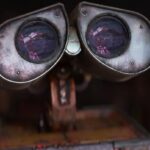
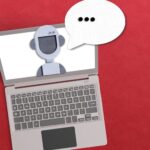
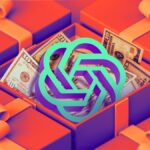
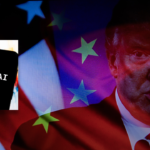
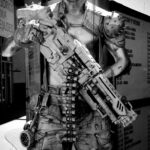
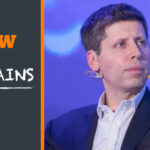
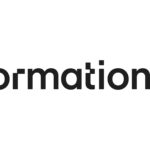
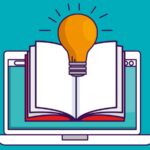
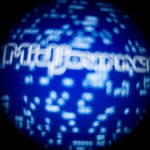
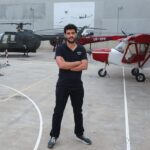
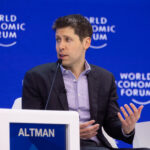
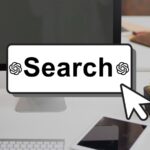
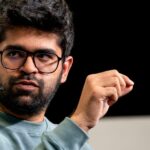
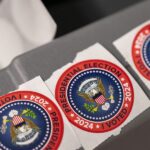
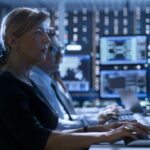
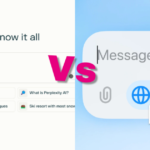
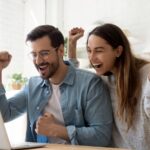
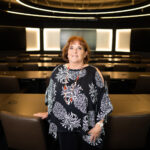
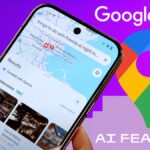
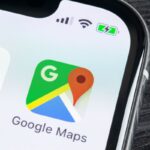
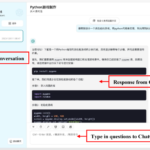
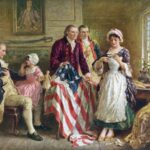
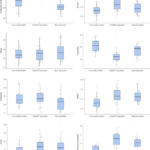

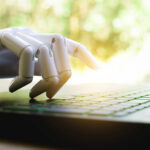
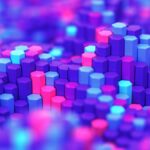
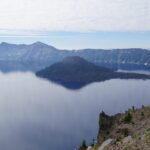
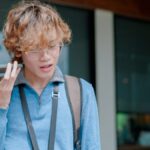
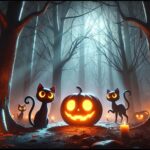
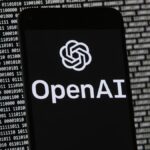
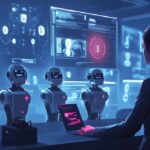
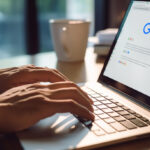
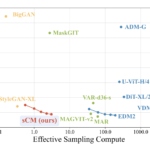
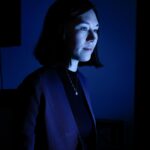
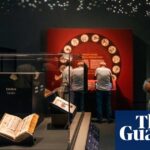
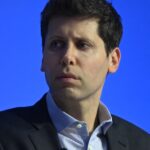
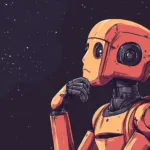
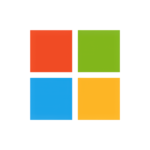
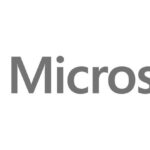
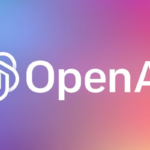
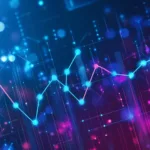
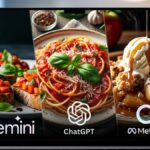
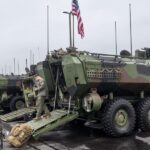

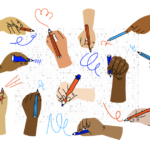
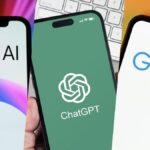
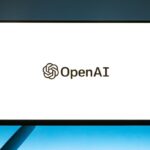
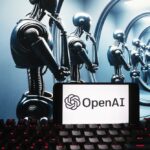
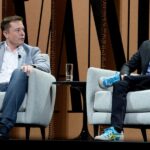
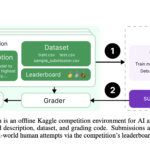
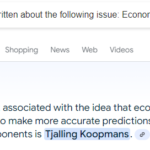
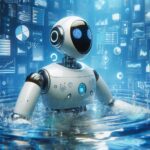
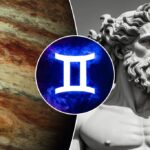
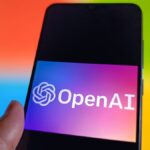
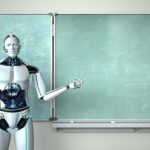
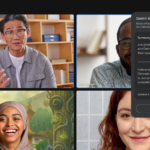
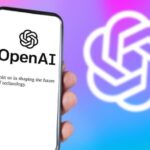
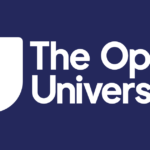
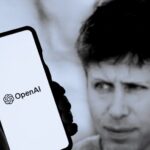
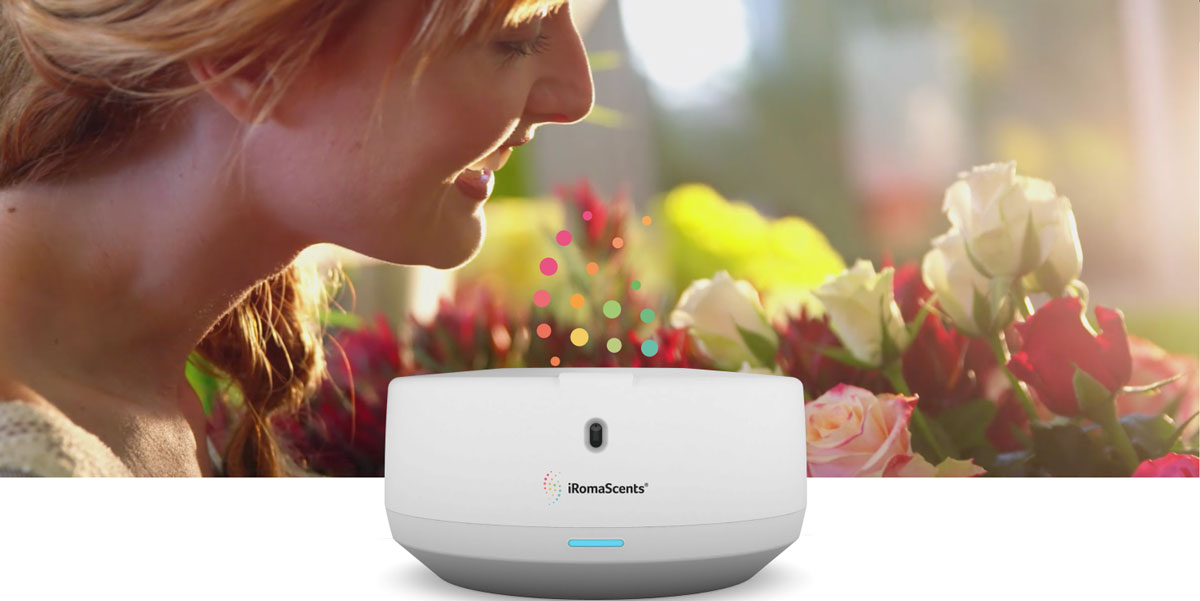

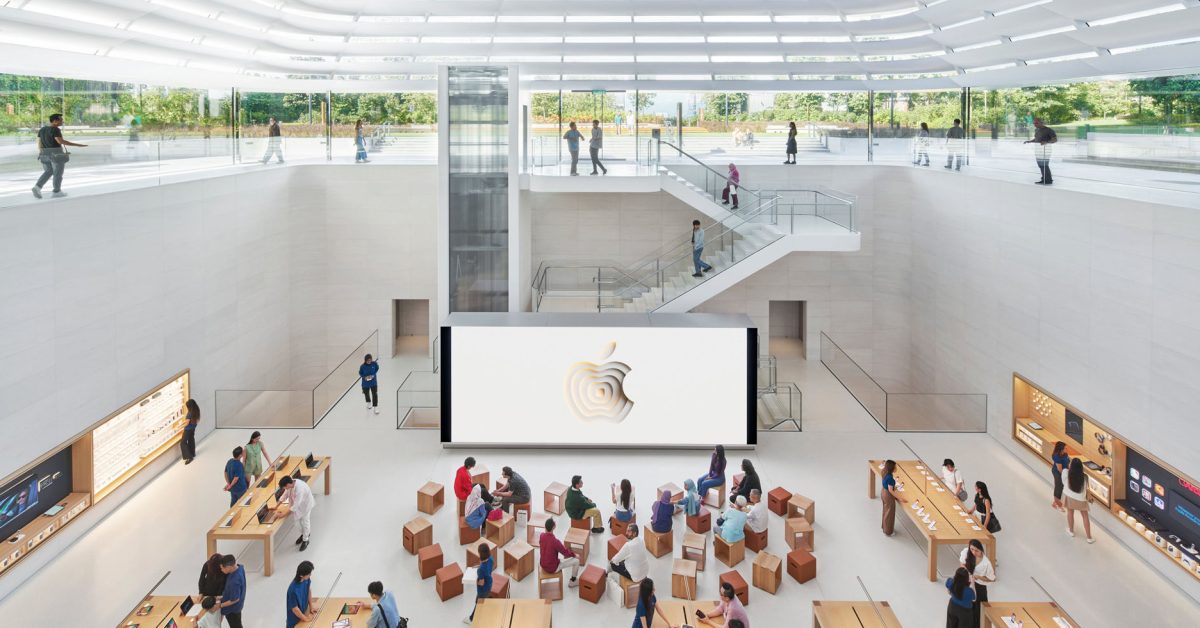


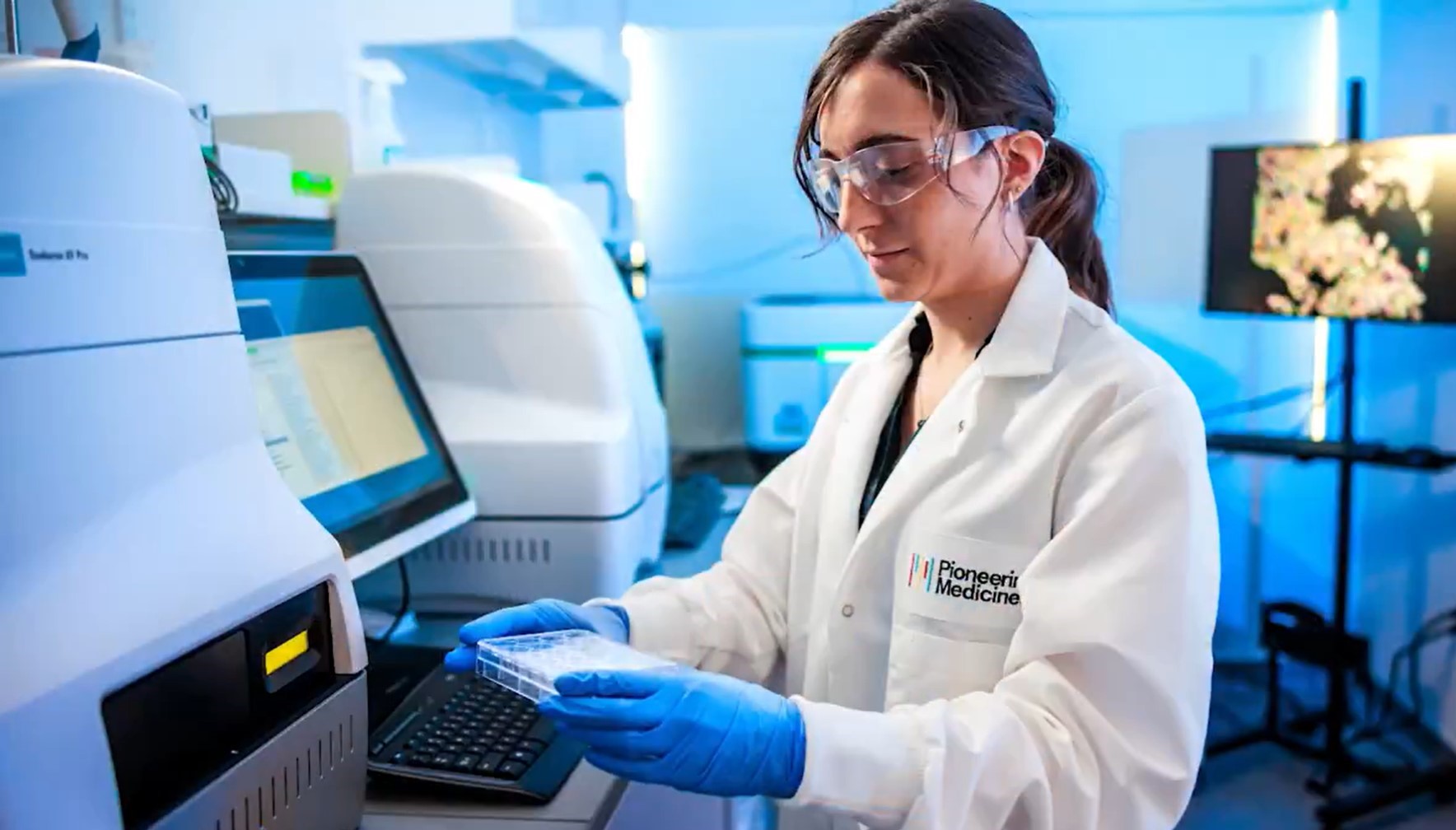
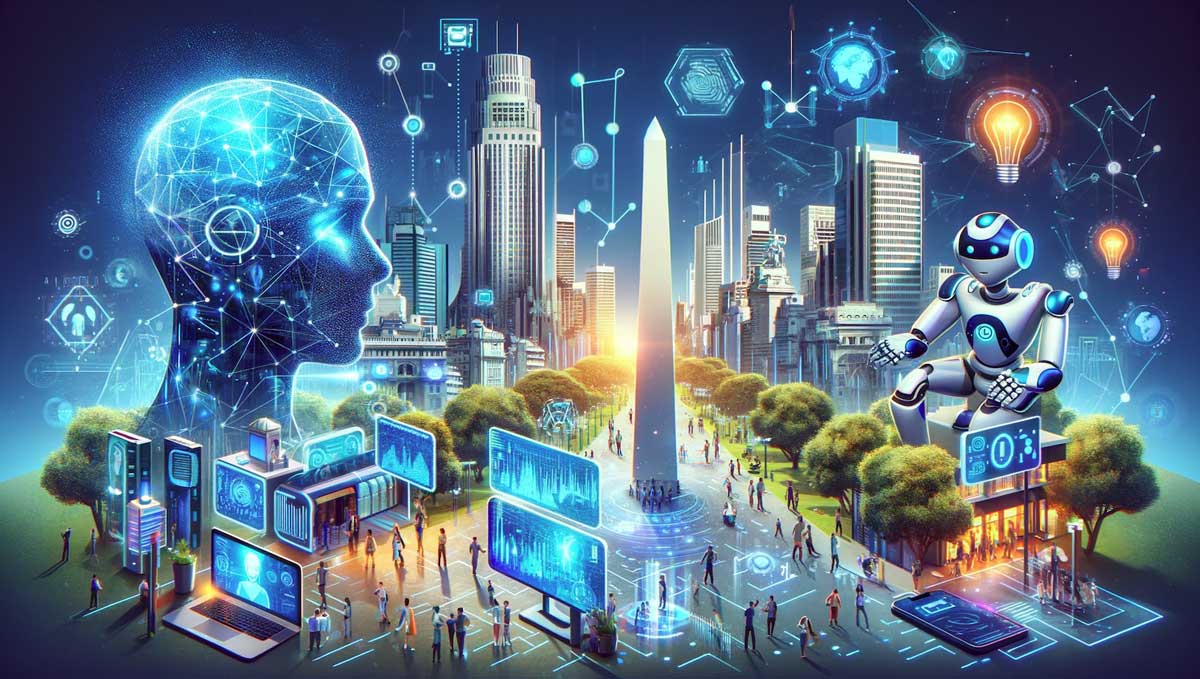

Trending
-
Startups12 meses ago
Remove.bg: La Revolución en la Edición de Imágenes que Debes Conocer
-
Tutoriales12 meses ago
Cómo Comenzar a Utilizar ChatGPT: Una Guía Completa para Principiantes
-
Recursos12 meses ago
Cómo Empezar con Popai.pro: Tu Espacio Personal de IA – Guía Completa, Instalación, Versiones y Precios
-
Startups10 meses ago
Startups de IA en EE.UU. que han recaudado más de $100M en 2024
-
Startups12 meses ago
Deepgram: Revolucionando el Reconocimiento de Voz con IA
-
Recursos12 meses ago
Perplexity aplicado al Marketing Digital y Estrategias SEO
-
Recursos12 meses ago
Suno.com: La Revolución en la Creación Musical con Inteligencia Artificial
-
Noticias10 meses ago
Dos periodistas octogenarios deman a ChatGPT por robar su trabajo