Noticias
Hot Trend Of Accessing Generative AI Via Simple Phone Call Gets Huge Uplift Via OpenAI’s New 1-800-ChatGPT

Using a conventional phone to access generative AI via voice-only just got a big boost via OpenAI’s … [+]
In today’s column, I discuss the hot trend of being able to access generative AI and large language models (LLMs) via a simple phone call. No smartphone is required — just use any mobile phone or even old-fashioned landline, and you immediately have unfettered voice access to a full-on generative AI capability.
Nice.
This trend has notably been given a huge boost due to OpenAI announcing their newly available 1-800-CHATGPT (1-800-242-8478). Yes, OpenAI ChatGPT, the 600-pound gorilla or elephant in the room, has grandly made phone usage of generative AI a widespread phenomenon that is going to spur other AI makers to do likewise. This is decidedly an earth-shattering upping of the ante in the fiercely contested AI one-upmanship taking place.
In the case of ChatGPT access, you are limited to up to 15 minutes of free phone-based usage per month. No registration is required. The AI simply notes the phone number that you are calling from to keep track of your allowed usage (must be a U.S.-based line for now). If you happen to have more than one phone, voila, you can essentially get more time per month by using up the 15 minutes permitted per separate phone line. For those outside the U.S. or who otherwise don’t want to make a phone call per se, OpenAI has also established a text-message-based approach to the same catchy phone number via the use of WhatsApp.
Before you get started partying, it turns out that there is more to being astute and safe about using generative AI over the phone than perhaps meets the eye. There are certainly celebratory upsides, but lots of disconcerting downsides too.
Let’s talk about it.
This analysis of an innovative AI breakthrough is part of my ongoing Forbes column coverage on the latest in AI including identifying and explaining various impactful AI complexities (see the link here). For my coverage of the top-of-the-line ChatGPT o1 model and its advanced functionality, see the link here and the link here.
How AI Works Via Phone Is Straightforward
Before we dive into the pluses and minuses, let’s make sure we are all on the same page about how accessing generative AI via a regular phone takes place.
The fundamentals are straightforward.
I’m assuming that you might have already used a generative AI capability via the web or possibly downloaded an app for use on your smartphone. If so, you know that once you have reached the generative AI, perhaps having to log in first, you then enter a prompt. Based on the prompt, the generative AI generates a response. All of this is typically done on a text-to-text basis, whereby you enter text as your prompt and get a text-based response from the AI.
You normally proceed with a back-and-forth of you entering a prompt and the AI provides a response. This happens on a turn-by-turn basis. Modern-day generative AI is amazingly fluent-like. You can easy-peasy carry on a compelling written conversation with the AI.
Voice Enters Into The Generative AI Realm
Some generative AI now allows a voice-to-text feature. This involves you speaking to the AI and your spoken words are converted into text. The text then gets fed into the AI. All else is roughly the same thereafter. In addition, some generative AI currently allows for a text-to-voice feature. That involves the AI producing text as a response, but rather than showing you the text, the system reads aloud the text to you.
Here’s the deal with regular phone-based access to generative AI.
You dial a phone number that automatically connects you to the designated generative AI.
The AI starts the conversation by doing a text-to-voice telling you a standardized opening. For example, when calling ChatGPT you generally get this introductory salutation:
- “Hi, I’m ChatGPT, an AI assistant. Our conversation may be reviewed for safety. By continuing this call, you agree to OpenAI’s terms and privacy policy. So, how can I help you?”
On any subsequent calls that you make to the ChatGPT phone line, the AI will detect that you previously called and will therefore start the opening by saying “Hello, again” and follow with the rest of the standard opening.
You then carry on a conversation with the AI, entirely via voice. Your voice utterances are converted into text, the AI processes the text as normally would be the case, and the AI generates a text response that is then said to you via a text-to-voice function.
Voila, this process continues until either you hang up or your time limit is reached.
Things That Can Go Wrong Right Away
In the real world, not everything is wine and roses.
Let’s see how that applies to generative AI being accessed via a regular phone.
First, some people are undoubtedly going to misdial and reach some number other than the designated one. Oopsie. The problem too is that this might occur on a massive scale. Currently, there are 300 million weekly active users of ChatGPT. How many of those users will opt to access ChatGPT via regular phone? In addition, how many new users who haven’t used ChatGPT will be attracted to using phone-based access?
We don’t know what the volume is going to be, but the odds are that it could be a rather large number. The point is that even if some small percentage misdials, there could be a slew of people calling other numbers inadvertently. On a tremendously beguiling scale. This could be an exasperating mess.
Second, and I deplore saying so, there is a distinct possibility that some evildoers will try to grab-up other phone numbers that are specifically similar to the designated phone number. Here’s their scam. They hope that people will misdial to their dastardly number. They then try to sell the person on swamp land or some other rip-off. People will be perhaps confused and assume that they have reached the correct number, a reputable number. Baddies will deceptively take advantage of them by asking for credit card numbers, social security numbers, and the like.
Sad face.
More Voice Issues To Be Thinking About
The next thing to consider is whether you are able to be adequately heard by the generative AI.
Imagine this. You tell the AI to find all the stores that are near the city named Pinole. Though voice-to-text translation is pretty good these days, there is a solid chance that your utterance will be misheard. The AI responds by telling you about the stores that are near the city named Binhole, a completely different city.
You and the AI go back and forth trying to iron this out. Meanwhile, your allotted time, let’s say 15 minutes, is rapidly dwindling. You decide this is fruitless and in anger vow to never call the number again.
Even if your commentary is readily interpretable, including an accent, there is the issue of potential background noise. Suppose you are standing on the streets of New York City and trying to get the generative AI to tell you which museum has your favorite painting. The background noise could be marring your perfect pronunciation.
Rinse and repeat.
Another frustration will be dropped lines. If you are in a rideshare car and the driver doesn’t speak your language, you might be tempted to call the generative AI and ask it to convert your destination instructions into that other language. You make the connection to the AI, which maybe can’t connect at first or at all due to a bad reception — and then start a conversation. Midway through, the line drops.
All in all, there might be a lot of angst involved.
Considerations Of A Private Nature
I’ve previously covered that many of the generative AI apps stipulate in their licensing agreements that whatever text you enter is fully accessible to the AI maker, see my analysis at the link here. The issue is this. Your text-based prompts under those stipulations can be examined by the AI researchers and AI developers of the AI maker. They can also reuse your entered data to further data train the AI. Bottom-line is that you are potentially getting yourself into a privacy intrusion and undercut any semblance of confidentiality.
Most users don’t know of this.
What about phone-based access to generative AI?
It is conceivable that an AI maker will decide to employ the same licensing requirements.
Realize that your utterances are being converted into text and that the transcribed text will be stored and made available to the AI maker. Keep this in mind. You’ll need to decide what kind of remarks or commentary you are willing to say to the generative AI phone-based capability. Also, it would be wise to find out beforehand what licensing stipulations the AI maker has established for their phone-based generative AI.
An added twist occurs with the voice aspects in the context of phone-based interaction.
When you enter text into a conventional generative AI online, the only communication being conveyed is the text. Period, end of story. In contrast, when you speak via phone, your voice is being captured too.
Your actual voice.
So what?
If the licensing allows the AI maker to exploit your voice, they could potentially use it to make a synthetic voice that sounds like you. Or, if not going quite that far, they might use your voice recording to further train the AI on how to interpret voice utterances. The gist is that your voice is possibly going to be used in ways that you might not have thought would occur.
Be wary and on your toes.
Making The Connection Of You To You
I’m guessing that some might be thinking that since you don’t need to register to use the generative AI for its phone-based functionality, you are essentially acting anonymously. Ergo, it doesn’t matter what you say, nor whether they record you or not. They just have some random person’s data that they have collected.
You can go on your merry way.
Well, maybe yes, maybe not.
The usual method of tracking you is going to be via the phone number you are using to make the call. That is something you are freely giving up. They could presumably try to pair the phone number with other databases. Doing so might enable the AI maker to figure out your name, address, age, and a wide variety of personal data.
The twofer is they can match what you’ve said to who you are.
Envision that you have called the AI several times to ask questions about sailboats. The next thing you know, the AI maker behind the scenes sells your name and phone number to a company that makes and sells sailboats. Of course, they can do this with just the phone number alone, not necessarily having to go through the trouble of matching your phone number to who you are. They simply sell your phone number and the fact that you have made inquiries about sailboats, the rest is up to the buyer for that information.
An interesting angle is that if an AI maker goes that route, they are likely to be embroiled in numerous federal, state, and local laws on such matters. The FTC is already pursuing various companies for AI-based scams, see my coverage at the link here. It would seem doubtful that the major generative AI vendors would go down that bumpy path. Presumably, hopefully, not.
The other issue is that once the use of generative AI via phone becomes a common practice, fly-by-nights could set up similar arrangements. Call this or that 800 number and get a full hour with interactive AI. Call now. Don’t wait.
How can they afford to do this?
They lean into the data in the manner I’ve described above.
Multimodal Is Not Likely Included For Now
A typical phone-based approach is going to assume that the user has a voice-only phone.
Thus, the AI cannot ask the user to take a picture of something. Without having a visual clue of what the user is discussing, the generative AI might have a limited ability to provide on-target responses.
For example, I am walking through a beautiful outdoor park and happen to notice a plant that looks potentially dangerous. I want to quickly find out if the plant is harmful. With smartphones, you can usually load an app or make an online connection that allows you to activate the camera on your phone. If the camera isn’t activated for the app, you can typically take a photo and send the picture to the app.
In the case of a typical phone-based generative AI, you have to be good enough at describing things to do what you need to do. For the plant, I might tell the AI that it is green in color, has leaves that are three-pronged, and appears to grow near the base of trees. Is that sufficient for the AI to figure out what plant it is? Probably a stretch.
The other side of this same coin is that the generative AI cannot display to the user a result in any pictorial way. Nor can the AI give the result in a text format. Why would someone want their result in text versus machine-produced voice? It could be that the person can’t adequately hear the AI, or maybe they hear it but want to write down what the AI has said. Having a text option would be handy, but again we are assuming that the user is calling on a conventional phone that lacks a multi-modal capacity.
Gradually, you can bet that most of the phone-based generative AI offerings will readily switch to multi-modal mode if a user is calling from a suitably equipped smartphone. The moment you make the connection to the AI, it will detect what your device is and what it can do. From then on, the AI will inform you of the ways to provide input and the means of producing outputs to the device that you are using.
We Live In Exciting Times
Gosh, some of you might be thinking, this discussion is all doom and gloom.
Isn’t there anything upbeat to say about this emerging means of using generative AI?
Yes, absolutely, there is lots to say.
The very exciting prospect is that people who have not yet experienced generative AI due to lack of an Internet connection or not having Wi-Fi will now be able to readily use generative AI. There might be millions upon millions of people who either can’t afford the equipment for such access or don’t live in a place where access is feasible.
The reach of a regular phone call is an incredible expansion of possibilities. I dare say that making phone calls is a lot simpler, easier, and readily possible. You don’t need to download anything to use the AI. You can use the AI pretty much anywhere and at any time, assuming you have access to a phone.
Some would assert that this is a vital step in the democratization of AI (read about the essentials at the link here). That’s a catchphrase that says we don’t want to end up in a situation of those that have AI and those that don’t have AI. The have-nots are presumably going to be at a disadvantage to the haves. Phone access ought to go a long way toward leveling the playing field concerning the access constraint.
All in all, you’ve got the widespread ubiquity of phones, the ease of using a regular phone, the relatively low cost of the phone and hopefully low cost for usage, and access to generative AI that only requires being able to speak. No typing skills are needed. No dealing with logins. Etc.
Just call and start using generative AI.
Boom, drop the mic.
Keep Our Wits About Us At All Times
I hope that the last bit of rah-rah gives you a sense of how important this new trend is.
And though I certainly don’t want to spoil the party, I ask that we all keep our heads and realize that in some sense we are also opening a Pandora’s box. How so? As I’ve repeatedly stated, people are using generative AI for all kinds of purposes, including mental health guidance. They simply access generative AI and start asking for therapy that would be seemingly akin to meeting with a human therapist, and most of the AI apps readily comply, see my analysis at the link here.
The good news is that phone-based access to generative AI implies that a bunch more people can now use AI for their mental health assistance. The bad news is roughly the same, namely, we are amid a massive scale experiment of people using everyday generative AI to give them mental health guidance. What if the AI isn’t doing this prudently? What if people avoid seeking human therapists since they assume AI is all they need?
The population-level consequences are potentially staggering and we upping the ante via phone-based generative AI access, see my predictions on what might arise at a population-level, at the link here.
A final contemplative thought for now.
In 1876, Alexander Graham Bell purportedly transmitted the first-ever recognizable speech message to his assistant Thomas A. Watson by saying, “Mr. Watson come here, I want you.” A stellar moment in history. Something never to be forgotten.
You might one day want to tell your kids that you were one of the first to use a phone-based generative AI. Something you’ll never forget. Think about your options, decide what seems appropriate to your needs and concerns, and make that call.
Get going and remember the date that you did so.
Noticias
O3 de OpenAI aún no es AGI, pero simplemente hizo algo que ninguna otra IA ha hecho
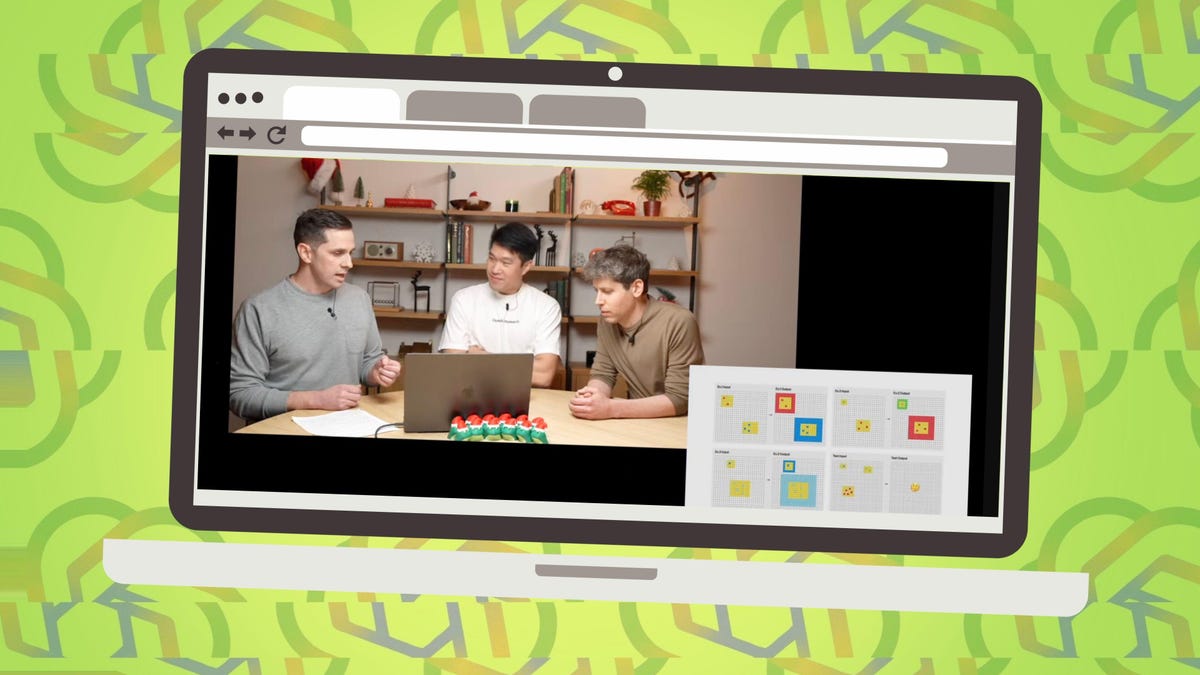
Sam Altman y los diputados de OpenAI analizan el rendimiento del nuevo modelo o3 en la prueba ARC-AGI.
OpenAI/ZDNET
El último modelo de lenguaje grande de OpenAI aún no está disponible, pero ya tenemos algunas formas de saber qué puede y qué no puede hacer.
El lanzamiento “o3” de OpenAI se dio a conocer el 20 de diciembre en forma de un video infomercial, lo que significa que la mayoría de las personas ajenas a la empresa no tienen idea de lo que realmente es capaz de hacer. (Se está dando acceso anticipado a los grupos externos de pruebas de seguridad).
Además: 15 formas en que la IA me ahorró tiempo en el trabajo en 2024
Aunque el video presentó mucha discusión sobre varios logros de referencia, el mensaje del cofundador y director ejecutivo de OpenAI, Sam Altman, en el video fue muy breve. Su afirmación más importante, y además vaga, fue que o3 “es un modelo increíblemente inteligente”.
ARC-AGI puso a prueba o3
OpenAI planea lanzar la versión “mini” de o3 a finales de enero y la versión completa algún tiempo después, dijo Altman.
Un outsider, sin embargo, ha tenido la oportunidad de poner a prueba a o3, en cierto sentido.
La prueba, en este caso, se llama “Corpus de Abstracción y Razonamiento para la Inteligencia General Artificial” o ARC-AGI. Es una colección de “desafíos para los sistemas inteligentes”, un nuevo punto de referencia. El ARC-AGI se anuncia como “el único punto de referencia diseñado específicamente para medir la adaptabilidad a la novedad”. Eso significa que está destinado a evaluar la adquisición de nuevas habilidades, no sólo el uso de conocimientos memorizados.
Además: ¿Por qué la ética se está convirtiendo en el mayor desafío de la IA?
Algunos consideran la AGI, inteligencia artificial general, como el Santo Grial: el logro de un nivel de inteligencia artificial que podría igualar o superar la inteligencia humana. La idea de ARC-AGI es guiar la IA hacia “sistemas artificiales más inteligentes y más parecidos a los humanos”.
El modelo o3 obtuvo una precisión del 76% en ARC-AGI en una evaluación coordinada formalmente por OpenAI y el autor de ARC-AGI, François Chollet, científico de la unidad de inteligencia artificial de Google.
Un cambio en las capacidades de la IA
En el sitio web de ARC-AGI, Chollet escribió la semana pasada que la puntuación del 76% es la primera vez que la IA supera la puntuación de un humano en el examen, como lo ejemplifican las respuestas de los trabajadores humanos de Mechanical Turk que tomaron la prueba y quienes, en promedio, obtuvo una puntuación ligeramente superior al 75% de aciertos.
Chollet escribió que la puntuación alta es “un aumento sorprendente e importante de la función escalonada en las capacidades de la IA, que muestra una capacidad novedosa de adaptación a tareas nunca antes vista en los modelos de la familia GPT”. Y añadió: “Toda la intuición sobre las capacidades de la IA deberá actualizarse para o3”.
El logro marca “un verdadero avance” y “un cambio cualitativo en las capacidades de la IA”, declaró Chollet. Chollet predice que la capacidad de o3 para “adaptarse a tareas que nunca antes había enfrentado” significa que “debe planificar que estas capacidades sean competitivas con el trabajo humano en un plazo bastante corto”.
Los comentarios de Chollet son dignos de mención porque nunca ha sido un defensor de la IA. En 2019, cuando creó ARC-AGI, me dijo en una entrevista que tuvimos para ZDNET que el flujo constante de “artículos de prensa grandilocuentes” de empresas de IA “sugieren engañosamente que la IA a nivel humano quizás esté a unos años de distancia”, mientras que consideraba tal hipérbole “una ilusión”.
Las preguntas ARC-AGI son fáciles de entender para las personas y bastante fáciles de resolver. Cada desafío muestra de tres a cinco ejemplos de la pregunta y la respuesta correcta, y luego al examinado se le presenta una pregunta similar y se le pide que proporcione la respuesta que falta.
La forma básica de ARC-AGI es tener de tres a cinco ejemplos de entrada y salida, que representan la pregunta y su respuesta, y luego un ejemplo final de entrada para el cual se debe proporcionar la respuesta proporcionando la imagen de salida correcta. Es bastante fácil para un humano descubrir qué imagen producir tocando píxeles de colores, incluso si no puede articular la regla per se.
Premio ARCP
Las preguntas no están basadas en texto sino en imágenes. Primero se muestra una cuadrícula de píxeles con formas coloreadas, seguida de una segunda versión que ha sido modificada de alguna manera. La pregunta es: ¿Cuál es la regla que cambia la imagen inicial en la segunda imagen?
En otras palabras, el desafío no depende directamente del lenguaje natural, el área célebre de los grandes modelos de lenguaje. En cambio, prueba la formulación de patrones abstractos en el dominio visual.
Pruebe ARC-AGI usted mismo
Puede probar ARC-AGI usted mismo en el sitio web del desafío de Chollet. Respondes al desafío “dibujando” en una cuadrícula vacía, completando cada píxel con el color correcto para crear la cuadrícula correcta de píxeles de colores como “respuesta”.
Es divertido, como jugar al Sudoku o al Tetris. Lo más probable es que, incluso si no puedes articular verbalmente cuál es la regla, descubrirás rápidamente qué cuadros deben colorearse para producir la solución. La parte que lleva más tiempo es tocar cada píxel de la cuadrícula para asignar su color.
Además: Por qué el avance cuántico de Google es “realmente notable” y qué sucederá después
Una respuesta correcta produce una animación de lanzamiento de confeti en la página web y el mensaje: “Has resuelto el rompecabezas diario del premio ARC. Aún eres más (generalmente) inteligente que la IA”.
Tenga en cuenta que cuando o3 o cualquier otro modelo realiza la prueba, no actúa directamente sobre los píxeles. En cambio, el equivalente se introduce en la máquina como una matriz de filas y columnas de números que deben transformarse en una matriz diferente como respuesta. Por lo tanto, los modelos de IA no “ven” la prueba de la misma manera que lo hace un humano.
Lo que aún no está claro
A pesar de los logros de o3, es difícil hacer declaraciones definitivas sobre las capacidades de o3. Debido a que el modelo de OpenAI es de código cerrado, todavía no está claro exactamente cómo el modelo resuelve el desafío.
Al no ser parte de OpenAI, Chollet tiene que especular sobre cómo o3 está haciendo lo que está haciendo.
Conjetura que el logro es el resultado de que OpenAI cambió la “arquitectura” de o3 con respecto a la de sus predecesores. Una arquitectura en IA se refiere a la disposición y relación de los elementos funcionales que dan estructura al código.
Además: si ChatGPT produce código generado por IA para tu aplicación, ¿a quién pertenece realmente?
Chollet especula en el blog que “en el momento de la prueba, el modelo busca en el espacio de posibles Cadenas de Pensamiento (CoT) que describen los pasos necesarios para resolver la tarea, de una manera tal vez no muy diferente a la búsqueda en árbol de Monte Carlo al estilo AlphaZero”.
El término cadena de pensamiento se refiere a un enfoque cada vez más popular en la IA generativa en el que el modelo de IA puede detallar la secuencia de cálculos que realiza en busca de la respuesta final. AlphaZero es el famoso programa de inteligencia artificial de la unidad DeepMind de Google que venció a los humanos en el ajedrez en 2016. Una búsqueda de árboles de Monte Carlo es un enfoque informático que existe desde hace décadas.
En un intercambio de correo electrónico, Chollet me contó un poco más sobre su pensamiento. Le pregunté cómo llegó a esa idea de una búsqueda de cadenas de pensamiento. “Claramente, cuando el modelo está ‘pensando’ durante horas y generando millones de tokens en el proceso de resolver un único rompecabezas, debe estar haciendo algún tipo de búsqueda”, respondió Chollet.
Chollet añadió:
Es completamente obvio por las características de latencia/coste del modelo que está haciendo algo completamente diferente a la serie GPT. No es la misma arquitectura, ni nada remotamente parecido. El factor definitorio del nuevo sistema es una gran cantidad de búsquedas en el momento de la prueba. Anteriormente, 4 años de ampliación de la misma arquitectura (la serie GPT) no habían producido ningún progreso en ARC, y ahora este sistema, que claramente tiene una nueva arquitectura, está creando un cambio funcional gradual en las capacidades, por lo que la arquitectura lo es todo.
Hay una serie de advertencias aquí. OpenAI no reveló cuánto dinero se gastó en una de sus versiones de o3 para resolver ARC-AGI. Esa es una omisión significativa porque un criterio de ARC-AGI es el costo en dólares reales de usar chips GPU como proxy de la “eficiencia” del modelo de IA.
Chollet me dijo en un correo electrónico que el enfoque de o3 no equivale a un enfoque de “fuerza bruta”, pero bromeó: “Por supuesto, también se podría definir la fuerza bruta como ‘lanzar una cantidad excesiva de cómputo a un problema simple’. ‘ en cuyo caso se podría decir que es fuerza bruta”.
Además, Chollet señala que o3 fue entrenado para realizar la prueba ARC-AGI utilizando el conjunto de datos de entrenamiento de la competencia. Eso significa que aún no está claro cómo abordaría el examen una versión limpia de o3, sin preparación para el examen.
También: El generador de vídeo Sora AI de OpenAI ya está aquí: cómo probarlo
Chollet me dijo en un correo electrónico: “Será interesante ver qué puntuación obtiene el sistema base sin información relacionada con ARC, pero en cualquier caso, el hecho de que el sistema esté ajustado para ARC a través del conjunto de entrenamiento no invalida su rendimiento”. “Para eso está el conjunto de entrenamiento. Hasta ahora nadie había podido lograr puntuaciones similares, incluso después de entrenar en millones de tareas ARC generadas”.
o3 todavía falla en algunas tareas fáciles
A pesar de la incertidumbre, una cosa parece muy clara: quienes anhelan AGI se sentirán decepcionados. Chollet enfatiza que la prueba ARC-AGI es “una herramienta de investigación” y que “aprobar ARC-AGI no equivale a alcanzar AGI”.
“De hecho, no creo que o3 sea AGI todavía”, escribe Chollet en el blog ARC-AGI. “O3 todavía falla en algunas tareas muy fáciles, lo que indica diferencias fundamentales con la inteligencia humana”.
Para demostrar que todavía no estamos al nivel de inteligencia humana, Chollet señala algunos de los problemas simples en ARC-AGI que o3 no puede resolver. Uno de esos problemas implica simplemente mover un cuadrado de color en una cantidad determinada, un patrón que rápidamente se vuelve claro para un ser humano.
Un problema de ejemplo de ARC-AGI donde falló el modelo o3.
Premio ARCP
Chollet planea presentar una nueva versión de ARC-AGI en enero. Predice que reducirá drásticamente los resultados de o3. “Sabrás que AGI está aquí cuando el ejercicio de crear tareas que sean fáciles para los humanos comunes pero difíciles para la IA se vuelva simplemente imposible”, concluye.
Noticias
Prueba de manejo del modelo Gemini-Exp-1206 de Google en análisis de datos y visualizaciones
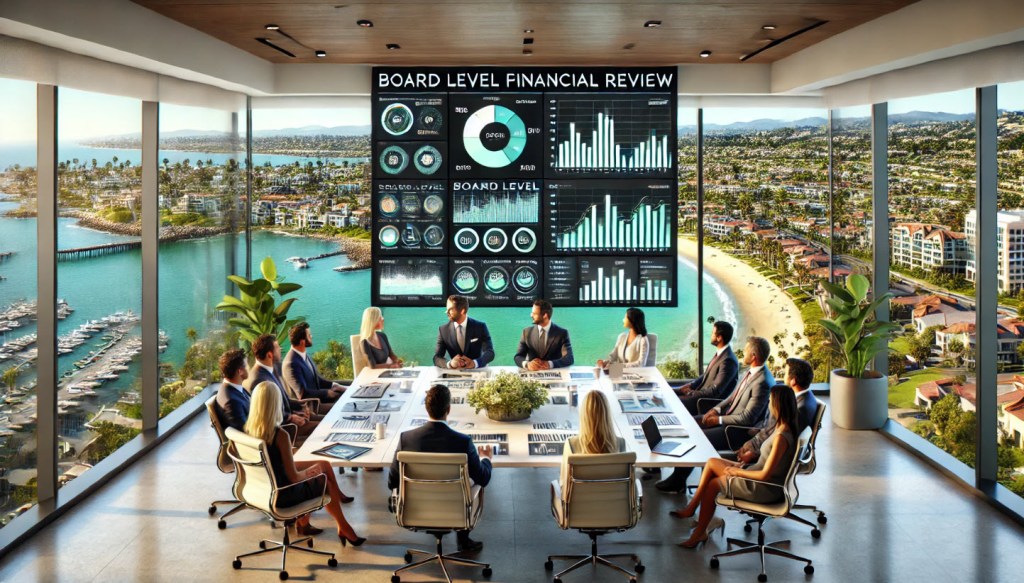
Únase a nuestros boletines diarios y semanales para obtener las últimas actualizaciones y contenido exclusivo sobre la cobertura de IA líder en la industria. Más información
Uno de los últimos modelos experimentales de Google, Gemini-Exp-1206, muestra el potencial de aliviar uno de los aspectos más agotadores del trabajo de cualquier analista: lograr que sus datos y visualizaciones se sincronicen perfectamente y proporcionen una narrativa convincente, sin tener que trabajar toda la noche. .
Los analistas de inversiones, los banqueros junior y los miembros de equipos de consultoría que aspiran a puestos de socios asumen sus roles sabiendo que largas horas de trabajo, fines de semana y pasar toda la noche ocasionalmente podrían darles una ventaja interna en un ascenso.
Lo que consume gran parte de su tiempo es realizar análisis de datos avanzados y al mismo tiempo crear visualizaciones que refuercen una historia convincente. Lo que hace que esto sea más desafiante es que cada firma bancaria, fintech y consultora, como JP Morgan, McKinsey y PwC, tiene formatos y convenciones únicos para el análisis y visualización de datos.
VentureBeat entrevistó a miembros de equipos de proyectos internos cuyos empleadores habían contratado a estas empresas y las habían asignado al proyecto. Los empleados que trabajan en equipos dirigidos por consultores dijeron que producir imágenes que condensen y consoliden la enorme cantidad de datos es un desafío persistente. Uno dijo que era común que los equipos de consultores trabajaran durante la noche y hicieran un mínimo de tres o cuatro iteraciones de las visualizaciones de una presentación antes de decidirse por una y prepararla para las actualizaciones a nivel de tablero.
Un caso de uso convincente para probar el último modelo de Google
El proceso en el que confían los analistas para crear presentaciones que respalden una historia con visualizaciones y gráficos sólidos tiene tantos pasos manuales y repeticiones que resultó ser un caso de uso convincente para probar el último modelo de Google.
Al lanzar el modelo a principios de diciembre, Patrick Kane de Google escribió: “Ya sea que esté enfrentando desafíos complejos de codificación, resolviendo problemas matemáticos para proyectos escolares o personales, o brindando instrucciones detalladas de varios pasos para elaborar un plan de negocios personalizado, Gemini-Exp-1206 le ayudará a navegar tareas complejas con mayor facilidad”. Google notó el rendimiento mejorado del modelo en tareas más complejas, incluido el razonamiento matemático, la codificación y el seguimiento de una serie de instrucciones.
VentureBeat llevó el modelo Exp-1206 de Google a una prueba exhaustiva esta semana. Creamos y probamos más de 50 scripts de Python en un intento de automatizar e integrar análisis y visualizaciones intuitivas y fáciles de entender que pudieran simplificar los datos complejos que se analizan. Dado que los hiperescaladores dominan los ciclos de noticias actuales, nuestro objetivo específico era crear un análisis de un mercado tecnológico determinado y al mismo tiempo crear tablas de apoyo y gráficos avanzados.
A través de más de 50 iteraciones diferentes de scripts de Python verificados, nuestros hallazgos incluyeron:
- Cuanto mayor es la complejidad de una solicitud de código Python, más “piensa” el modelo e intenta anticipar el resultado deseado. Exp-1206 intenta anticipar lo que se necesita a partir de un mensaje complejo determinado y variará lo que produce incluso con el más mínimo cambio de matiz en un mensaje. Vimos esto en cómo el modelo alternaría entre formatos de tipos de tablas colocadas directamente encima del gráfico de araña del análisis de mercado de hiperescalador que creamos para la prueba.
- Obligar al modelo a intentar realizar análisis y visualización de datos complejos y producir un archivo Excel genera una hoja de cálculo con varias pestañas. Sin que nunca le pidieran una hoja de cálculo de Excel con varias pestañas, Exp-1206 creó una. El análisis tabular principal solicitado estaba en una pestaña, las visualizaciones en otra y una tabla auxiliar en la tercera.
- Decirle al modelo que repita los datos y recomiende las 10 visualizaciones que decida que mejor se ajustan a los datos ofrece resultados beneficiosos y reveladores. Con el objetivo de reducir el tiempo que supone tener que crear tres o cuatro iteraciones de presentaciones de diapositivas antes de una revisión por parte de la junta, obligamos al modelo a producir múltiples iteraciones conceptuales de imágenes. Estos podrían limpiarse e integrarse fácilmente en una presentación, ahorrando muchas horas de trabajo manual creando diagramas en diapositivas.
Impulsando a Exp-1206 hacia tareas complejas y en capas
El objetivo de VentureBeat era ver hasta dónde se podía llevar el modelo en términos de complejidad y tareas en capas. Su desempeño en la creación, ejecución, edición y ajuste de 50 scripts de Python diferentes mostró cuán rápido el modelo intenta captar matices en el código y reaccionar de inmediato. El modelo se flexiona y se adapta según el historial de indicaciones.
El resultado de ejecutar el código Python creado con Exp-1206 en Google Colab mostró que la granularidad matizada se extendía al sombreado y la translucidez de las capas en un gráfico de araña de ocho puntos que fue diseñado para mostrar cómo se comparan seis competidores hiperescaladores. Los ocho atributos que le pedimos a Exp-1206 que identificara en todos los hiperescaladores y que anclara el gráfico de araña se mantuvieron consistentes, mientras que las representaciones gráficas variaron.
Batalla de los hiperescaladores
Elegimos los siguientes hiperescaladores para comparar en nuestra prueba: Alibaba Cloud, Amazon Web Services (AWS), Digital Realty, Equinix, Google Cloud Platform (GCP), Huawei, IBM Cloud, Meta Platforms (Facebook), Microsoft Azure, NTT Global Data. Centros, Oracle Cloud y Tencent Cloud.
A continuación, escribimos un mensaje de 11 pasos de más de 450 palabras. El objetivo era ver qué tan bien Exp-1206 puede manejar la lógica secuencial y no perder su lugar en un proceso complejo de varios pasos. (Puede leer el mensaje en el apéndice al final de este artículo).
Luego enviamos el mensaje en Google AI Studio, seleccionando el modelo Gemini Experimental 1206, como se muestra en la siguiente figura.
A continuación, copiamos el código en Google Colab y lo guardamos en un cuaderno Jupyter (Comparación de Hyperscaler – Gemini Experimental 1206.ipynb), luego ejecutamos el script de Python. El script se ejecutó sin problemas y creó tres archivos (indicados con las flechas rojas en la parte superior izquierda).
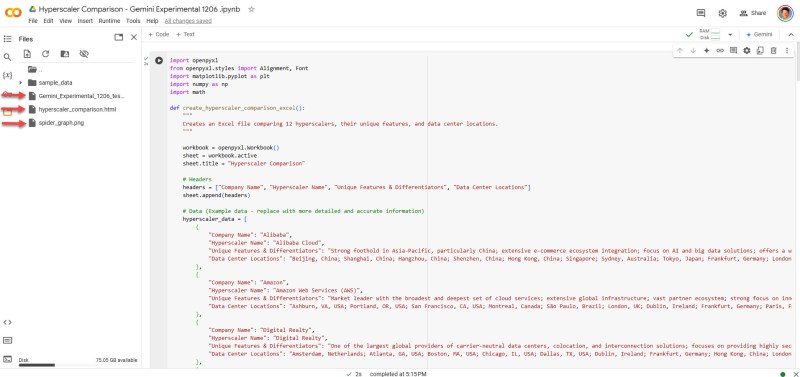
Análisis comparativo de Hyperscaler y un gráfico, en menos de un minuto
La primera serie de instrucciones en el mensaje pedía a Exp-1206 que creara un script de Python que comparara 12 hiperescaladores diferentes por su nombre de producto, características y diferenciadores únicos y ubicaciones de centros de datos. A continuación se muestra cómo resultó el archivo de Excel que se solicitó en el script. Me llevó menos de un minuto formatear la hoja de cálculo para reducirla y ajustarla a las columnas.
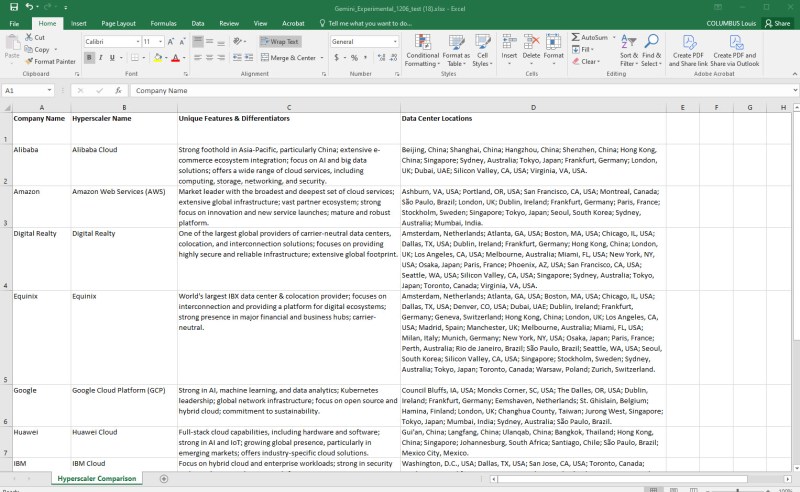
La siguiente serie de comandos solicitó una tabla de los seis principales hiperescaladores comparados en la parte superior de una página y el gráfico de araña a continuación. Exp-1206 eligió por sí solo representar los datos en formato HTML, creando la siguiente página.
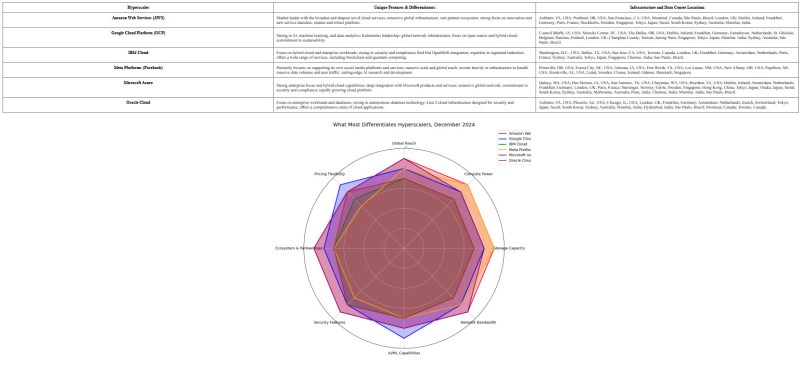
La secuencia final de comandos se centró en la creación de un gráfico de araña para comparar los seis hiperescaladores principales. Le asignamos a Exp-1206 la tarea de seleccionar los ocho criterios para la comparación y completar el gráfico. Esa serie de comandos se tradujo a Python y el modelo creó el archivo y lo proporcionó en la sesión de Google Colab.
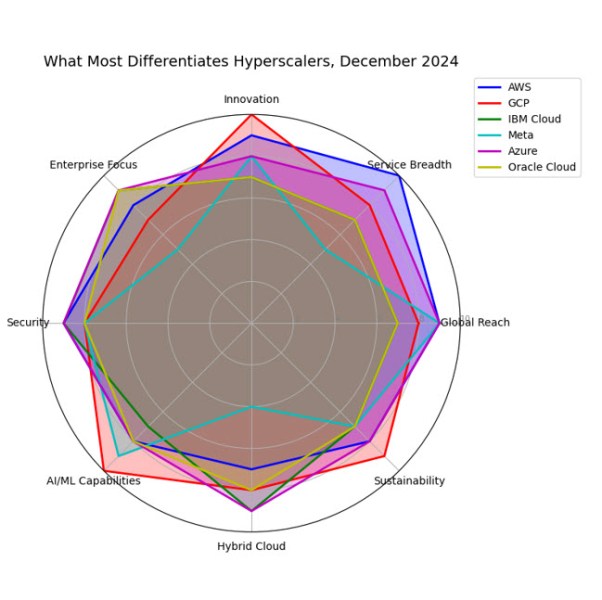
Un modelo diseñado específicamente para ahorrar tiempo a los analistas
VentureBeat ha aprendido que en su trabajo diario, los analistas continúan creando, compartiendo y ajustando bibliotecas de indicaciones para modelos de IA específicos con el objetivo de optimizar los informes, el análisis y la visualización en todos sus equipos.
Los equipos asignados a proyectos de consultoría a gran escala deben considerar cómo modelos como Gemini-Exp-1206 pueden mejorar enormemente la productividad y aliviar la necesidad de semanas laborales de más de 60 horas y noches ocasionales en vela. Una serie de indicaciones automatizadas pueden realizar el trabajo exploratorio de observar las relaciones en los datos, lo que permite a los analistas producir imágenes con mucha mayor certeza sin tener que dedicar una cantidad excesiva de tiempo a llegar allí.
Apéndice:
Prueba rápida de Google Gemini Experimental 1206
Escriba un script de Python para analizar los siguientes hiperescaladores que han anunciado una presencia de centro de datos e infraestructura global para sus plataformas y cree una tabla comparándolos que capture las diferencias significativas en cada enfoque en presencia de centro de datos e infraestructura global.
Haga que la primera columna de la tabla sea el nombre de la empresa, la segunda columna sean los nombres de cada uno de los hiperescaladores de la empresa que tienen presencia de centro de datos e infraestructura global, la tercera columna sea lo que hace que sus hiperescaladores sean únicos y una inmersión profunda en los más diferenciados. características, y la cuarta columna son las ubicaciones de los centros de datos para cada hiperescalador a nivel de ciudad, estado y país. Incluya los 12 hiperescaladores en el archivo de Excel. No hagas web scraping. Genere un archivo de Excel del resultado y formatee el texto en el archivo de Excel para que no contenga corchetes ({}), comillas (‘), asteriscos dobles (**) ni ningún código HTML para mejorar la legibilidad. Nombra el archivo de Excel, Gemini_Experimental_1206_test.xlsx.
A continuación, cree una tabla de tres columnas de ancho y siete columnas de profundidad. La primera columna se titula Hiperescalador, la segunda Características únicas y diferenciadores y la tercera, Infraestructura y ubicaciones de centros de datos. Pon en negrita los títulos de las columnas y céntralos. Los títulos de los hiperescaladores también están en negrita. Verifique dos veces para asegurarse de que el texto dentro de cada celda de esta tabla se ajuste y no pase a la siguiente celda. Ajuste la altura de cada fila para asegurarse de que todo el texto quepa en la celda deseada. Esta tabla compara Amazon Web Services (AWS), Google Cloud Platform (GCP), IBM Cloud, Meta Platforms (Facebook), Microsoft Azure y Oracle Cloud. Centre la tabla en la parte superior de la página de resultados.
A continuación, tomemos Amazon Web Services (AWS), Google Cloud Platform (GCP), IBM Cloud, Meta Platforms (Facebook), Microsoft Azure y Oracle Cloud y defina los ocho aspectos más diferenciadores del grupo. Utilice esos ocho aspectos diferenciadores para crear un gráfico de araña que compare estos seis hiperescaladores. Cree un único gráfico de araña grande que muestre claramente las diferencias en estos seis hiperescaladores, utilizando diferentes colores para mejorar su legibilidad y la capacidad de ver los contornos o huellas de diferentes hiperescaladores. Asegúrese de titular el análisis, Lo que más diferencia a los hiperescaladores, diciembre de 2024. Asegúrese de que la leyenda sea completamente visible y no esté encima del gráfico.
Agregue el gráfico de la araña en la parte inferior de la página. Centre el gráfico de araña debajo de la tabla en la página de salida.
Estos son los hiperescaladores que se incluirán en el script Python: Alibaba Cloud, Amazon Web Services (AWS), Digital Realty, Equinix, Google Cloud Platform (GCP), Huawei, IBM Cloud, Meta Platforms (Facebook), Microsoft Azure, NTT Global Data. Centros, Oracle Cloud, Tencent Cloud.
Noticias
Implementación y evaluación de un modelo de enseñanza de pasantía quirúrgica optimizado utilizando ChatGPT | Educación Médica BMC

Los avances tecnológicos están haciendo avanzar significativamente la educación médica. Actualmente, el desarrollo del plan de estudios médico enfatiza la mejora de los métodos de enseñanza a través de la simulación médica, la discusión de la literatura y la investigación. Existe una creciente defensa de la integración de la inteligencia artificial y las pautas clínicas en la enseñanza para cultivar mejor el razonamiento clínico y las habilidades de pensamiento lógico de los estudiantes.
Múltiples estudios han demostrado los beneficios potenciales de ChatGPT en la educación médica. Los chatbots como ChatGPT pueden ser una herramienta poderosa para mejorar la alfabetización sanitaria, especialmente entre estudiantes y jóvenes estudiantes. [6]. En primer lugar, ChatGPT ofrece acceso rápido e inmediato a amplia información médica, lo que ayuda a los estudiantes de medicina novatos a analizar datos médicos complejos. [7]. En segundo lugar, al crear escenarios y estudios de casos, ChatGPT ayuda a los estudiantes a perfeccionar y mejorar sus habilidades de planificación de diagnóstico y tratamiento, mejorando así sus capacidades de razonamiento clínico y su preparación para situaciones clínicas del mundo real. [8]. En tercer lugar, ChatGPT puede respaldar las tareas académicas respondiendo preguntas y redactando resúmenes. Su capacidad para crear esquemas y revisiones de la literatura puede agilizar la investigación médica. Además, también facilita el resumen de publicaciones relevantes y destaca hallazgos importantes, lo que ayuda a los investigadores médicos a navegar por la gran cantidad de material disponible en línea. [9]. Finalmente, ChatGPT permite el aprendizaje personalizado para los estudiantes al actuar como tutor o asistente virtual, ayudándolos con las tareas y fomentando experiencias de aprendizaje interactivas. [10].
En este estudio, ChatGPT se utilizó en cuatro funciones clave en las fases de educación médica previa, en clase y posterior a la clase. Durante la fase de preparación previa a la clase, los estudiantes pudieron consultar ChatGPT sobre cualquier problema que encontraron, lo que facilitó una comprensión inicial de conceptos, terminología y casos médicos fundamentales. En un estudio, se pudo generar una serie de imágenes a partir de texto descriptivo utilizando un modelo de aprendizaje profundo basado en redes generativas adversarias. La herramienta se utiliza en el proceso narrativo visual para facilitar el aprendizaje mejorado por la tecnología y mejorar las habilidades de razonamiento lógico. [11]. Los modelos de aprendizaje profundo basados en redes generativas adversarias desempeñan un papel clave en la simulación de varios tipos de entornos de aprendizaje y ayudan a desarrollar habilidades prácticas en modelos de asistentes de enseñanza virtuales. Los resultados experimentales muestran que este modelo mejora el efecto de aprendizaje de los estudiantes y mejora su motivación y capacidad de aprendizaje. [12]. En el aula, se empleó ChatGPT para simular las interacciones con los pacientes, proporcionando una plataforma para que los estudiantes practiquen habilidades de diagnóstico y comunicación en un entorno seguro y controlado. En sus interacciones con ChatGPT, los estudiantes son libres de practicar habilidades de diagnóstico y comunicación sin los riesgos que podría representar un paciente real. Un diagnóstico falso o una falta de comunicación no tiene un impacto real en el paciente, lo que permite a los estudiantes aprender mediante prueba y error. ChatGPT está disponible y los estudiantes pueden practicar a su propio ritmo y necesidades de aprendizaje, sin depender de un tiempo y lugar específicos. Esta flexibilidad hace que el aprendizaje sea más eficiente y conveniente. ChatGPT puede simular una variedad de escenarios clínicos y características del paciente para brindar una experiencia interactiva diversa. Los estudiantes están expuestos a diferentes condiciones y antecedentes de pacientes, mejorando así su capacidad para afrontar situaciones complejas. Después de clase, los estudiantes pueden interactuar con ChatGPT individualmente o en grupos de estudio, discutiendo preguntas de práctica proporcionadas por la herramienta, abordando preguntas difíciles o desafiantes y explorando el material desde varias perspectivas. A lo largo del proceso interactivo, los estudiantes evaluaron continuamente su comprensión del material, identificaron sus debilidades y ajustaron sus estrategias de aprendizaje y áreas de enfoque de manera oportuna para enfocarse en áreas específicas para revisión y refuerzo, asegurando que se mantuvieran en el camino correcto. [13]. De manera similar, los instructores podrían utilizar ChatGPT para recopilar recursos didácticos y estudios de casos relevantes durante la fase de preparación de la lección. Al aprovechar ChatGPT, podrían mejorar la participación de los estudiantes en el aula y utilizar la herramienta después de clase para recopilar y analizar los comentarios de los estudiantes sobre el proceso de enseñanza. Además, los estudiantes podrían utilizar ChatGPT para resolver rápidamente cualquier confusión relacionada con el conocimiento profesional. Con la capacitación del modelo ChatGPT, los estudiantes de medicina y los médicos pueden mejorar su razonamiento clínico y sus habilidades de toma de decisiones, mejorando así el desempeño del análisis y diagnóstico de casos. Además, ChatGPT proporciona a los estudiantes de medicina una experiencia de aprendizaje personalizada y eficiente a través de conversaciones simuladas, tutorías inteligentes y preguntas y respuestas automatizadas, profundizando así la comprensión de los conocimientos médicos de los estudiantes. [14].
Los resultados de este estudio indican que las puntuaciones teóricas de los grupos de estudio fueron significativamente más altas que las de los grupos de control, lo que refleja mejores resultados de aprendizaje. No se observaron diferencias significativas en las puntuaciones entre los dos grupos de estudio ni entre los dos grupos de control. Esto sugiere que la aplicación de ChatGPT en los grupos de estudio resultó en una comprensión y dominio superiores del conocimiento teórico en comparación con los métodos de enseñanza tradicionales utilizados en los grupos de control.
Los resultados de satisfacción docente de este estudio indican que los estudiantes de los grupos de estudio que utilizaron ChatGPT informaron puntuaciones de satisfacción total significativamente más altas, así como mejores calificaciones en la organización del curso y los métodos de enseñanza, en comparación con los grupos de control. Las diferencias en la satisfacción con el contenido del curso y los instructores fueron relativamente menores, lo que sugiere que el uso de ChatGPT como ayuda didáctica, a través de su novedoso y atractivo formato interactivo de preguntas y respuestas, su fuerte interactividad y su enfoque estructurado, parece mejorar la participación de los estudiantes. y participación en el aprendizaje. Esto indica que ChatGPT puede fomentar eficazmente un mayor interés y promover resultados educativos. La diferencia más notable entre los métodos de enseñanza radica en la ejecución en el aula; La capacidad de ChatGPT para simular varios escenarios y realizar análisis de casos, combinada con el acceso a recursos didácticos adicionales, mejora significativamente las habilidades de aplicación clínica de los estudiantes de medicina.
La evaluación del estudio sobre el cumplimiento del aprendizaje abarcó cuatro aspectos. Los hallazgos indican que no hubo diferencias significativas entre los grupos en cuanto al establecimiento de planes de aprendizaje. Sin embargo, para los otros tres aspectos (preparación autónoma previa a la clase y revisión posterior a la clase, participación en la enseñanza en el aula y búsqueda de retroalimentación y asistencia), los grupos de estudio exhibieron calificaciones significativamente más altas en comparación con los grupos de control. En muchos estudios y análisis estadísticos, una “puntuación más alta” suele considerarse un resultado positivo, lo que significa que el grupo de estudio obtuvo mejores resultados en algo. Los indicadores de evaluación de este estudio son todos positivos y se puede considerar que una “puntuación más alta” indica un mejor desempeño del grupo de investigación, lo que es un resultado positivo. Esto sugiere que la incorporación de ChatGPT como ayuda didáctica mejora el cumplimiento del aprendizaje de los estudiantes al promover el aprendizaje activo, fomentar el aprendizaje basado en la investigación y mejorar su interés y capacidad para el aprendizaje autónomo.
Si bien las mejoras en el cumplimiento son evidentes, la profundización continua de la comprensión antes, durante y después de la clase también contribuye a mejorar el pensamiento lógico y las habilidades analíticas. En particular, el estudio encontró una tasa relativamente baja de preguntas y solicitudes de ayuda de los estudiantes, durante y después de clase. Las diferencias observadas entre los grupos de estudio y control pueden atribuirse a la capacidad de ChatGPT para ayudar a los estudiantes a superar la timidez y no juzgar los errores. La herramienta de inteligencia artificial ayuda a los estudiantes a superar las dudas, permitiéndoles hacer preguntas de forma libre y repetida sin temor a ser juzgados o interacciones negativas. Al generar materiales de aprendizaje basados en el estado de aprendizaje y las necesidades de cada estudiante, ChatGPT les permite adoptar un enfoque más autónomo del aprendizaje y tener una experiencia educativa adaptada a sus preferencias. Estas interacciones facilitan la aclaración oportuna, una comprensión más profunda y el dominio del material.
ChatGPT también puede adaptar planes y materiales de aprendizaje individualizados para cada estudiante para adaptarse a los diferentes estilos y habilidades de aprendizaje dentro del aula. Este enfoque personalizado fomenta un circuito de retroalimentación positiva, mejorando las capacidades de aprendizaje de los estudiantes.
La aplicación de ChatGPT en la educación médica sigue siendo un tema de considerable debate. Si bien ChatGPT ofrece funcionalidades innovadoras y ventajas potenciales, también plantea varias preocupaciones éticas y prácticas, el potencial de uso indebido, particularmente en los ámbitos de la educación y el mundo académico. [15]. Como chatbot, ChatGPT carece de la capacidad de pensar críticamente como un ser humano, lo que limita su capacidad para interpretar y analizar información médica más allá de sus algoritmos programados. No posee el juicio ni el discernimiento necesarios para los aspectos éticos o legales de la práctica médica y puede plantear riesgos relacionados con violaciones de datos y privacidad. [16, 17].
El auge de herramientas de inteligencia artificial como ChatGPT ha llevado a la deshonestidad académica, con informes de estudiantes que utilizan la tecnología para hacer trampa en sus trabajos de ensayo. [18]. Algunas investigaciones sugieren que ChatGPT puede no ser un recurso confiable para problemas complejos que requieren habilidades y conocimientos avanzados. [19]. Además, los académicos han estado preocupados por la confiabilidad de ChatGPT como fuente creíble de información. [20]. Según muchos educadores, ChatGPT puede ser utilizado fácilmente para hacer trampa por parte de estudiantes que toman cursos de comunicación y filosofía, pero es fácil de identificar. Una preocupación creciente es que los estudiantes eventualmente perderán la capacidad de generar ideas originales y no podrán presentar argumentos adecuados para demostrar un punto. [21]. La accesibilidad tecnológica es un desafío. El uso eficaz de ChatGPT depende de la conectividad de la red y la disponibilidad del dispositivo, lo que puede resultar problemático en diferentes regiones y entre poblaciones estudiantiles específicas. Se deben desarrollar políticas para utilizar ChatGPT en diferentes entornos técnicos. [22]. Una preocupación es la posible devaluación del aprendizaje cooperativo en la educación médica, particularmente en enfoques tradicionales como ABP, CBL y TBL. La colaboración y el trabajo en equipo son cruciales en estos enfoques, y ChatGPT puede reducir involuntariamente la importancia de las interacciones entre humanos. Mantener un equilibrio entre la tecnología y las relaciones es esencial para un aprendizaje eficaz. Si bien ChatGPT mejora el ABP mediante instrucción personalizada, los educadores deben enfatizar la importancia duradera del aprendizaje basado en el paciente y el trabajo en equipo. A pesar de las capacidades de simulación y los conocimientos teóricos de ChatGPT, no puede reemplazar la experiencia práctica obtenida a través de interacciones en el mundo real, especialmente en la educación médica. Reconocer las limitaciones de los modelos es esencial para evitar una dependencia excesiva del aprendizaje por simulación. Integrar perfectamente ChatGPT en los planes de estudio existentes es un desafío que requiere que los educadores inviertan tiempo en diseñar e integrar componentes impulsados por IA que se alineen con los objetivos generales de aprendizaje. [23]. Dadas estas consideraciones, es esencial utilizar ChatGPT con prudencia como herramienta auxiliar de aprendizaje, complementando en lugar de reemplazar los métodos educativos y las técnicas de investigación tradicionales, y siendo consciente de las limitaciones de ChatGPT.
-
Startups7 meses ago
Remove.bg: La Revolución en la Edición de Imágenes que Debes Conocer
-
Recursos8 meses ago
Cómo Empezar con Popai.pro: Tu Espacio Personal de IA – Guía Completa, Instalación, Versiones y Precios
-
Recursos8 meses ago
Suno.com: La Revolución en la Creación Musical con Inteligencia Artificial
-
Recursos7 meses ago
Perplexity aplicado al Marketing Digital y Estrategias SEO
-
Estudiar IA7 meses ago
Curso de Inteligencia Artificial de UC Berkeley estratégico para negocios
-
Tutoriales8 meses ago
Cómo Comenzar a Utilizar ChatGPT: Una Guía Completa para Principiantes
-
Eventos8 meses ago
La nueva era de la inteligencia artificial por el Washington Post – Mayo 2024
-
Noticias6 meses ago
Dos periodistas octogenarios deman a ChatGPT por robar su trabajo