Noticias
Justin Bedecarre Helped OpenAI Find an Office — Now He’s Helping JLL With AI Clients – Commercial Observer
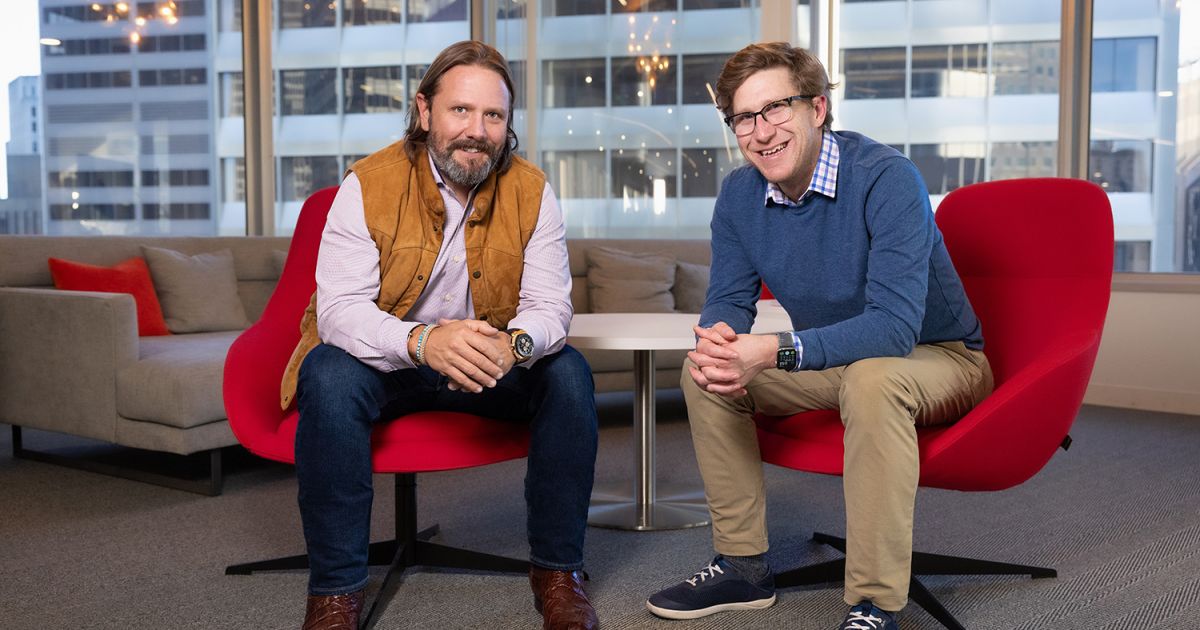
Unless you’ve been living under a rock, you know that generative artificial intelligence, or gen AI, is the next big thing in tech.
And that is enough to make it one of the most closely watched strains of business for the highly challenged commercial real estate industry — more specifically, for office landlords. With acres of space to fill and with loans on buildings coming due, it’s a key potential tenant for that space.
Which is why some eyebrows in real estate went skyward when it became known that none of the major property brokerages was representing OpenAI, the company behind gen AI giant ChatGPT, in its New York office quest.
It was represented instead by San Francisco-based Raise Commercial Real Estate, an 8-year-old boutique brokerage that specializes in AI and other cutting-edge tech companies and their real estate needs. OpenAI’s search came to an end in October when the company signed a lease for 90,000 square feet at the Puck Building in SoHo. By the time it actually did the deal, Newmark was OpenAI’s brokerage house.
Then, on Oct. 1, JLL (JLL) announced its intent to buy Raise. JLL said Raise’s cloud-based platform would enable it to serve clients demanding the latest in tech-related services. Justin Bedecarre, co-founder and CEO of Raise, would become JLL’s head of Americas leasing innovation, reporting to Andy Poppink, JLL’s CEO for markets advisory and onetime captain of Stanford University’s basketball team.
According to data from JLL senior analyst Chris Pham, JLL repped a little less than 60 percent of all AI deals in the San Francisco Bay area from 2023 through the first three quarters of this year. So, the acquisition of Raise enhances JLL in this area.
Beddecarre and Felipe Gomez-Kraus, a former Raise colleague and the executive managing director in brokerage in JLL’s San Francisco office, hopped on Microsoft Teams in early December to explain what an AI-empowered world for commercial real estate might look like.
This interview has been edited for length and clarity.
Commercial Observer: What happened with Raise Commercial, and what are you doing now?
Justin Bedecarre: Raise was celebrated for many years as a technology-powered commercial real estate brokerage and, as of a couple of months ago, we joined forces with JLL, so now we’re all one JLL.
Is this a kind of ‘If you can’t beat em, join em’ situation?
Bedecarre: We saw an opportunity to have the best of both worlds by joining forces with JLL. We complement each other very well with JLL’s scale and our technology platform, and so it was very much a positive sum mentality by coming together.
So what are you doing now for JLL?
Bedecarre: We are doing a lot of the same things, but at a bigger scale. We’re serving a lot of AI companies with our platform and with our broker services. We’re scaling our technology platform across the leasing business, and so it’s a lot of the same but at a bigger scale. And, with what we’ve done in the Bay Area in particular — commanding, you know, almost two-thirds of AI companies, helping them to client build and manage offices — we’re off to the races.
Felipe Gomez-Kraus: When we were building Raise, it was going to allow us to serve clients in a digital-first collaborative workplace, which was the first of its kind. So, coming together with JLL was also in order to make it so that JLL clients can benefit from having a collaborative workplace where they can interact with the market and with their teams nationally and globally. When Justin says we’re doing the same, it is really to bring that platform to the brokerage teams and the clients of JLL, and to continue to provide the type of service that should be happening in 2025.
Did you guys find that JLL among the other real estate services firms was ahead in terms of understanding tech and cutting-edge startups and what they needed from their real estate?
Bedecarre: Without question — both on the decision and execution around getting technology to our brokers and our clients, but also in the clients we serve. We cover more market share around AI companies than any other firm. It’s definitely the best of both worlds in terms of what we’re able to do here with all of our now-JLL colleagues to serve the best companies in the world.
Isn’t it risky for a big legacy firm like JLL, which is dealing with the overall reduction in demand for offices, to make this kind of acquisition?
Bedecarre: I would posit that it’s a risk for a quote-unquote legacy firm to not try to innovate and meet the continued demands of companies that are digital first or digital native; and that are all feeling the impacts of technology writ large, including that of AI.
So I would say that stagnation is a reversal of fortune for most legacy companies. One of the reasons we came together with JLL, and were so happy to do so, was the very forward-thinking nature of the company. And that’s also reflected in the practice groups that are developed to really understand the needs of occupiers of tomorrow, landlords of tomorrow, and how to best position both assets and the occupants for the right match.
Gomez-Kraus: The foundation of your question is the reduction in demand. I would actually counter that and say that, particularly around AI companies, the demand has gone up. We’re really looking at the future and the growth areas for office occupancy, and that’s very much the case with AI coming.
But what with traditional, non-tech companies like banks and other financial and professional services firms reducing their demand and trying for fancier offices, and since work from home isn’t entirely going away, can AI replace traditional office demand?
FGK: You describe the flight to quality, which is absolutely what we’re seeing. Workplaces today, more than ever, need to be well located in the right transit lines, amenitized properly in the common spaces. And the workplaces themselves need to be thoughtfully designed for how work happens today. We believe that that will be a part of the way that the world works moving forward.
But, with that, I often hear it said, “You build a church for Sundays.” That means that as long as you’re bringing together people for any period of time to do whatever their best work might be, it should be in a space that is well designed, whether it’s individual head-down work or collaborative spaces, as well as other things that come into play as a reward for talent.
You talk about legacy firms, financial services, accounting firms and the like — they are competing for talent with a broader range of companies. I don’t think it is in many of those companies’ best interests to be in secondary markets or submarkets and in Class B, B-minus buildings. It’s demonstrated that this is one of the factors to hire and retain talent.
So we don’t think that AI is going to replace the reduction in footprint. But companies that existed pre-pandemic, they are absolutely rightsizing and flying to quality. Another thing that’s happening is that there’s a resurgence of net new companies that are being built, and those companies that are being built from today forward are being funded with an eye toward the velocity and intensity and the creativity that happens when people are together and they’re not forced to start as they were in the pandemic, fully remote.
Lately JLL’s stock has been on a tear. Do you think that’s at least in part due to the notion that JLL is ahead of the game in implementing AI and understanding what’s going on in high tech?
Bedecarre: There are a lot of factors that go into why we joined JLL: the culture, the vision, and how the firm wants to serve its brokers and our clients and our teams. It’s been incredibly positive; the talent density is extremely high at JLL. And we serve the best companies in the world, both investors and landlords and tenants.
What do you think is the most important thing landlords need to know in attracting cutting-edge tech companies to their offices?
Gomez-Kraus: There’s a variety of ways that you could position an asset to make it more attractive for the types of companies that are excited to bring people together to work, and that want to make those workplaces inspiring and reflective of their culture. This is outside of the building’s location, which obviously is paramount and is even more important today.
In 2019 and 2020, when we were at sub-3 percent vacancy in San Francisco, there were buildings in secondary submarkets that might have been on an island, but had to be considered because there was no real space available of any size downtown. I mean figurative islands that are away from transit routes, which allow San Francisco to draw people in from the East Bay or the South Bay.
The things that landlords can do from an amenitization standpoint — bringing in thoughtfully curated food and beverage options, health and wellness — is incredibly important to this young cohort and the companies that want to keep these, let’s say, AI-specific researchers who do very heavy work.
It’s in a company’s best interest to make it as comfortable for that person to spend a long period of time at the office or in its surroundings, which means not just a one-to-one desk ratio, but rather spaces where that person can go, be inspired and motivated, sit in a nontraditional work space, collaborate with colleagues in scrum rooms, or in what they call “me space” versus “we space.” You see from a design standpoint it’s looking a lot more like a hotel lobby than a traditional office.
This smacks of the startup environment in 2014, `15, and `16, in San Francisco, where there was ample access to funding for the right companies. That fuels headcount growth and office needs, though today’s hybrid work formats change some of those leasing decisions.
I think that term length is important. For the smaller-sized offices, I think they should try to do everything to reduce friction, and that can represent itself in creating spec suites that are well designed, furnished, and reduce friction to get in, but then also keeping term length on the shorter side. As they become larger, and want to both plant a flag and have more visibility, that could be in the same building or in a neighboring building.
Isn’t energy use also an issue, especially for tech companies?
Bedecarre: Not necessarily for your typical tech company, or even largely AI companies. Your typical office user might be manufacturing things in the office. Some of our clients build satellites that are literally in space right now. They need extra power to build the thing in their office.
When you’re thinking about tech companies and AI companies, their large language models and whatnot are done off site, in separate facilities. Those spaces are designed specifically. But for the typical office user versus an AI company, there’s not an exceptional difference in need for power.
Now you guys were in the middle of OpenAI’s search for space in New York. Can you talk about that?
Gomez-Kraus: We can’t speak about individual clients, but I think one of the things that you’ll see across the talent density for companies that are building the technology of tomorrow is that we’re seeing again what we saw in the runup to 2019, 2020 — where the talent density in the Bay Area, and the level of competition for that talent, causes many of these companies to go look in other incredible cities like New York and London and elsewhere.
How important is planting a flag in New York for AI in general?
Bedecarre: I don’t think having a space for its own sake anywhere in particular is important to these companies, but the drivers for putting an office location anywhere are absolutely related to the available density of talent — and potentially to the density of the firms and organizations to which they are selling and where they’re finding their partners.
Gomez-Kraus: The only thing I would add to that is the concentration of investment dollars. After the Bay Area, New York provides the highest concentration of funding going to startups and AI companies. That’s what creates these ecosystems.
What is there specific about AI that you think landlords should know?
Gomez-Kraus: There are a lot of similarities between the occupier profile of an AI company and what was seen when the Googles and the Metas of the world were in their run-up. They were building a technology of tomorrow.
They firmly believe that people are better together for the philosophy of what they’re developing, the creativity that is born in it. They are committed to delivering excellent spaces, not only for them to inspire the people that they have, but also from a recruiting standpoint, because, make no bones about it, much of this is rooted in the war for talent, just as it was in 2013.
One of the ways that a company could demonstrate their commitment to their employee back then — beyond the stock options — was truly to have a holistic approach to their health and wellness, their experience, their commutes, their comfort. A lot of that is reflected in the quality of the workplaces that they deliver.
One last thing: What is going on in San Francisco? It seems like we hear that San Francisco has more vacant office than any central business district across the country, and its problems still linger.
Bedecarre: If you know anyone who has the capital to invest, there’s probably not a better bet than San Francisco.
I care a lot about the city and the region as a whole. We’ve had to reinvent ourselves many times over. Looking back from the dot-com crash, every time we rebounded. And that’s very much the case now. More people are coming back to the office. Those numbers just keep on going up. Also, the political environment has improved immensely for businesses to be starting and growing in San Francisco.
Noticias
O3 de OpenAI aún no es AGI, pero simplemente hizo algo que ninguna otra IA ha hecho
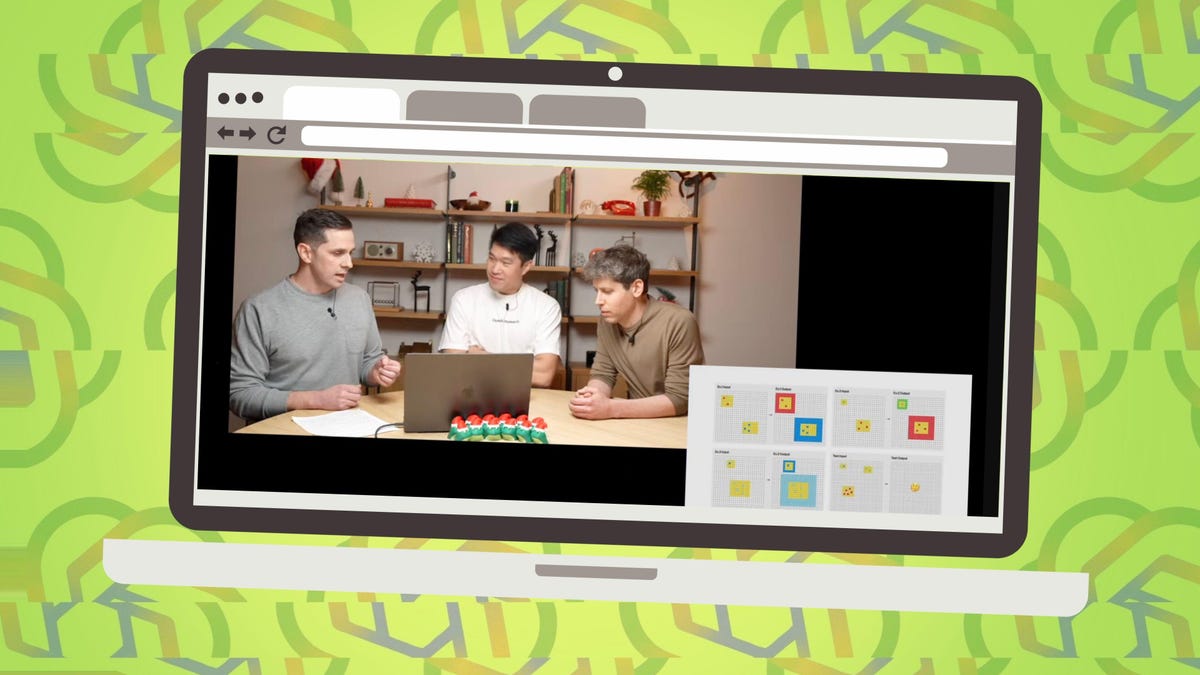
Sam Altman y los diputados de OpenAI analizan el rendimiento del nuevo modelo o3 en la prueba ARC-AGI.
OpenAI/ZDNET
El último modelo de lenguaje grande de OpenAI aún no está disponible, pero ya tenemos algunas formas de saber qué puede y qué no puede hacer.
El lanzamiento “o3” de OpenAI se dio a conocer el 20 de diciembre en forma de un video infomercial, lo que significa que la mayoría de las personas ajenas a la empresa no tienen idea de lo que realmente es capaz de hacer. (Se está dando acceso anticipado a los grupos externos de pruebas de seguridad).
Además: 15 formas en que la IA me ahorró tiempo en el trabajo en 2024
Aunque el video presentó mucha discusión sobre varios logros de referencia, el mensaje del cofundador y director ejecutivo de OpenAI, Sam Altman, en el video fue muy breve. Su afirmación más importante, y además vaga, fue que o3 “es un modelo increíblemente inteligente”.
ARC-AGI puso a prueba o3
OpenAI planea lanzar la versión “mini” de o3 a finales de enero y la versión completa algún tiempo después, dijo Altman.
Un outsider, sin embargo, ha tenido la oportunidad de poner a prueba a o3, en cierto sentido.
La prueba, en este caso, se llama “Corpus de Abstracción y Razonamiento para la Inteligencia General Artificial” o ARC-AGI. Es una colección de “desafíos para los sistemas inteligentes”, un nuevo punto de referencia. El ARC-AGI se anuncia como “el único punto de referencia diseñado específicamente para medir la adaptabilidad a la novedad”. Eso significa que está destinado a evaluar la adquisición de nuevas habilidades, no sólo el uso de conocimientos memorizados.
Además: ¿Por qué la ética se está convirtiendo en el mayor desafío de la IA?
Algunos consideran la AGI, inteligencia artificial general, como el Santo Grial: el logro de un nivel de inteligencia artificial que podría igualar o superar la inteligencia humana. La idea de ARC-AGI es guiar la IA hacia “sistemas artificiales más inteligentes y más parecidos a los humanos”.
El modelo o3 obtuvo una precisión del 76% en ARC-AGI en una evaluación coordinada formalmente por OpenAI y el autor de ARC-AGI, François Chollet, científico de la unidad de inteligencia artificial de Google.
Un cambio en las capacidades de la IA
En el sitio web de ARC-AGI, Chollet escribió la semana pasada que la puntuación del 76% es la primera vez que la IA supera la puntuación de un humano en el examen, como lo ejemplifican las respuestas de los trabajadores humanos de Mechanical Turk que tomaron la prueba y quienes, en promedio, obtuvo una puntuación ligeramente superior al 75% de aciertos.
Chollet escribió que la puntuación alta es “un aumento sorprendente e importante de la función escalonada en las capacidades de la IA, que muestra una capacidad novedosa de adaptación a tareas nunca antes vista en los modelos de la familia GPT”. Y añadió: “Toda la intuición sobre las capacidades de la IA deberá actualizarse para o3”.
El logro marca “un verdadero avance” y “un cambio cualitativo en las capacidades de la IA”, declaró Chollet. Chollet predice que la capacidad de o3 para “adaptarse a tareas que nunca antes había enfrentado” significa que “debe planificar que estas capacidades sean competitivas con el trabajo humano en un plazo bastante corto”.
Los comentarios de Chollet son dignos de mención porque nunca ha sido un defensor de la IA. En 2019, cuando creó ARC-AGI, me dijo en una entrevista que tuvimos para ZDNET que el flujo constante de “artículos de prensa grandilocuentes” de empresas de IA “sugieren engañosamente que la IA a nivel humano quizás esté a unos años de distancia”, mientras que consideraba tal hipérbole “una ilusión”.
Las preguntas ARC-AGI son fáciles de entender para las personas y bastante fáciles de resolver. Cada desafío muestra de tres a cinco ejemplos de la pregunta y la respuesta correcta, y luego al examinado se le presenta una pregunta similar y se le pide que proporcione la respuesta que falta.
La forma básica de ARC-AGI es tener de tres a cinco ejemplos de entrada y salida, que representan la pregunta y su respuesta, y luego un ejemplo final de entrada para el cual se debe proporcionar la respuesta proporcionando la imagen de salida correcta. Es bastante fácil para un humano descubrir qué imagen producir tocando píxeles de colores, incluso si no puede articular la regla per se.
Premio ARCP
Las preguntas no están basadas en texto sino en imágenes. Primero se muestra una cuadrícula de píxeles con formas coloreadas, seguida de una segunda versión que ha sido modificada de alguna manera. La pregunta es: ¿Cuál es la regla que cambia la imagen inicial en la segunda imagen?
En otras palabras, el desafío no depende directamente del lenguaje natural, el área célebre de los grandes modelos de lenguaje. En cambio, prueba la formulación de patrones abstractos en el dominio visual.
Pruebe ARC-AGI usted mismo
Puede probar ARC-AGI usted mismo en el sitio web del desafío de Chollet. Respondes al desafío “dibujando” en una cuadrícula vacía, completando cada píxel con el color correcto para crear la cuadrícula correcta de píxeles de colores como “respuesta”.
Es divertido, como jugar al Sudoku o al Tetris. Lo más probable es que, incluso si no puedes articular verbalmente cuál es la regla, descubrirás rápidamente qué cuadros deben colorearse para producir la solución. La parte que lleva más tiempo es tocar cada píxel de la cuadrícula para asignar su color.
Además: Por qué el avance cuántico de Google es “realmente notable” y qué sucederá después
Una respuesta correcta produce una animación de lanzamiento de confeti en la página web y el mensaje: “Has resuelto el rompecabezas diario del premio ARC. Aún eres más (generalmente) inteligente que la IA”.
Tenga en cuenta que cuando o3 o cualquier otro modelo realiza la prueba, no actúa directamente sobre los píxeles. En cambio, el equivalente se introduce en la máquina como una matriz de filas y columnas de números que deben transformarse en una matriz diferente como respuesta. Por lo tanto, los modelos de IA no “ven” la prueba de la misma manera que lo hace un humano.
Lo que aún no está claro
A pesar de los logros de o3, es difícil hacer declaraciones definitivas sobre las capacidades de o3. Debido a que el modelo de OpenAI es de código cerrado, todavía no está claro exactamente cómo el modelo resuelve el desafío.
Al no ser parte de OpenAI, Chollet tiene que especular sobre cómo o3 está haciendo lo que está haciendo.
Conjetura que el logro es el resultado de que OpenAI cambió la “arquitectura” de o3 con respecto a la de sus predecesores. Una arquitectura en IA se refiere a la disposición y relación de los elementos funcionales que dan estructura al código.
Además: si ChatGPT produce código generado por IA para tu aplicación, ¿a quién pertenece realmente?
Chollet especula en el blog que “en el momento de la prueba, el modelo busca en el espacio de posibles Cadenas de Pensamiento (CoT) que describen los pasos necesarios para resolver la tarea, de una manera tal vez no muy diferente a la búsqueda en árbol de Monte Carlo al estilo AlphaZero”.
El término cadena de pensamiento se refiere a un enfoque cada vez más popular en la IA generativa en el que el modelo de IA puede detallar la secuencia de cálculos que realiza en busca de la respuesta final. AlphaZero es el famoso programa de inteligencia artificial de la unidad DeepMind de Google que venció a los humanos en el ajedrez en 2016. Una búsqueda de árboles de Monte Carlo es un enfoque informático que existe desde hace décadas.
En un intercambio de correo electrónico, Chollet me contó un poco más sobre su pensamiento. Le pregunté cómo llegó a esa idea de una búsqueda de cadenas de pensamiento. “Claramente, cuando el modelo está ‘pensando’ durante horas y generando millones de tokens en el proceso de resolver un único rompecabezas, debe estar haciendo algún tipo de búsqueda”, respondió Chollet.
Chollet añadió:
Es completamente obvio por las características de latencia/coste del modelo que está haciendo algo completamente diferente a la serie GPT. No es la misma arquitectura, ni nada remotamente parecido. El factor definitorio del nuevo sistema es una gran cantidad de búsquedas en el momento de la prueba. Anteriormente, 4 años de ampliación de la misma arquitectura (la serie GPT) no habían producido ningún progreso en ARC, y ahora este sistema, que claramente tiene una nueva arquitectura, está creando un cambio funcional gradual en las capacidades, por lo que la arquitectura lo es todo.
Hay una serie de advertencias aquí. OpenAI no reveló cuánto dinero se gastó en una de sus versiones de o3 para resolver ARC-AGI. Esa es una omisión significativa porque un criterio de ARC-AGI es el costo en dólares reales de usar chips GPU como proxy de la “eficiencia” del modelo de IA.
Chollet me dijo en un correo electrónico que el enfoque de o3 no equivale a un enfoque de “fuerza bruta”, pero bromeó: “Por supuesto, también se podría definir la fuerza bruta como ‘lanzar una cantidad excesiva de cómputo a un problema simple’. ‘ en cuyo caso se podría decir que es fuerza bruta”.
Además, Chollet señala que o3 fue entrenado para realizar la prueba ARC-AGI utilizando el conjunto de datos de entrenamiento de la competencia. Eso significa que aún no está claro cómo abordaría el examen una versión limpia de o3, sin preparación para el examen.
También: El generador de vídeo Sora AI de OpenAI ya está aquí: cómo probarlo
Chollet me dijo en un correo electrónico: “Será interesante ver qué puntuación obtiene el sistema base sin información relacionada con ARC, pero en cualquier caso, el hecho de que el sistema esté ajustado para ARC a través del conjunto de entrenamiento no invalida su rendimiento”. “Para eso está el conjunto de entrenamiento. Hasta ahora nadie había podido lograr puntuaciones similares, incluso después de entrenar en millones de tareas ARC generadas”.
o3 todavía falla en algunas tareas fáciles
A pesar de la incertidumbre, una cosa parece muy clara: quienes anhelan AGI se sentirán decepcionados. Chollet enfatiza que la prueba ARC-AGI es “una herramienta de investigación” y que “aprobar ARC-AGI no equivale a alcanzar AGI”.
“De hecho, no creo que o3 sea AGI todavía”, escribe Chollet en el blog ARC-AGI. “O3 todavía falla en algunas tareas muy fáciles, lo que indica diferencias fundamentales con la inteligencia humana”.
Para demostrar que todavía no estamos al nivel de inteligencia humana, Chollet señala algunos de los problemas simples en ARC-AGI que o3 no puede resolver. Uno de esos problemas implica simplemente mover un cuadrado de color en una cantidad determinada, un patrón que rápidamente se vuelve claro para un ser humano.
Un problema de ejemplo de ARC-AGI donde falló el modelo o3.
Premio ARCP
Chollet planea presentar una nueva versión de ARC-AGI en enero. Predice que reducirá drásticamente los resultados de o3. “Sabrás que AGI está aquí cuando el ejercicio de crear tareas que sean fáciles para los humanos comunes pero difíciles para la IA se vuelva simplemente imposible”, concluye.
Noticias
Prueba de manejo del modelo Gemini-Exp-1206 de Google en análisis de datos y visualizaciones
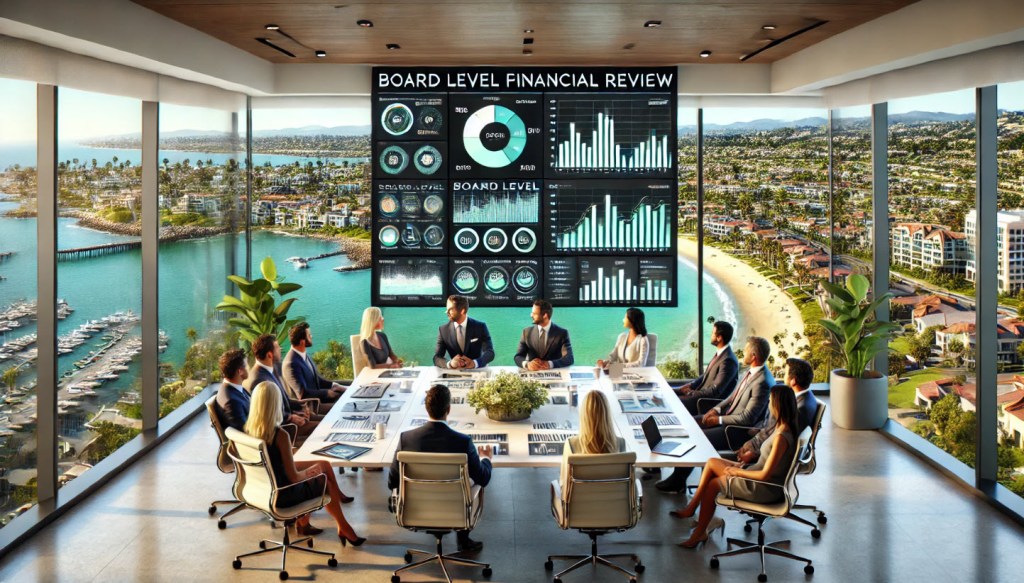
Únase a nuestros boletines diarios y semanales para obtener las últimas actualizaciones y contenido exclusivo sobre la cobertura de IA líder en la industria. Más información
Uno de los últimos modelos experimentales de Google, Gemini-Exp-1206, muestra el potencial de aliviar uno de los aspectos más agotadores del trabajo de cualquier analista: lograr que sus datos y visualizaciones se sincronicen perfectamente y proporcionen una narrativa convincente, sin tener que trabajar toda la noche. .
Los analistas de inversiones, los banqueros junior y los miembros de equipos de consultoría que aspiran a puestos de socios asumen sus roles sabiendo que largas horas de trabajo, fines de semana y pasar toda la noche ocasionalmente podrían darles una ventaja interna en un ascenso.
Lo que consume gran parte de su tiempo es realizar análisis de datos avanzados y al mismo tiempo crear visualizaciones que refuercen una historia convincente. Lo que hace que esto sea más desafiante es que cada firma bancaria, fintech y consultora, como JP Morgan, McKinsey y PwC, tiene formatos y convenciones únicos para el análisis y visualización de datos.
VentureBeat entrevistó a miembros de equipos de proyectos internos cuyos empleadores habían contratado a estas empresas y las habían asignado al proyecto. Los empleados que trabajan en equipos dirigidos por consultores dijeron que producir imágenes que condensen y consoliden la enorme cantidad de datos es un desafío persistente. Uno dijo que era común que los equipos de consultores trabajaran durante la noche y hicieran un mínimo de tres o cuatro iteraciones de las visualizaciones de una presentación antes de decidirse por una y prepararla para las actualizaciones a nivel de tablero.
Un caso de uso convincente para probar el último modelo de Google
El proceso en el que confían los analistas para crear presentaciones que respalden una historia con visualizaciones y gráficos sólidos tiene tantos pasos manuales y repeticiones que resultó ser un caso de uso convincente para probar el último modelo de Google.
Al lanzar el modelo a principios de diciembre, Patrick Kane de Google escribió: “Ya sea que esté enfrentando desafíos complejos de codificación, resolviendo problemas matemáticos para proyectos escolares o personales, o brindando instrucciones detalladas de varios pasos para elaborar un plan de negocios personalizado, Gemini-Exp-1206 le ayudará a navegar tareas complejas con mayor facilidad”. Google notó el rendimiento mejorado del modelo en tareas más complejas, incluido el razonamiento matemático, la codificación y el seguimiento de una serie de instrucciones.
VentureBeat llevó el modelo Exp-1206 de Google a una prueba exhaustiva esta semana. Creamos y probamos más de 50 scripts de Python en un intento de automatizar e integrar análisis y visualizaciones intuitivas y fáciles de entender que pudieran simplificar los datos complejos que se analizan. Dado que los hiperescaladores dominan los ciclos de noticias actuales, nuestro objetivo específico era crear un análisis de un mercado tecnológico determinado y al mismo tiempo crear tablas de apoyo y gráficos avanzados.
A través de más de 50 iteraciones diferentes de scripts de Python verificados, nuestros hallazgos incluyeron:
- Cuanto mayor es la complejidad de una solicitud de código Python, más “piensa” el modelo e intenta anticipar el resultado deseado. Exp-1206 intenta anticipar lo que se necesita a partir de un mensaje complejo determinado y variará lo que produce incluso con el más mínimo cambio de matiz en un mensaje. Vimos esto en cómo el modelo alternaría entre formatos de tipos de tablas colocadas directamente encima del gráfico de araña del análisis de mercado de hiperescalador que creamos para la prueba.
- Obligar al modelo a intentar realizar análisis y visualización de datos complejos y producir un archivo Excel genera una hoja de cálculo con varias pestañas. Sin que nunca le pidieran una hoja de cálculo de Excel con varias pestañas, Exp-1206 creó una. El análisis tabular principal solicitado estaba en una pestaña, las visualizaciones en otra y una tabla auxiliar en la tercera.
- Decirle al modelo que repita los datos y recomiende las 10 visualizaciones que decida que mejor se ajustan a los datos ofrece resultados beneficiosos y reveladores. Con el objetivo de reducir el tiempo que supone tener que crear tres o cuatro iteraciones de presentaciones de diapositivas antes de una revisión por parte de la junta, obligamos al modelo a producir múltiples iteraciones conceptuales de imágenes. Estos podrían limpiarse e integrarse fácilmente en una presentación, ahorrando muchas horas de trabajo manual creando diagramas en diapositivas.
Impulsando a Exp-1206 hacia tareas complejas y en capas
El objetivo de VentureBeat era ver hasta dónde se podía llevar el modelo en términos de complejidad y tareas en capas. Su desempeño en la creación, ejecución, edición y ajuste de 50 scripts de Python diferentes mostró cuán rápido el modelo intenta captar matices en el código y reaccionar de inmediato. El modelo se flexiona y se adapta según el historial de indicaciones.
El resultado de ejecutar el código Python creado con Exp-1206 en Google Colab mostró que la granularidad matizada se extendía al sombreado y la translucidez de las capas en un gráfico de araña de ocho puntos que fue diseñado para mostrar cómo se comparan seis competidores hiperescaladores. Los ocho atributos que le pedimos a Exp-1206 que identificara en todos los hiperescaladores y que anclara el gráfico de araña se mantuvieron consistentes, mientras que las representaciones gráficas variaron.
Batalla de los hiperescaladores
Elegimos los siguientes hiperescaladores para comparar en nuestra prueba: Alibaba Cloud, Amazon Web Services (AWS), Digital Realty, Equinix, Google Cloud Platform (GCP), Huawei, IBM Cloud, Meta Platforms (Facebook), Microsoft Azure, NTT Global Data. Centros, Oracle Cloud y Tencent Cloud.
A continuación, escribimos un mensaje de 11 pasos de más de 450 palabras. El objetivo era ver qué tan bien Exp-1206 puede manejar la lógica secuencial y no perder su lugar en un proceso complejo de varios pasos. (Puede leer el mensaje en el apéndice al final de este artículo).
Luego enviamos el mensaje en Google AI Studio, seleccionando el modelo Gemini Experimental 1206, como se muestra en la siguiente figura.
A continuación, copiamos el código en Google Colab y lo guardamos en un cuaderno Jupyter (Comparación de Hyperscaler – Gemini Experimental 1206.ipynb), luego ejecutamos el script de Python. El script se ejecutó sin problemas y creó tres archivos (indicados con las flechas rojas en la parte superior izquierda).
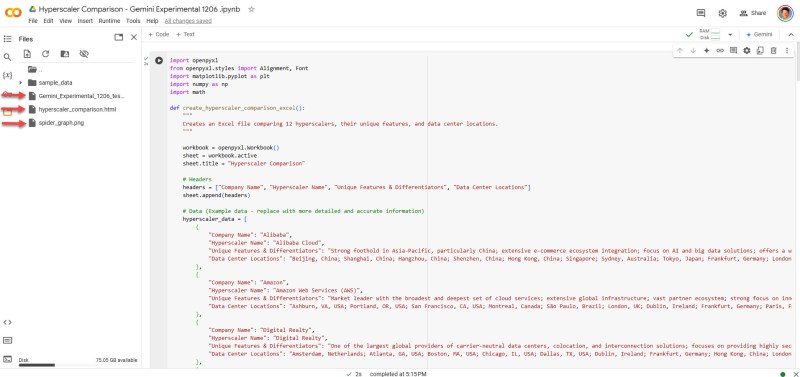
Análisis comparativo de Hyperscaler y un gráfico, en menos de un minuto
La primera serie de instrucciones en el mensaje pedía a Exp-1206 que creara un script de Python que comparara 12 hiperescaladores diferentes por su nombre de producto, características y diferenciadores únicos y ubicaciones de centros de datos. A continuación se muestra cómo resultó el archivo de Excel que se solicitó en el script. Me llevó menos de un minuto formatear la hoja de cálculo para reducirla y ajustarla a las columnas.
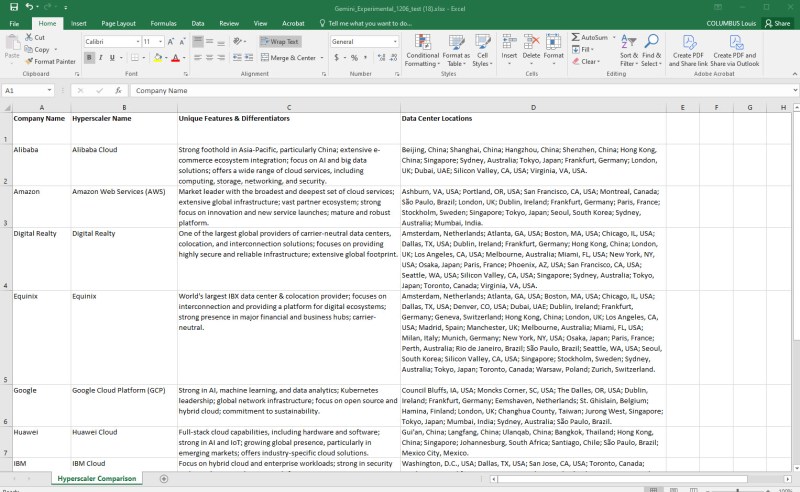
La siguiente serie de comandos solicitó una tabla de los seis principales hiperescaladores comparados en la parte superior de una página y el gráfico de araña a continuación. Exp-1206 eligió por sí solo representar los datos en formato HTML, creando la siguiente página.
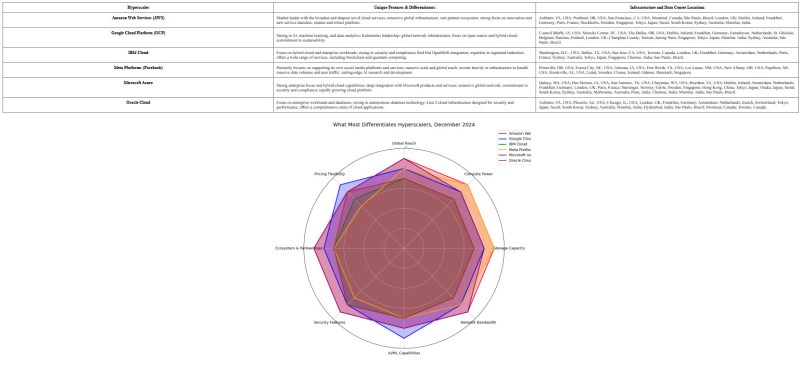
La secuencia final de comandos se centró en la creación de un gráfico de araña para comparar los seis hiperescaladores principales. Le asignamos a Exp-1206 la tarea de seleccionar los ocho criterios para la comparación y completar el gráfico. Esa serie de comandos se tradujo a Python y el modelo creó el archivo y lo proporcionó en la sesión de Google Colab.
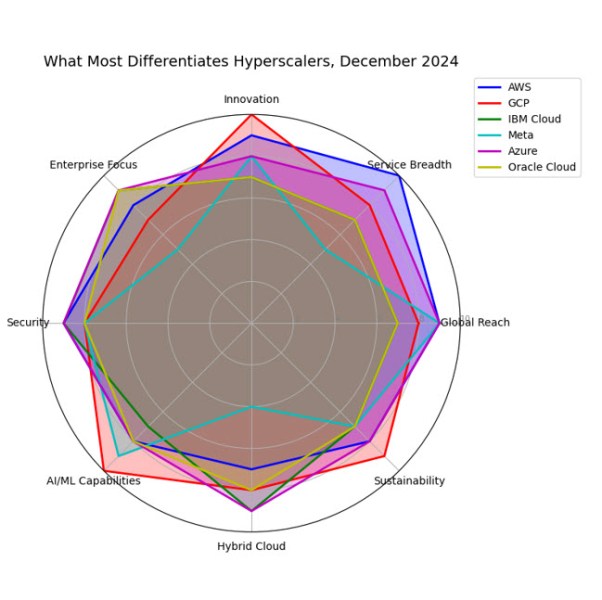
Un modelo diseñado específicamente para ahorrar tiempo a los analistas
VentureBeat ha aprendido que en su trabajo diario, los analistas continúan creando, compartiendo y ajustando bibliotecas de indicaciones para modelos de IA específicos con el objetivo de optimizar los informes, el análisis y la visualización en todos sus equipos.
Los equipos asignados a proyectos de consultoría a gran escala deben considerar cómo modelos como Gemini-Exp-1206 pueden mejorar enormemente la productividad y aliviar la necesidad de semanas laborales de más de 60 horas y noches ocasionales en vela. Una serie de indicaciones automatizadas pueden realizar el trabajo exploratorio de observar las relaciones en los datos, lo que permite a los analistas producir imágenes con mucha mayor certeza sin tener que dedicar una cantidad excesiva de tiempo a llegar allí.
Apéndice:
Prueba rápida de Google Gemini Experimental 1206
Escriba un script de Python para analizar los siguientes hiperescaladores que han anunciado una presencia de centro de datos e infraestructura global para sus plataformas y cree una tabla comparándolos que capture las diferencias significativas en cada enfoque en presencia de centro de datos e infraestructura global.
Haga que la primera columna de la tabla sea el nombre de la empresa, la segunda columna sean los nombres de cada uno de los hiperescaladores de la empresa que tienen presencia de centro de datos e infraestructura global, la tercera columna sea lo que hace que sus hiperescaladores sean únicos y una inmersión profunda en los más diferenciados. características, y la cuarta columna son las ubicaciones de los centros de datos para cada hiperescalador a nivel de ciudad, estado y país. Incluya los 12 hiperescaladores en el archivo de Excel. No hagas web scraping. Genere un archivo de Excel del resultado y formatee el texto en el archivo de Excel para que no contenga corchetes ({}), comillas (‘), asteriscos dobles (**) ni ningún código HTML para mejorar la legibilidad. Nombra el archivo de Excel, Gemini_Experimental_1206_test.xlsx.
A continuación, cree una tabla de tres columnas de ancho y siete columnas de profundidad. La primera columna se titula Hiperescalador, la segunda Características únicas y diferenciadores y la tercera, Infraestructura y ubicaciones de centros de datos. Pon en negrita los títulos de las columnas y céntralos. Los títulos de los hiperescaladores también están en negrita. Verifique dos veces para asegurarse de que el texto dentro de cada celda de esta tabla se ajuste y no pase a la siguiente celda. Ajuste la altura de cada fila para asegurarse de que todo el texto quepa en la celda deseada. Esta tabla compara Amazon Web Services (AWS), Google Cloud Platform (GCP), IBM Cloud, Meta Platforms (Facebook), Microsoft Azure y Oracle Cloud. Centre la tabla en la parte superior de la página de resultados.
A continuación, tomemos Amazon Web Services (AWS), Google Cloud Platform (GCP), IBM Cloud, Meta Platforms (Facebook), Microsoft Azure y Oracle Cloud y defina los ocho aspectos más diferenciadores del grupo. Utilice esos ocho aspectos diferenciadores para crear un gráfico de araña que compare estos seis hiperescaladores. Cree un único gráfico de araña grande que muestre claramente las diferencias en estos seis hiperescaladores, utilizando diferentes colores para mejorar su legibilidad y la capacidad de ver los contornos o huellas de diferentes hiperescaladores. Asegúrese de titular el análisis, Lo que más diferencia a los hiperescaladores, diciembre de 2024. Asegúrese de que la leyenda sea completamente visible y no esté encima del gráfico.
Agregue el gráfico de la araña en la parte inferior de la página. Centre el gráfico de araña debajo de la tabla en la página de salida.
Estos son los hiperescaladores que se incluirán en el script Python: Alibaba Cloud, Amazon Web Services (AWS), Digital Realty, Equinix, Google Cloud Platform (GCP), Huawei, IBM Cloud, Meta Platforms (Facebook), Microsoft Azure, NTT Global Data. Centros, Oracle Cloud, Tencent Cloud.
Noticias
Implementación y evaluación de un modelo de enseñanza de pasantía quirúrgica optimizado utilizando ChatGPT | Educación Médica BMC

Los avances tecnológicos están haciendo avanzar significativamente la educación médica. Actualmente, el desarrollo del plan de estudios médico enfatiza la mejora de los métodos de enseñanza a través de la simulación médica, la discusión de la literatura y la investigación. Existe una creciente defensa de la integración de la inteligencia artificial y las pautas clínicas en la enseñanza para cultivar mejor el razonamiento clínico y las habilidades de pensamiento lógico de los estudiantes.
Múltiples estudios han demostrado los beneficios potenciales de ChatGPT en la educación médica. Los chatbots como ChatGPT pueden ser una herramienta poderosa para mejorar la alfabetización sanitaria, especialmente entre estudiantes y jóvenes estudiantes. [6]. En primer lugar, ChatGPT ofrece acceso rápido e inmediato a amplia información médica, lo que ayuda a los estudiantes de medicina novatos a analizar datos médicos complejos. [7]. En segundo lugar, al crear escenarios y estudios de casos, ChatGPT ayuda a los estudiantes a perfeccionar y mejorar sus habilidades de planificación de diagnóstico y tratamiento, mejorando así sus capacidades de razonamiento clínico y su preparación para situaciones clínicas del mundo real. [8]. En tercer lugar, ChatGPT puede respaldar las tareas académicas respondiendo preguntas y redactando resúmenes. Su capacidad para crear esquemas y revisiones de la literatura puede agilizar la investigación médica. Además, también facilita el resumen de publicaciones relevantes y destaca hallazgos importantes, lo que ayuda a los investigadores médicos a navegar por la gran cantidad de material disponible en línea. [9]. Finalmente, ChatGPT permite el aprendizaje personalizado para los estudiantes al actuar como tutor o asistente virtual, ayudándolos con las tareas y fomentando experiencias de aprendizaje interactivas. [10].
En este estudio, ChatGPT se utilizó en cuatro funciones clave en las fases de educación médica previa, en clase y posterior a la clase. Durante la fase de preparación previa a la clase, los estudiantes pudieron consultar ChatGPT sobre cualquier problema que encontraron, lo que facilitó una comprensión inicial de conceptos, terminología y casos médicos fundamentales. En un estudio, se pudo generar una serie de imágenes a partir de texto descriptivo utilizando un modelo de aprendizaje profundo basado en redes generativas adversarias. La herramienta se utiliza en el proceso narrativo visual para facilitar el aprendizaje mejorado por la tecnología y mejorar las habilidades de razonamiento lógico. [11]. Los modelos de aprendizaje profundo basados en redes generativas adversarias desempeñan un papel clave en la simulación de varios tipos de entornos de aprendizaje y ayudan a desarrollar habilidades prácticas en modelos de asistentes de enseñanza virtuales. Los resultados experimentales muestran que este modelo mejora el efecto de aprendizaje de los estudiantes y mejora su motivación y capacidad de aprendizaje. [12]. En el aula, se empleó ChatGPT para simular las interacciones con los pacientes, proporcionando una plataforma para que los estudiantes practiquen habilidades de diagnóstico y comunicación en un entorno seguro y controlado. En sus interacciones con ChatGPT, los estudiantes son libres de practicar habilidades de diagnóstico y comunicación sin los riesgos que podría representar un paciente real. Un diagnóstico falso o una falta de comunicación no tiene un impacto real en el paciente, lo que permite a los estudiantes aprender mediante prueba y error. ChatGPT está disponible y los estudiantes pueden practicar a su propio ritmo y necesidades de aprendizaje, sin depender de un tiempo y lugar específicos. Esta flexibilidad hace que el aprendizaje sea más eficiente y conveniente. ChatGPT puede simular una variedad de escenarios clínicos y características del paciente para brindar una experiencia interactiva diversa. Los estudiantes están expuestos a diferentes condiciones y antecedentes de pacientes, mejorando así su capacidad para afrontar situaciones complejas. Después de clase, los estudiantes pueden interactuar con ChatGPT individualmente o en grupos de estudio, discutiendo preguntas de práctica proporcionadas por la herramienta, abordando preguntas difíciles o desafiantes y explorando el material desde varias perspectivas. A lo largo del proceso interactivo, los estudiantes evaluaron continuamente su comprensión del material, identificaron sus debilidades y ajustaron sus estrategias de aprendizaje y áreas de enfoque de manera oportuna para enfocarse en áreas específicas para revisión y refuerzo, asegurando que se mantuvieran en el camino correcto. [13]. De manera similar, los instructores podrían utilizar ChatGPT para recopilar recursos didácticos y estudios de casos relevantes durante la fase de preparación de la lección. Al aprovechar ChatGPT, podrían mejorar la participación de los estudiantes en el aula y utilizar la herramienta después de clase para recopilar y analizar los comentarios de los estudiantes sobre el proceso de enseñanza. Además, los estudiantes podrían utilizar ChatGPT para resolver rápidamente cualquier confusión relacionada con el conocimiento profesional. Con la capacitación del modelo ChatGPT, los estudiantes de medicina y los médicos pueden mejorar su razonamiento clínico y sus habilidades de toma de decisiones, mejorando así el desempeño del análisis y diagnóstico de casos. Además, ChatGPT proporciona a los estudiantes de medicina una experiencia de aprendizaje personalizada y eficiente a través de conversaciones simuladas, tutorías inteligentes y preguntas y respuestas automatizadas, profundizando así la comprensión de los conocimientos médicos de los estudiantes. [14].
Los resultados de este estudio indican que las puntuaciones teóricas de los grupos de estudio fueron significativamente más altas que las de los grupos de control, lo que refleja mejores resultados de aprendizaje. No se observaron diferencias significativas en las puntuaciones entre los dos grupos de estudio ni entre los dos grupos de control. Esto sugiere que la aplicación de ChatGPT en los grupos de estudio resultó en una comprensión y dominio superiores del conocimiento teórico en comparación con los métodos de enseñanza tradicionales utilizados en los grupos de control.
Los resultados de satisfacción docente de este estudio indican que los estudiantes de los grupos de estudio que utilizaron ChatGPT informaron puntuaciones de satisfacción total significativamente más altas, así como mejores calificaciones en la organización del curso y los métodos de enseñanza, en comparación con los grupos de control. Las diferencias en la satisfacción con el contenido del curso y los instructores fueron relativamente menores, lo que sugiere que el uso de ChatGPT como ayuda didáctica, a través de su novedoso y atractivo formato interactivo de preguntas y respuestas, su fuerte interactividad y su enfoque estructurado, parece mejorar la participación de los estudiantes. y participación en el aprendizaje. Esto indica que ChatGPT puede fomentar eficazmente un mayor interés y promover resultados educativos. La diferencia más notable entre los métodos de enseñanza radica en la ejecución en el aula; La capacidad de ChatGPT para simular varios escenarios y realizar análisis de casos, combinada con el acceso a recursos didácticos adicionales, mejora significativamente las habilidades de aplicación clínica de los estudiantes de medicina.
La evaluación del estudio sobre el cumplimiento del aprendizaje abarcó cuatro aspectos. Los hallazgos indican que no hubo diferencias significativas entre los grupos en cuanto al establecimiento de planes de aprendizaje. Sin embargo, para los otros tres aspectos (preparación autónoma previa a la clase y revisión posterior a la clase, participación en la enseñanza en el aula y búsqueda de retroalimentación y asistencia), los grupos de estudio exhibieron calificaciones significativamente más altas en comparación con los grupos de control. En muchos estudios y análisis estadísticos, una “puntuación más alta” suele considerarse un resultado positivo, lo que significa que el grupo de estudio obtuvo mejores resultados en algo. Los indicadores de evaluación de este estudio son todos positivos y se puede considerar que una “puntuación más alta” indica un mejor desempeño del grupo de investigación, lo que es un resultado positivo. Esto sugiere que la incorporación de ChatGPT como ayuda didáctica mejora el cumplimiento del aprendizaje de los estudiantes al promover el aprendizaje activo, fomentar el aprendizaje basado en la investigación y mejorar su interés y capacidad para el aprendizaje autónomo.
Si bien las mejoras en el cumplimiento son evidentes, la profundización continua de la comprensión antes, durante y después de la clase también contribuye a mejorar el pensamiento lógico y las habilidades analíticas. En particular, el estudio encontró una tasa relativamente baja de preguntas y solicitudes de ayuda de los estudiantes, durante y después de clase. Las diferencias observadas entre los grupos de estudio y control pueden atribuirse a la capacidad de ChatGPT para ayudar a los estudiantes a superar la timidez y no juzgar los errores. La herramienta de inteligencia artificial ayuda a los estudiantes a superar las dudas, permitiéndoles hacer preguntas de forma libre y repetida sin temor a ser juzgados o interacciones negativas. Al generar materiales de aprendizaje basados en el estado de aprendizaje y las necesidades de cada estudiante, ChatGPT les permite adoptar un enfoque más autónomo del aprendizaje y tener una experiencia educativa adaptada a sus preferencias. Estas interacciones facilitan la aclaración oportuna, una comprensión más profunda y el dominio del material.
ChatGPT también puede adaptar planes y materiales de aprendizaje individualizados para cada estudiante para adaptarse a los diferentes estilos y habilidades de aprendizaje dentro del aula. Este enfoque personalizado fomenta un circuito de retroalimentación positiva, mejorando las capacidades de aprendizaje de los estudiantes.
La aplicación de ChatGPT en la educación médica sigue siendo un tema de considerable debate. Si bien ChatGPT ofrece funcionalidades innovadoras y ventajas potenciales, también plantea varias preocupaciones éticas y prácticas, el potencial de uso indebido, particularmente en los ámbitos de la educación y el mundo académico. [15]. Como chatbot, ChatGPT carece de la capacidad de pensar críticamente como un ser humano, lo que limita su capacidad para interpretar y analizar información médica más allá de sus algoritmos programados. No posee el juicio ni el discernimiento necesarios para los aspectos éticos o legales de la práctica médica y puede plantear riesgos relacionados con violaciones de datos y privacidad. [16, 17].
El auge de herramientas de inteligencia artificial como ChatGPT ha llevado a la deshonestidad académica, con informes de estudiantes que utilizan la tecnología para hacer trampa en sus trabajos de ensayo. [18]. Algunas investigaciones sugieren que ChatGPT puede no ser un recurso confiable para problemas complejos que requieren habilidades y conocimientos avanzados. [19]. Además, los académicos han estado preocupados por la confiabilidad de ChatGPT como fuente creíble de información. [20]. Según muchos educadores, ChatGPT puede ser utilizado fácilmente para hacer trampa por parte de estudiantes que toman cursos de comunicación y filosofía, pero es fácil de identificar. Una preocupación creciente es que los estudiantes eventualmente perderán la capacidad de generar ideas originales y no podrán presentar argumentos adecuados para demostrar un punto. [21]. La accesibilidad tecnológica es un desafío. El uso eficaz de ChatGPT depende de la conectividad de la red y la disponibilidad del dispositivo, lo que puede resultar problemático en diferentes regiones y entre poblaciones estudiantiles específicas. Se deben desarrollar políticas para utilizar ChatGPT en diferentes entornos técnicos. [22]. Una preocupación es la posible devaluación del aprendizaje cooperativo en la educación médica, particularmente en enfoques tradicionales como ABP, CBL y TBL. La colaboración y el trabajo en equipo son cruciales en estos enfoques, y ChatGPT puede reducir involuntariamente la importancia de las interacciones entre humanos. Mantener un equilibrio entre la tecnología y las relaciones es esencial para un aprendizaje eficaz. Si bien ChatGPT mejora el ABP mediante instrucción personalizada, los educadores deben enfatizar la importancia duradera del aprendizaje basado en el paciente y el trabajo en equipo. A pesar de las capacidades de simulación y los conocimientos teóricos de ChatGPT, no puede reemplazar la experiencia práctica obtenida a través de interacciones en el mundo real, especialmente en la educación médica. Reconocer las limitaciones de los modelos es esencial para evitar una dependencia excesiva del aprendizaje por simulación. Integrar perfectamente ChatGPT en los planes de estudio existentes es un desafío que requiere que los educadores inviertan tiempo en diseñar e integrar componentes impulsados por IA que se alineen con los objetivos generales de aprendizaje. [23]. Dadas estas consideraciones, es esencial utilizar ChatGPT con prudencia como herramienta auxiliar de aprendizaje, complementando en lugar de reemplazar los métodos educativos y las técnicas de investigación tradicionales, y siendo consciente de las limitaciones de ChatGPT.
-
Startups7 meses ago
Remove.bg: La Revolución en la Edición de Imágenes que Debes Conocer
-
Recursos8 meses ago
Cómo Empezar con Popai.pro: Tu Espacio Personal de IA – Guía Completa, Instalación, Versiones y Precios
-
Recursos8 meses ago
Suno.com: La Revolución en la Creación Musical con Inteligencia Artificial
-
Recursos7 meses ago
Perplexity aplicado al Marketing Digital y Estrategias SEO
-
Estudiar IA7 meses ago
Curso de Inteligencia Artificial de UC Berkeley estratégico para negocios
-
Tutoriales8 meses ago
Cómo Comenzar a Utilizar ChatGPT: Una Guía Completa para Principiantes
-
Eventos8 meses ago
La nueva era de la inteligencia artificial por el Washington Post – Mayo 2024
-
Noticias6 meses ago
Dos periodistas octogenarios deman a ChatGPT por robar su trabajo