Noticias
OpenAI Researcher Dan Roberts on What Physics Can Teach Us About AI
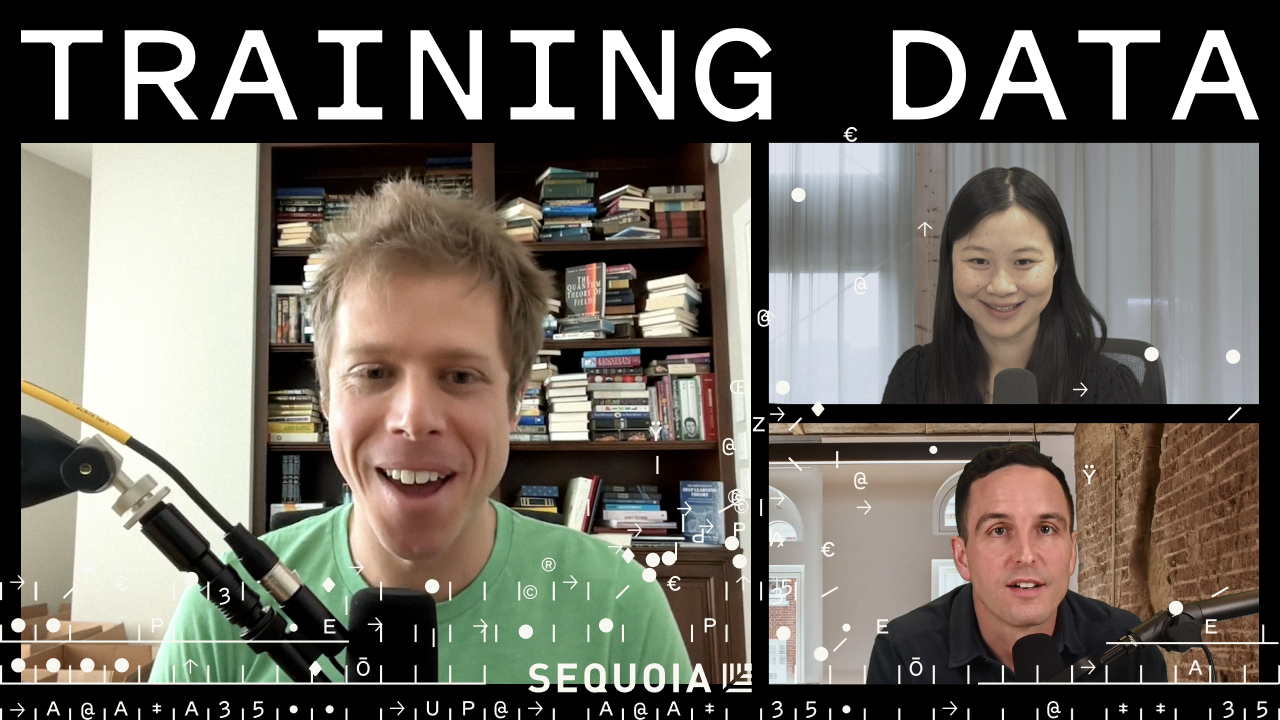
Contents
Dan Roberts: In the 40s, the physicists went to the Manhattan Project. Even if they were doing other things, that was, that was the place to be. And so now AI is the same thing and, you know, basically OpenAI is that place. So maybe, maybe we don’t need a public sector organized Manhattan Project, but it, you know, it can be OpenAI.
Sonya Huang: Joining us for this episode is Dan Roberts, a former Sequoia AI Fellow who recently joined OpenAI as a researcher. This episode was recorded on Dan’s second-to-last day at Sequoia, before he knew that he would go on to become a core contributor to o1, also known as Strawberry.
Dan is a quantum physicist who did undergraduate research on invisibility cloaks before getting a PhD at MIT and doing his postdoc at the legendary Princeton IAS. Dan has researched and written extensively about the intersection of physics and AI.
There are two main things we hope to learn from Dan in this episode. First, what can physics teach us about AI? What are the physical limits on an intelligence explosion and the limits of scaling laws, and how can we ever hope to understand neural nets? And second, what can AI teach us about physics and math and how the world works?
Thank you so much for joining us today, Dan.
The path to physics
Dan Roberts: Thanks. Delighted to be here on my, probably, second-to-last day at Sequoia. Depending on when this airs and how you’re going to talk about it.
Pat Grady: You will always be part of the Sequoia family, Dan.
Dan Roberts: Thanks, I appreciate it.
Sonya Huang: Maybe just to get started tell us a little bit about “Who is Dan?” You have a fascinating backstory. I think you worked on invisibility cloaks back in college. Like, what led you to become a theoretical physicist in the first place?
Dan Roberts: Yeah, I think–and this is, you know, my stock answer at this point, but I think it’s true–I was just an annoying three year old who never grew up. I asked “why” all the time. Curious. How does everything work? I have a 19-month at home right now, and I can see the way he followed the washing machine repairman around and had to look inside the washing machine. So like, I think I just kept that going. And when you’re more quantitatively oriented than not, rather than going to philosophy I think you sort of veer into physics. And that was sort of what interested me. How does the world work? What is all this other stuff that’s out there?
The question that you didn’t ask, but maybe I’ll just answer it ahead of time–the sort of inward facing stuff felt less quantitative and more in the realm of the humanities. Like, so “What is all this stuff?” That’s pretty physics-y. “What am I? Who am I? What does it mean to be me?” That felt not very science-y at all. But with AI it sort of seems like we can think about both “What does it mean to be intelligent,” and also “What is all this other stuff?” in some of the same frameworks. And so that’s been very exciting for me.
Sonya Huang: So we should be trying to recruit your 19-month-old right now is what you’re saying?
Dan Roberts: Oh yeah. Absolutely. He grew out of one of his Sequoia onesies, but he fits into a Sequoia toddler t-shirt now. And so he definitely is ready to be a future founder.
Sonya Huang: I guess, at what point did you know that you wanted to think about AI? At what point did that switch start to flip?
Dan Roberts: Yeah. So, I think like many people, when I discovered computers, I wanted to understand how they worked and how to program them. In undergrad, I took an AI class and it was very much good old fashioned AI. A lot of the ideas in that class actually are coming back to be relevant, but at the time it was, it seemed not very practical. It was a lot of, you know, “If this happens, do that.” And there was also some game-playing there. That was sort of interesting. It was very algorithmic, but it didn’t seem related to what it means to be intelligent.
What does it mean to be intelligent?
Pat Grady: Can we just pick up on that real quick? Like, what do you think it means to be intelligent?
Dan Roberts: That’s a great question.
Pat Grady: I can see wheels turning.
Dan Roberts: Yeah. Well, this is one of those questions where I don’t have a stock answer, but I think it’s important to not say nonsense. One of the things that’s exciting to me about AI is the ability to have systems that do what humans do, and to be able to understand them from “What are the lines of Python that cause that system to do something?” to, you know, to trace that through and understand what are the outputs, and you know, how the system can like, see and classify what it means. You know, “What is a cat? What is not a cat?” Or can write poetry and, you know, it’s just a few lines of code. Whereas, if you’re trying to study humans and ask, “What does it mean for humans to be intelligent?” you have to go from biology, through neuroscience, through psychology, up to other higher level versions of ways of approaching this question.
And so, I think maybe a nice answer for intelligence, at least the things that are interesting to me, is “The things that humans do.” And now, if you pull back the answer that I just said a second ago, that’s how I connect it to AI. AI is taking pieces of what humans do, and we’re understanding, at least an example that’s kind of simple and easy to study, that we might use to understand better what what it is that humans do.
The path to AI
Sonya Huang: So Dan, you mentioned when you were, you know, studying AI in college, there was a lot of what sounds like kind of hard-coded logic and brute force type approaches. Was there a moment that kind of clicked for you, of like, “Oh, okay. This is different”? Was there a key result or a moment where it was like, “Okay, we’re going bigger places than kind of the ‘if this, then that’ logic of the past”?
Dan Roberts: Yeah, actually it didn’t click. I sort of wrote it off. And it would be great if there was a separation between like ten years between then and the next thing that I’m going to say, but actually the writing-off maybe lasted a year or two, because then I went to the UK for the first part of grad school. I spent a very long time in grad school and I discovered machine learning and a more statistical approach to artificial intelligence, where you have a bunch of examples of large amounts of data. Or at the time, maybe we wouldn’t have said large amounts of data, but we would have said, you have data examples of a task that you want to perform. And there’s different ways that machine learning works, but you write down a very flexible algorithm that can adapt to these examples and start to perform in a way similar to the examples that you have.
And that approach borrows a lot from physics. It also, at the time, I started… So graduated college in 2009. Discovered machine learning in 2010, 2011. 2012 is the big year for deep learning. And so, you know, there’s not a big separation here between write-off and rediscovery. But I think–and machine learning clearly existed in 2009, it just wasn’t related to the class that I took–but this approach made a lot of sense to me. And it started to have, you know, I got lucky, and then it started to have real progress and seemed to fit in a framework that I understood scientifically. And I got very excited about it.
Sonya Huang: Why do you think there are so many people who come from a similar background or similar path to you? Like, a lot of ex-physicists working on AI. Like, is that a coincidence? Is that herd behavior, or do you think there’s something that makes physicists particularly well suited to understanding AI?
Dan Roberts: I think the answer is yes to all the ways you…
Sonya Huang: All the above.
Dan Roberts: You know, physicists infiltrate lots of different subjects, and we get parodied about the way that we go about trying to use our hammers to tackle these things that may or may not be nails. Throughout history, there are a lot of times that physicists have contributed to things that look like machine learning. I think in the near term, the path that physicists used to take when they didn’t remain in academia often was going into quantitative finance, then data science. And I think machine learning, and its realization in industry, was exciting because, again, it’s something that feels a lot like actual physics and is working towards a problem that’s very interesting and exciting for a lot of people.
You know, you’re doing this podcast because you’re excited about AI. Everyone’s excited about AI. And in many ways it’s a research problem that feels a lot like the physics that people were doing. But I think the methods of physics actually are different from the methods of traditional computer science and very well suited for studying large scale machine learning that we use to work on AI.
Traditionally physics involves a lot of interplay between theory and experiment. You come up with some sort of model that you have some sort of theoretical intuition about. Then you go and do a bunch of experiments and you validate that model or not. And then there’s this tight feedback loop between collecting data, coming up with theories, coming up with toy models. Understanding moves forward by that. And, you know, we get these really nice explanatory theories. And I think the way that big deep learning systems work, you have this tight feedback loop where you can do a number of experiments. The sort of math that we use is very well suited to the math that a lot of physicists are familiar with. And so I think it’s very natural for a lot of physicists to work on this. And those tools, a number of them differ from the traditional, at least theoretical, computer science and theoretical machine learning tools for studying the theoretical side of machine learning, and maybe also differentiation between just being an awesome engineer and also being a scientist. And there’s tools from doing science that are helpful in studying these systems.
Pat Grady: Dan, you wrote this, what I thought was a beautiful article, Black Holes and the Intelligence Explosion, and in there you talk about this concept of, sort of the microscopic point of view, and then the system level point of view and how physics really equips people to think about the system level point of view, and that has a sort of complimentary benefit to understanding these systems. Can you just take a minute and kind of explain sort of microscopic versus system level, and how the physics influence helps to understand the system level?
Dan Roberts: Sure. So, let me start with an analogy that I think is, like, very, you know, goes even further than an analogy. But going back, what year is it? Maybe like 200 years or so around the time of the Industrial Revolution, there was steam engines and steam power and a lot of technology that resulted from this and ultimately powered industrialization. And in the beginning, there was a lot of engineering of these steam engines, you know, and there was this high level theory of how this work called thermodynamics, where, you know, and I imagine everyone’s seen this in high school, perhaps where, you know, there’s the Ideal Gas Law that tells you that there’s some relationship between pressure and volume and temperature. And, you know, these are very macro level things. Like you can buy a thermometer. You can also measure the volume of your room and you can buy a barometer as well. Maybe people don’t or look up on the weather report. But, you know, these are like measurements that we use and we talk about. But then underlying this, and it took us a bit later to validate this and understand it, there’s the notion of atoms and molecules, the air molecules bouncing around. And somehow we now understand that those air molecules give rise to things like temperature and pressure and volume. I guess it is easier to understand that the gases, the molecules, are confined to a room.
But there’s a precise way in which you can start with a statistical understanding of those molecules and derive thermodynamics, like, derive the Ideal Gas Law from it. And you can go further than that to derive that it’s ideal because it’s wrong. It’s just a toy model. But you can, there are corrections to it, and you can sort of understand, you know, from the microscopic perspective, which is the molecules, which we don’t really interact with. You know, we don’t see them, we don’t interact with them on a day-to-day basis, but their statistical aggregate properties give rise to, sort of, this behavior that we do see at the macro scale.
And part of, to get to your question, you know, I think there’s a similar thing going on with deep learning systems. And I wrote a book with Sho Yaida and Boris Hanin on how to apply these sorts of ideas to deep learning, and, at least in an initial framework that allows you to start doing this in an initial way. And to answer your question, the sort of micro perspective is you have neurons and weights and biases, and we can talk about in detail how that works, but when people think of the architecture, there’s some very specific, some people say circuits, there’s specific ways in which these things, you know, there’s an input signal which might be an image or text, and then there’s many parameters. And, you know, it’s very simple to write down. It’s not that many lines of code even taking into account the machine learning libraries, but it’s, you know, it’s like a very simple set of equations. But there’s a lot of weights. There’s a lot of numbers that you have to know in order to get it to do something. And that’s sort of the micro… that’s like the molecules perspective.
And then there’s like the macro perspective, which is, well, what did it do? Did it produce a poem? Did it solve a math problem? How does, how do we go from that, those weights and biases to, to that macro perspective? And so for statistical physics to thermodynamics, we understand that completely. And you can imagine trying to do the same sort of thing, literally applying the same sorts of methods to understand how does the underlying micro statistical behavior of these models lead to the sort of macro or, as you said, system level perspective?
Sonya Huang: Dan, maybe speaking of scaling laws, and I think you were at our event, AI Ascent, Andre Karpathy mentioned that current AI systems are like 5 or 6 orders of magnitude off in efficiency compared to biological neural nets. How do you think about that? Do you think scaling laws get us there? Just a combination of scaling laws plus hardware getting more efficient or, like, do you think that there’s kind of big step function leaps that need to come in research?
Dan Roberts: There’s maybe two things that could be meant here. One is that the way humans seem to work at a similar scale to AI systems is much more efficient. You know, we don’t need to see trillions of tokens before we speak. You know, my toddler is already starting to speak in sentences. And he’s been exposed to far less tokens than a typical large language model. And so there’s some sort of disconnect between human efficiency at learning and what large language models do. Of course, they’re very different systems. They’re designed, you know, the way in which they learn is right now very different. And so in some sense that’s to be expected. So, there’s this gap here that you could imagine bridging.
There’s another thing that I think is not what you meant, but I think is sort of the thing to answer about with respect to scaling laws, which is, and I talked about this a bit in the article, but lots of people seem to talk about this, which is “What is the final GPT?” You know, there’s GPT-4 right now, and it could be other companies as well, but since I’m going to join OpenAI let me represent my new company, right? So is it going to be six? Is it going to be seven? At some point, assuming we have to scale things up, there are things that are going to break. Whether they’re economic, we’re going to run out of, you know, we’re going to try to train a model that’s larger than the world’s GDP or GWP, however, the D works for the world. Or we’re going to run out of, you know, we’re not going to be able to produce enough GPUs. Or we’re not going to be able to put, you know, it’s going to cover the surface of the Earth. You know, a lot of these things are going to break down at some point. And so probably the economic one happens first. So how many, you know, how many more iterations do we get before we run out of actually being able to scale practically? And where does that get us? And then, I think, to tie those two perspectives together, there’s scaling on its own. And of course, it’s impossible to disentangle this because people are making things more efficient.
But you know, there’s like, you could imagine there’s the, take literally what GPT-2 was, which was the initial big model, and keep scaling it up. Is that going to get us to some, you know, super different, exciting, economically powerful, or however you want to define what the end state of AI research and AI startups and AI in industry is? Or do we need lots of new exciting ideas? And, and again, of course, you can’t really disentangle these, but I think the general scaling hypothesis is that it’s just the scaling and it’s not the ideas that matter. Whereas, the “How do we get to be efficient like humans?” I think requires, like, non-trivial ideas. And to answer your question, the reason I’m excited about joining OpenAI is that I think there is high leverage to be had in the ideas, you know, in going beyond scaling, and that we will need that in order to get to the next steps. I have no idea what I’ll be working on, but when this airs I guess I will know what I’m working on, but you know that that’s what’s really exciting to me.
The pendulum between scale and ideas
Pat Grady: Dan, is there almost like a pendulum that swings back and forth between scale and ideas in terms of how people apply their efforts in the world of AI? Like, transformers came out. Great idea. Since then, we’ve largely been in this race to scale. It feels like things are starting to asymptote for a bunch of practical reasons that you mentioned. Is the pendulum swinging back toward ideas as the currency? You know, it’s less now about who can, you know, have the biggest GPU cluster and more about finding new architectural breakthroughs, whether that’s, you know, reasoning or something else?
Dan Roberts: Yeah. That’s a really great question. I think there’s this article by Richard Sutton called The Bitter Lesson, not the bitter pill, and it basically gives the argument that ideas aren’t important, that scale is what you need, and that all the ideas are always trumped by scaling, by scaling things up. It says a bunch of things, but maybe that’s a high level takeaway. And, you know, there’s a sense of this where there are a lot of interesting ideas that came out in the 80s and 90s that people didn’t really have scale to explore. And then, I remember when, after AlphaGo and DeepMind was writing a lot of papers, people were rediscovering those papers and re-implementing them in deep learning systems. But this was sort of still before people realized, “No, the thing that you need to do is scale up.” And even now, with transformers, people are exploring other architectures or even simpler architectures that we knew before that seem to be able to, you know, there’s a notion, you know, maybe scaling laws don’t come from as long as the architecture isn’t sick in some way.
They come from sort of the underlying data process and having large amounts of data rather than from having a special idea. I think the real answer is that there’s a balance between the two. That scale is hugely important, and maybe it was just not understood how important. And we also didn’t have the resources to to scale things up at various times, you know, the things that have to go into producing these GPU clusters that are producing these models are, you know, you guys know this as well, like there’s a lot of parts along the supply chain, or along the product chain, whatever you actually call it, in order to make those things happen and to deploy them. And even, you know, the way GPUs were originally, they’ve now co-evolved to be well suited for these models. And the reason, in some sense, you can think of transformers was a good idea was because it was designed to be well-suited to train on the systems that we had at the time. And so sure, these other architectures could do it at an ideal scientific level, but at a practical level, it was important to to get something that was that that was able to to reach that scale.
So I think, you know, if you brought in ideas to be that sort of thing that’s married with scale in some way, then I still think ultimately, like, you know, someone came up with the idea of deep learning. That was an important idea. You know, there’s Pitts and McCulloch came up with the original idea for the neuron. Rosenblatt came up with the original perceptron. And there’s like a lot of people, from going back like 80 or so years, of people just making important discoveries that were ideas that contribute. So, I think it’s both. But it’s easy to see how, you know, if you’re bottlenecked, people think about ideas. And then if you unlock a new capacity of scale somehow, then you just see a huge set of results. And it seems like scale is super important. And I really think it’s more of a synergy between the two.
The Manhattan Project of AI
Sonya Huang: Maybe on the topic of the race to scale, Dan, you mentioned kind of just the economic constraints and realities, which I guess are more, like, practically a ceiling in the private sector. You also mentioned the Manhattan Project earlier in terms of things that physicists have been involved with. Like, do you think we need a Manhattan Project style thing for AI? Like at the nation state or at the international level?
Dan Roberts: Well, one thing I can say is that part of the process that led me to OpenAI is I was talking with your partner, Shaun Maguire, who brought me to Sequoia in the first place, and trying to figure out is there a startup that makes sense for me to work on that has the right mix of, sort of, scientific questions, research questions, and also as a business? And I think it was Shaun that said–and I don’t mean the analogy in terms of the impact of, you know, in terms of like negative impact of what you might think of the Manhattan Project, but just in terms of the scale and the organization–he said, “You know, in the 40s, the physicists’ physicist went to the Manhattan Project. Even if they were doing other things, that was the place to be.” And so now AI is the same thing, and basically said OpenAI is that place. So maybe, maybe we don’t need a public sector organized Manhattan Project, but it, you know, it can be OpenAI.
Sonya Huang: OpenAI as the Manhattan Project. I love that.
Dan Roberts: Well, maybe that’s not a direct quote that we want to be taken out of context, but I think in terms of…
Sonya Huang: The metaphorical Manhattan Project.
Dan Roberts:Yeah. In terms of scale and ambition. I mean, I think a lot of physicists would love to work at OpenAI for a lot of the same reasons that they probably were excited to… Well, okay, there’s a number of different reasons. Maybe we just have to leave it as a nuanced thing rather than making broad claims.
AI’s black box
Sonya Huang: Can we talk a little bit about this… Like, can we ever understand AI, especially as we go to these deep neural nets, or do you think it’s a hopeless black box, so to speak?
Dan Roberts: Yeah, I think within the… This is my answer to the “What are you a contrarian about?” Although maybe, you know, on the internet everyone takes every side of every position, so it’s hard to say you have a contrarian position. But I think within AI communities, you know, I think my contrarian position is that we can really understand these systems. And in, you know, physics systems are extremely complicated, and we have made a huge amount of progress in understanding them. I think these systems sit in the same framework. And, you know, another principle that Sho and I talk about in our book, and that’s a principle of physics, is that there’s this often extreme simplicity at very large scales, basically due to the statistical averaging, or more technically, the central limit theorem. Things can simplify–and I’m not saying this is what happens exactly in large language models, Of course not–but I do think that we can apply sort of the methods that we have and also, you know, maybe hopefully have AI that can help us do this in the future. And by AI, I mean tools. Not like individual intelligences just going running on their own and solving these problems. But I guess I feel at the extreme end that this is not going to be an art, that the science will will catch up and that it will will be able to make extreme leaps in really understanding how these systems work and behave.
What can AI teach us about physics?
Pat Grady: So, Dan, we’ve talked a bunch about what physics can teach us about AI. Can we talk a bit about what AI can teach us about physics? Are you optimistic about domains like physics and math and how these emerging models can, you know, probe further into those domains?
Dan Roberts: Yes, I’m definitely optimistic. I guess my perspective is that math will be easier than physics, which maybe betrays the fact that I’m a physicist, not a mathematician. And I’ll say–I can give explain why I think that in a second–but, you know, I still have a lot of friends that work in physics and they, you know, there’s like a growing sense and maybe even approaching a dread, that maybe this is actually the answer to “Why do physicists work on AI?” Because, you know, if what you care about is the answer to your physics question and you want to make it happen as soon as possible, what is the highest leverage thing you can do? Maybe it’s not work on the physics question you care about, but it’s work on AI to make the, you know, because you think that the AI might end up solving those questions very rapidly anyway. And I don’t know the extent that anyone really takes it seriously, but I think within the theoretical physics community that I come from, that this is sort of a thing that somewhat gets thrown around and discussed.
I think maybe to give a more object-level answer, I think what’s exciting about math–and maybe when you have Noam Brown on, if you have him on, he’ll talk about this, but this is something that that he’s talked about for a while before he joined OpenAI–I think the that we have, you know, we made a lot of progress in terms of solving games by doing more than just looking up what is the strategy that we should use to play the games, but also being able to simulate forward and, you know, the way that if I’m in a very hard position in a particular game, rather than just playing with intuition, I might sit and think about what I should do. Yeah, sometimes this goes under the name inference-time compute rather than raining-time compute or pre-training. And you know, there’s a sense in which what it means to do reasoning is very related to this ability to sit and think. So we know how to do it for games because there’s a very clear winning and loss signal. So you can simulate ahead and sort of figure out what it means to do good or not. And I think math, in some parts of math–again, I’m not a mathematician and well, you know, I’m always scared about talking about math publicly and saying something wrong that will upset mathematicians–but it seems like certain types of math problems are not as constrained as games, but are still constrained enough where there’s a notion of like finding a proof, right? You know, there’s definite problems in terms of search, in terms of how do you figure out what is the next, you know, move in the proof, but the fact that we might call it a move suggests that there’s things in math that feel a lot like games. And so we might think the fact that we can do well at games maybe means that we can do well at certain types of mathematical discovery.
Can AI help solve physics’ biggest problems?
Pat Grady: Well, I was going to say since you mentioned Noam, he likes to use the example with test-time compute of whether it could help to prove the Riemann hypothesis. Is there a similar problem or hypothesis in the world of physics that you are optimistic AI can help to solve sometime in our lifetimes?
Dan Roberts: Yeah. So, I mean, there’s a Millennium Problem relating to physics–and if I try to remember exactly what it is I’m sure I will butcher it and then no one will believe that I’m actually a physicist–but it’s related to the, you know, it’s a mathematical physics question related to the Yang-Mills mass gap. But I think, what I wanted to say is that I think some of the flavor of what physicists care about and doing physics feels a little different. This is where I might get in trouble. It feels a little different than some of, like, the mathematical proof type things. Physicists are known to be more informal and, you know, hand-wavy. But also, on the other hand, connected to, in some sense, connected to the interplay between experiment and the sort of models that physicists study is maybe what saves them is that, you know, they have things that are informal and hand-wavy, but very explanatory. And then the mathematicians, it’s like we were saying earlier, the engineers discovered all the exciting industrial machines, and the physicists maybe cleaned up a bunch of the theory about how that works. And then the mathematicians come later and clean up, like, formalize everything and clean it up even more. And so there’s a mathematician or mathematical physicist that cleans up a lot of, you know, make proper and try and, you know, understand in formal ways some of the stuff that physicists do.
But rather than talking about–I mean, I think the key point there is that the sort of questions that are interesting to physicists maybe don’t look like proofs, and maybe it’s not about how do we, given a particular model, how do we actually solve it? Like once things are set up correctly, like it’s often, you know, senior or you know, people that are trained in the field are able to sort of figure out how to analyze those systems. It’s more the other stuff. Like, what is the right model to study? Does it capture the right sort of problems? Does this relate to the thing that you care about? What are the insights you can draw from it? And so for AI to help there, I think it would look different than the way we’re sort of trying to build AI systems for math. So rather than here’s, you know, here’s the word problem going, you know, solve this high school level problem or, you know, prove the Riemann hypothesis.
It’s like, you know, the questions in physics are like, “What is quantum gravity? What happens when something goes into a black hole?” And that’s not like, you know, start generating tokens on that. What does that even look like? And you know, if you go to a physics department, you know, people hang out at the blackboard. They chat about things. Like, maybe they sketch mathematical things, but you know, there’s a lot of other things that go into this. And so maybe the sort of data that you need to collect looks more like that. Or maybe it looks like the random emails and conversations on Slack and the scratch work. And so, I mean, there are definitely tools that we can use, like “Help me understand this new paper so I don’t have to spend two weeks trying to study it and understand it.” You know, maybe let me ask questions about it. I think there are problems with the way that’s currently implemented.
But, you know, I think there are a lot of tools that will help accelerate physicists just like Mathematica, which is a software package that does integrals, and it does a lot more than that. Sorry, Stephen Wolfram. But I use it to do integrals and, you know, sometimes it doesn’t know integrals and you can look them up in like these integral tables. Anyway. You know, I think, you know, and this applies to other branches of science too. Like, I think the ways in which the questions are asked and what it means to do science in different fields, maybe can look further and further from gains, let’s just say. And so to the extent that that’s true, I think we’ll need to. And not even clear that we’ll need lots of ideas or I mean, we we will need lots of ideas, but it’s more just like, we’ll have to, I think, we’ll just have to approach them all differently. And maybe not, like maybe eventually we’ll have a universal thing that knows how to do all of it. But initially, like at least to me, a lot of these things feel a little different from each other.
Sonya Huang: You’ll have a front row seat to it, in part because you’re also on the prize committee for the AI Math Olympiad, which is something I’m personally super interested in.
Maybe to your last point on, kind of like, maybe eventually this stuff generalizes. Like, why do you think people are so focused on solving the hardest problems today, like physics, math? Those were the subjects that everyone was terrified of in school, right? Where it feels like there’s a lot more other domains that are also unsolved for now. Like do you think, do you think going for the hardest domains first kind of lets you get towards a generalized intelligence? Like how does solving these different domains kind of fit together in the grander puzzle?
Dan Roberts: Yeah. The first thing that comes to mind when you said that is to just push back and say, “Well, it’s not hard. These are the easy domains.” I mean, I’m bad at biology. It doesn’t make any sense to me at all. My girlfriend actually is in bioengineering and is in biotech. And so, like, what she does just makes no sense to me. I can’t understand any of it, where physics makes complete sense to me. I think maybe a better answer or a less glib answer is that, like I was trying to say about math, there are constraints. And, you know, in particular with math, a lot of it is unembodied. You don’t have to go and do experiments in the real world. You know, they’re sort of self-sufficient and that’s close to, like, what generating text, like the way language models work, or even the way some reinforcement learning systems work for games. And so, I think the further that you go from that, the messier things become, the harder it probably is, and also the harder it is to get the right kind of data to train these systems.
If you want to build an AI–and people are trying to do this, but it seems difficult–if you want to build an AI system that solves biology, I guess you need to also make sure robotics works, you know, so that it can do those sorts of experiments and like it has to understand that sort of data. Or maybe it has humans do it. But, you know, there’s a lot, you know, for a self-sustaining AI biologist, it seems like there’s a lot of things that are going to go into it. I mean, on the way, we’ll have things like AlphaFold 3, which just came out and which I didn’t get a chance to read the details of, but, you know, I saw that they’re trying to use it for drug discovery. And so, you know, I think each of these fields will have things developed along the way. But I think the less constraints there are and the sort of messier and more embodied it is, the harder it will be to accomplish.
Sonya Huang: No, that makes sense. So, like hard for a human is not the same thing–doesn’t correlate to hard for machine.
Dan Roberts: Yeah. Plus, also maybe humans disagree about what’s hard or not.
Sonya Huang: Some of us think more like machines, I guess. And then I guess the second question was like, do you think it all coalesces into, like, one big model that understands everything? Because right now it seems like there’s a lot of domain-specific problem solving that’s happening.
Dan Roberts: Yeah. I mean, the way things are going, it seems like the answers should be yes. It’s really dangerous to speculate in this field because everything you say is wrong. Usually much sooner than later.
Sonya Huang: Good thing we’re on record. We’ll hold you to it.
Dan Roberts: Yeah, exactly. But also, like, what does it mean to be different? You know, like, there’s a trivial way to make both things–make the question meaningless–by, like you say, the model is the union of all those other models. But there’s also a sense in which mixture of experts was originally meant to be that, it’s not that in practice at all. But, you know, there is a sliding scale here. But, you know, it does seem like people, at least the big labs, are going for the one big model and have a belief that that’s, you know, well… I don’t know, but maybe I will in the future, understand what the philosophy is there.
Lightning round
Pat Grady: Dan, we have a handful of more general questions to kind of close things out here. So I’ll start with the high level one. If we think kind of short-term, medium-term, long-term and call it, you know, five months, five years, five decades, what are you most excited or optimistic about in the world of AI?
Dan Roberts: Five years ago was after the transformer model came out, but it was around maybe when GPT-2 came out. So it seems like, you know, for the last five years we’ve been doing scaling. I imagine within the next five years, we’ll see that scaling will terminate. And maybe it will terminate in, you know, a utopia of some kind, you know, that people are excited about where we’re all post-economic and so forth, and we’ll have to shut down all your funds and, you know, return monopoly money because money won’t matter. Or we’ll see that we need lots of ideas. Maybe there will be another AI winter.
I imagine that–and again, scary to really speculate–but I imagine, like something will be something will be interestingly different within five years about AI. And it might just be that AI is over and we’re on to the, you know, we’re on to the next exciting investment opportunity. And, you know, everyone else will shift elsewhere. And you know, I’m not saying that. That’s not what’s motivating me about AI, but, you know. So maybe five years is enough time to see that. And I think in, in one year, I mean, or there’s a five. I messed this up. Whatever. Maybe it was five months, I don’t remember. I’m sorry.
Pat Grady: It’s five months. It’s okay, it’s okay. These are approximations. I know you said physicists are very hand-wavy. Venture capitalists are very hand-wavy. These are approximations.
Dan Roberts: In physics there’s–I like to joke that there’s like three numbers. There’s zero, one and infinity. And, you know, those are the only numbers that matter. You know, things are either arbitrarily small, arbitrarily large, or about order one.
So. Okay. Good. Thanks for reminding me. But yeah, for five months, I mean, I’m excited to well, to learn what’s exciting at the forefront of a huge research lab like OpenAI. And I think one thing that will be interesting will be the delta between the next generation of models, right? Because there’s ways in which things are scaling up in terms of, it’s not really public, I guess, aside from Meta, but in terms of size of data, size of models. And we see scaling laws that relate to, you know, something like the loss and, you know, it’s hard to translate that into actual capabilities. And so what will it feel like to talk to the next generation model? What will it look like? Will it have a huge economic impact or not? And I think I, you know, in terms of estimating velocity, right, you need a few points. You can’t just have one point. And we sort of have, you know, we’re starting to have that with GPT-3 to GPT-4. .But, you know, I feel like with the next delta, we’ll get to really see what the velocity looks like and what it feels like going from model to model to model. And maybe I’ll be able to make a better prediction in five months from now, but then I guess I probably won’t be able to tell it to you guys.
Sonya Huang: Thanks, Dan. One thing that stood out to me is just like your writing is so accessible and light and funny, and that’s not what I’m used to when I read super technical stuff. Like, do you think all technical writing should be informal and funny? Like, is that deliberate?
Dan Roberts: It’s definitely deliberate. It goes into, I think in some sense it’s inherited. I mean, I definitely am a not serious person, but I also think it’s an inherited, sort of, from the style of the field that I came from. But I’ll tell you a story. I was at lunch. I was a postdoc at the Institute for Advanced Study in Princeton, and I was having lunch and joking around with this professor, Nati Seiberg, who’s a professor at the institute. And then we got into, I think we were talking about someone, someone asked a question about, like, “What is a good title?” And I was like, “Oh, the title has to be a joke.” And he was on board with that. And then I was explaining that for me, the reason to write a paper is for the jokes. Like you have a bunch of jokes in mind, and then you want people to read those jokes. And so you have to package it into the science product, and people want to read the science product, and they’re forced to suffer through the jokes. And Nati, who is this Israeli professor, he was like, “I don’t get it. Why? Why can’t you just do the science? Why do you need the, like… the jokes are great too, but like, you should write for the science, not for the jokes.”
And I was adamant that I write for the jokes. But I think it’s, I think it’s what you said that at some point, you know, you learn about the scientific method and, you know, the, the formal ways of doing things, and you learn all these rules and then you grow up a bit. Or maybe, I had a roommate who was a linguist, he’s now a professor of linguistics at UT Austin, and like, he emphasized that you can–he would tell me which rules that I could break or what, like where the rules come from and why they’re important or not. And you sort of realize that you can break these rules. And, like, the ultimate goal should be, is the reader going to read it and understand it and enjoy it. So you don’t want to do things that compromise their ability to read and understand. But you don’t want to obscure things. You want to make it, you know, if it’s more enjoyable, people are more likely to read it and take the point. It’s also more fun if you’re writing it. So I think that’s where that comes from.
Sonya Huang: Dan, thank you so much for joining us today. We learned a lot. We enjoyed your jokes and I hope you have. I hope you have a wonderful second-to-last day at Sequoia. Thank you for spending part of it with us. We really appreciate it.
Dan Roberts: Thanks. I was absolutely delighted to be here chatting with you guys. This was wonderful.
Noticias
13 sencillos consejos y trucos de Google Gemini para lograr la máxima productividad

Mucho más que un simple chatbot talentoso, Google Gemini ofrece funcionalidad de IA multimodal y una interactividad potente e indolora. El nivel más alto de Google One, uno de los mejores servicios de almacenamiento en la nube de la actualidad, incluye convenientemente acceso a su versión más potente.
Emitir indicaciones sencillas y generar texto escrito competente resulta fácil para los nuevos usuarios de Gemini, pero su conjunto de herramientas en constante expansión proporciona impresionantes mejoras en la productividad. Estas son algunas de las formas más útiles de utilizar el agente de inteligencia artificial de Google en constante mejora para ahorrar tiempo y aumentar el rendimiento.
Relacionado
Primeros pasos con Google Gemini: conceptos básicos del último asistente de Android
Si quieres probar Géminis, estos consejos y trucos te ayudarán
13 Actualizar la gestión del tiempo y las rutinas de autocuidado
Aprenda hábitos eficientes y prácticas efectivas.
Alimentar a Géminis con una lista completa de tareas pendientes, completa con tareas recurrentes y actividades únicas, puede generar un cronograma conciso que priorice su rutina personal, las próximas fechas límite importantes o los nuevos hábitos que está tratando de adquirir. Alternativamente, brinde al agente de IA un resumen detallado de cómo pasó los últimos días, y podrá brindarle consejos sobre cómo optimizar su agenda para que todo se ajuste más cómodamente.
En su vida profesional, delinear las necesidades y los recursos de un proyecto le permite a Gemini analizar las formas más rentables y rentables de lograr sus objetivos. Después de todo, nada ayuda más a desarrollar la eficiencia de una máquina que preguntarle a una máquina.
12 Obtenga esquemas sencillos de videos de YouTube
No pierdas el tiempo sentado viendo vídeos extendidos
A veces, los creadores de información se van por la tangente y los expertos educados se vuelven prolijos. En lugar de esperar vídeos largos para ir al grano, o intentar mantener todos los elementos en orden en tu cabeza, pídele a Gemini un resumen. Puede seleccionar los puntos más importantes a lo largo de una producción y presentarlos en un formato fácil de leer.
Funciona especialmente bien con vídeos instructivos, como tutoriales de reparación y cocina, pero resulta útil con todo tipo de contenido. Incluso puede ayudar a evitar anuncios tediosos para aquellos que aún no están suscritos a YouTube Premium.
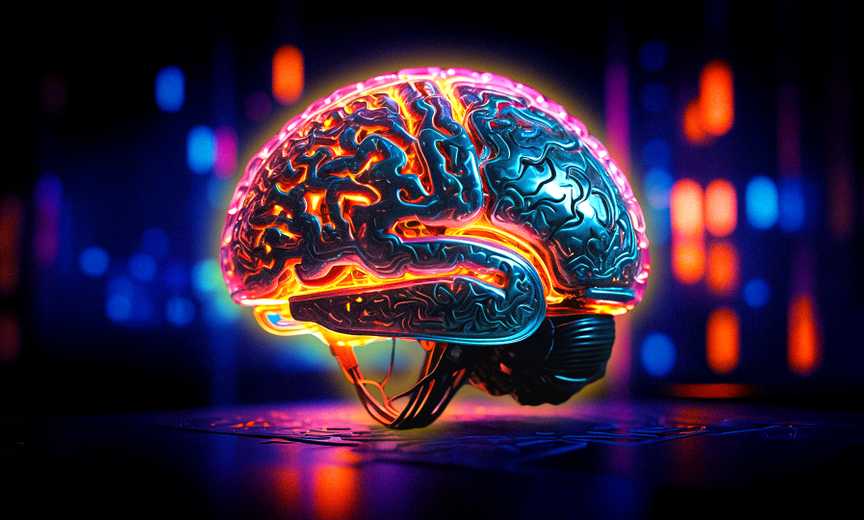
Relacionado
El vicepresidente senior de Arm explica el verdadero impacto de la IA, y no es lo que piensas
Nuestra entrevista con el ejecutivo de Arm, Chris Bergey
11 Resumir conjuntos de datos grandes y complejos
Analizar cantidades abrumadoras de información
Si tiene una hoja de cálculo con demasiadas páginas y columnas para entenderla rápidamente, pídale a Gemini que la desglose por usted. Puede extraer inferencias generales de toda la colección de datos, investigar tendencias dentro de categorías individuales e incluso ayudar a desarrollar gráficos para visualizar la información. Puede solicitar resultados más simples o más complejos si todavía tiene problemas para comprender un documento o necesita información más detallada.
10 Autocompletar hojas de cálculo según el contexto
Complete rápidamente la entrada de datos repetitivos
Fuente: Google
La función mejorada Smart Fill de Gemini en Google Sheets detecta patrones y proporciona soluciones automatizadas para la tediosa entrada de texto. Transfiere rápidamente datos relativamente simples de una columna o formato a otro. Gemini Autofill agrega una capa de integración de IA, identifica relaciones más complejas y sugiere formas de registrarlas de manera clara y efectiva. Estas dos características ejemplifican la utilidad de lo que algunos llaman un “autocompletado glorificado”.
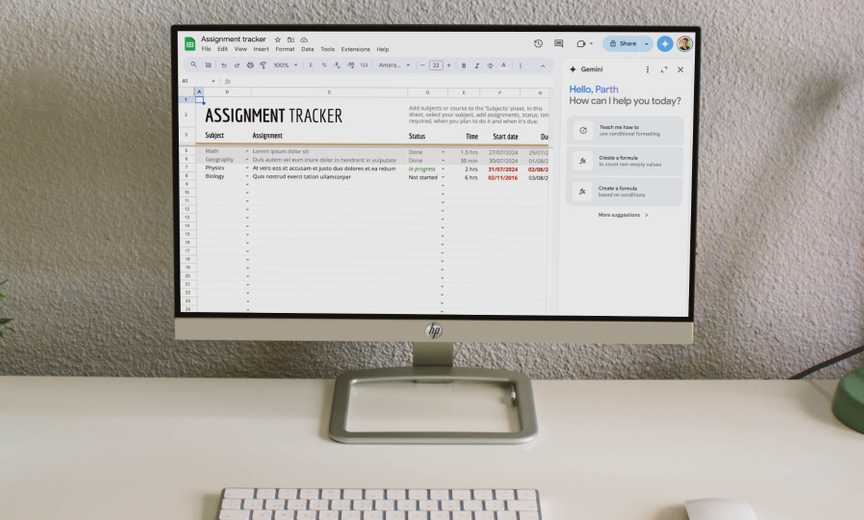
Relacionado
8 formas de usar Gemini en Google Sheets
Google Sheets con Gemini garantiza que tu día sea más fácil
9 Analice fácilmente la calidad SEO de los sitios web
Consulte las entradas del blog y los editoriales para comprobar su exhaustividad.
Un mensaje rápido le permite a Gemini examinar qué tan bien usted o un medio crearon un sitio. Puede compilar un informe sobre la cohesión de encabezados, meta descripciones, palabras clave y enlaces entrelazados. También puede usarlo junto con la página de resultados del motor de búsqueda para ver qué tan bien se está conectando con lo que quiere el público. Sobresale especialmente en generar preguntas de personas que también hacen y fragmentos destacados.
Con acceso a la herramienta de prueba de datos estructurados de Google, pocos agentes de inteligencia artificial pueden igualar la capacidad de Gemini para analizar la estructura y navegación de una página. Sin embargo, al igual que con otras solicitudes directas de análisis, asegúrese de comprobar los sentidos de las respuestas de Géminis. En lugar de confiar en que sus consejos de SEO sean correctos, utilice sus afirmaciones para comparar la página con las técnicas de SEO actuales y establecidas, y prepárese para solicitar revisiones o una segunda opinión.
8 Solucionar problemas y verificar el código
Rebotar ideas de programación de un modelo programado.
Los programadores han utilizado durante mucho tiempo patos de goma como oyentes inanimados para ayudar a encontrar problemas que se escapan de las grietas. Géminis puede responder, lo que lo hace aún más efectivo. Puede brindarle numerosos puntos de partida y métodos para abordar una tarea de programación y evaluar la eficiencia con la que se ejecutará su código ya escrito.
Querrá comparar sus sugerencias con sus conocimientos y recursos y, a veces, se topa con callejones sin salida o alucina, pero es mucho más efectivo que un juguete de baño.
7 Crea itinerarios de viaje inspiradores
Deje que Géminis actúe como trampolín para sus planes de vacaciones
Utilice Gemini para Google Sheets para crear planes de viaje perfectos, ya sea para un viaje de trabajo o recreativo. Puede dirigirlo a los sitios más famosos, mostrarle más atracciones que pasan desapercibidas e incluso presentarle algunos lugares locales oscuros. Naturalmente, siempre puedes utilizar sus itinerarios de muestra para ayudarte a pensar dónde quieres ir y qué quieres hacer, eliminando el estrés de planificar salidas de larga distancia.
6 Diseñe indicaciones de IA más efectivas
Cómo Gemini te ayuda a usar Gemini
Decirle al asistente de inteligencia artificial que necesita ayuda con el mensaje de Géminis correcto puede desbloquear estrategias que de otro modo tendría que investigar y adivinar. Esto tiene sentido, porque ¿qué conoce mejor a Géminis que él? Describir sus necesidades, incluido el contexto, el formato y el grado de especificidad, puede reducir la cantidad de tiempo necesario para obtener información útil. También puede adaptar el resultado al tono adecuado para comunicarse con grupos específicos de personas.
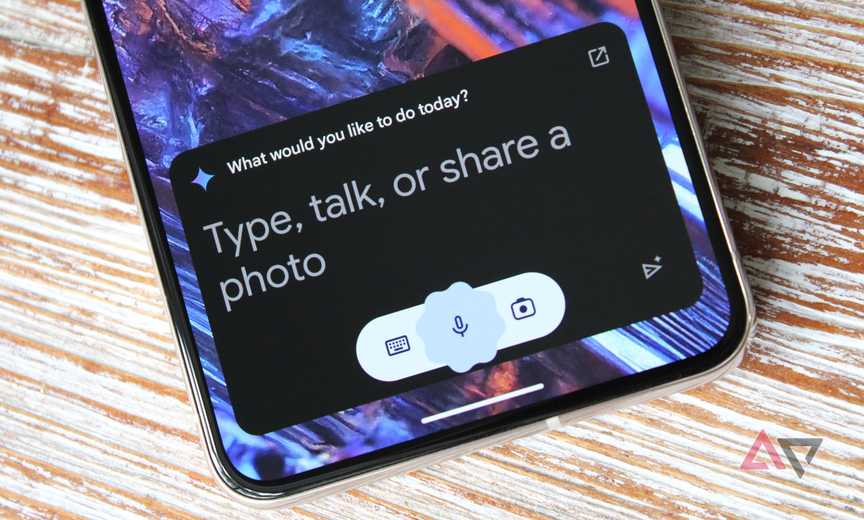
Relacionado
Aquí se explica cómo avisar a Géminis, según Google
Google publicó una hoja de trucos para aprovechar al máximo su herramienta de IA
5 Simplifique la redacción técnica para lectores generales
Reduzca la jerga y optimice los temas complicados
Para un escritor técnico que explica temas avanzados a generalistas, o para un profano que intenta comprender las complejidades de un campo de alta tecnología, Gemini hace maravillas al simplificar temas confusos. Reemplaza la terminología específica de un campo con una redacción sencilla y ayuda a lectores y escritores de ambos extremos del espectro a mantenerse en sintonía.
4 Encuentre respuestas a preguntas oscuras
Cuando la Búsqueda de Google no es suficiente
Es difícil obtener las respuestas exactas a algunas preguntas, pero el vasto Gráfico de conocimiento de Google vincula su enorme variedad de información de maneras poderosas. Mejora su capacidad para investigar y crear contenido informativo en profundidad, especialmente cuando utiliza Gemini en Google Docs.
Gemini y otros LLM cometen errores y siempre necesitarás volver a verificar sus respuestas, pero copiar y pegar rápidamente lo hace fácil. Al menos, buscar en la web a través de Gemini le brinda el trampolín perfecto para responder consultas extremadamente específicas.
3 Implementar fórmulas avanzadas de hojas de cálculo
Omita el proceso de dominar Google Sheets
Ya no necesitas pasar incontables horas dominando hojas de cálculo para implementar las fórmulas más efectivas. Gemini para Google Sheets ya hace un trabajo fantástico al sugerir las operaciones correctas y siempre está mejorando. La gestión competente de hojas de cálculo puede hacer o deshacer un equipo, lo que la convierte en una poderosa herramienta profesional.
2 Prepárese para entrevistas y reuniones importantes
Haga que Géminis haga las preguntas para evitar que lo tomen por sorpresa
Fuente: Policía de Android
No hay nada mejor que practicar para entrevistas individuales y paneles de discusión grupales. Proporcione a Gemini su tesis, currículum o tema y recursos de discusión, y pídale que le interrogue sobre contenido relacionado. Rápidamente desarrollarás una comprensión más profunda de tu propio material e incluso podría ayudarte a conseguir un trabajo o un ascenso.
1 Ajuste los agentes de IA de Gems personalizados
Tenga a mano asistentes de IA personalizados en todo momento
Fuente: Google
Los agentes de IA de Google Gems le permiten personalizar un agente específico para los temas, formatos y tonos que necesita habitualmente. Desarrolla el tuyo propio y mantenlo a tu lado para tus tareas más habituales. Esto puede reducir en gran medida la cantidad de tiempo y esfuerzo que se necesita para usar la IA, de otra manera que Gemini te ayuda a usarla.
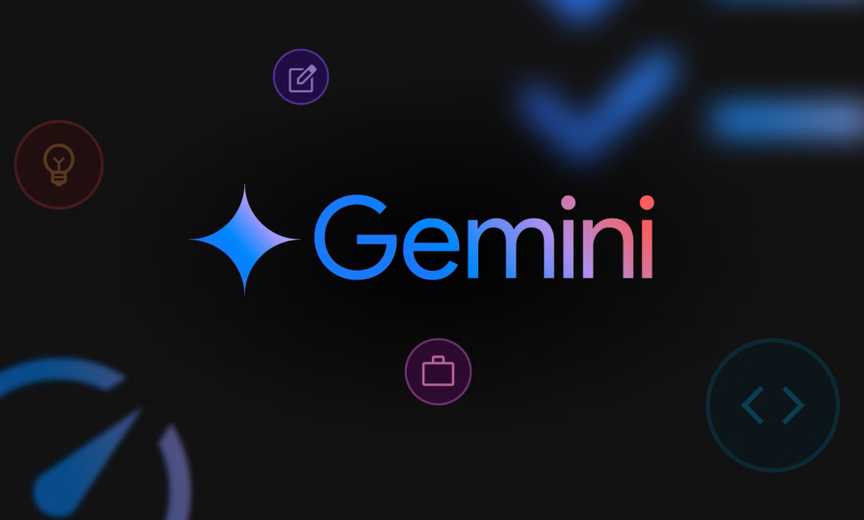
Relacionado
Cómo crear chatbots de IA personalizados con Gemini Gems
Crea un chatbot personalizado usando Gemini Gems
Las posibilidades casi infinitas siguen aumentando
A medida que Google integre aún más Gemini en servicios potentes como la aplicación Workspace, su efectividad solo mejorará, especialmente considerando el procesamiento de IA en el dispositivo que permiten los mejores teléfonos inteligentes de la actualidad. Reduce la complejidad de la capacitación, la comunicación, el resumen y la creación, y puede ayudarlo a mantener sus pensamientos en orden cuando aparentemente tiene demasiada información que manejar.
Si utiliza estos u otros consejos de productividad con frecuencia, probablemente se beneficiará de una suscripción Gemini Advanced, que brinda acceso a un procesamiento más potente que el Gemini estándar.
Noticias
7 razones por las que Google Gemini es mejor que ChatGPT
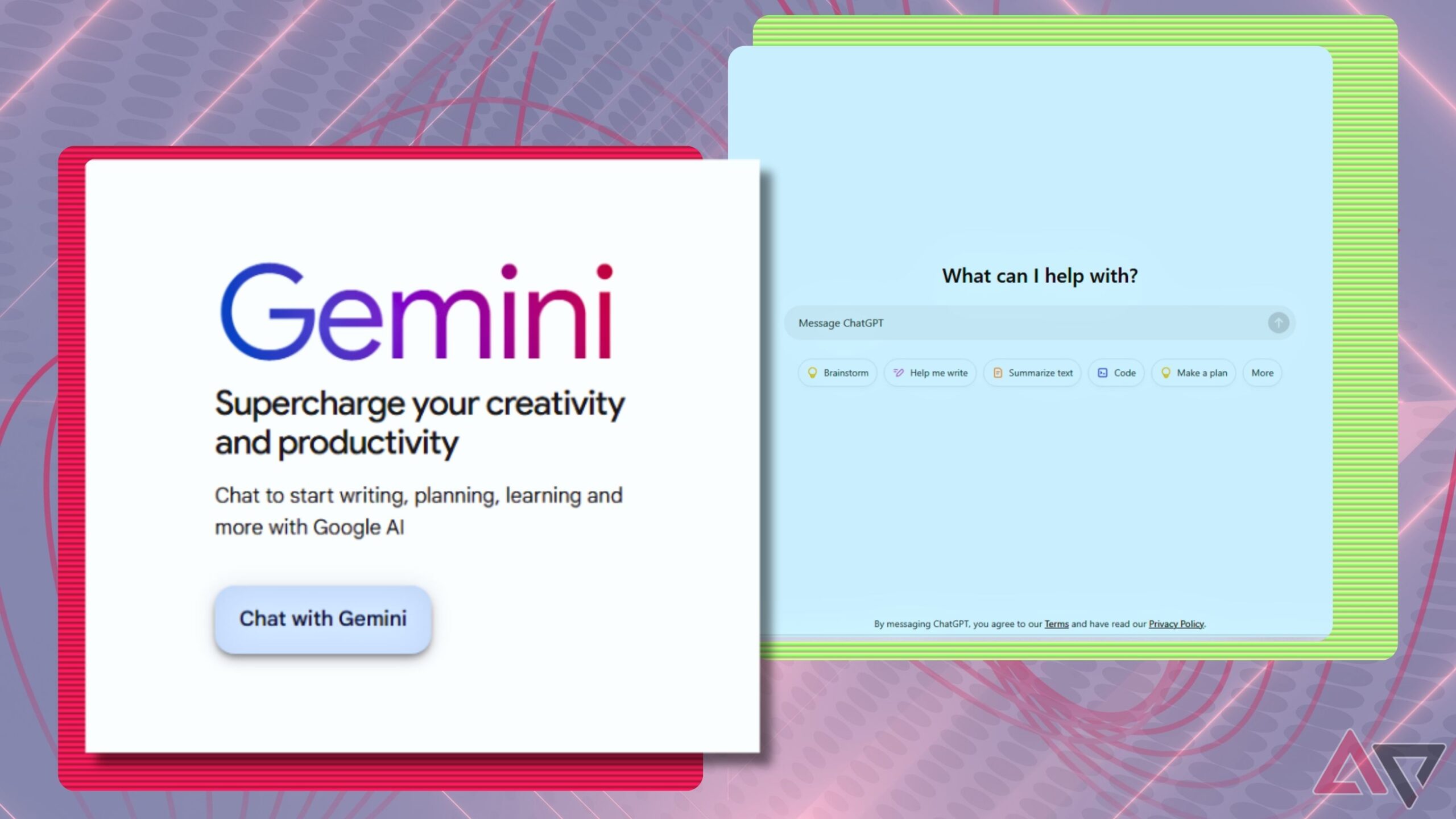
Con el año 2024 casi detrás de nosotros, los chatbots de IA se han vuelto esenciales para muchos, con ChatGPT como la opción preferida. De hecho, Sam Altman de OpenAI señaló recientemente que ChatGPT es ahora el octavo sitio web más visitado a nivel mundial. Sin embargo, Google ha estado mejorando constantemente su propio chatbot, Gemini, y se está poniendo al día rápidamente. Aquí hay algunas razones por las que Google Gemini podría ser una mejor opción que ChatGPT para usted.
Relacionado
ChatGPT vs. Gemini: ¿Cuál da las mejores respuestas?
Gemini le ofrece a ChatGPT una competencia muy necesaria
7 Gemini ofrece múltiples respuestas para cada consulta
Más respuestas significan más perspectivas
Si bien ChatGPT es excelente para responder preguntas, solo proporciona una respuesta a la vez. Google Gemini, sin embargo, genera tres respuestas diferentes a cada pregunta de forma predeterminada; simplemente puedes tocar el Mostrar borradores para ver estas respuestas alternativas. Por el contrario, con ChatGPT, debes hacer clic manualmente en el botón regenerar si deseas una respuesta diferente.
Como se muestra en la captura de pantalla anterior, cuando le pedí a Gemini que me ayudara a escribir una solicitud de licencia, me ofreció tres respuestas únicas en diferentes estilos. Esta función es particularmente útil para tareas creativas, como redactar correos electrónicos o documentos, ya que ofrece instantáneamente múltiples opciones para elegir.
6 Géminis ofrece respuestas más concisas
Respuestas directas sin detalles innecesarios.
Cuando se trata de estilo de respuesta, Gemini de Google a menudo proporciona respuestas más concisas y directas en comparación con ChatGPT. ChatGPT tiende a ser más detallado, incluso con preguntas sencillas. Si bien puede pedirle que acorte o resuma, si prefiere respuestas rápidas y sencillas, Géminis es la mejor opción.
Por ejemplo, como se muestra arriba, les pregunté a ambos chatbots cómo funcionan las vacunas. La respuesta de ChatGPT tuvo alrededor de 350 palabras, mientras que Gemini devolvió una respuesta más breve que cubría todos los puntos esenciales.
5 Géminis puede comprender consultas más complejas
Maneja mensajes más largos gracias a un límite de token más alto
Una de las ventajas destacadas de Gemini sobre ChatGPT es su ventana de contexto significativamente mayor (límite de token). Mientras que el modelo GPT-4 de OpenAI puede manejar hasta 128.000 tokens, Google Gemini 1.5 Pro cuenta con un límite de tokens de un millón. En pocas palabras, esto significa que Gemini puede procesar consultas mucho más largas y complejas con facilidad. Puede profundizar en su solicitud, recogiendo detalles que podrían perderse en modelos con límites de token más bajos.
Por ejemplo, si necesita trabajar con archivos PDF largos o desea que un chatbot de IA resuma un documento de texto grande, Gemini manejará la tarea de manera más efectiva que ChatGPT.
4 Google Gemini ya está disponible
Integrado en Android: no es necesario realizar descargas adicionales
La mejor parte de usar Gemini es su perfecta disponibilidad en dispositivos Android. Si bien Google ofrece una aplicación Gemini independiente, también puedes acceder a ella simplemente cambiando tu asistente predeterminado a Gemini en la configuración de Android. De hecho, en dispositivos más nuevos como la serie Pixel 9, Google incluso incluye Gemini como asistente predeterminado, reemplazando al Asistente de Google.
Por el contrario, usar ChatGPT en Android requiere descargar una aplicación separada de Play Store, lo cual no es tan conveniente como Gemini. Además, Gemini se está integrando en el conjunto de aplicaciones y servicios de Google, pero hablaremos más sobre eso a continuación.
3 Géminis puede acceder a los servicios de Google
Se integra perfectamente con Drive, YouTube, Gmail y más
Dado que Gemini es un producto de Google, se beneficia de una integración perfecta con los numerosos servicios de Google que utilizamos a diario. Google ha implementado extensiones Gemini para YouTube, Gmail, Docs, Drive y más en los últimos meses, lo que permite que Gemini funcione de forma nativa dentro de estas aplicaciones.
Y como Gemini se conecta a través de tu cuenta de Google, ya tiene la información que necesita. Por ejemplo, puede pedirle a Gemini que busque un correo electrónico específico, resuma un correo electrónico extenso o inserte sus respuestas directamente en Google Docs sin copiar ni pegar. Incluso puedes hacer que resuma videos de YouTube o muestre fotos de viajes anteriores.
Le pedí a Gemini que obtuviera detalles sobre mis vuelos recientes con una aerolínea específica y mostró exactamente lo que necesitaba. No tuve que buscar en mi Gmail para buscar manualmente. Con estas integraciones, Gemini ofrece una experiencia más conectada que ChatGPT, que carece de soporte integrado para servicios populares y, a menudo, requiere que usted ingrese información manualmente.
2 El plan gratuito de Gemini permite la creación ilimitada de imágenes
Genera tantas imágenes como quieras sin límites
Si utiliza con frecuencia chatbots de IA para generar imágenes, Gemini puede ser la mejor opción. Incluso en su plan gratuito, Google permite la creación ilimitada de imágenes con tantos ajustes como necesites. No solo eso, sino que la compañía también brinda acceso a su último modelo Imagen 3 AI sin restricciones. Mientras tanto, el plan gratuito de ChatGPT limita a los usuarios a tres imágenes cada 24 horas, lo que puede no ser suficiente para muchos.
1 El plan pago de Gemini ofrece más valor
Incluye 2 TB de almacenamiento de Google y una prueba gratuita más larga
Si bien tanto ChatGPT como Gemini ofrecen planes pagos a $20 por mes, el plan AI One Premium de Google ofrece notablemente más valor. Por el mismo precio, obtienes acceso a Gemini Advanced (impulsado por el modelo Gemini Pro de alto rendimiento) y 2 TB adicionales de almacenamiento de Google, utilizables en Drive, Fotos y otros servicios.
Con el plan pago, también te beneficias de la integración de Gemini en aplicaciones de Google Workspace como Docs y Gmail. Además, Google ofrece una prueba gratuita de dos meses para la versión paga de Gemini, mientras que ChatGPT no ofrece ninguna prueba gratuita. El plan pago de ChatGPT simplemente otorga acceso a todos los modelos de IA con mayor uso, sin ningún beneficio adicional.
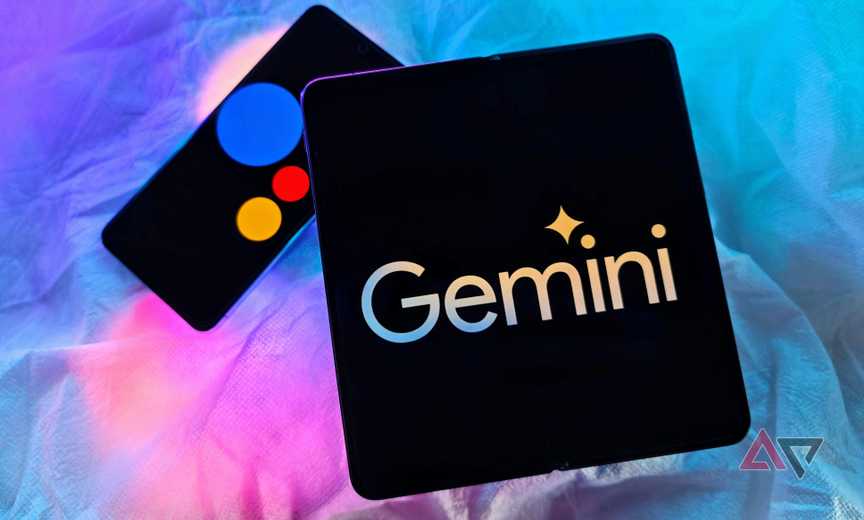
Relacionado
5 características que Google Gemini debería robarle a ChatGPT
No dejes atrás estas gemas, Géminis: róbalas de ChatGPT
Gemini está cerrando la brecha con ChatGPT
Si bien OpenAI pudo haber tenido una ventaja inicial en la carrera de los chatbots de IA, Google rápidamente ha cerrado la brecha. Dado lo integrales que son los servicios de Google en la vida diaria, ya sea mirar videos en YouTube o navegar con Maps, la integración de Gemini en estas plataformas le brinda una ventaja sustancial sobre ChatGPT. Gemini es sin lugar a dudas una de las mejores alternativas a ChatGPT disponibles en la actualidad.
Noticias
OpenAI lanza el modo de voz avanzado ChatGPT para usuarios web
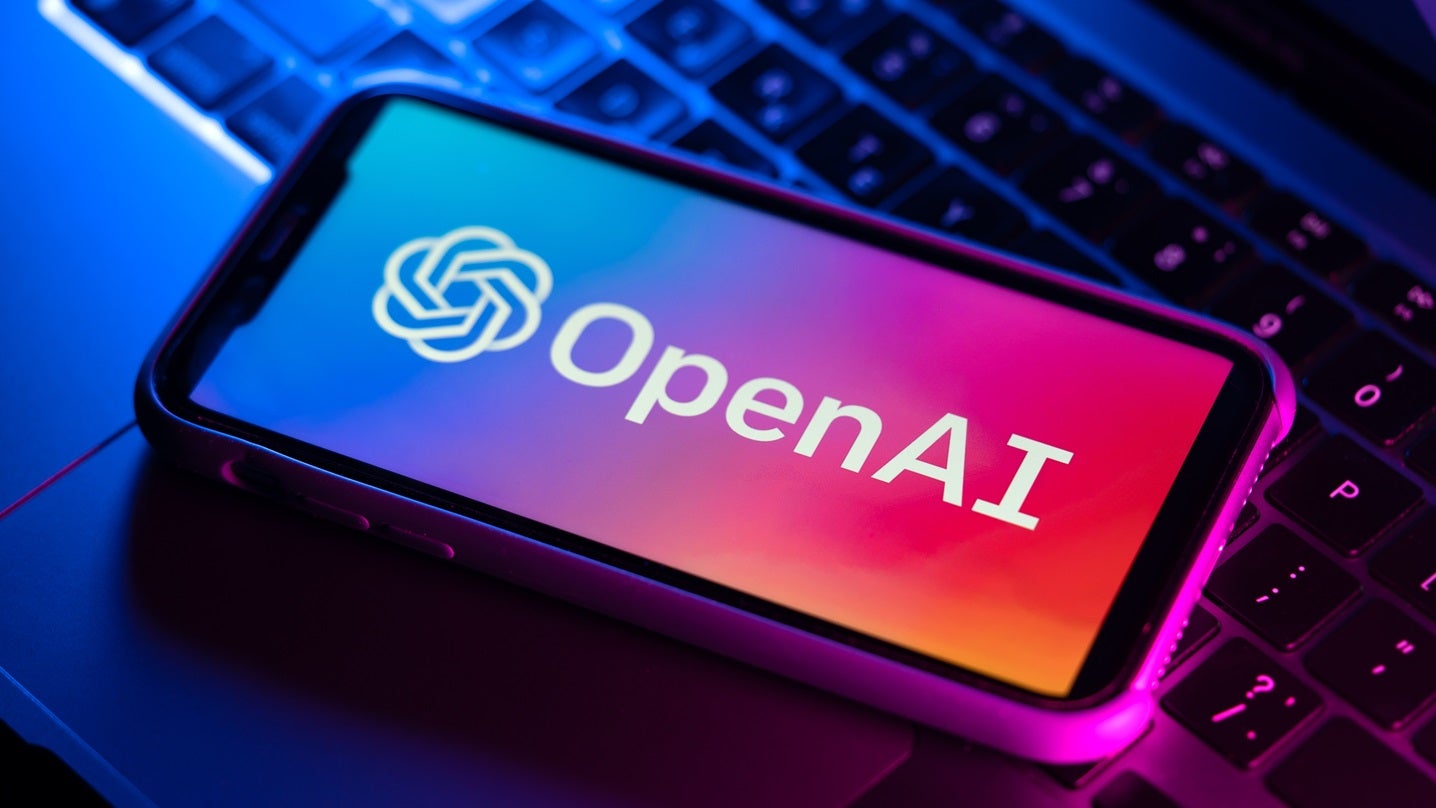
OpenAI ha ampliado la disponibilidad del modo de voz avanzado de ChatGPT, llevando la función a los navegadores web.
La introducción del modo de voz avanzado en la web fue anunciada por el director de producto de OpenAI, Kevin Weil, en la plataforma de redes sociales X.
Esta funcionalidad avanzada ahora es accesible para los suscriptores de los planes pagos de OpenAI, incluidos Plus, Enterprise, Teams o Edu.
Weil afirmó que la función, que aprovecha las capacidades de audio nativas de GPT-4 para conversaciones naturales en tiempo real, estaría disponible para los clientes de pago a partir de esta semana.
La medida se produce tras el lanzamiento exitoso de la misma función en aplicaciones de iOS y Android en septiembre de 2024.
Weil dijo que OpenAI planea lanzar la función para usuarios gratuitos en “las próximas semanas”.
Acceda a los perfiles de empresa más completos del mercado, impulsados por GlobalData. Ahorre horas de investigación. Obtenga una ventaja competitiva.
Perfil de la empresa: muestra gratuita
¡Gracias!
Su correo electrónico de descarga llegará en breve
Confiamos en la calidad única de nuestros perfiles de empresa. Sin embargo, queremos que tome la decisión más beneficiosa para su negocio, por eso le ofrecemos una muestra gratuita que puede descargar enviando el siguiente formulario.
Por GlobalData
En un comunicado de prensa sobre X, Weil dijo: “Lanzamos el modo de voz avanzado en nuestras aplicaciones de iOS y Android en septiembre, y recientemente lo llevamos a nuestras aplicaciones de escritorio”.
Weil añadió: “Ahora estamos entusiasmados de añadir la web a la mezcla. Esto significa que ahora puedes hablar con ChatGPT directamente desde tu navegador.
“Utilizo el modo de voz para explorar ideas y hacer preguntas rápidas sin tener que escribir. A @thirdweil y a los niños les encanta; siempre piden “hablar con ChatGPT” mientras conducimos. Y recientemente lo usé como traductor universal en Seúl y Tokio, manteniendo conversaciones de negocios con personas con las que no tenía un idioma común”.
En octubre de 2024, OpenAI introdujo la integración de búsqueda web en su chatbot de inteligencia artificial (IA) generativa ChatGPT.
Con la actualización, los suscriptores de ChatGPT Plus y Team tienen acceso a la información directamente a través de la interfaz de chat. Esto amplía las capacidades del bot más allá de sus limitaciones de datos históricos anteriores.
-
Recursos6 meses ago
Suno.com: La Revolución en la Creación Musical con Inteligencia Artificial
-
Startups6 meses ago
Remove.bg: La Revolución en la Edición de Imágenes que Debes Conocer
-
Recursos6 meses ago
Cómo Empezar con Popai.pro: Tu Espacio Personal de IA – Guía Completa, Instalación, Versiones y Precios
-
Recursos6 meses ago
Perplexity aplicado al Marketing Digital y Estrategias SEO
-
Eventos6 meses ago
La nueva era de la inteligencia artificial por el Washington Post – Mayo 2024
-
Noticias4 meses ago
Dos periodistas octogenarios deman a ChatGPT por robar su trabajo
-
Estudiar IA6 meses ago
Curso de Inteligencia Artificial de UC Berkeley estratégico para negocios
-
Tutoriales6 meses ago
Cómo Comenzar a Utilizar ChatGPT: Una Guía Completa para Principiantes