Noticias
Sam Altman Reveals This Prior Flaw In OpenAI Advanced AI o1 During ChatGPT Pro Announcement But Nobody Seemed To Widely Notice
Published
5 meses agoon
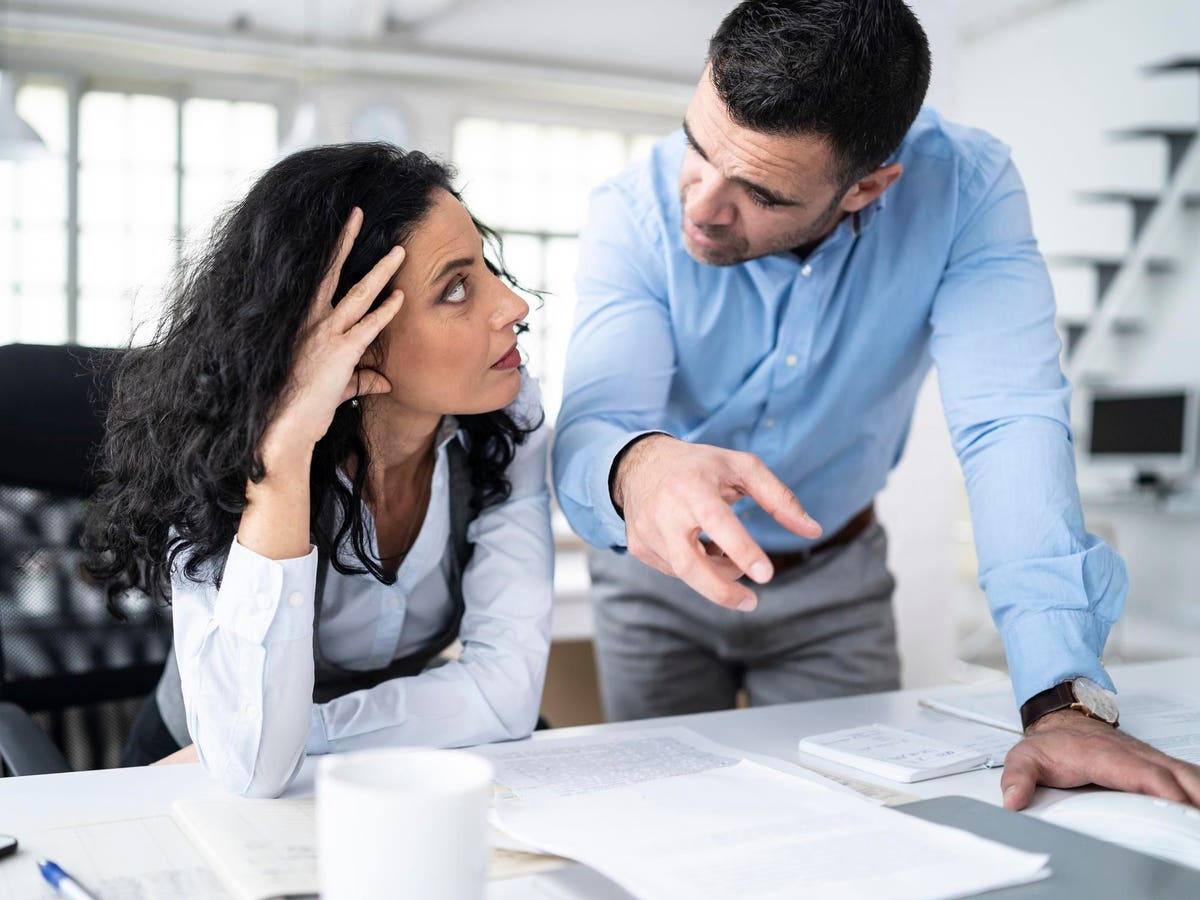
A hidden flaw or inconvenience in OpenAI o1 got recently aired and though fixed it raises … [+] significant considerations about present-day and future AI.
getty
In today’s column, I examine a hidden flaw in OpenAI’s advanced o1 AI model that Sam Altman revealed during the recent “12 Days Of OpenAI” video-streamed ChatGPT Pro announcement. His acknowledgment of the flaw was not especially noted in the media since he covered it quite nonchalantly in a subtle hand-waving fashion and claimed too that it was now fixed. Whether the flaw or some contend “inconvenience” was even worthy of consideration is another intriguing facet that gives pause for thought about the current state of AI and how far or close we are to the attainment of artificial general intelligence (AGI).
Let’s talk about it.
This analysis of an innovative proposition is part of my ongoing Forbes column coverage on the latest in AI including identifying and explaining various impactful AI complexities (see the link here). For my analysis of the key features and vital advancements in the OpenAI o1 AI model, see the link here and the link here, covering various aspects such as chain-of-thought reasoning, reinforcement learning, and the like.
How Humans Respond To Fellow Humans
Before I delve into the meat and potatoes of the matter, a brief foundational-setting treatise might be in order.
When you converse with a fellow human, you normally expect them to timely respond as based on the nature of the conversation. For example, if you say “hello” to someone, the odds are that you expect them to respond rather quickly with a dutiful reply such as hello, hey, howdy, etc. There shouldn’t be much of a delay in such a perfunctory response. It’s a no-brainer, as they say.
On the other hand, if you ask someone to explain the meaning of life, the odds are that any seriously studious response will start after the person has ostensibly put their thoughts into order. They would presumably give in-depth consideration to the nature of human existence, including our place in the universe, and otherwise assemble a well-thought-out answer. This assumes that the question was asked in all seriousness and that the respondent is aiming to reply in all seriousness.
The gist is that the time to respond will tend to depend on the proffered remark or question.
A presented simple comment or remark involving no weighty question or arduous heaviness ought to get a fast response. The responding person doesn’t need to engage in much mental exertion in such instances. You get a near-immediate response. If the presented utterance has more substance to it, we will reasonably allow time for the other person to undertake a judicious reflective moment. A delay in responding is perfectly fine and fully expected in that case.
That is the usual cadence of human-to-human discourse.
Off-Cadence Timing Of Advanced o1 AI
For those that had perchance made use of the OpenAI o1 AI advanced model, you might have noticed something that was outside of the cadence that I just mentioned. The human-to-AI cadence bordered on being curious and possibly annoying.
The deal was this.
You were suitably forewarned when using o1 that to get the more in-depth answers there would be more extended time after entering a prompt and before getting a response from the AI. Wait time went up. This has to do with the internally added capabilities of advanced AI functionality including chain-of-thought reasoning, reinforcement learning, and so on, see my explanation at the link here. The response latency time had significantly increased.
Whereas in earlier and less advanced generative AI and LLMs we had all gotten used to near instantaneous responses, by and large, there was a willingness to wait longer to get more deeply mined responses via advanced o1 AI. That seems like a fair tradeoff. People will wait longer if they can get better answers. They won’t wait longer if the answers aren’t going to be better than when the response time was quicker.
You can think of this speed-of-response as akin to playing chess. The opening move of a chess game is usually like a flash. Each side quickly makes their initial move and countermove. Later in the game, the time to respond is bound to slow down as each player puts concentrated thoughts into the matter. Just about everyone experiences that expected cadence when playing chess.
What was o1 doing in terms of cadence?
Aha, you might have noticed that when you gave o1 a simple prompt, including even merely saying hello, the AI took about as much time to respond as when answering an extremely complex question. In other words, the response time was roughly the same for the simplest of prompts and the most complicated and deep-diving fully answered responses.
It was a puzzling phenomenon and didn’t conform to any reasonable human-to-AI experience expected cadence.
In coarser language, that dog don’t hunt.
Examples Of What This Cadence Was Like
As an illustrative scenario, consider two prompts, one that ought to be quickly responded to and the other that fairly we would allow more time to see a reply.
First, a simple prompt that ought to lead to a simple and quick response.
- My entered prompt: “Hi.”
- Generative AI response: “Hello, how can I help you?”
The time between the prompt and the response was about 10 seconds.
Next, I’ll try a beefy prompt.
- My entered prompt: “Tell me how all of existence first began, covering all known theories.”
- Generative AI response: “Here is a summary of all available theories on the topic…”
The time for the AI to generate a response to that beefier question was about 12 seconds.
I think we can agree that the first and extremely simple prompt should have had a response time of just a few seconds at most. The response time shouldn’t be nearly the same as when responding to the question about all of human existence. Yet, it was.
Something is clearly amiss.
But you probably wouldn’t have complained since the aspect that you could get in-depth answers was worth the irritating and eyebrow-raising length of wait time for the simpler prompts. I dare say most users just shrugged their shoulders and figured it was somehow supposed to work that way.
Sam Altman Mentioned That This Has Been Fixed
During the ChatGPT Pro announcement, Sam Altman brought up the somewhat sticky matter and noted that the issue had been fixed. Thus, you presumably should henceforth expect a fast response time to simple prompts. And, as already reasonably expected, only prompts requiring greater intensity of computational effort ought to take up longer response times.
That’s how the world is supposed to work. The universe has been placed back into proper balance. Hooray, yet another problem solved.
Few seemed to catch onto his offhand commentary on the topic. Media coverage pretty much skipped past that portion and went straight to the more exciting pronouncements. The whole thing about the response times was likely perceived as a non-issue and not worthy of talking about.
Well, for reasons I’m about to unpack, I think it is worthy to ruminate on.
Turns out there is a lot more to this than perhaps meets the eye. It is a veritable gold mine of intertwining considerations about the nature of contemporary AI and the future of AI. That being said, I certainly don’t want to make a mountain out of a molehill, but nor should we let this opportune moment pass without closely inspecting the gold nuggets that were fortuitously revealed.
Go down the rabbit hole with me, if you please.
Possible Ways In Which This Happened
Let’s take a moment to examine various ways in which the off-balance cadence in the human-to-AI interaction might have arisen. OpenAI considers their AI to be proprietary and they don’t reveal the innermost secrets, ergo I’ll have to put on my AI-analysis detective hat and do some outside-the-box sleuthing.
First, the easiest way to explain things is that an AI maker might decide to hold back all responses until some timer says to release the response.
Why do this?
A rationalization is that the AI maker wants all responses to come out roughly on the same cadence. For example, even if a response has been computationally determined in say 2 seconds, the AI is instructed to keep the response at bay until the time reaches say 10 seconds.
I think you can see how this works out to a seemingly even cadence. A tough-to-answer query might require 12 entire seconds. The response wasn’t ready until after the timer was done. That’s fine. At that juncture, you show the user the response. Only when a response takes less than the time limit will the AI hold back the response.
In the end, the user would get used to seeing all responses arising at above 10 seconds and fall into a mental haze that no matter what happens, they will need to wait at least that long to see a response. Boom, the user is essentially being behaviorally trained to accept that responses will take that threshold of time. They don’t know they are being trained. Nothing tips them to this ruse.
Best of all, from the AI maker’s perspective, no one will get upset about timing since nothing ever happens sooner than the hidden limit anyway. Elegant and the users are never cognizant of the under-the-hood trickery.
The Gig Won’t Last And Questions Will Be Asked
The danger for the AI maker comes to the fore when software sophisticates start to question the delays. Any proficient software developer or AI specialist would right away be suspicious that the simplest of entries is causing lengthy latency. It’s not a good look. Insiders begin to ask what’s up with that.
If a fake time limit is being used, that’s often frowned upon by insiders who would shame those developers undertaking such an unseemly route. There isn’t anything wrong per se. It is more of a considered low-brow or discreditable act. Just not part of the virtuous coding sense of ethos.
I am going to cross out that culprit and move toward a presumably more likely suspect.
It goes like this.
I refer to this other possibility as the gauntlet walk.
A brief tale will suffice as illumination. Imagine that you went to the DMV to get up-to-date license tags for your car. In theory, if all the paperwork is already done, all you need to do is show your ID and they will hand you the tags. Some modernized DMVs have an automated kiosk in the lobby that dispenses tags so that you can just scan your ID and viola, you instantly get your tags and walk right out the door. Happy face.
Sadly, some DMVs are not yet modernized. They treat all requests the same and make you wait as though you were there to have surgery done. You check in at one window. They tell you to wait over there. Your name is called, and you go to a pre-processing window. The agent then tells you to wait in a different spot until your name is once again called. At the next processing window, they do some of the paperwork but not all of it. On and on this goes.
The upshot is that no matter what your request consists of you are by-gosh going to walk the full gauntlet. Tough luck to you. Live with it.
A generative AI app or large language model (LLM) could be devised similarly. No matter what the prompt contains, an entire gauntlet of steps is going to occur. Everything must endure all the steps. Period, end of story.
In that case, you would typically have responses arriving outbound at roughly the same time. This could vary somewhat because the internal machinery such as the chain of thought mechanism is going to pass through the tokens without having to do nearly the same amount of computational work, see my explanation at the link here. Nonetheless, time is consumed even when the content is being merely shunted along.
That could account for the simplest of prompts taking much longer than we expect them to take.
How It Happens Is A Worthy Question
Your immediate thought might be why in the heck would a generative AI app or LLM be devised to treat all prompts as though they must walk the full gauntlet. This doesn’t seem to pass the smell test. It would seem obvious that a fast path like at Disneyland should be available for prompts that don’t need the whole kit-and-kaboodle.
Well, I suppose you could say the same about the DMV. Here’s what I mean. Most DMVs were probably set up without much concern toward allowing multiple paths. The overall design takes a lot more contemplation and building time to provide sensibly shaped forked paths. If you are in a rush to get a DMV underway, you come up with a single path that covers all the bases. Therefore, everyone is covered. Making everyone wait the same is okay because at least you know that nothing will get lost along the way.
Sure, people coming in the door who have trivial or simple requests will need to wait as long as those with the most complicated of requests, but that’s not something you need to worry about upfront. Later, if people start carping about the lack of speediness, okay, you then try to rejigger the process to allow for multiple paths.
The same might be said for when trying to get advanced AI out the door. You are likely more interested in making sure that the byzantine and innovative advanced capabilities work properly, versus whether some prompts ought to get the greased skids.
A twist to that is the idea that you are probably more worried about maximum latencies than you would be about minimums. This stands to reason. Your effort to optimize is going to focus on trying to keep the AI from running endlessly to generate a response. People will only wait so long to get a response, even for highly complex prompts. Put your elbow grease toward the upper bounds versus the lower bounds.
The Tough Call On Categorizing Prompts
An equally tough consideration is exactly how you determine which prompts are suitably deserving of quick responses.
Well, maybe you just count the number of words in the prompt.
A prompt with just one word would seem unlikely to be worthy of the full gauntlet. Let it pass through or maybe skip some steps. This though doesn’t quite bear out. A prompt with a handful of words might be easy-peasy, while another prompt with the same number of words might be a doozy. Keep in mind that prompts consist of everyday natural language, which is semantically ambiguous, and you can open a can of worms with just a scant number of words.
This is not like sorting apples or widgets.
All in all, a prudent categorization in this context cannot do something blindly such as purely relying on the number of words. The meaning of the prompt comes into the big picture. A five-word prompt that requires little computational analysis is likely only discerned as a small chore by determining what the prompt is all about.
Note that this means you indubitably have to do some amount of initial processing to gauge what the prompt constitutes. Once you’ve got that first blush done, you can have the AI flow the prompt through the other elements with a kind of flag that indicates this is a fly-by-night request, i.e., work on it quickly and move it along.
You could also establish a separate line of machinery for the short ones, but that’s probably more costly and not something you can concoct overnight. DMVs often kept the same arrangement inside the customer-facing processing center and merely adjusted by allowing the skipping of windows. Eventually, newer avenues were developed such as the use of automated kiosks.
Time will tell in the case of AI.
There is a wide variety of highly technical techniques underlying prompt-assessment and routing issues, which I will be covering in detail in later postings so keep your eyes peeled. Some of the techniques are:
- (1) Prompt classification and routing
- (2) Multi-tier model architecture
- (3) Dynamic attention mechanisms
- (4) Adaptive token processing
- (5) Caching and pre-built responses
- (6) Heuristic cutoffs for contextual expansion
- (7) Model layer pruning on demand
I realize that seems relatively arcane. Admittedly, it’s one of those inside baseball topics that only heads-down AI researchers and developers are likely to care about. It is a decidedly niche aspect of generative AI and LLMs. In the same breath, we can likely agree that it is an important arena since people aren’t likely to use models that make them wait for simple prompts.
AI makers that seek widespread adoption of their AI wares need to give due consideration to the gauntlet walk problem.
Put On Your Thinking Cap And Get To Work
A few final thoughts before finishing up.
The prompt-assessment task is crucial in an additional fashion. The AI could inadvertently arrive at false positives and false negatives. Here’s what that foretells. Suppose the AI assesses that a prompt is simple and opts to therefore avoid full processing, but then the reality is that the answer produced is insufficient and the AI misclassified the prompt.
Oops, a user gets a shallow answer.
They are irked.
The other side of the coin is not pretty either. Suppose the AI assesses that a prompt should get the full treatment, shampoo and conditioner included, but essentially wastes time and computational resources such that the prompt should have been categorized as simple. Oops, the user waited longer than they should have, plus they paid for computational resources they needn’t have consumed.
Awkward.
Overall, prompt-assessment must strive for the Goldilocks principle. Do not be too cold or too hot. Aim to avoid false positives and false negatives. It is a dicey dilemma and well worth a lot more AI research and development.
My final comment is about the implications associated with striving for artificial general intelligence (AGI). AGI is considered the aspirational goal of all those pursuing advances in AI. The belief is that with hard work we can get AI to be on par with human intelligence, see my in-depth analysis of this at the link here.
How do the prompt-assessment issue and the vaunted gauntlet walk relate to AGI?
Get yourself ready for a mind-bending reason.
AGI Ought To Know Better
Efforts to get modern-day AI to respond appropriately such that simple prompts get quick response times while hefty prompts take time to produce are currently being devised by humans. AI researchers and developers go into the code and make changes. They design and redesign the processing gauntlet. And so on.
It seems that any AGI worth its salt would be able to figure this out on its own.
Do you see what I mean?
An AGI would presumably gauge that there is no need to put a lot of computational mulling toward simple prompts. Most humans would do the same. Humans interacting with fellow humans would discern that waiting a long time to respond is going to be perceived as an unusual cadence when in discourse covering simple matters. Humans would undoubtedly self-adjust, assuming they have the mental capacity to do so.
In short, if we are just a stone’s throw away from attaining AGI, why can’t AI figure this out on its own? The lack of AI being able to self-adjust and self-reflect is perhaps a telltale sign. The said-to-be sign is that our current era of AI is not on the precipice of becoming AGI.
Boom, drop the mic.
Get yourself a glass of fine wine and find a quiet place to reflect on that contentious contention. When digging into it, you’ll need to decide if it is a simple prompt or a hard one, and judge how fast you think you can respond to it. Yes, indeed, humans are generally good at that kind of mental gymnastics.
You may like
Noticias
El Proyecto Stargate de Openai tiene como objetivo construir infraestructura de IA en países asociados de todo el mundo
Published
3 horas agoon
19 mayo, 2025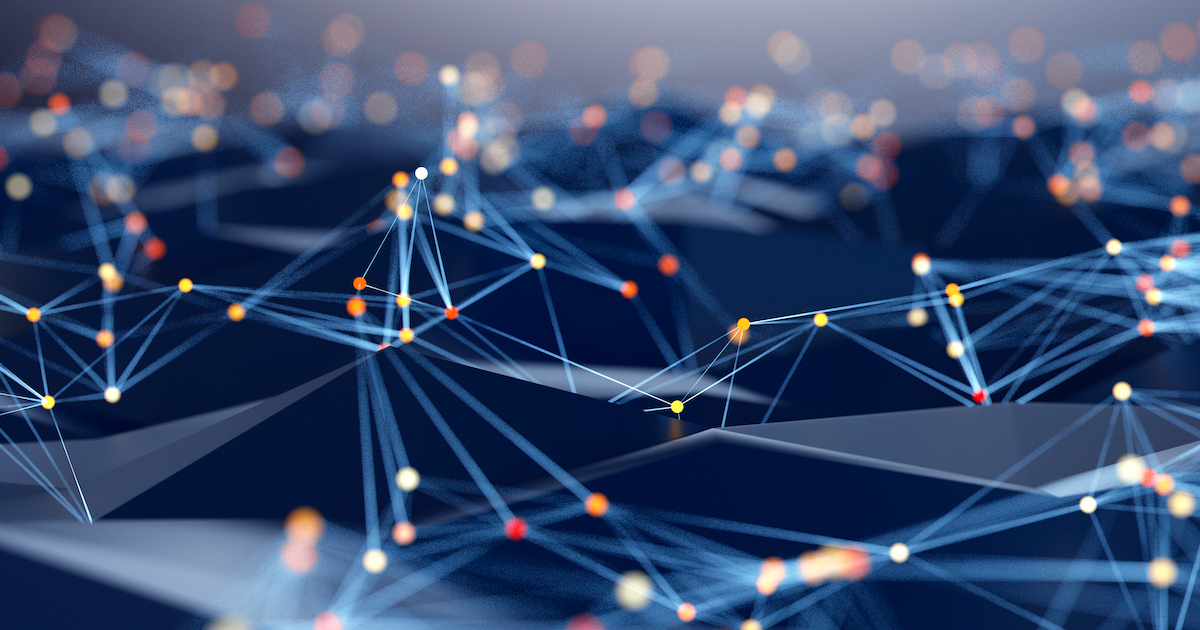
Operai ha anunciado una nueva iniciativa llamada “OpenAi para países” como parte de su proyecto Stargate, con el objetivo de ayudar a las naciones a desarrollar infraestructura de IA basada en principios democráticos. Esta expansión sigue al plan de inversión inicial de $ 500 millones de la compañía para la infraestructura de IA en los Estados Unidos.
“Introducción a OpenAi para países, una nueva iniciativa para apoyar a países de todo el mundo que desean construir sobre los rieles demócratas de IA”, declaró Openai en su anuncio. La compañía informa que su proyecto Stargate, reveló por primera vez en enero con el presidente Trump y los socios Oracle y Softbank, ha comenzado la construcción de su primer campus de supercomputación en Abilene, Texas.
Según OpenAI, la iniciativa responde al interés internacional en un desarrollo similar de infraestructura. “Hemos escuchado de muchos países pidiendo ayuda para construir una infraestructura de IA similar: que quieren sus propios Stargates y proyectos similares”, explicó la compañía, señalando que dicha infraestructura será “la columna vertebral del futuro crecimiento económico y el desarrollo nacional”.
La compañía enfatizó su visión de la IA democrática como tecnología que incorpora principios que protegen las libertades individuales y evitan la concentración de control del gobierno. Operai cree que este enfoque “contribuye a una amplia distribución de los beneficios de la IA, desalienta la concentración de poder y ayuda a avanzar en nuestra misión”.
El proyecto Stargate opera a través de un consorcio de principales compañías de tecnología que se desempeñan como inversores y socios técnicos. SoftBank, Openai, Oracle y MGX proporcionan la financiación inicial de capital, con las responsabilidades financieras de manejo de SoftBank, mientras que OpenAI administra las operaciones.
En el lado técnico, cinco compañías tecnológicas importantes forman la base de la implementación del proyecto. “Arm, Microsoft, Nvidia, Oracle y OpenAI son los socios de tecnología iniciales clave”, según OpenAI. El desarrollo de infraestructura aprovecha las relaciones establecidas entre estas compañías, particularmente basándose en la colaboración de larga data de OpenAI con Nvidia que se remonta a 2016 y su asociación más reciente con Oracle.
La compañía describe un marco integral de asociación para colaborar con naciones extranjeras.
“Openai está ofreciendo un nuevo tipo de asociación para la era de la inteligencia. A través de colaboraciones de infraestructura formal y en coordinación con el gobierno de los Estados Unidos”, explica el anuncio, destacando la alineación de la compañía con los intereses de política exterior estadounidense en el desarrollo tecnológico.
El modelo de asociación incluye múltiples componentes que abordan la infraestructura, el acceso y el desarrollo económico. Operai planea “asociarse con países para ayudar a construir capacidad de centro de datos en el país” para respaldar la soberanía de los datos al tiempo que permite la personalización de la IA para las necesidades locales.
Los ciudadanos de los países participantes recibirían servicios de “CHATGPT personalizados” adaptados a idiomas y culturas locales, destinados a mejorar la prestación de atención médica, educación y servicios públicos. Operai describe esto como “ai de, por y para las necesidades de cada país en particular”.
La compañía también enfatiza las inversiones de seguridad y el desarrollo económico a través de un enfoque de financiación de inicio donde “los países asociados también invertirían en la expansión del proyecto global de Stargate, y por lo tanto en el liderazgo continuo de IA liderado por Estados Unidos”, reforzando la conexión de la iniciativa con el liderazgo tecnológico estadounidense.
Las asociaciones internacionales de OpenAI incorporan amplios protocolos de seguridad diseñados para proteger los modelos de IA y la propiedad intelectual. La compañía ha desarrollado un enfoque de seguridad para abordar las posibles vulnerabilidades.
“Salvaguardar nuestros modelos es un compromiso continuo y un pilar central de nuestra postura de seguridad”, Estados Openai, que describe su marco de seguridad como “riguroso” y “evolucionando continuamente”. Este marco abarca la seguridad de la información, la gobernanza y la protección de la infraestructura física.
La arquitectura de seguridad se adapta a las capacidades del modelo de coincidencia, con OpenAi señalando que “nuestras medidas de seguridad no son estáticas; escaman con las capacidades de nuestros modelos e incorporan protecciones de vanguardia”. Estas protecciones incluyen seguridad respaldada por hardware, arquitectura de mudanza cero y salvaguardas criptográficas.
El acceso al personal representa otra dimensión de seguridad crítica. “Operai mantendrá una supervisión explícita y continua sobre todo el personal con acceso a nuestros sistemas de información, propiedad intelectual y modelos”, enfatiza la compañía, y agrega que “ninguna persona o entidad obtendrá dicho acceso sin nuestra aprobación directa”.
Antes de implementar modelos internacionalmente, OpenAI realiza evaluaciones de riesgos a través de su marco de preparación. “Cada implementación de nuevos modelos se someterá a una evaluación de riesgos antes de la implementación”, reconociendo que algunos modelos avanzados pueden presentar riesgos incompatibles con ciertos entornos.
El CEO de Operai, Sam Altman, expresó entusiasmo por el progreso en el sitio de Texas, tuiteando:
Genial ver el progreso en el primer Stargate en Abilene con nuestros socios en Oracle Today. Será la instalación de entrenamiento de IA más grande del mundo. La escala, la velocidad y la habilidad de las personas que construyen esto es increíble.
Sin embargo, el desarrollo masivo de infraestructura ha planteado preocupaciones ambientales. Greg Osuri, fundador de Akash Network, cuestionó el enfoque de sostenibilidad del proyecto:
Este centro de datos está generando 360 MW quemando gas natural, causando una fuerte contaminación y emitiendo hasta 1 millón de toneladas métricas de carbono cada año. Entiendo que las opciones son limitadas, pero me gustaría comprender sus planes futuros para cambiar a fuentes más limpias o sostenibles.
Zach DeWitt, socio de Wing VC, comentó las implicaciones más amplias de este movimiento:
Operai parece estar construyendo y vendiendo productos en cada capa de la pila de IA: chips, centros de datos, API y la capa de aplicación. No está claro qué capa (s) se comercializarán y no se comercializarán y OpenAi está cubriendo sus apuestas de arriba a abajo por la pila de IA. Muy inteligente.
La compañía ha especificado limitaciones geográficas para su estrategia de expansión internacional, manteniendo restricciones sobre las cuales las naciones pueden acceder a su tecnología a través de su documentación de “países y territorios respaldados”.
Noticias
¿Qué es Codex, el último agente de codificación de IA de OpenAI capaz de multitarea? | Noticias tecnológicas
Published
8 horas agoon
18 mayo, 2025
Operai el viernes 16 de mayo, introdujo una nueva herramienta de IA llamada Codex que está diseñada para manejar múltiples tareas relacionadas con la ingeniería de software al mismo tiempo, desde la generación del código para nuevas funciones hasta responder preguntas sobre la base de código de un usuario, solucionar errores y sugerir solicitudes de revisión del código
La herramienta de codificación basada en la nube y el agente de IA ejecuta estas tareas en su propio entorno de Sandbox en la nube que se ha precargado con el repositorio de código de un usuario.
Codex ha sido publicado bajo Vista previa de investigación. Sin embargo, todos los usuarios de ChatGPT Pro, Enterprise y Team tienen acceso a la herramienta de codificación AI. “Los usuarios tendrán acceso generoso sin costo adicional durante las próximas semanas para que pueda explorar qué puede hacer Codex, después de lo cual lanzaremos el acceso limitado a la tarifa y las opciones de precios flexibles que le permiten comprar un uso adicional a pedido”, dijo Openii en una publicación de blog.
La historia continúa debajo de este anuncio
Los clientes de ChatGPT Plus y EDU recibirán acceso en una fecha posterior, agregó la inicio de IA respaldada por Microsoft.
Hoy estamos presentando Codex.
Es un agente de ingeniería de software que se ejecuta en la nube y hace tareas por usted, como escribir una nueva característica de arreglar un error.
Puede ejecutar muchas tareas en paralelo.
– Sam Altman (@sama) 16 de mayo de 2025
https://platform.twitter.com/widgets.js
La última oferta de Openai llega en un momento en que AI está listo para interrumpir el sector de ingeniería de software, lo que aumenta los temores generalizados del desplazamiento laboral. La CEO de Microsoft, Satya Nadella, dijo recientemente que el 30 por ciento del código de la compañía ahora está generado por IA. Unas semanas más tarde, el gigante de la tecnología anunció que está despidiendo a los 6,000 empleados o al 3 por ciento de su fuerza laboral, y los programadores se han impactado más.
“Todavía sigue siendo esencial que los usuarios revisen y validen manualmente todo el código generado por el agente antes de la integración y la ejecución”, señaló Openai en su publicación de blog de anuncios de Codex.
¿Qué es Codex?
Con Codex, los desarrolladores pueden delegar tareas de programación simples a un agente de IA. Tiene su propia interfaz única a la que se puede acceder desde la barra lateral en la aplicación Web CHATGPT.
La historia continúa debajo de este anuncio
Codex funciona con Codex-1, un modelo AI que es una variación del modelo de razonamiento O3 de OpenAI. Excepto que Codex-1 se ha entrenado específicamente en una amplia gama de tareas de codificación del mundo real para analizar y generar código “que refleja estrechamente el estilo humano y las preferencias de relaciones públicas, se adhiere precisamente a las instrucciones”.
https://www.youtube.com/watch?v=hhhdpnbfh6nu
Sus resultados se han ajustado más bien utilizando el aprendizaje de refuerzo para que Codex-1 pueda “ejecutar las pruebas hasta que reciba un resultado de aprobación”. En términos de rendimiento y precisión, OpenAi dijo que Codex-1 le fue mejor que su modelo O3 AI cuando se evaluó en su punto de referencia SWE interno, así como en el La versión de la empresa validada (Bench SWE verificado).
¿Cómo funciona Codex?
Codex puede leer y editar archivos, así como ejecutar comandos, incluidos arneses de prueba, revestimientos y comprobantes de tipo. Por lo general, lleva entre un minuto a 30 minutos completar una tarea dependiendo del nivel de dificultad, según OpenAI.
El agente de codificación de IA realiza cada tarea en un entorno aislado distinto y aislado que se precarga con la base de código del usuario que sirve como contexto. “Al igual que los desarrolladores humanos, los agentes de Codex funcionan mejor cuando se les proporciona entornos de desarrollo configurados, configuraciones de pruebas confiables y documentación clara”, dijo Openii.
La historia continúa debajo de este anuncio
Los usuarios pueden hacer que el Codex funcione de manera más efectiva para ellos al incluir archivos de agentes.md colocados dentro de su repositorio. “Estos son archivos de texto, similares a ReadMe.md, donde puede informar a Codex cómo navegar por su base de código, que comandan ejecutarse para las pruebas y la mejor manera de cumplir con las prácticas estándar de su proyecto”, dijo Openii.
Otra característica única de Codex es que Muestra su pensamiento y trabajo con cada paso a medida que completa la (s) tarea (s). En el pasado, varios desarrolladores han señalado que los agentes de codificación de IA producen scripts de codificación que no siguen los estándares y son difíciles de depurar.
“Codex proporciona evidencia verificable de sus acciones a través de citas de registros de terminales y salidas de prueba, lo que le permite rastrear cada paso tomado durante la finalización de la tarea”, dijo Openii.
Una vez que Codex completa una tarea, comete sus cambios en su entorno. Sin embargo, los usuarios también pueden revisar los resultados, solicitar más revisiones, abrir una solicitud de extracción de GitHub o realizar directamente cambios en el entorno de desarrollo local.
La historia continúa debajo de este anuncio
¿Cómo usar Codex? ¿Cuáles son sus casos de uso?
Para que Codex comience a generar código, los usuarios deben ingresar un mensaje y hacer clic en ‘Código’. Si desean que los agentes de codificación de IA respondan preguntas o proporcionen sugerencias, entonces los usuarios deben seleccionar la opción ‘Preguntar’ antes de enviar el mensaje.
Cuando OpenAI abrió el acceso temprano a Codex para socios externos, utilizaron la herramienta AI Coding Agent para acelerar el desarrollo de características, los problemas de depuración, escribir y ejecutar pruebas, y refactorizar grandes bases de código. Otro probador temprano utilizó códigos para acelerar las tareas pequeñas pero repetitivas, como mejorar la cobertura de la prueba y la reparación de fallas de integración “.
También se puede utilizar para escribir herramientas de depuración y ayudar a los desarrolladores a comprender partes desconocidas de la base de código al aparecer en el contexto relevante y los cambios pasados.
Los desarrolladores de OpenAI también están utilizando Codex internamente para refactorizar, renombrar y escribir pruebas, así como andamios nuevas características, componentes de cableado, corrección de errores y documentación de redacción.
La historia continúa debajo de este anuncio
“Según los aprendizajes de los primeros evaluadores, recomendamos asignar tareas bien escoltas a múltiples agentes simultáneamente, y experimentar con diferentes tipos de tareas y indicaciones para explorar las capacidades del modelo de manera efectiva”, dijo la compañía.
¿Cuál es la diferencia entre Codex y Codex CLI?
En abril de este año, Openai lanzó otra herramienta de agente de codificación de IA llamada Codex CLI. Se dice que es una herramienta de línea de comandos de código abierto capaz de leer, modificar y ejecutar código localmente en el terminal de un usuario.
El agente de codificación integra los modelos de OpenAI con la interfaz de línea de comandos (CLI) del cliente utilizada para ejecutar programas, administrar archivos y más.
Codex CLI funciona con el último modelo O4-Mini de OpenAI de forma predeterminada. Sin embargo, los usuarios pueden elegir su modelo OperaI preferido a través de la opción API de respuestas. Codex CLI solo puede ejecutarse en sistemas MacOS y Linux por ahora, con soporte para Windows todavía en la etapa experimental.
La historia continúa debajo de este anuncio
https://www.youtube.com/watch?v=o-zfxbfamku
En la publicación del blog del viernes, OpenAI también anunció actualizaciones a Codex CLI. Una versión más pequeña de Codex-1 está llegando a Codex CLI. “Está disponible ahora como el modelo predeterminado en Codex CLI y en la API como Codex-Mini-Latest”, dijo Openii.
La compañía también ha simplificado el proceso de inicio de sesión de desarrolladores para Codex CLI. En lugar de tener que generar y configurar manualmente un token API, los desarrolladores ahora pueden usar su cuenta ChatGPT para iniciar sesión en Codex CLI y seleccionar la organización API que desean usar. “Los usuarios de Plus y Pro que inician sesión en Codex CLI con CHATGPT también pueden comenzar a canjear $ 5 y $ 50 en créditos API gratuitos, respectivamente, más tarde hoy durante los próximos 30 días”, dijo Openii.
Noticias
Cómo los modelos O3 y O4-Mini de OpenAI están revolucionando el análisis visual y la codificación
Published
13 horas agoon
18 mayo, 2025
En abril de 2025, Openai presentó sus modelos más avanzados hasta la fecha, O3 y O4-Mini. Estos modelos representan un gran paso adelante en el campo de la inteligencia artificial (IA), ofreciendo nuevas capacidades en análisis visual y soporte de codificación. Con sus fuertes habilidades de razonamiento y su capacidad para trabajar con texto y imágenes, O3 y O4-Mini pueden manejar una variedad de tareas de manera más eficiente.
El lanzamiento de estos modelos también destaca su impresionante rendimiento. Por ejemplo, O3 y O4-Mini lograron una notable precisión del 92.7% en la resolución de problemas matemáticos en el punto de referencia de AIME, superando el rendimiento de sus predecesores. Este nivel de precisión, combinado con su capacidad para procesar diversos tipos de datos, como código, imágenes, diagramas y más, abre nuevas posibilidades para desarrolladores, científicos de datos y diseñadores de UX.
Al automatizar tareas que tradicionalmente requieren un esfuerzo manual, como la depuración, la generación de documentación e interpretación de datos visuales, estos modelos están transformando la forma en que se construyen aplicaciones impulsadas por la IA. Ya sea en desarrollo, ciencia de datos u otros sectores, O3 y O4-Mini son herramientas poderosas que respaldan la creación de sistemas más inteligentes y soluciones más efectivas, lo que permite a las industrias abordar los desafíos complejos con mayor facilidad.
Avances técnicos clave en modelos O3 y O4-Mini
Los modelos O3 y O4-Mini de OpenAI traen mejoras importantes en la IA que ayudan a los desarrolladores a trabajar de manera más eficiente. Estos modelos combinan una mejor comprensión del contexto con la capacidad de manejar el texto y las imágenes juntos, haciendo que el desarrollo sea más rápido y preciso.
Manejo de contexto avanzado e integración multimodal
Una de las características distintivas de los modelos O3 y O4-Mini es su capacidad para manejar hasta 200,000 tokens en un solo contexto. Esta mejora permite a los desarrolladores ingresar archivos de código fuente completos o grandes bases de código, lo que hace que el proceso sea más rápido y eficiente. Anteriormente, los desarrolladores tenían que dividir grandes proyectos en partes más pequeñas para el análisis, lo que podría conducir a ideas o errores perdidos.
Con la nueva ventana de contexto, los modelos pueden analizar el alcance completo del código a la vez, proporcionando sugerencias, correcciones de error y optimizaciones más precisas y confiables. Esto es particularmente beneficioso para los proyectos a gran escala, donde comprender todo el contexto es importante para garantizar una funcionalidad fluida y evitar errores costosos.
Además, los modelos O3 y O4-Mini aportan el poder de las capacidades multimodales nativas. Ahora pueden procesar las entradas de texto y visuales, eliminando la necesidad de sistemas separados para la interpretación de imágenes. Esta integración permite nuevas posibilidades, como la depuración en tiempo real a través de capturas de pantalla o escaneos de interfaz de usuario, generación de documentación automática que incluye elementos visuales y una comprensión directa de los diagramas de diseño. Al combinar texto y imágenes en un flujo de trabajo, los desarrolladores pueden moverse de manera más eficiente a través de tareas con menos distracciones y retrasos.
Precisión, seguridad y eficiencia a escala
La seguridad y la precisión son fundamentales para el diseño de O3 y O4-Mini. El marco de alineación deliberativa de OpenAI asegura que los modelos actúen en línea con las intenciones del usuario. Antes de ejecutar cualquier tarea, el sistema verifica si la acción se alinea con los objetivos del usuario. Esto es especialmente importante en entornos de alto riesgo como la atención médica o las finanzas, donde incluso pequeños errores pueden tener consecuencias significativas. Al agregar esta capa de seguridad, Operai asegura que la IA funcione con precisión y reduce los riesgos de resultados no deseados.
Para mejorar aún más la eficiencia, estos modelos admiten el encadenamiento de herramientas y las llamadas API paralelas. Esto significa que la IA puede ejecutar múltiples tareas al mismo tiempo, como generar código, ejecutar pruebas y analizar datos visuales, sin tener que esperar a que una tarea finalice antes de comenzar otra. Los desarrolladores pueden ingresar una maqueta de diseño, recibir comentarios inmediatos sobre el código correspondiente y ejecutar pruebas automatizadas mientras la IA procesa el diseño visual y genera documentación. Este procesamiento paralelo acelera los flujos de trabajo, lo que hace que el proceso de desarrollo sea más suave y productivo.
Transformación de flujos de trabajo de codificación con características con IA
Los modelos O3 y O4-Mini introducen varias características que mejoran significativamente la eficiencia del desarrollo. Una característica clave es el análisis de código en tiempo real, donde los modelos pueden analizar instantáneamente capturas de pantalla o escaneos de interfaz de usuario para detectar errores, problemas de rendimiento y vulnerabilidades de seguridad. Esto permite a los desarrolladores identificar y resolver problemas rápidamente.
Además, los modelos ofrecen depuración automatizada. Cuando los desarrolladores encuentran errores, pueden cargar una captura de pantalla del problema, y los modelos identificarán la causa y sugerirán soluciones. Esto reduce el tiempo dedicado a la resolución de problemas y permite a los desarrolladores avanzar con su trabajo de manera más eficiente.
Otra característica importante es la generación de documentación con el contexto. O3 y O4-Mini pueden generar automáticamente documentación detallada que permanece actualizada con los últimos cambios en el código. Esto elimina la necesidad de que los desarrolladores actualicen manualmente la documentación, asegurando que permanezca preciso y actualizado.
Un ejemplo práctico de las capacidades de los modelos está en la integración de API. O3 y O4-Mini pueden analizar las colecciones Postman a través de capturas de pantalla y generar automáticamente asignaciones de punto final API. Esto reduce significativamente el tiempo de integración en comparación con los modelos más antiguos, acelerando el proceso de vinculación de servicios.
Avances en el análisis visual
Los modelos O3 y O4-Mini de OpenAI traen avances significativos en el procesamiento de datos visuales, ofreciendo capacidades mejoradas para analizar imágenes. Una de las características clave es su OCR avanzado (reconocimiento de caracteres ópticos), que permite que los modelos extraen e interpreten el texto de las imágenes. Esto es especialmente útil en áreas como ingeniería de software, arquitectura y diseño, donde los diagramas técnicos, los diagramas de flujo y los planes arquitectónicos son parte integral de la comunicación y la toma de decisiones.
Además de la extracción de texto, O3 y O4-Mini pueden mejorar automáticamente la calidad de las imágenes borrosas o de baja resolución. Utilizando algoritmos avanzados, estos modelos mejoran la claridad de la imagen, asegurando una interpretación más precisa del contenido visual, incluso cuando la calidad de imagen original es subóptima.
Otra característica poderosa es su capacidad para realizar un razonamiento espacial 3D de los planos 2D. Esto permite a los modelos analizar diseños 2D e inferir relaciones 3D, lo que los hace muy valiosos para industrias como la construcción y la fabricación, donde es esencial visualizar espacios físicos y objetos de planes 2D.
Análisis de costo-beneficio: cuándo elegir qué modelo
Al elegir entre los modelos O3 y O4-Mini de OpenAI, la decisión depende principalmente del equilibrio entre el costo y el nivel de rendimiento requerido para la tarea en cuestión.
El modelo O3 es el más adecuado para tareas que exigen alta precisión y precisión. Se destaca en campos como la investigación y el desarrollo complejos (I + D) o aplicaciones científicas, donde son necesarias capacidades de razonamiento avanzado y una ventana de contexto más amplia. La gran ventana de contexto y las poderosas habilidades de razonamiento de O3 son especialmente beneficiosas para tareas como el entrenamiento del modelo de IA, el análisis de datos científicos y las aplicaciones de alto riesgo donde incluso pequeños errores pueden tener consecuencias significativas. Si bien tiene un costo más alto, su precisión mejorada justifica la inversión para las tareas que exigen este nivel de detalle y profundidad.
En contraste, el modelo O4-Mini proporciona una solución más rentable y sigue ofreciendo un rendimiento fuerte. Ofrece velocidades de procesamiento adecuadas para tareas de desarrollo de software a mayor escala, automatización e integraciones de API donde la eficiencia y la velocidad son más críticas que la precisión extrema. El modelo O4-Mini es significativamente más rentable que el O3, que ofrece una opción más asequible para los desarrolladores que trabajan en proyectos cotidianos que no requieren las capacidades avanzadas y la precisión del O3. Esto hace que el O4-Mini sea ideal para aplicaciones que priorizan la velocidad y la rentabilidad sin necesidad de la gama completa de características proporcionadas por el O3.
Para los equipos o proyectos centrados en el análisis visual, la codificación y la automatización, O4-Mini proporciona una alternativa más asequible sin comprometer el rendimiento. Sin embargo, para proyectos que requieren análisis en profundidad o donde la precisión es crítica, el modelo O3 es la mejor opción. Ambos modelos tienen sus fortalezas, y la decisión depende de las demandas específicas del proyecto, asegurando el equilibrio adecuado de costo, velocidad y rendimiento.
El resultado final
En conclusión, los modelos O3 y O4-Mini de OpenAI representan un cambio transformador en la IA, particularmente en la forma en que los desarrolladores abordan la codificación y el análisis visual. Al ofrecer un manejo de contexto mejorado, capacidades multimodales y un razonamiento potente, estos modelos permiten a los desarrolladores a optimizar los flujos de trabajo y mejorar la productividad.
Ya sea para una investigación impulsada por la precisión o tareas rentables de alta velocidad, estos modelos proporcionan soluciones adaptables para satisfacer diversas necesidades. Son herramientas esenciales para impulsar la innovación y resolver desafíos complejos en todas las industrias.
Related posts
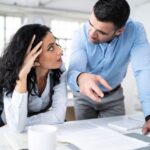
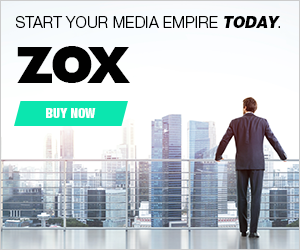

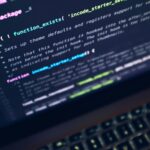
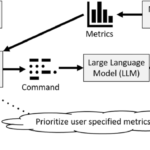
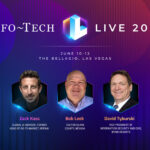
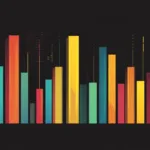
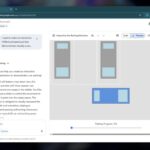
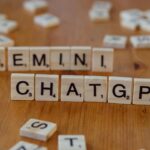
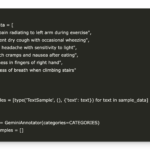
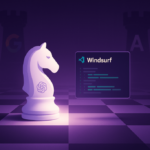
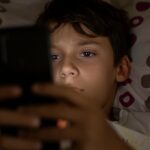
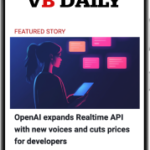
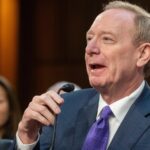
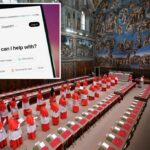
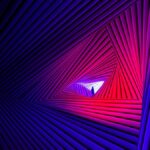
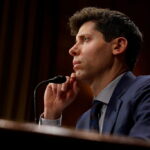
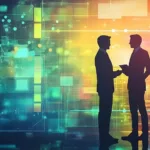
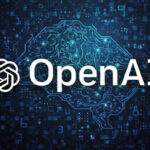
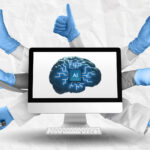
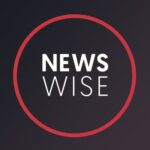
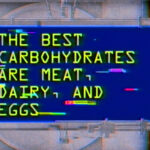
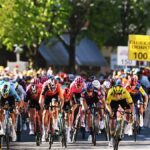
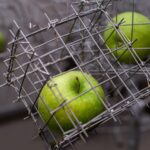
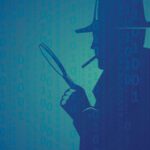
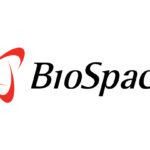
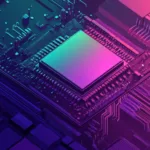
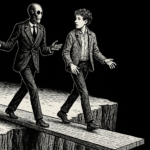
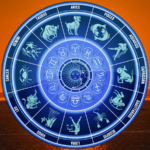
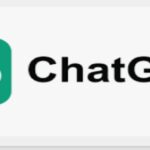
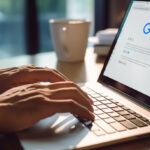
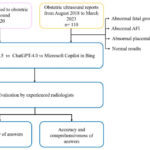
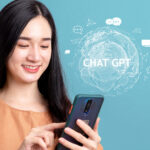
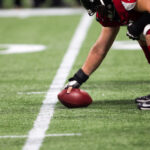
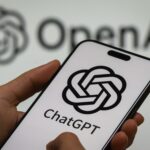
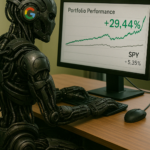
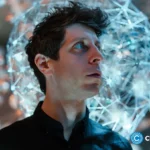
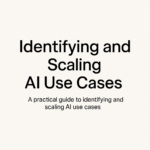
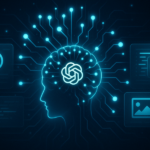
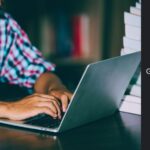
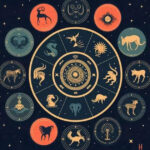
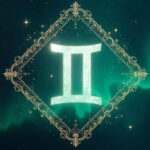
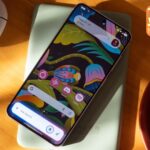
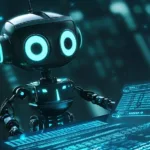
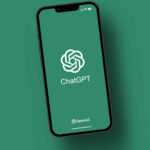
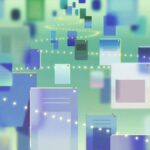
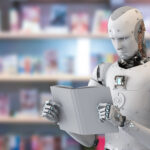
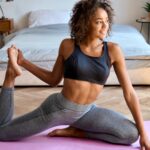
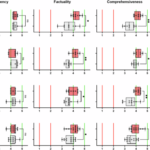
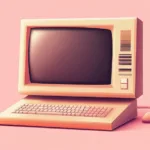
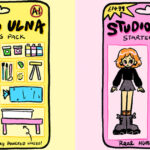
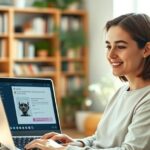
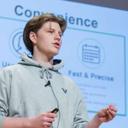
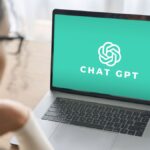
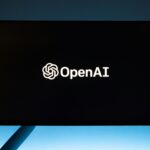
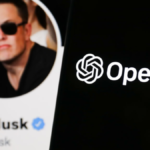
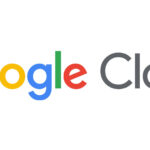
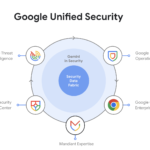
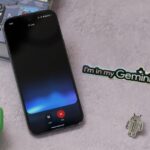
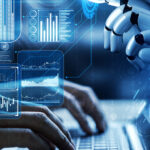
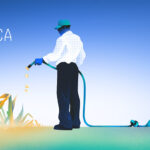
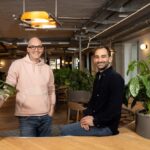
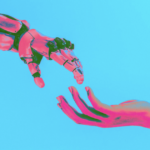
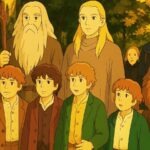
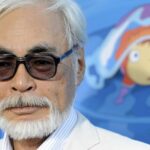
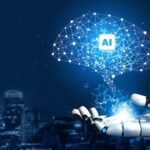
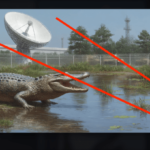
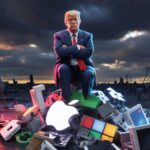
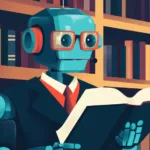
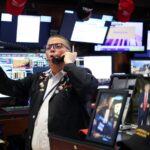
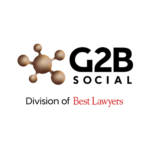
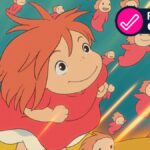
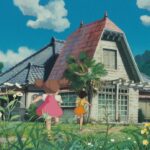
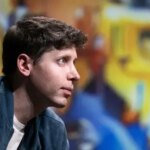
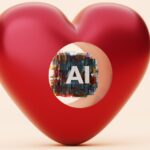
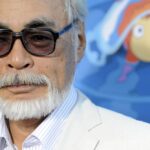
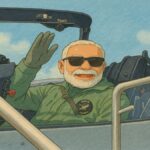
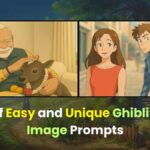
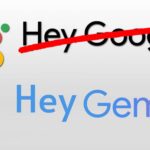
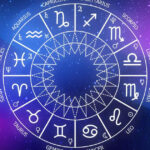
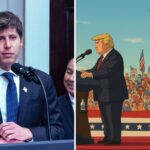
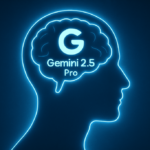
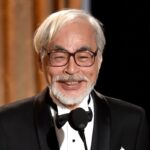
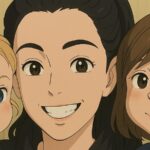
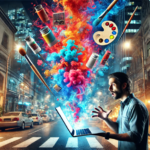
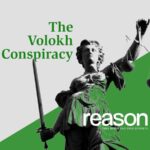
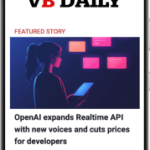
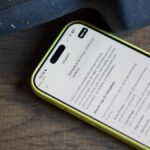
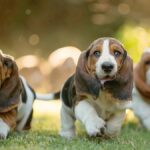
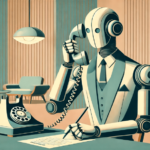
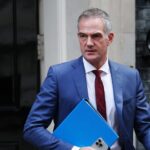
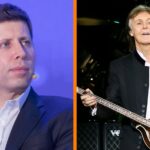
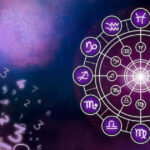
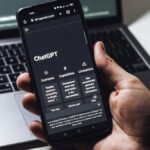
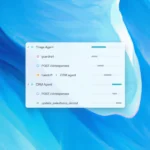
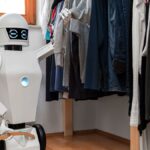
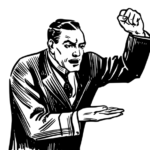
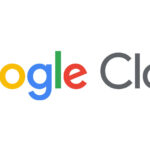
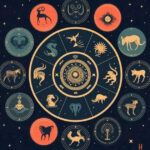
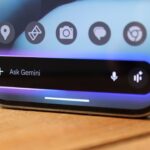
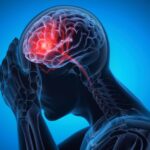
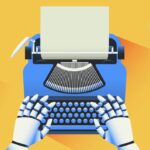
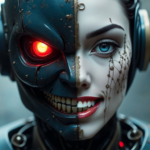
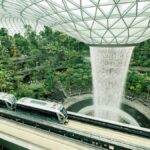
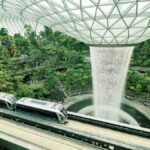
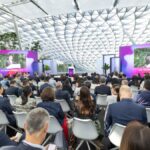
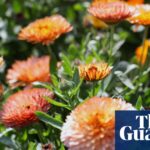

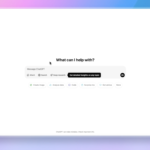
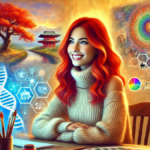
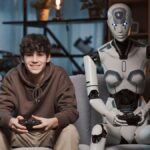
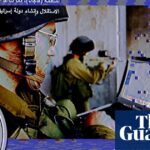
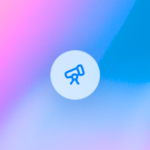
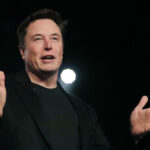
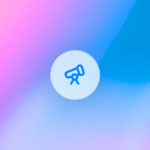
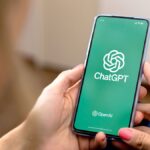
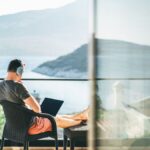
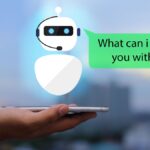
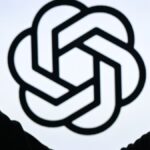
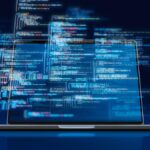
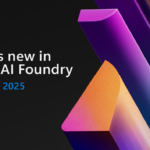
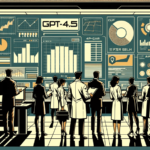
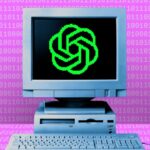
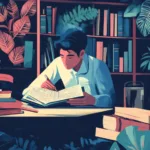
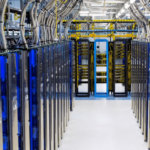
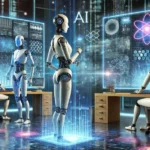
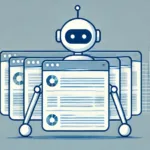
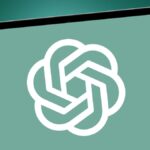
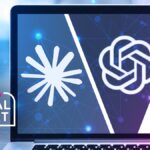
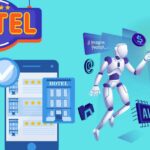
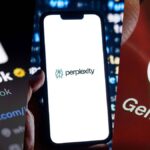
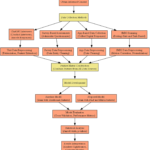
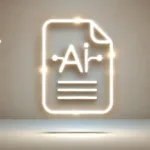
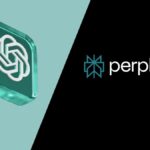
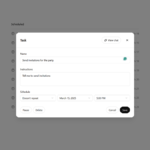
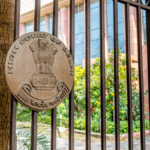
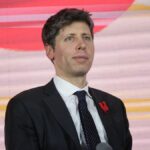
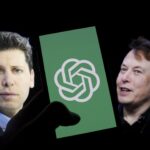
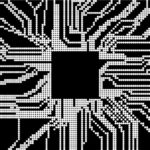
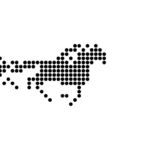
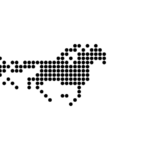
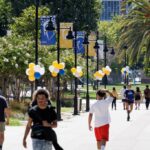
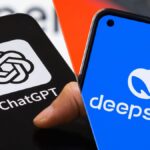
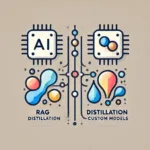
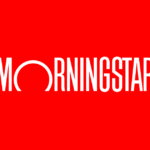
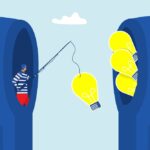
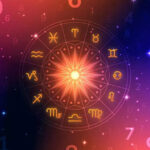
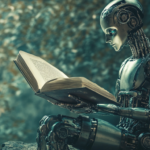
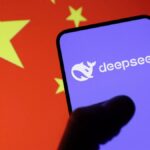
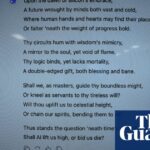
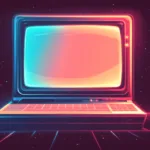

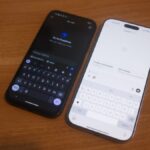
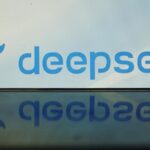

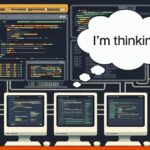
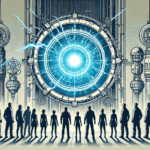
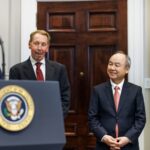
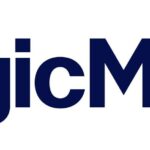
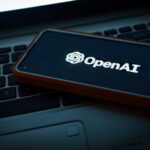

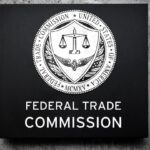
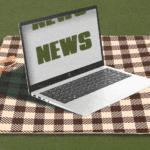
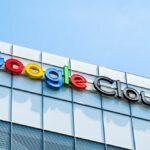
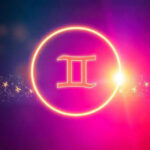
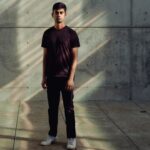
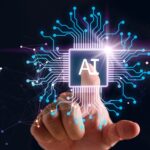
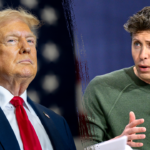
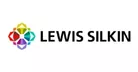
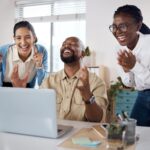
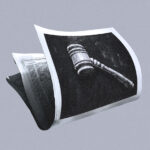
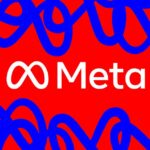

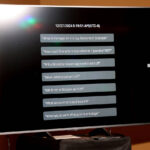

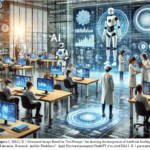
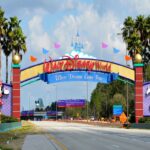
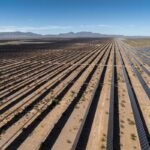
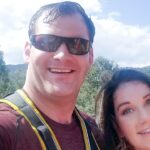
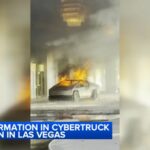
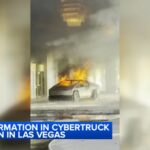
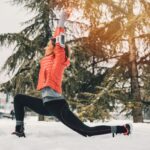
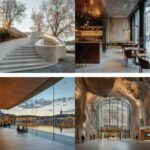
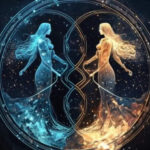
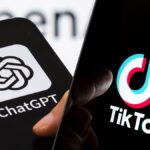
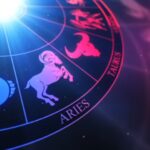
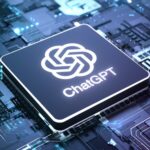
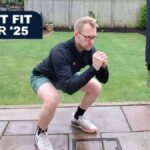
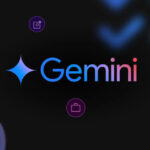
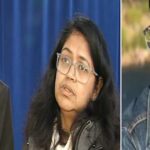
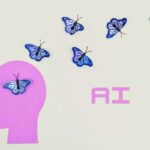
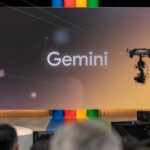
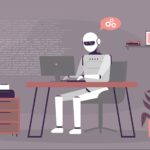
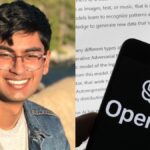
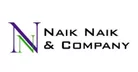

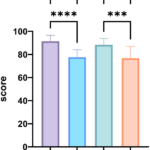
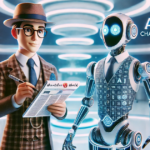
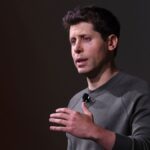
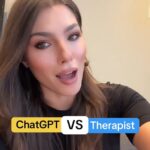
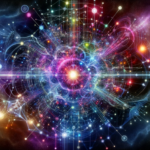
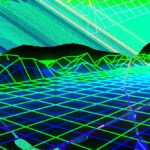
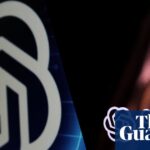
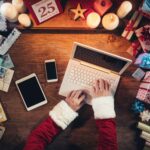
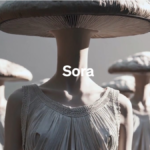
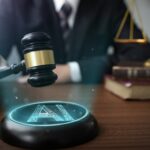
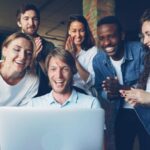
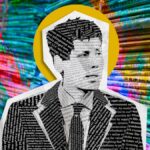

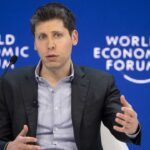
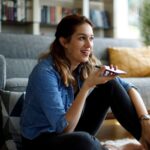
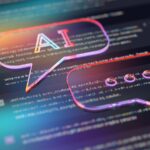
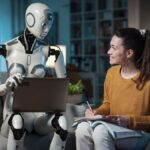
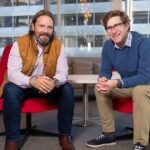
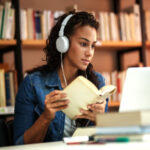
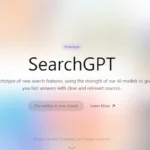
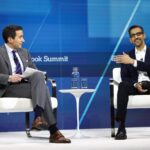
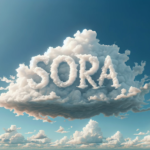
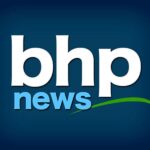
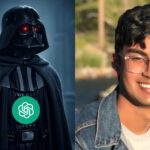
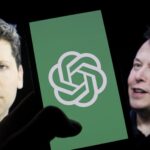
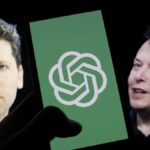
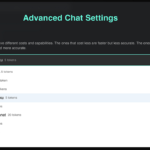
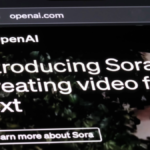
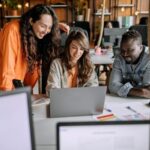
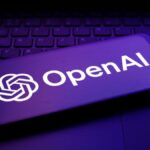
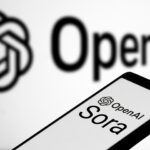
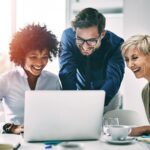
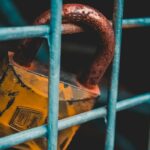
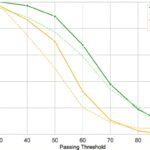
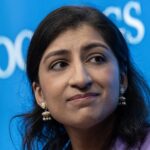
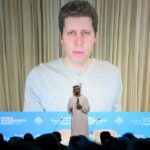
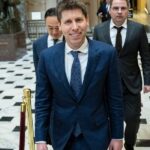
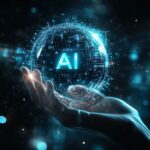
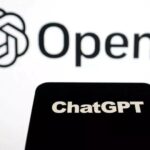
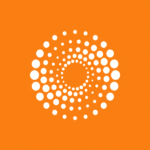
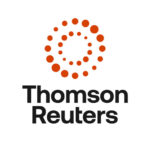
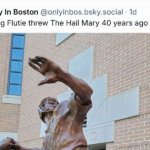

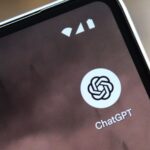
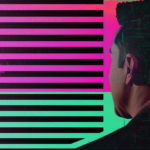
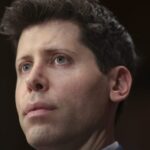
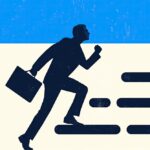
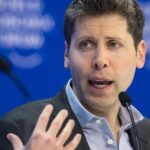
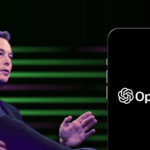
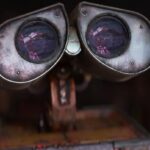
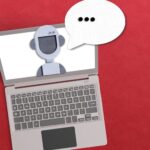
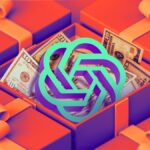
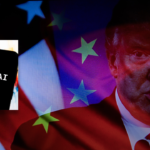
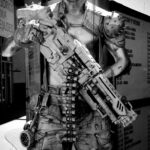
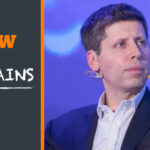
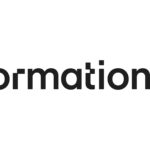
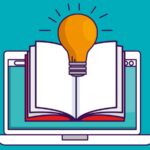
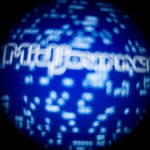
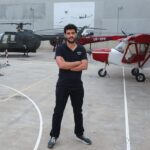
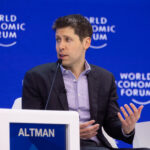
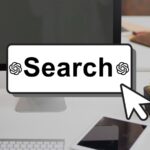
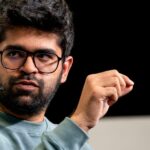
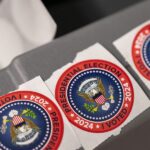
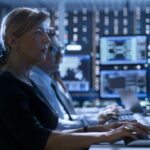
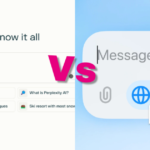
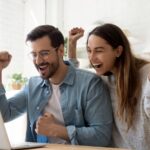
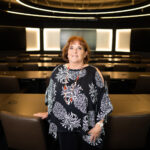
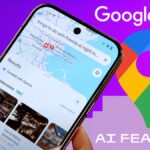
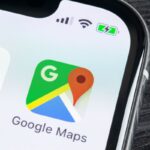
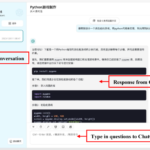
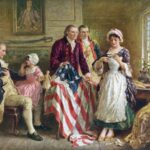
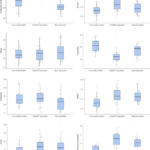

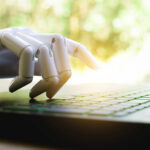
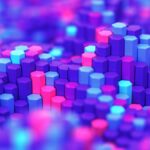
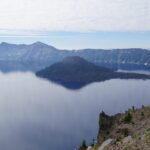
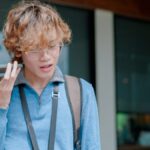
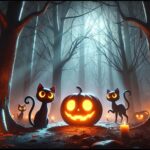
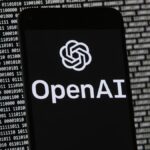
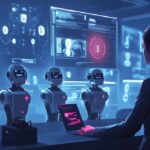
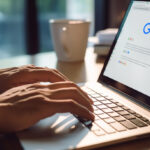
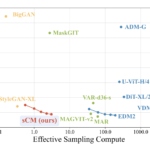
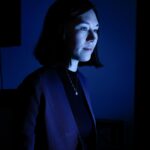
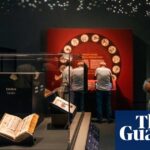
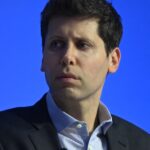
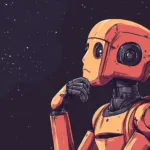
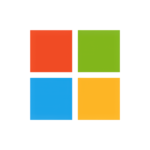
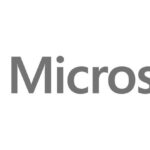
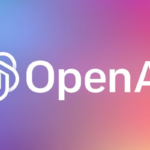
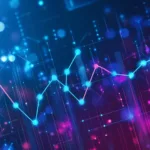
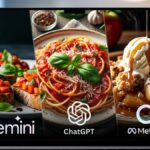
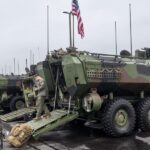

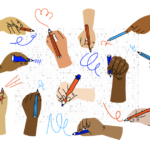
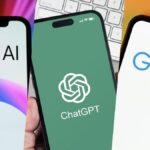
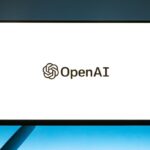
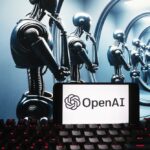
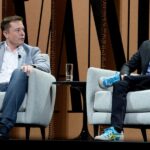
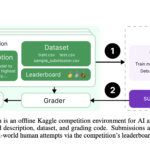
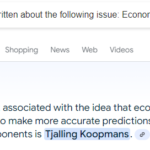
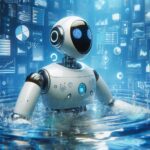
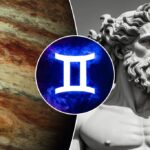
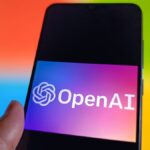
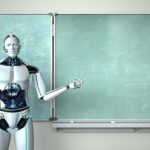
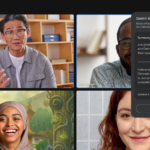
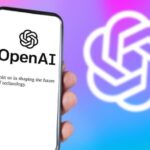
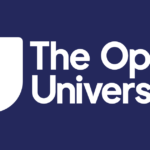
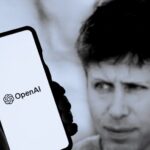
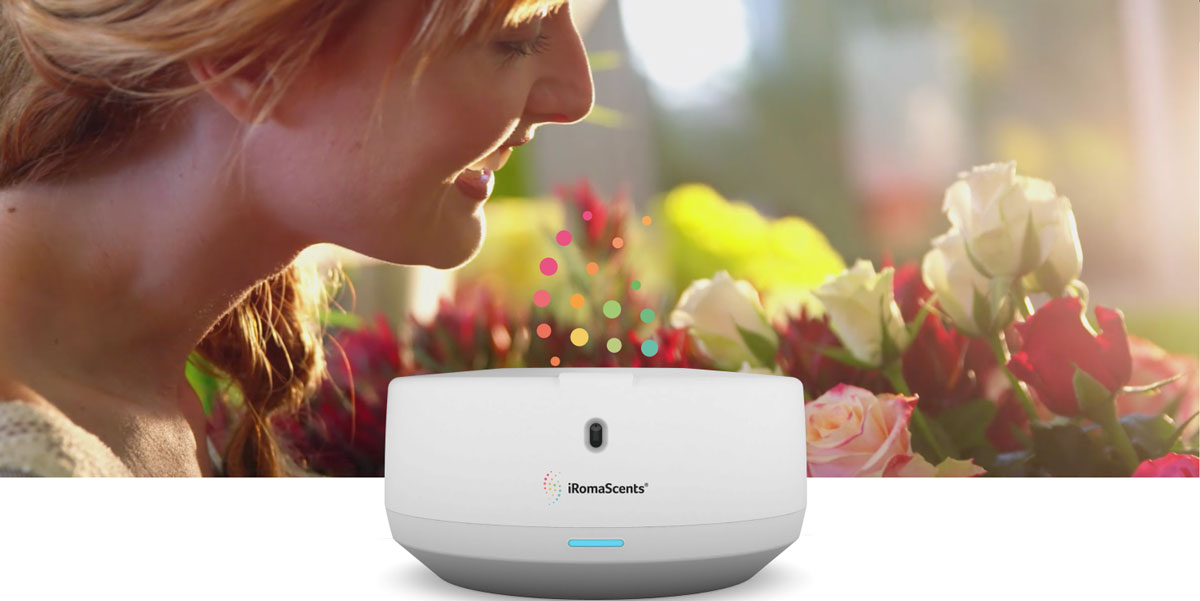

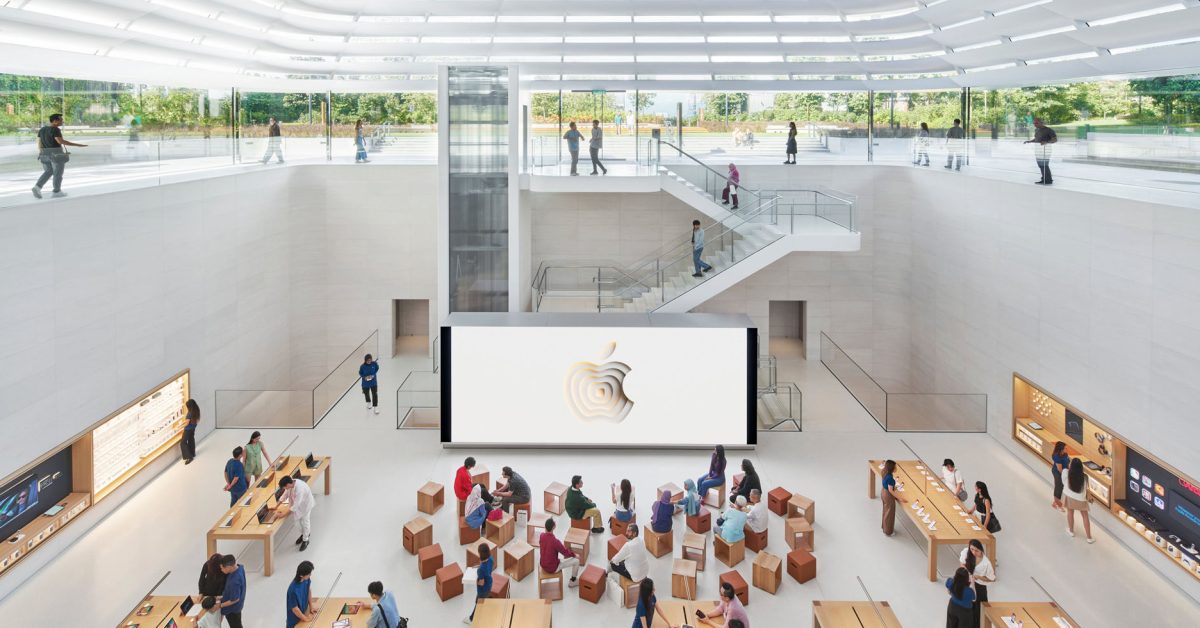


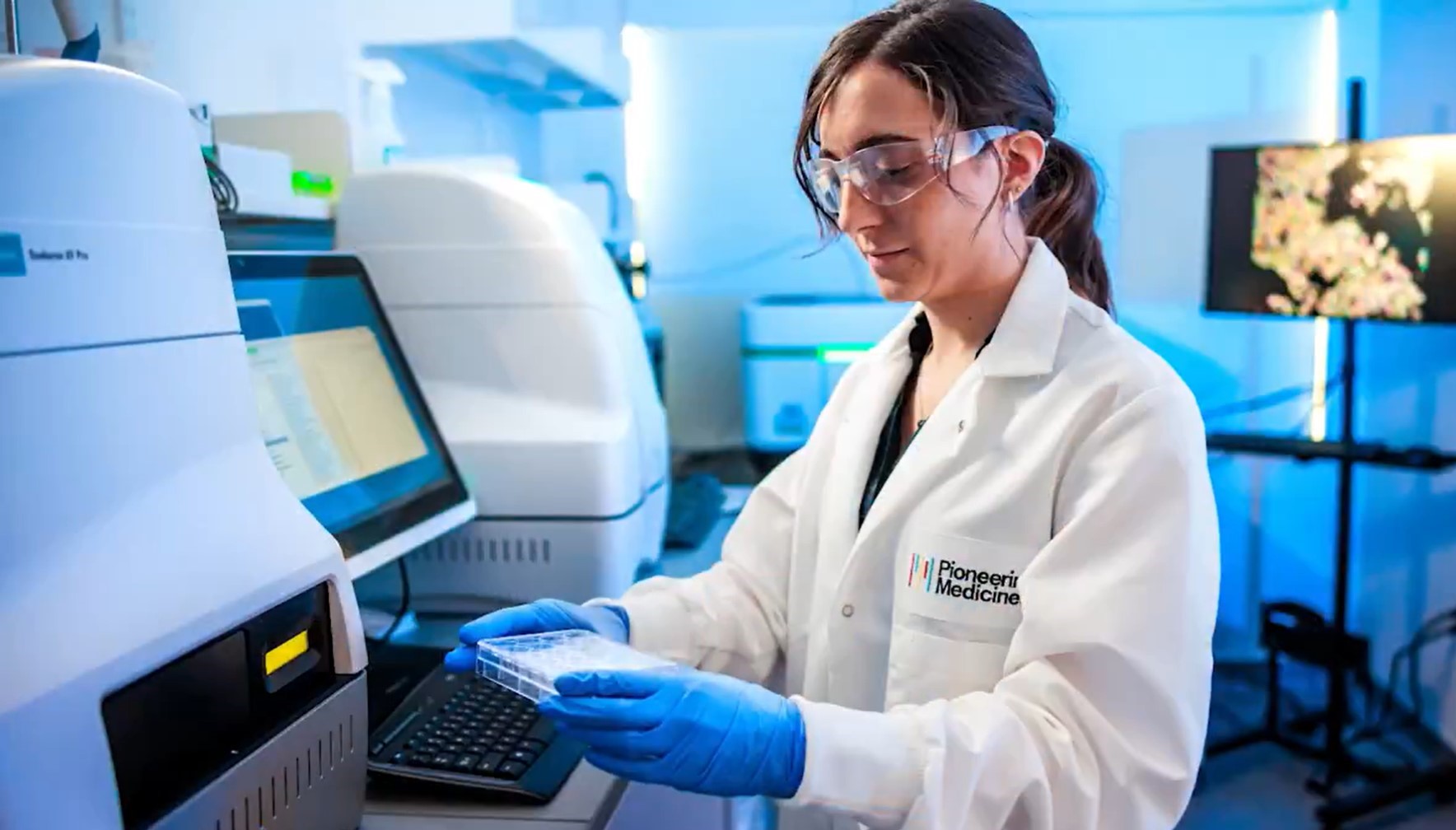
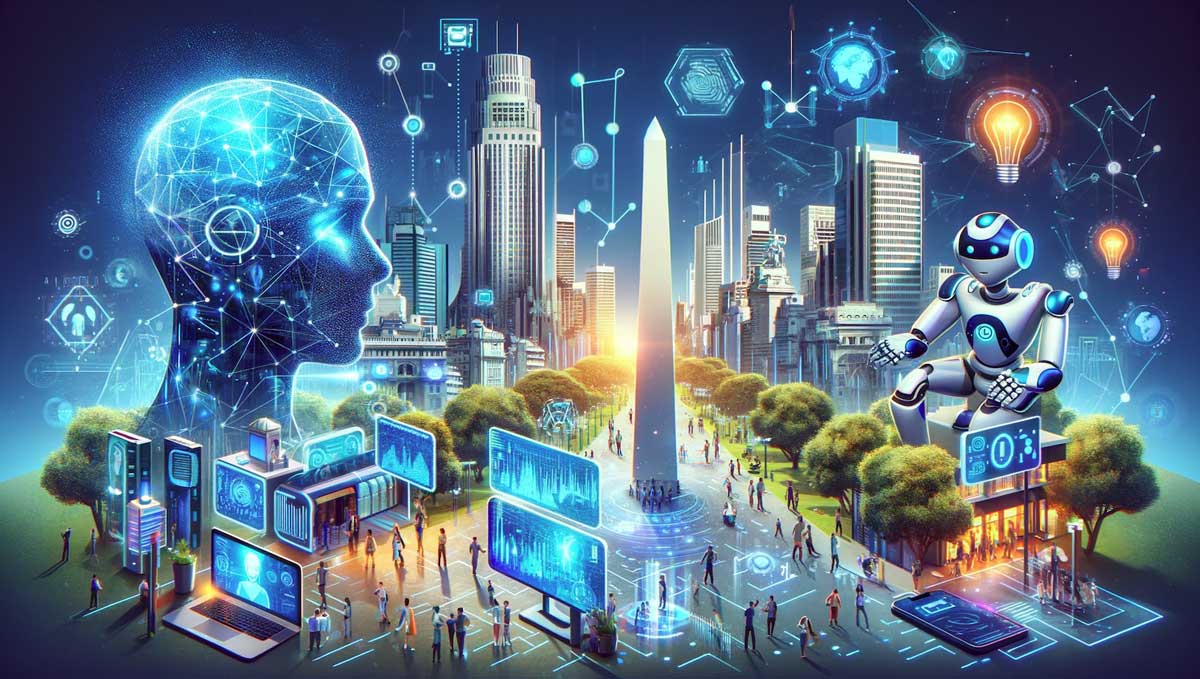

Trending
-
Startups12 meses ago
Remove.bg: La Revolución en la Edición de Imágenes que Debes Conocer
-
Tutoriales1 año ago
Cómo Comenzar a Utilizar ChatGPT: Una Guía Completa para Principiantes
-
Startups10 meses ago
Startups de IA en EE.UU. que han recaudado más de $100M en 2024
-
Recursos1 año ago
Cómo Empezar con Popai.pro: Tu Espacio Personal de IA – Guía Completa, Instalación, Versiones y Precios
-
Startups1 año ago
Deepgram: Revolucionando el Reconocimiento de Voz con IA
-
Recursos12 meses ago
Perplexity aplicado al Marketing Digital y Estrategias SEO
-
Recursos1 año ago
Suno.com: La Revolución en la Creación Musical con Inteligencia Artificial
-
Noticias10 meses ago
Dos periodistas octogenarios deman a ChatGPT por robar su trabajo