Noticias
Sam Altman Reveals This Prior Flaw In OpenAI Advanced AI o1 During ChatGPT Pro Announcement But Nobody Seemed To Widely Notice
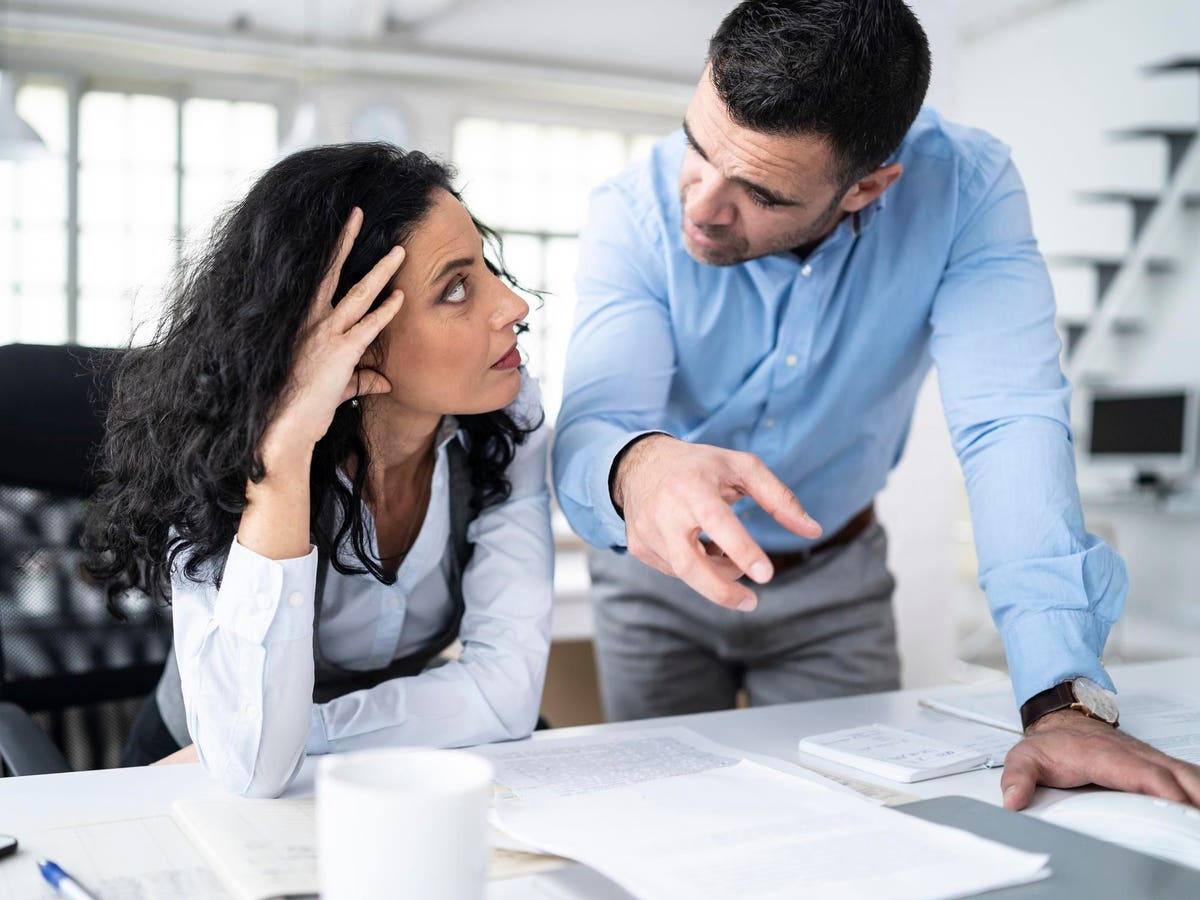
A hidden flaw or inconvenience in OpenAI o1 got recently aired and though fixed it raises … [+]
getty
In today’s column, I examine a hidden flaw in OpenAI’s advanced o1 AI model that Sam Altman revealed during the recent “12 Days Of OpenAI” video-streamed ChatGPT Pro announcement. His acknowledgment of the flaw was not especially noted in the media since he covered it quite nonchalantly in a subtle hand-waving fashion and claimed too that it was now fixed. Whether the flaw or some contend “inconvenience” was even worthy of consideration is another intriguing facet that gives pause for thought about the current state of AI and how far or close we are to the attainment of artificial general intelligence (AGI).
Let’s talk about it.
This analysis of an innovative proposition is part of my ongoing Forbes column coverage on the latest in AI including identifying and explaining various impactful AI complexities (see the link here). For my analysis of the key features and vital advancements in the OpenAI o1 AI model, see the link here and the link here, covering various aspects such as chain-of-thought reasoning, reinforcement learning, and the like.
How Humans Respond To Fellow Humans
Before I delve into the meat and potatoes of the matter, a brief foundational-setting treatise might be in order.
When you converse with a fellow human, you normally expect them to timely respond as based on the nature of the conversation. For example, if you say “hello” to someone, the odds are that you expect them to respond rather quickly with a dutiful reply such as hello, hey, howdy, etc. There shouldn’t be much of a delay in such a perfunctory response. It’s a no-brainer, as they say.
On the other hand, if you ask someone to explain the meaning of life, the odds are that any seriously studious response will start after the person has ostensibly put their thoughts into order. They would presumably give in-depth consideration to the nature of human existence, including our place in the universe, and otherwise assemble a well-thought-out answer. This assumes that the question was asked in all seriousness and that the respondent is aiming to reply in all seriousness.
The gist is that the time to respond will tend to depend on the proffered remark or question.
A presented simple comment or remark involving no weighty question or arduous heaviness ought to get a fast response. The responding person doesn’t need to engage in much mental exertion in such instances. You get a near-immediate response. If the presented utterance has more substance to it, we will reasonably allow time for the other person to undertake a judicious reflective moment. A delay in responding is perfectly fine and fully expected in that case.
That is the usual cadence of human-to-human discourse.
Off-Cadence Timing Of Advanced o1 AI
For those that had perchance made use of the OpenAI o1 AI advanced model, you might have noticed something that was outside of the cadence that I just mentioned. The human-to-AI cadence bordered on being curious and possibly annoying.
The deal was this.
You were suitably forewarned when using o1 that to get the more in-depth answers there would be more extended time after entering a prompt and before getting a response from the AI. Wait time went up. This has to do with the internally added capabilities of advanced AI functionality including chain-of-thought reasoning, reinforcement learning, and so on, see my explanation at the link here. The response latency time had significantly increased.
Whereas in earlier and less advanced generative AI and LLMs we had all gotten used to near instantaneous responses, by and large, there was a willingness to wait longer to get more deeply mined responses via advanced o1 AI. That seems like a fair tradeoff. People will wait longer if they can get better answers. They won’t wait longer if the answers aren’t going to be better than when the response time was quicker.
You can think of this speed-of-response as akin to playing chess. The opening move of a chess game is usually like a flash. Each side quickly makes their initial move and countermove. Later in the game, the time to respond is bound to slow down as each player puts concentrated thoughts into the matter. Just about everyone experiences that expected cadence when playing chess.
What was o1 doing in terms of cadence?
Aha, you might have noticed that when you gave o1 a simple prompt, including even merely saying hello, the AI took about as much time to respond as when answering an extremely complex question. In other words, the response time was roughly the same for the simplest of prompts and the most complicated and deep-diving fully answered responses.
It was a puzzling phenomenon and didn’t conform to any reasonable human-to-AI experience expected cadence.
In coarser language, that dog don’t hunt.
Examples Of What This Cadence Was Like
As an illustrative scenario, consider two prompts, one that ought to be quickly responded to and the other that fairly we would allow more time to see a reply.
First, a simple prompt that ought to lead to a simple and quick response.
- My entered prompt: “Hi.”
- Generative AI response: “Hello, how can I help you?”
The time between the prompt and the response was about 10 seconds.
Next, I’ll try a beefy prompt.
- My entered prompt: “Tell me how all of existence first began, covering all known theories.”
- Generative AI response: “Here is a summary of all available theories on the topic…”
The time for the AI to generate a response to that beefier question was about 12 seconds.
I think we can agree that the first and extremely simple prompt should have had a response time of just a few seconds at most. The response time shouldn’t be nearly the same as when responding to the question about all of human existence. Yet, it was.
Something is clearly amiss.
But you probably wouldn’t have complained since the aspect that you could get in-depth answers was worth the irritating and eyebrow-raising length of wait time for the simpler prompts. I dare say most users just shrugged their shoulders and figured it was somehow supposed to work that way.
Sam Altman Mentioned That This Has Been Fixed
During the ChatGPT Pro announcement, Sam Altman brought up the somewhat sticky matter and noted that the issue had been fixed. Thus, you presumably should henceforth expect a fast response time to simple prompts. And, as already reasonably expected, only prompts requiring greater intensity of computational effort ought to take up longer response times.
That’s how the world is supposed to work. The universe has been placed back into proper balance. Hooray, yet another problem solved.
Few seemed to catch onto his offhand commentary on the topic. Media coverage pretty much skipped past that portion and went straight to the more exciting pronouncements. The whole thing about the response times was likely perceived as a non-issue and not worthy of talking about.
Well, for reasons I’m about to unpack, I think it is worthy to ruminate on.
Turns out there is a lot more to this than perhaps meets the eye. It is a veritable gold mine of intertwining considerations about the nature of contemporary AI and the future of AI. That being said, I certainly don’t want to make a mountain out of a molehill, but nor should we let this opportune moment pass without closely inspecting the gold nuggets that were fortuitously revealed.
Go down the rabbit hole with me, if you please.
Possible Ways In Which This Happened
Let’s take a moment to examine various ways in which the off-balance cadence in the human-to-AI interaction might have arisen. OpenAI considers their AI to be proprietary and they don’t reveal the innermost secrets, ergo I’ll have to put on my AI-analysis detective hat and do some outside-the-box sleuthing.
First, the easiest way to explain things is that an AI maker might decide to hold back all responses until some timer says to release the response.
Why do this?
A rationalization is that the AI maker wants all responses to come out roughly on the same cadence. For example, even if a response has been computationally determined in say 2 seconds, the AI is instructed to keep the response at bay until the time reaches say 10 seconds.
I think you can see how this works out to a seemingly even cadence. A tough-to-answer query might require 12 entire seconds. The response wasn’t ready until after the timer was done. That’s fine. At that juncture, you show the user the response. Only when a response takes less than the time limit will the AI hold back the response.
In the end, the user would get used to seeing all responses arising at above 10 seconds and fall into a mental haze that no matter what happens, they will need to wait at least that long to see a response. Boom, the user is essentially being behaviorally trained to accept that responses will take that threshold of time. They don’t know they are being trained. Nothing tips them to this ruse.
Best of all, from the AI maker’s perspective, no one will get upset about timing since nothing ever happens sooner than the hidden limit anyway. Elegant and the users are never cognizant of the under-the-hood trickery.
The Gig Won’t Last And Questions Will Be Asked
The danger for the AI maker comes to the fore when software sophisticates start to question the delays. Any proficient software developer or AI specialist would right away be suspicious that the simplest of entries is causing lengthy latency. It’s not a good look. Insiders begin to ask what’s up with that.
If a fake time limit is being used, that’s often frowned upon by insiders who would shame those developers undertaking such an unseemly route. There isn’t anything wrong per se. It is more of a considered low-brow or discreditable act. Just not part of the virtuous coding sense of ethos.
I am going to cross out that culprit and move toward a presumably more likely suspect.
It goes like this.
I refer to this other possibility as the gauntlet walk.
A brief tale will suffice as illumination. Imagine that you went to the DMV to get up-to-date license tags for your car. In theory, if all the paperwork is already done, all you need to do is show your ID and they will hand you the tags. Some modernized DMVs have an automated kiosk in the lobby that dispenses tags so that you can just scan your ID and viola, you instantly get your tags and walk right out the door. Happy face.
Sadly, some DMVs are not yet modernized. They treat all requests the same and make you wait as though you were there to have surgery done. You check in at one window. They tell you to wait over there. Your name is called, and you go to a pre-processing window. The agent then tells you to wait in a different spot until your name is once again called. At the next processing window, they do some of the paperwork but not all of it. On and on this goes.
The upshot is that no matter what your request consists of you are by-gosh going to walk the full gauntlet. Tough luck to you. Live with it.
A generative AI app or large language model (LLM) could be devised similarly. No matter what the prompt contains, an entire gauntlet of steps is going to occur. Everything must endure all the steps. Period, end of story.
In that case, you would typically have responses arriving outbound at roughly the same time. This could vary somewhat because the internal machinery such as the chain of thought mechanism is going to pass through the tokens without having to do nearly the same amount of computational work, see my explanation at the link here. Nonetheless, time is consumed even when the content is being merely shunted along.
That could account for the simplest of prompts taking much longer than we expect them to take.
How It Happens Is A Worthy Question
Your immediate thought might be why in the heck would a generative AI app or LLM be devised to treat all prompts as though they must walk the full gauntlet. This doesn’t seem to pass the smell test. It would seem obvious that a fast path like at Disneyland should be available for prompts that don’t need the whole kit-and-kaboodle.
Well, I suppose you could say the same about the DMV. Here’s what I mean. Most DMVs were probably set up without much concern toward allowing multiple paths. The overall design takes a lot more contemplation and building time to provide sensibly shaped forked paths. If you are in a rush to get a DMV underway, you come up with a single path that covers all the bases. Therefore, everyone is covered. Making everyone wait the same is okay because at least you know that nothing will get lost along the way.
Sure, people coming in the door who have trivial or simple requests will need to wait as long as those with the most complicated of requests, but that’s not something you need to worry about upfront. Later, if people start carping about the lack of speediness, okay, you then try to rejigger the process to allow for multiple paths.
The same might be said for when trying to get advanced AI out the door. You are likely more interested in making sure that the byzantine and innovative advanced capabilities work properly, versus whether some prompts ought to get the greased skids.
A twist to that is the idea that you are probably more worried about maximum latencies than you would be about minimums. This stands to reason. Your effort to optimize is going to focus on trying to keep the AI from running endlessly to generate a response. People will only wait so long to get a response, even for highly complex prompts. Put your elbow grease toward the upper bounds versus the lower bounds.
The Tough Call On Categorizing Prompts
An equally tough consideration is exactly how you determine which prompts are suitably deserving of quick responses.
Well, maybe you just count the number of words in the prompt.
A prompt with just one word would seem unlikely to be worthy of the full gauntlet. Let it pass through or maybe skip some steps. This though doesn’t quite bear out. A prompt with a handful of words might be easy-peasy, while another prompt with the same number of words might be a doozy. Keep in mind that prompts consist of everyday natural language, which is semantically ambiguous, and you can open a can of worms with just a scant number of words.
This is not like sorting apples or widgets.
All in all, a prudent categorization in this context cannot do something blindly such as purely relying on the number of words. The meaning of the prompt comes into the big picture. A five-word prompt that requires little computational analysis is likely only discerned as a small chore by determining what the prompt is all about.
Note that this means you indubitably have to do some amount of initial processing to gauge what the prompt constitutes. Once you’ve got that first blush done, you can have the AI flow the prompt through the other elements with a kind of flag that indicates this is a fly-by-night request, i.e., work on it quickly and move it along.
You could also establish a separate line of machinery for the short ones, but that’s probably more costly and not something you can concoct overnight. DMVs often kept the same arrangement inside the customer-facing processing center and merely adjusted by allowing the skipping of windows. Eventually, newer avenues were developed such as the use of automated kiosks.
Time will tell in the case of AI.
There is a wide variety of highly technical techniques underlying prompt-assessment and routing issues, which I will be covering in detail in later postings so keep your eyes peeled. Some of the techniques are:
- (1) Prompt classification and routing
- (2) Multi-tier model architecture
- (3) Dynamic attention mechanisms
- (4) Adaptive token processing
- (5) Caching and pre-built responses
- (6) Heuristic cutoffs for contextual expansion
- (7) Model layer pruning on demand
I realize that seems relatively arcane. Admittedly, it’s one of those inside baseball topics that only heads-down AI researchers and developers are likely to care about. It is a decidedly niche aspect of generative AI and LLMs. In the same breath, we can likely agree that it is an important arena since people aren’t likely to use models that make them wait for simple prompts.
AI makers that seek widespread adoption of their AI wares need to give due consideration to the gauntlet walk problem.
Put On Your Thinking Cap And Get To Work
A few final thoughts before finishing up.
The prompt-assessment task is crucial in an additional fashion. The AI could inadvertently arrive at false positives and false negatives. Here’s what that foretells. Suppose the AI assesses that a prompt is simple and opts to therefore avoid full processing, but then the reality is that the answer produced is insufficient and the AI misclassified the prompt.
Oops, a user gets a shallow answer.
They are irked.
The other side of the coin is not pretty either. Suppose the AI assesses that a prompt should get the full treatment, shampoo and conditioner included, but essentially wastes time and computational resources such that the prompt should have been categorized as simple. Oops, the user waited longer than they should have, plus they paid for computational resources they needn’t have consumed.
Awkward.
Overall, prompt-assessment must strive for the Goldilocks principle. Do not be too cold or too hot. Aim to avoid false positives and false negatives. It is a dicey dilemma and well worth a lot more AI research and development.
My final comment is about the implications associated with striving for artificial general intelligence (AGI). AGI is considered the aspirational goal of all those pursuing advances in AI. The belief is that with hard work we can get AI to be on par with human intelligence, see my in-depth analysis of this at the link here.
How do the prompt-assessment issue and the vaunted gauntlet walk relate to AGI?
Get yourself ready for a mind-bending reason.
AGI Ought To Know Better
Efforts to get modern-day AI to respond appropriately such that simple prompts get quick response times while hefty prompts take time to produce are currently being devised by humans. AI researchers and developers go into the code and make changes. They design and redesign the processing gauntlet. And so on.
It seems that any AGI worth its salt would be able to figure this out on its own.
Do you see what I mean?
An AGI would presumably gauge that there is no need to put a lot of computational mulling toward simple prompts. Most humans would do the same. Humans interacting with fellow humans would discern that waiting a long time to respond is going to be perceived as an unusual cadence when in discourse covering simple matters. Humans would undoubtedly self-adjust, assuming they have the mental capacity to do so.
In short, if we are just a stone’s throw away from attaining AGI, why can’t AI figure this out on its own? The lack of AI being able to self-adjust and self-reflect is perhaps a telltale sign. The said-to-be sign is that our current era of AI is not on the precipice of becoming AGI.
Boom, drop the mic.
Get yourself a glass of fine wine and find a quiet place to reflect on that contentious contention. When digging into it, you’ll need to decide if it is a simple prompt or a hard one, and judge how fast you think you can respond to it. Yes, indeed, humans are generally good at that kind of mental gymnastics.
Noticias
Lo que se puso bien y mal
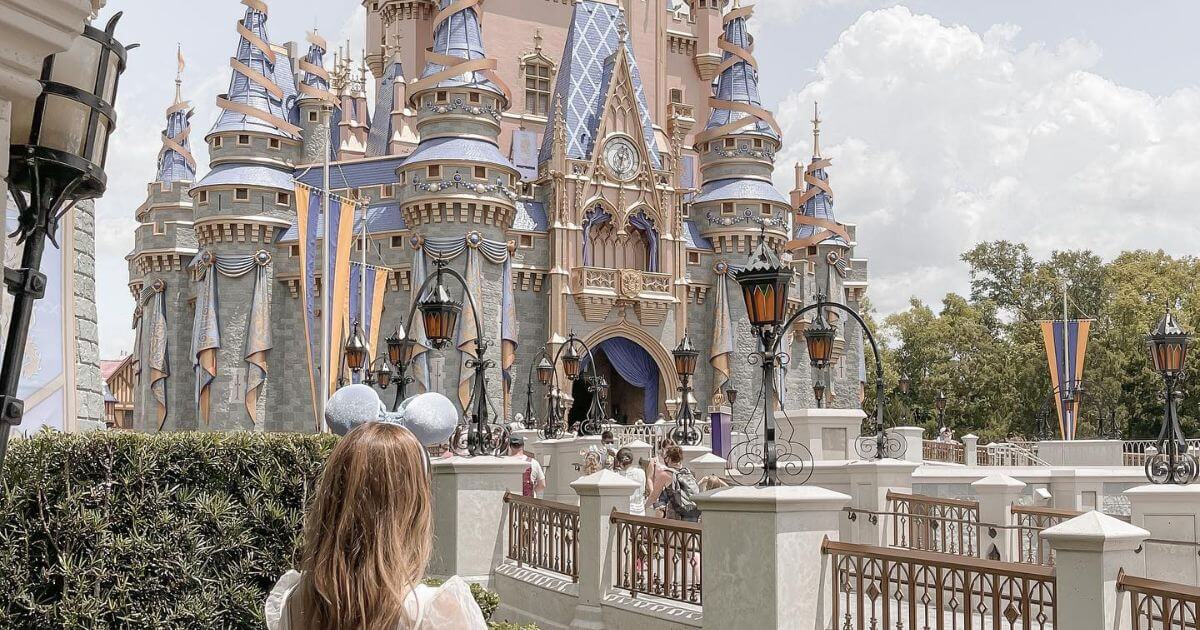
Han pasado casi 30 años desde que fui a Disney World. Mis recuerdos de Disney son felices, pero no recuerdo ningún detalle más allá de usar oídos, hacer que los personajes firmen mi libro especial de autógrafos y permanezcan despierto hasta tarde para ver el espectáculo de fuegos artificiales en Epcot.
Tengo dos hijas, casi 4.5 y 2.5, que están obsesionados con las princesas, por lo que cuando descubrí que mi familia estaría en Orlando durante unos días en junio, decidí buscar ir a Disney World por el día. Haremos un viaje más grande de Disney World en un par de años, pero los niños menores de 3 años son gratuitos (una de las pocas cosas que sabía sobre Disney), así que pensé que aprovecharíamos eso y les daríamos una gran sorpresa.
El único problema es que pensar en planificar un día en Disney es abrumador. Hay Tanta información Acerca de cómo optimizar su tiempo en los parques.
Decidí pedirle a ChatGPT que planifique mi día, y luego tuve a Mary Helen Law, propietaria de la compañía de planificación de Disney Minnie Mouse Counselors y uno de los principales especialistas en viajes de Conde Nast Traveler, revise el itinerario. Siga leyendo para escuchar qué chatgpt se hizo bien y mal y qué tenía que decir un experto en Disney.
Conocer al experto
Mary Helen Law, fundador de Mini Mouse Counselores
Mary Helen es una madre y experta en viajes. Comenzó su carrera como agente de viajes en 2018 mientras trabajaba en marketing y desarrollo de negocios. En 2019 decidió dejar su trabajo diario para expandir su negocio. Desde entonces, ha ayudado a cientos de familias a planificar vacaciones mágicas en todo el mundo y es uno de los principales especialistas en viajes de Conde Nast Traveler.
My Disney World Chatgpt Planning de planificación
Primero, aquí está el aviso que le di a Chatgpt para crear nuestro itinerario de Disney World:
¿Puedes planificar el día de mi familia en Disney World? Seremos yo, mi esposo y mis dos hijas. Serán 2.5 y 4.5 para el viaje, y aman a Ariel, Elsa y Ana, Moana, Belle, 101 Dalmatians, Cenicienta y Mary Poppins.
Nos gustaría ir a dos parques diferentes en el transcurso del día, pero necesitaremos un descanso de tres a cuatro horas en la mitad del día para una siesta. Nos gustaría hacer un almuerzo sentado en un restaurante temático que nuestras niñas les gustaría en función de sus intereses. ¿Puede planificar un itinerario para el día para los parques que recomendaría? Además, debe haber una parada de bocadillos por la mañana y la tarde.
¿Qué chatgpt hizo lo correcto sobre la planificación de un viaje a Disney World?
Hay muchas cosas que ChatGPT se equivocó sobre la planificación de un viaje a Disney (más sobre eso en un momento), pero sí recomendó paseos y actividades que encajarían bien en función de los intereses de mis hijas, como ir al viaje “Under the Sea” y conocer a Ariel, ver “cuentos encantados con Belle”, con un almuerzo en el restaurante de invitados y ver la festival de la fantasía de Magic Kingdom.
Cuando mi hermana usó un planificador de Disney el año pasado, tuvo la experiencia opuesta. El planificador acaba de recomendar todos los paseos más populares, como Tron, en el que mi sobrino no habría tenido interés, por lo que al menos Chatgpt prestó atención a lo que le dije que le gustaban a mis chicas.
También le pregunté a ChatGPT si tenía algún consejo para tener un día exitoso en Disney, y obtuve una buena información, como usar la aplicación de Disney para verificar los tiempos de espera de viaje y pedir comida con anticipación, y que podríamos usar el programa Rider Switch en caso de que mi hijo menor fuera demasiado pequeño para viajar.
También me dio algunas recomendaciones excelentes sobre qué empacar para el día, como protector solar, toallitas para bebés y bocadillos. Law estuvo de acuerdo en que había algunas pepitas de buena información, pero señaló que ChatGPT no incluía empacar un cargador de teléfono portátil, algo que dijo que necesitaríamos.
Qué chatgpt se equivocó sobre nuestro itinerario del día de Disney
Tres cosas principales para recordar sobre ChatGPT es que solo responde a lo que le da, se está retirando de la información en Internet y puede que no siempre sea correcto, y tampoco hay un elemento humano para ayudar a racionalizar la información.
Por ejemplo, le dije a ChatGPT que quería ir a dos parques, por lo que me dio un itinerario basado en ese aviso. Nunca hubiera sugerido que no haga dos parques porque sería poco realista dadas las edades de mis hijos.
ChatGPT carece de la capacidad de decir que no o sugerir ideas alternativas
Chatgpt hizo lo que le pedí, pero si hubiera abrazado las sugerencias, supongo que nunca habríamos regresado al parque después de una siesta y está muy frustrado.
Law, por otro lado, echó un vistazo a mi aviso y me dijo que realmente recomendaría no saltar en el parque y que deberíamos quedarnos en Magic Kingdom todo el día versus tratar de irme y volver.
Law me explicó que debido a que no nos quedamos en un resort de Disney, pasaremos mucho más tiempo pasando del estacionamiento a los parques, y que mi estimación de 30 minutos probablemente fue más como una hora y media. ChatGPT no sabe cuánto tiempo lleva llegar al estacionamiento y regresar a un hotel y no pudo estimar con precisión la logística detrás de esto.
También recomendó una siesta de cochecito en el carrusel de progreso con aire acondicionado, que según ella generalmente era un lugar más tranquilo, en lugar de tratar de irse y volver al parque. ChatGPT también recomendó este lugar y el Salón de Presidentes actualmente cerrado como un gran lugar para tomar un descanso, pero en general necesitaba un humano con más conocimiento de cómo funcionan las cosas en Disney para ayudarme a entender lo que era realista en lugar de no para nuestro viaje.
Chatgpt no incluyó ningún tiempo de espera para los paseos
Si nos fijamos en el itinerario que Chatgpt me dio por Disney, es como si tuviéramos el parque para nosotros mismos. Según ChatGPT, estaríamos en camino o en una nueva atracción cada 30 minutos.
Incluso sé lo suficiente sobre Disney para saber que eso no sonó bien. Law dijo que probablemente estaríamos en el extremo inferior de los tiempos de espera desde que iremos a principios de junio, pero acordamos que la cantidad de cosas que el itinerario dijo que logramos no parecía realista.
En cambio, ella me acompañó a través de la aplicación de Disney y me mostró cómo podré ver cuáles son los tiempos de espera para cada viaje, cuáles son los tiempos de show y cómo ver qué personajes están.
También me habló de las otras formas en que podemos reducir los tiempos de espera comprando pases de rayos o el pase Premier, que es un programa más nuevo (aunque costoso) que Disney está probando que le da una entrada a cada experiencia de Lightning Lane.
Usar ChatGPT sería excelente para preguntar qué paseos serían apropiados para mis niñas en función de su edad e intereses para que tengamos una idea de qué apuntar durante todo el día, pero la información sobre cómo usar la aplicación para ahorrar tiempo que la ley me dio será mucho más útil. También ayudó a establecer el nivel de mis expectativas sobre lo que podremos lograr en un día, lo que me ayudará a no estresarse por no poder hacerlo todo una vez que lleguemos allí.
Chatgpt se equivocó con cosas importantes que habrían arruinado nuestro día en Disney
Recuerde, soy un novato en Disney, así que tomé toda la información que me dio al pie de la letra.
El problema, dice Law, es que “ChatGPT simplemente no puede mantenerse al día con la cantidad que cambia Disney”. Se extrae de fuentes en todo Internet y no puede discernir lo que es correcto o no, así que terminé con cosas en el itinerario que no son precisos.
¿Uno de los mayores errores? El itinerario dijo que podríamos conocer a Ana y Elsa, los personajes favoritos de mis niñas, en el Princess Fairytale Hall, que no es cierto. Se encuentran y saludan en Epcot en el Royal Sommerhus.
Law sintió mi decepción y me aseguró que las chicas podrían saludar a Ana, Elsa y Olaf en la feria de amistad mágica de Mickey o en el desfile de Magic Kingdom.
¿Otras cosas importantes que Chatgpt se equivocó que habría descarrilado nuestro día? Sugirió conocer a Ariel a las 9 am cuando no está disponible hasta las 10 de la mañana; dijo que podríamos ingresar al parque a las 8 a.m., lo cual es incorrecto teniendo en cuenta que Magic Kingdom abre a las 8:30 a.m. para las personas que permanecen en la propiedad y las 9 a.m. para las personas que se mantienen fuera de la propiedad; y dijo que deberíamos usar Genie+ o un paso rápido para reducir los tiempos de espera, los cuales son servicios que ya no existen.
Es fácil suponer que lo que ChatGPT escupe es exacto, pero en nuestro caso todos estos errores habrían causado una frustración significativa para el día.
¿Debería usar ChatGPT para cualquier parte de su planificación de Disney?
Law dijo que podía ver que ChatGPT era útil para “cosas de espectro muy amplio” al planificar un viaje a Disney, como recomendaciones para qué recurre para quedarse o tener una idea general de qué personajes son los parques (aunque tenga en cuenta, ChatGPT me dio información incorrecta sobre esto).
“Creo que hay mucha seguridad laboral en lo que [travel planners] Haga por las relaciones que tenemos y el conocimiento “, dice, pero dice que no cree que sea una mala idea usar ChatGPT para obtener algunas ideas iniciales antes de hablar con un planificador.
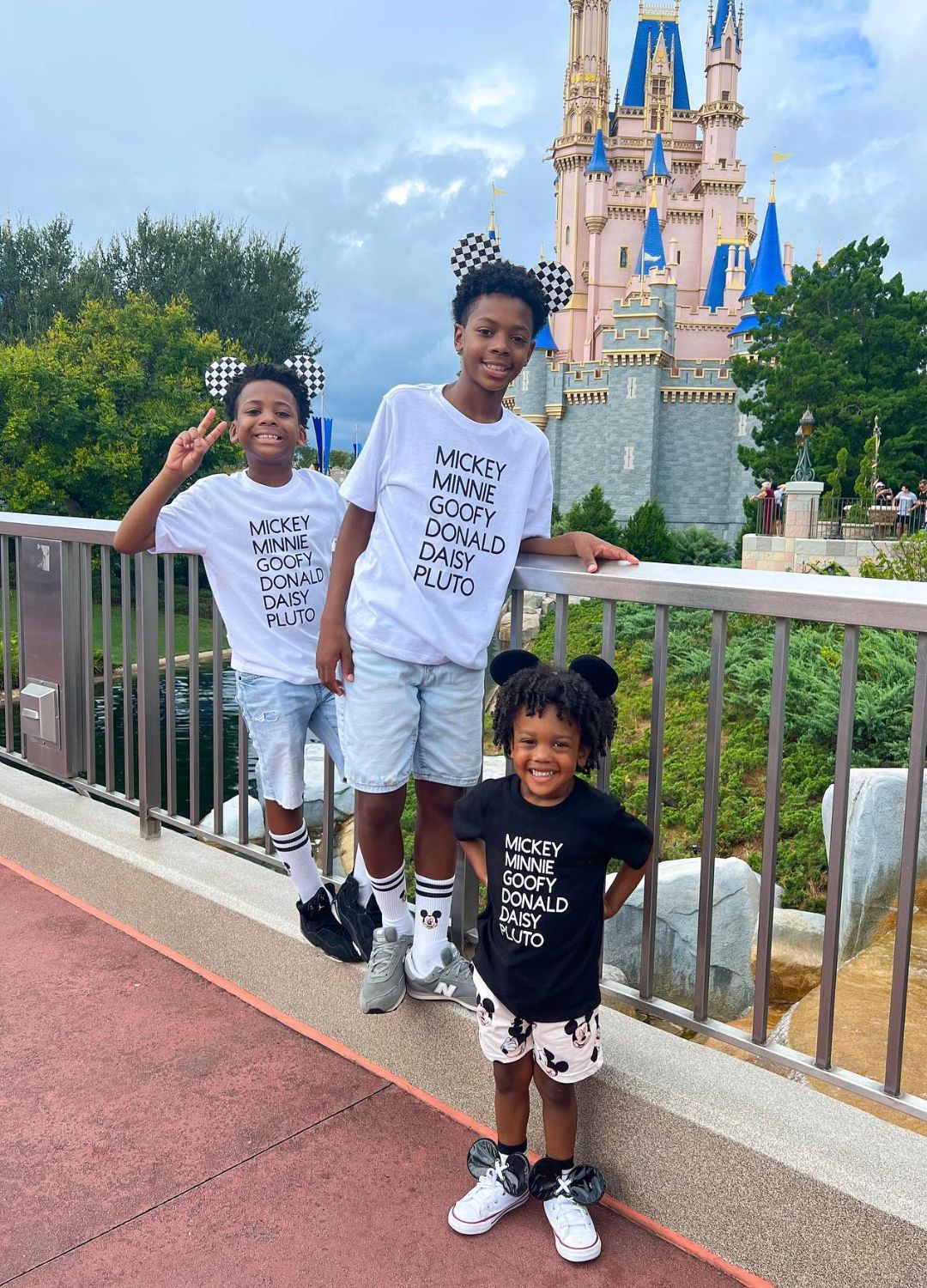
¿Debería usar un planificador de Disney para su viaje de Disney?
No tiene que usar un planificador de Disney para planificar su viaje, pero después de mi experiencia con ChatGPT, usaré uno, ya que todavía no sé por dónde comenzar con toda la información.
Trabajar con un planificador de Disney es a menudo gratuito, ya que Disney le paga a una comisión al planificador, pero si no es así, podría valer la pena la inversión solo para asegurarse de obtener la información más precisa.
Si no desea usar un planificador, pregúntele a los amigos que hayan estado en Disney para sus consejos e itinerarios. Puede ser más fácil entender lo que es realista en lugar de no para su familia si tiene hijos de edad similar y aún reducirá el trabajo para usted (Everymom también tiene consejos de mamás para viajar a Disney World con niños pequeños, Disney con un bebé e incluso Disney World mientras está embarazada).
Veredicto final? ChatGPT podría ser bueno para algunos aspectos de la planificación de viajes, pero el itinerario que me dio en base a mi aviso no era realista y tenía muchos errores. Para algo tan complicado como Disney World, tener ideas y juicio humanos se siente como una mejor manera de tratar de garantizar más magia de Disney que los dolores de cabeza.
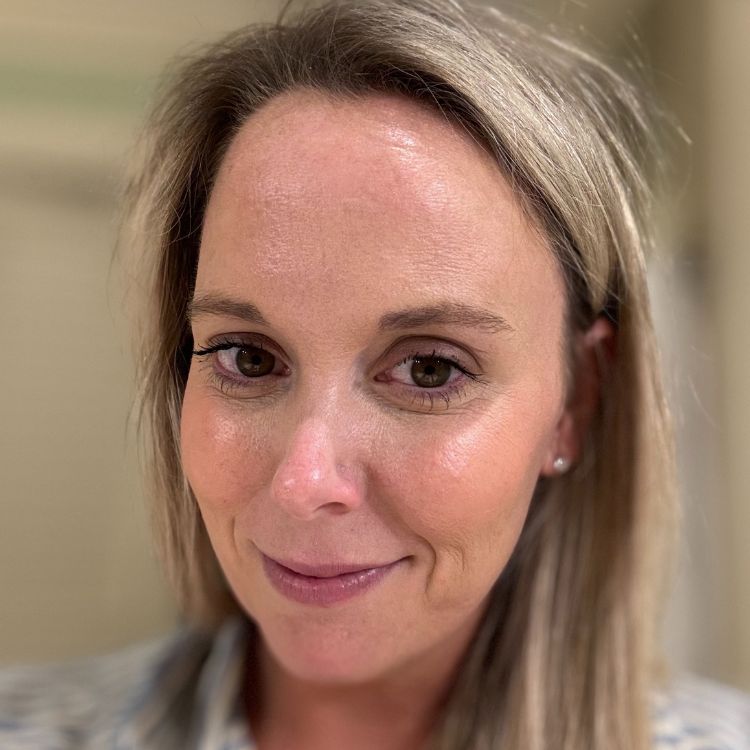
Sobre el autor
Elliott Harrell, escritor colaborador
Elliott es madre de dos niñas y tiene su sede en Raleigh, NC. Pasa sus días dirigiendo un equipo de ventas y lavando la ropa y sus noches escribiendo sobre las cosas que ama. Le apasiona todas las cosas de la maternidad y la salud de las mujeres. Cuando no está trabajando, escribiendo o criando, puede encontrarla probar un nuevo restaurante en la ciudad o trabajar en su último proyecto de aguja.
Noticias
Operai vs Musk Legal Feud se intensifica; Informe de la IEA
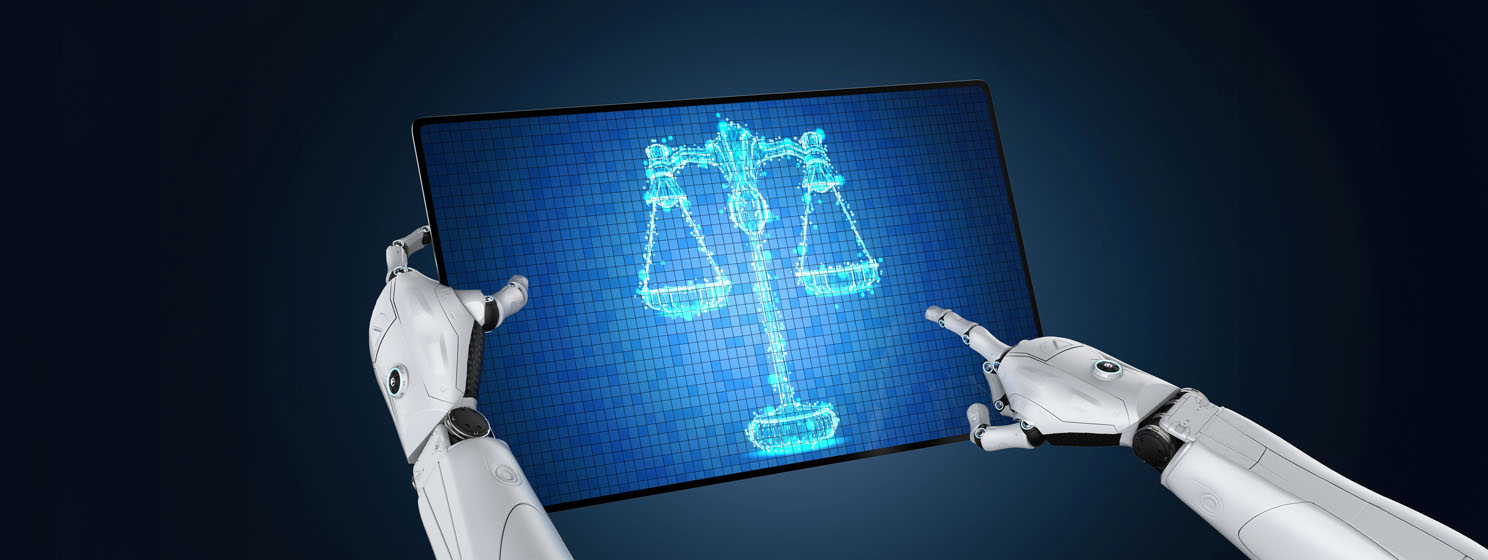
Operai, la compañía detrás de ChatGPT, disparó la semana pasada con un mostrador contra Elon Musk, marcando otro capítulo en lo que se ha convertido en una batalla legal muy pública entre Elon Musk, Sam Altman, Operai y unos pocos otros.
Musk ha estado en guerra con Operai y el CEO Sam Altman durante casi un año, acusando a la compañía de abandonar su misión original. La demanda original de Musk se centra en las afirmaciones de que OpenAI violó su acuerdo de fundadores y se separó de sus raíces originales sin fines de lucro en busca de ganancia comercial, específicamente a través de la creación de Operai Global LLC, su armado con fines de lucro, así como en su búsqueda de convertir su entidad sin fines de lucro en una compañía con fines de lucro.
Pero esta semana, Operai respondió. La compañía presentó una respuesta legal acusando a Musk de participar en “prácticas comerciales ilegales e injustas” diseñadas para interrumpir las operaciones de OpenAi y untar su reputación. Operai también afirma que Musk está haciendo principalmente todo esto para beneficiar a su compañía de IA, Xai.
Si solo estás poniendo al día con esta disputa, todavía estamos en sus primeras entradas, y ahora es el momento de ponerte al día. Nuestra cobertura anterior desglosa las presentaciones legales, la historia entre Musk y OpenAi, y lo que está en juego para ambas compañías.
La suscripción de $ 200 de Claude
La semana pasada, Anthrope lanzó un nuevo “Plan Max” para su IA Chatbot Claude, un nivel de suscripción de $ 100 y $ 200 por mes que ofrece lo que la compañía llama “uso ampliado”, que es solo otra forma de decir que podrá hacer más (tendrá menos límites) en Claude que antes. El nivel de $ 100/mes ofrece 5 veces más uso que el plan estándar Pro y el plan de $ 200/mes aumenta el uso de 20 veces el uso.
Un movimiento como este probablemente será celebrado por desarrolladores y nuevas empresas que tienen Claude integrado en algún lugar de su pila tecnológica. Pero debajo del capó, este movimiento es más que un rendimiento para sus usuarios; Se trata de rentabilidad para Anthrope, la empresa matriz para Claude.
Anthrope probablemente espere que este nuevo plan Max abra un nuevo canal de ingresos. Después de todo, se rumorea que el PRO Pro de $ 200/mes de OpenAI ha traído $ 300 millones adicionales después de su lanzamiento.
Este cambio de precios también resalta una tendencia más grande que se ha desarrollado detrás de escena del auge de la IA. A pesar de miles de millones en el gasto, ninguna de estas compañías de IA líderes ha obtenido ganancias todavía, y los inversores están comenzando a preocuparse, por lo que están comenzando a preguntar cuándo y de dónde provendrá un retorno de su inversión.
Ofrecer un producto más costoso es una forma de acercarse a la rentabilidad que los inversores están comenzando a presionar a estas compañías de inteligencia artificial para que produzcan, pero es poco probable que confiar en esa corriente de ingresos de los modelos de suscripción por sí solos sea poco probable que cualquiera de las empresas allí, especialmente cuando comienza a analizar cómo los consumidores demandan bien y servicios de IA.
El informe de la IEA explora el consumo de energía de IA
La Agencia Internacional de Energía (IEA) publicó un informe la semana pasada titulado Energía y ai, que exploró la creciente relación entre la inteligencia artificial y el consumo de energía global.
En 301 páginas, es un informe denso, pero aquí hay algunas conclusiones que se destacaron:
1. AI está aumentando la demanda de electricidad
Según el informe, se proyecta que el consumo de electricidad por parte de los centros de datos sea más del doble para 2030, y la IA es el impulsor número uno de ese crecimiento. Se espera que Estados Unidos sea responsable de más de la mitad del aumento global. Al final de la década, el uso de electricidad del Centro de datos de EE. UU. Podría exceder la potencia total utilizada para producir acero, aluminio, cemento, productos químicos y todos los demás bienes intensivos en energía combinados.
2. ¿De dónde vendrá el poder?
No se trata solo de construir más centros de datos; La IEA señala que varias redes de energía en todo el mundo ya están bajo una fuerte tensión. Sin actualizaciones significativas de infraestructura, especialmente nuevas líneas de transmisión, que pueden tardar de 4 a 8 años en construirse, muchos de los planes de expansión del centro de datos que seguimos escuchando pueden retrasarse o cancelarse.
3. El impacto energético de la IA no se está tratando como el de Crypto.
Mientras estaba pasando por el informe, me di cuenta de que el tono en torno al consumo de energía de IA es muy diferente a la actitud que estas mismas agencias tenían hacia la minería de recompensa en bloque. A pesar de que los centros de datos podrían estar utilizando más potencia que todo Japón para 2030, la AIE no argumentó que la industria está consumiendo demasiada electricidad. En cambio, argumenta que las contribuciones de IA a la innovación, especialmente en la eficiencia energética y la optimización de la red, pueden justificar el consumo.
En general, el informe trae algunos de los componentes menos explorados pero cruciales de la industria de la inteligencia artificial a la superficie. Si bien las compañías de IA han estado diciendo durante un tiempo que Estados Unidos necesita más centros de datos para mantenerse competitivos, el informe de la AIE subraya una parte del argumento de que generalmente no escuchamos de las compañías de IA: que no se trata solo de los centros de datos, también se trata de las fuentes de energía. Si la generación de energía y las soluciones de entrega no se exploran e implementan rápidamente, tienen el potencial de ralentizar significativamente los planes que algunos de los gigantes tecnológicos tienen para la industria de la IA.
Para que la inteligencia artificial (IA) trabaje en la ley y prospere frente a los crecientes desafíos, necesita integrar un sistema de cadena de bloques empresarial que garantice la calidad y la propiedad de la entrada de datos, lo que permite mantener los datos seguros al tiempo que garantiza la inmutabilidad de los datos. Echa un vistazo a la cobertura de Coingeek sobre esta tecnología emergente para aprender más Por qué Enterprise Blockchain será la columna vertebral de AI.
RELOJ: Los micropagos son lo que permitirán a las personas confiar en la IA
https://www.youtube.com/watch?v=XC9XDZMHJ9Q title = “YouTube Video Player” FrameBorDer = “0” permitido = “acelerómetro; autoplay; portapapeles-write; cifrado-media; gyroscope; imagen-in-pinicure; web-share” referrerPolicy = “estricto-origin-when-cross-órigin” permitido aficionado = “>”> “>”
Noticias
ChatGPT predicts all 32 first-round picks in 2025
2025 NFL Draft offers tons of offensive and defensive line help
USA TODAY Sports’ Tyler Dragon breaks down the deepest part of the 2025 NFL Draft, offensive and defensive lineman.
Sports Pulse
Mock drafts can be like steak. Many people love consuming them, but each one is made a little differently.
And just as some people like their steaks with A1 sauce, others like to see what happens when AI completes a mock draft.
USA TODAY Sports consulted OpenAI’s AI chatbot, ChatGPT, for its take on a first-round mock draft ahead of the 2025 NFL draft. Artificial intelligence made its selection for each pick and added some of its own justifications for them as well.
Though each pick was one that ChatGPT eventually landed on, a human writer made sure that each selection was a (relatively) realistic pick for each of the 32 teams.
Here’s how the first round of the 2025 NFL draft could go, according to ChatGPT:
2025 NFL mock draft: ChatGPT’s first-round picks
1. Tennessee Titans: Cam Ward, QB, Miami (FL)
With the first overall pick in the 2025 NFL draft, ChatGPT did not stray from the status quo. The AI chatbot pointed to Tennessee’s major need for a quarterback with Will Levis not proving he’s a long-term answer and “a new regime possibly wanting their own guy.”
The Titans hired Mike Borgonzi as their new general manager in January, and head coach Brian Callahan will be entering his second season in charge in Nashville.
2. Cleveland Browns: Travis Hunter, CB/WR, Colorado
Though quarterback is a draft need for Cleveland, ChatGPT ultimately did not go for the No. 2 quarterback in the draft – Colorado’s Shedeur Sanders – with the No. 2 overall pick. Instead, it selected Sanders’ teammate, Hunter, pointing to his versatility to play both wide receiver and cornerback at an “elite level—basically two first-rounders in one,” it wrote.
3. New York Giants: Abdul Carter, Edge, Penn State
No quarterback for “Big Blue” if ChatGPT has anything to say about it. The chatbot had also considered quarterback Shedeur Sanders and defensive tackle Mason Graham with the third pick, but it ultimately couldn’t pass up the opportunity to pair Carter with Kayvon Thibodeaux and Brian Burns in the Giants’ pass rush.
“That’s a nightmare for opposing QBs … If you can’t land your QB (like Ward), then ruin other people’s QBs instead.”
The OpenAI product also suggested Sanders’ NFL readiness was too questionable for New York to take that shot: “The Giants might not want to swing and miss again at QB this high.”
4. New England Patriots: Will Campbell, OT, LSU
ChatGPT is not concerned with Campbell’s arm size. It does like his three years of starting tackle experience in the SEC and the idea of getting quarterback Drake Maye better protection up front.
The artificial intelligence also considered defensive tackle Mason Graham to build the trenches on the other side of the ball and receiver Emeka Egbuka. However, the apparent need for help on the O-line was enough to make Campbell the No. 4 pick.
5. Jacksonville Jaguars: Mason Graham, DT, Michigan
“He’s the best interior defensive lineman in this class—quick off the snap, disruptive against both the run and pass,” ChatGPT wrote. “Jacksonville’s run defense and interior pass rush have been soft for years. Graham can anchor that front from Day 1.”
It’s hard to disagree with any of that analysis. The Jaguars allowed the second-most total offensive yards in 2024 and were second-worst in expected points added (EPA) per play, only behind the Carolina Panthers. They ranked 32nd in pass-rush win rate and 27th in run-stop win rate, according to ESPN.
6. Las Vegas Raiders: Armand Membou, OT, Missouri
Instead of bringing in some wide receiver help with a player like Arizona’s Tetairoa McMillan, ChatGPT opted to get a player with “trench cornerstone” upside. The AI chatbot wrote about Membou’s “elite traits” including “great feet, powerful hands and enough athleticism to hold his own against speed rushers off the edge.”
Membou would likely play right tackle or kick inside for Las Vegas in this scenario given Kolton Miller’s excellent play at left tackle, which ChatGPT acknowledged.
7. New York Jets: Tetairoa McMillan, WR, Arizona
After considering the Raiders as a landing spot for McMillan, ChatGPT ultimately had the Jets draft the big-bodied receiver with the No. 7 pick. The AI pointed to McMillan’s 6-foot-5 frame, body control and ball-tracking ability as parts of what make the Arizona product such an exciting prospect.
ChatGPT also liked pairing a big, downfield threat like McMillan with a veteran presence in Garrett Wilson. “Garrett Wilson is your route-running stud. McMillan gives you a massive red-zone threat and vertical presence,” it wrote.
8. Carolina Panthers: Emeka Egbuka, WR, Ohio State
ChatGPT continues to give the offense a lot of love in its mock draft. This time, it gives the Panthers another weapon for quarterback Bryce Young to throw to.
The AI wrote about Egbuka’s skills as a route-runner and versatility to play in the slot and outside – though mainly in the slot – as reasons for Carolina to bring him in. Given Adam Thielen’s age, adding Egbuka to pair with second-year receivers Xavier Legette and Jalen Coker is a good way for the Panthers to move forward in building its young receiver corps.
9. New Orleans Saints: Shedeur Sanders, QB, Colorado
Sanders’ brief slide ends within the top 10 as New Orleans takes another swing at bringing in its quarterback of the future. ChatGPT thinks the Saints are getting “tremendous value” by bringing in “a high-upside QB prospect with proven poise and experience” with the ninth overall pick.
The chatbot also wrote that the Saints have the option to let Sanders sit and develop behind incumbent veteran Derek Carr to begin the year if they so choose. “You’ve got options.”
10. Chicago Bears: Kelvin Banks Jr., OT, Texas
Darnell Wright is locked in on the right side for Chicago, but the Bears could still use a longer-term answer at left tackle that isn’t Braxton Jones, who’s missed 13 games across the last two seasons and is a free agent in 2026.
That’s why ChatGPT is bringing in another player with franchise tackle potential across from Wright and in front of franchise-quarterback hopeful Caleb Williams. And as the AI chatbot wrote, “Offensive tackle is always worth a top-10 pick, especially when you have a young QB to protect.”
11. San Francisco 49ers: Luther Burden III, WR, Missouri
A third wide receiver comes off the board within the first dozen picks. While there is a need for wide receiver help with Brandon Aiyuk recovering from an ACL tear and Deebo Samuel traded to the Commanders this offseason, it is not the team’s most pressing need. That would be on the defensive line, where San Francisco lost three out of its four starters.
Regardless, ChatGPT was a fan of Burden’s yards-after-catch abilities and how he pairs with running back Christian McCaffrey and tight end George Kittle in head coach Kyle Shanahan’s offensive system.
12. Dallas Cowboys: Tyler Booker, OG, Alabama
Once again, ChatGPT went for a depth need for a team rather than a more pressing need at another spot on the roster. It pointed to Dallas’ need for a successor to Zack Martin on the interior following the 34-year-old’s retirement earlier this offseason.
The AI chatbot also pointed to how successful previous Cowboys teams were when they had dominant offensive lines. Booker would be a nice option across from left guard Tyler Smith to fortify Dallas’ line in 2025 and beyond.
13. Miami Dolphins: Walter Nolen, DT, Mississippi
For the first time since the Jaguars took Mason Graham with the sixth overall pick, another defensive player comes off the board. ChatGPT did a nice job making this selection for Miami.
Calais Campbell headed to Arizona in free agency, and the Dolphins did not do much to address their interior defensive line earlier this offseason. They currently have four D-linemen on the roster: Zach Sieler, Benito Jones, Matt Dickerson and Neil Farrell. ChatGPT says Nolen has potential to reach “top-5-in-the-NFL type disruption if he continues to develop.”
14. Indianapolis Colts: Colston Loveland, TE, Michigan
ChatGPT filled the Colts’ clear biggest need with the No. 14 pick. “He gives the Colts something they don’t have—a legit TE1 with Pro Bowl upside—and makes the offense more dangerous from Day 1,” it wrote.
Indeed, Indianapolis is in dire need of an upgrade at tight end after no player at the position finished with more than 200 receiving yards for the Colts last year. Loveland’s large frame, good hands and route-running prowess were all enough to make him the best pick here, according to the AI chatbot.
15. Atlanta Falcons: Mike Green, Edge, Marshall
The Falcons have to keep taking swings at pass-rushing talent until they succeed. Even after trading for veteran Matthew Judon last offseason, Atlanta finished 27th in pass-rush win rate, according to ESPN.
ChatGPT appeared to be well aware of the Falcons’ need for a talented young edge rusher, and it sent the nation’s sack leader in 2024 to Atlanta with the 15th pick.
16. Arizona Cardinals: James Pearce Jr., Edge, Tennessee
Back-to-back edge rusher picks for teams with a need at the position. Arizona was even worse than Atlanta at rushing the passer according to ESPN’s analytics, ranking 28th in the league with a 33% win rate.
ChatGPT gave the Cardinals James Pearce Jr. – “one of the top pure edge rushers in the draft,” it wrote – to swing things in a better direction for the NFC West contender.
17. Cincinnati Bengals: Jalon Walker, Edge, Georgia
There is a bit of a run-on-edge rushers here through the halfway point of the first round. Walker is a special case because he has the versatility to play both on the edge and as an off-ball linebacker.
The Bengals need some insurance at edge with a disgruntled Trey Hendrickson recently requesting a trade out of Cincinnati. ChatGPT addressed that need and another Bengals need for an off-ball linebacker with a versatile athlete in Walker.
18. Seattle Seahawks: Jahdae Barron, CB, Texas
Cornerback is far from the top priority for Seattle in the draft, but there is an argument to be made. Devon Witherspoon is the only corner the Seahawks will have under contract past this year barring any contract extensions. Barron would provide excellent depth that would extend into the team’s future.
ChatGPT wrote that Barron’s versatility and athleticism gave him a high ceiling as a prospect in its justification for the pick.
19. Tampa Bay Buccaneers: Shemar Stewart, Edge, Texas A&M
After a long stretch of primarily offensive players, ChatGPT has flipped the script with a long run of only defensive players. This time it’s another edge rusher, Stewart, going to Tampa Bay with the 19th overall pick.
The AI chatbot pointed to his “explosive athleticism and ability to disrupt the quarterback” as a good fit for a Buccaneers pass-rush attack that could use enhancing. The team has veteran Haason Reddick for the coming season, but it could use a longer-term answer at the key position.
20. Denver Broncos: Matthew Golden, WR, Texas
Courtland Sutton got off to a great start building his chemistry with rookie quarterback Bo Nix last year, but the team still needs receiver help to go along with Sutton in the passing game. That’s where Golden comes in, as ChatGPT suggested his “explosive speed and ability to make plays after the catch” were part of what made him a good fit.
The chatbot wrote it was also considering Ashton Jeanty, but his slide continues out of the top 20 instead.
21. Pittsburgh Steelers: Jalen Milroe, QB, Alabama
As of the time of writing, the Steelers would be entering the 2025 season with Mason Rudolph as their starting quarterback and Skylar Thompson backing him up. ChatGPT gave them a younger option – Milroe – to develop before giving him a chance to take over as their future franchise quarterback.
“If the Steelers were to select him at pick No. 21,” the chatbot wrote, “it would be with the intent to develop his potential … aligning with their long-term strategic goals.”
22. Los Angeles Chargers: Ashton Jeanty, RB, Boise State
The slide finally stops for the best running back prospect in the 2025 NFL draft class. ChatGPT partnered the Heisman Trophy runner-up with head coach Jim Harbaugh in Los Angeles after running backs Gus Edwards and J.K. Dobbins hit free agency.
“Jeanty offers excellent speed, vision, and versatility as a dual-threat back,” it wrote. “He would add a dynamic element to the Chargers’ offense, complementing the passing game and making the running game much more potent.”
23. Green Bay Packers: Derrick Harmon, DT, Oregon
Despite the Packers’ more pressing need for an edge rusher, there were just too many off of the board at this point in the mock draft. ChatGPT went with a versatile defensive tackle with experience playing at the nose tackle and 3-technique positions on the defensive line.
Given that Green Bay generally needs help rushing the passer, regardless of which position is doing it, it doesn’t hurt to bring in the power conferences’ leader in pressures from the interior.
24. Minnesota Vikings: Grey Zabel, OG, North Dakota State
Minnesota poached a couple of interior offensive linemen – center Ryan Kelly and right guard Will Fries – from the Indianapolis Colts in free agency. However, some extra help could still be needed on the left side of the interior.
Zabel has played four out of the five positions on the offensive line and projects as a guard at the pro level. ChatGPT wrote, “His fit within the Vikings’ zone-blocking scheme and his technical prowess in the run game address a critical gap on the offensive line.”
25. Houston Texans: Donovan Jackson, OL, Ohio State
ChatGPT had this to say about why Jackson is a good fit in Houston: “Ultra-athletic, battle-tested interior lineman with positional versatility. He’s great at pulling, climbing to the second level, and would instantly help protect C.J. Stroud—his former college teammate.”
Indeed, Jackson played in all 13 of Ohio State’s games as a freshman with Stroud under center and was named a starter for the team the following year, Stroud’s final one with the Buckeyes. He could immediately replace Shaq Mason on the right side after the Texans released their former guard.
26. Los Angeles Rams: Maxwell Hairston, CB, Kentucky
No player invited to the 2025 NFL combine was faster than Kentucky cornerback Maxwell Hairston. The speedster is a scheme-versatile cornerback with excellent ball skills, tallying five interceptions in a full 2023 season and one in an injury-shortened 2024.
ChatGPT pointed out the Rams’ need to defend excellent receivers in a stacked NFC West: San Francisco’s Jauan Jennings and Brandon Aiyuk, Arizona’s Marvin Harrison Jr. and Seattle’s Jaxon Smith-Njigba and Cooper Kupp. The AI chatbot envisions Hairston as a rotational corner in Los Angeles’ secondary, if not a potential starter.
27. Baltimore Ravens: Donovan Ezeiruaku, Edge, Boston College
A 16.5-sack season from Ezeiruaku pushed the Boston College star firmly into first-round discussion. His 2024 tape showed a player that has the potential to be a plug-and-play, Week 1 starter for the team that drafts him, making him a great fit for a Ravens squad that needs help in its pass-rush attack.
ChatGPT wrote, “His proven track record of quarterback pressures and sacks positions him as a valuable asset to the Ravens’ defense.”
28. Detroit Lions: Princely Umanmielen, Edge, Mississippi
Given the amount of edge rusher talent off the board at this late stage of the first round, the Lions had to reach slightly to get the next best available. Most analysts project Umanmielen as a second-round pick, but ChatGPT listed him as a “Back-end Round 1 pass rusher with serious juice.”
The AI bot likes his upside and potential to contribute as a rotational pass rusher early on. “Twitched-up pass rusher with great first-step quickness and bend,” it wrote. “Still refining his game, but he’s shown flashes of Round 1 ability. Could rotate early and eventually start opposite Aidan Hutchinson.”
29. Washington Commanders: Azareye’h Thomas, CB, Florida State
ChatGPT wrote: “With good size (6’1″, 197 lbs) and solid production at Florida State, he could help immediately on Washington’s defense. His physical play style and coverage skills make him a solid pick for a team looking to improve at cornerback.”
Thomas recorded 53 tackles and an interception in 2024, one year after a 2023 season that featured 29 tackles, a forced fumble, 10 passes defensed and 0.5 sacks. ChatGPT pointed to Thomas’ size and coverage ability as major parts of what makes him a good fit for Washington’s defense.
30. Buffalo Bills: Nick Emmanwori, S, South Carolina
So many edge rushers are off the board, leaving the Bills almost no choice but to address a different position with the No. 30 pick. So that’s precisely what ChatGPT did, sending one of this draft class’s best athletes to Buffalo to bolster its defensive secondary.
“While players like Damar Hamlin and Taylor Rapp are on the roster, Emmanwori’s skill set offers a unique blend of coverage ability and physicality,” it wrote. “His proficiency in both zone and man coverage schemes, coupled with his tackling prowess, aligns well with the Bills’ defensive philosophy.”
31. Kansas City Chiefs: Josh Conerly Jr., OT, Oregon
ChatGPT wrote, “He gives KC a future starter at tackle with the athletic profile to thrive in their pass-heavy offense. He’s raw, but that’s what Reid’s staff does best — develop traits into production.”
It is hard to disagree with that logic, especially after Kansas City was forced to lean on left guard Joe Thuney to play at its left tackle spot for several games. The line will need even more help now that Thuney is gone via trade to the Bears.
32. Philadelphia Eagles: Josh Simmons, OT, Ohio State
“The Eagles value versatility, and Simmons’ ability to play both tackle spots adds value,” the chatbot wrote. “Additionally, having a young, athletic offensive lineman who can be developed into a potential starter when the team needs it fits their long-term strategy. Even though Simmons might not start immediately, his growth potential aligns well with the Eagles’ track record of developing players on the offensive line.”
This is all true. The Eagles greatly value what they have in offensive line coach Jeff Stoutland, who has been a massive factor in getting Philadelphia’s O-line to the outstanding level it’s played at in recent years. Simmons only makes the future of the Eagles’ offensive line even more formidable.
-
Startups11 meses ago
Remove.bg: La Revolución en la Edición de Imágenes que Debes Conocer
-
Tutoriales11 meses ago
Cómo Comenzar a Utilizar ChatGPT: Una Guía Completa para Principiantes
-
Startups9 meses ago
Startups de IA en EE.UU. que han recaudado más de $100M en 2024
-
Recursos11 meses ago
Cómo Empezar con Popai.pro: Tu Espacio Personal de IA – Guía Completa, Instalación, Versiones y Precios
-
Startups11 meses ago
Deepgram: Revolucionando el Reconocimiento de Voz con IA
-
Recursos11 meses ago
Perplexity aplicado al Marketing Digital y Estrategias SEO
-
Recursos11 meses ago
Suno.com: La Revolución en la Creación Musical con Inteligencia Artificial
-
Noticias9 meses ago
Dos periodistas octogenarios deman a ChatGPT por robar su trabajo