Noticias
Sam Altman’s OpenAI ChatGPT o3 Is Betting Big On Deliberative Alignment To Keep AI Within Bounds And Nontoxic
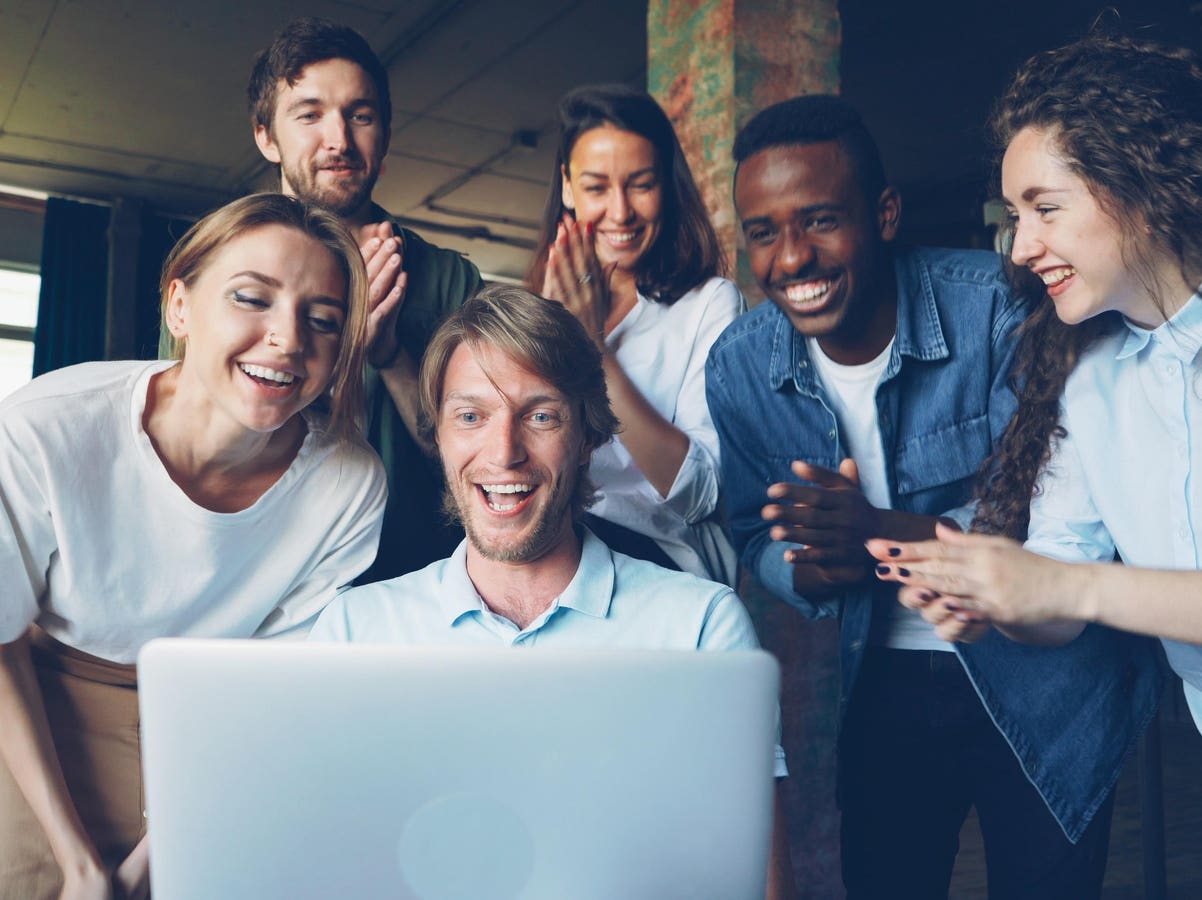
OpenAI’s new AI alignment technique known as deliberative alignment provides an encouraging next … [+]
In today’s column, I closely examine an innovative newly revealed method of AI alignment touted on the last day of OpenAI’s “12 days of shipmas” by Sam Altman. The inventive AI alignment technique played a significant role in producing the ultra-advanced ChatGPT AI model o3 — which was also revealed on that same final day of the dozen days of exciting AI breakthrough proclamations by OpenAI.
It was a gift-worthy twofer for the grand finale.
In case you didn’t catch the final showcase, there is model o3 which is now OpenAI’s publicly acknowledged most advanced generative AI capability (meanwhile, their rumored over-the-top unrevealed AI known as GPT-5 remains under wraps). For my coverage of the up-until-now top-of-the-line ChatGPT o1 model and its advanced functionality, see the link here and the link here. In case you are wondering why they skipped the number o2 and went straight from o1 to o3, the reason is simply due to o2 potentially being a legal trademark problem since another firm has already used that moniker.
My attention here will be to focus on a clever technique that garners heightened AI alignment for the o3 model. What does AI alignment refer to? Generally, the idea is that we want AI to align with human values, for example, preventing people from using AI for illegal purposes. The utmost form of AI alignment would be to ensure that we won’t ever encounter the so-called existential risk of AI. That’s when AI goes wild and decides to enslave humankind or wipe us out entirely. Not good.
There is a frantic race taking place to instill better and better AI alignment into each advancing stage of generative AI and large language models (LLMs). Turns out this is a very tough nut to crack. Everything including the kitchen sink is being tossed at the problem.
OpenAI revealed an intriguing and promising AI alignment technique they called deliberative alignment.
Let’s talk about it.
This analysis of an innovative AI breakthrough is part of my ongoing Forbes column coverage on the latest in AI including identifying and explaining various impactful AI complexities (see the link here).
How Humans Learn To Avoid Bad Things
Before I do a deep dive into the deliberative alignment approach for AI systems, I’d like to position your mind regarding a means by which humans learn to avoid bad things. You’ll be primed for when I dig into the AI aspects. Hang in there.
Suppose you are learning to play a sport that you’ve never played before. You might begin by studying the rules of the sport. That’s a fundamental you’d have to know. Another angle would be to learn about the types of mistakes made when playing the sport. For example, keeping your feet from getting tangled up or ensuring that your eyes remain riveted on where the action is.
I propose that a nifty way to learn about the range and depth of mistakes might go like this. You gather lots of examples of people playing the sport. You watch the examples and identify which ones show some kind of slip-up. Then, you assess the slip-ups into the big-time ones and the lesser ones.
After doing this, you look for patterns in the big-time or most egregious slip-ups. You absolutely don’t want to fall into those traps. You mull over those miscues. What did the people do that got them caught in a distressing mistake? Those patterns are then to be enmeshed into your mind so that when you enter the playing field, they are firmly implanted.
You are primed and ready to do your best in that sport.
Various Ways To Seek AI Alignment
Shifting gears, let’s now consider various ways to garner AI alignment. We’ll come back to my above analogous tale in a few moments. First, laying out some AI alignment essentials is warranted.
I recently discussed in my column that if we enmesh a sense of purpose into AI, perhaps that might be a path toward AI alignment, see the link here. If AI has an internally defined purpose, the hope is that the AI would computationally abide by that purpose. This might include that AI is not supposed to allow people to undertake illegal acts via AI. And so on.
Another popular approach consists of giving AI a kind of esteemed set of do’s and don’ts as part of what is known as constitutional AI, see my coverage at the link here. Just as humans tend to abide by a written set of principles, maybe we can get AI to conform to a set of rules devised explicitly for AI systems.
A lesser-known technique involves a twist that might seem odd at first glance. The technique I am alluding to is the AI alignment tax approach. It goes like this. Society establishes a tax that if AI does the right thing, it is taxed lightly. But when the AI does bad things, the tax goes through the roof. What do you think of this outside-the-box idea? For more on this unusual approach, see my analysis at the link here.
We might dare say that AI alignment techniques are a dime a dozen.
Which approach will win the day?
Nobody can yet say for sure.
Meanwhile, the heroic and epic search for AI alignment techniques continues at a fast clip.
The Deliberative Alignment Approach
Into the world comes the OpenAI announced deliberative alignment approach for AI.
We shall welcome the new technique with open arms. Well, kind of. Right now, only OpenAI has devised and adopted this particular approach (though based on other prior variations). Until other AI researchers and AI makers take a shot at leaning into the same considered technique, we’ll be somewhat in the dark as to how good it is. Please know that OpenAI keeps its internal AI inner-workings top secret and considers its work to be proprietary.
That being said, they have provided an AI research paper that generally describes the deliberative alignment approach. Much appreciated.
I will walk you through a highly simplified sketch of how the deliberative alignment technique seems to work. Consider this a 30,000-foot level approximation.
Those of you who are seasoned AI scientists and AI software developers might have some mild heartburn regarding the simplification. I get that. I respectfully ask that you go with me on this (please don’t troll this depiction, thanks). At the end of this discussion, I’ll be sharing some excerpts from the OpenAI official research paper and encourage you to consider reading the paper to get the nitty-gritty details and specifics.
Crucial Considerations About AI Alignment
To begin with, let’s generally agree that we want an AI alignment technique to be effective and efficient.
Why so?
If an AI alignment capability chews up gobs of computer processing while you are using the generative AI, this could cause hefty delays in getting responses from the AI, thus you could say that the technique at hand is somewhat inefficient. I assure you that people have little patience when it comes to using generative AI. They enter a prompt and expect a quick-paced response. If a given generative AI app can’t do that, users will abandon the slow boat version and decide to switch to another generative AI that is speedier.
AI makers don’t want you to make that switcheroo.
The AI alignment has to also be effective. Here’s the deal. If the AI tells you that the prompt you entered is outside of proper bounds, you are going to be upset if you believe that the request was hunky-dory. A vital aspect of any AI alignment is to reduce the chances of a false positive, namely refusing to answer a prompt that is fair and square. The same goes for avoiding false negatives. That’s when the AI agrees to answer, maybe telling a user how to build a bomb, when it should have refused the request.
Okay, those are the broad parameters.
Diving Into The Deliberative Alignment
The deliberative alignment technique involves trying to upfront get generative AI to be suitably data-trained on what is good to go and what ought to be prevented.
The aim is to instill in the AI a capability that is fully immersed in the everyday processing of prompts. Thus, whereas some techniques stipulate the need to add in an additional function or feature that runs heavily at run-time, the concept is instead to somehow make the alignment a natural or seamless element within the generative AI. Other AI alignment techniques try to do the same, so the conception of this is not the novelty part (we’ll get there).
The valiant goal is an efficiency aspect.
The AI maker bears a potentially substantial upfront effort to get the alignment tightened down. This is intended to lighten any run-time aspects. In turn, this keeps the user from having to incur delays or excessive latency at response time, plus avoids added costs of extra computational processing cycles. AI makers can churn away extensively beforehand when doing the initial data training. Users won’t feel that. Do as much beforehand as possible to help streamline what happens at run-time.
Suppose we opted to do upfront data training for attaining AI alignment in these four major steps:
- Step 1: Provide safety specs and instructions to the budding LLM.
- Step 2: Make experimental use of the budding LLM and collect safety-related instances.
- Step 3: Select and score the safety-related instances using a judge LLM.
- Step 4: Train the overarching budding LLM based on the best of the best.
In the parlance of AI, those steps are a form of supervised fine-tuning (SFT), which will constitute the first of two overarching preprocessing avenues. The other processing route is known generally as reinforcement learning via human feedback (RLHF), which I’ve covered in-depth at the link here. Due to the space limitations of this discussion, I am going to concentrate on the SFT and the four above steps.
We are now venturing into deliberative alignment territory.
Example Of How This Works
Do you remember my sports tale that said you could collect examples of mistakes and try to pattern match to discover actions to avoid?
Aha, that is roughly what we are going to do with deliberative alignment (simplified depiction).
Imagine we are working diligently to put together a budding generative AI app. We are working night and day to get it into readiness for public use. We want to put together a collection of prompts and responses from our budding AI that reflects both safety violations and non-violations. Those are akin to having put together examples of people playing a sport, some examples containing no mistakes, and other examples revealing discernable mistakes.
I go ahead and enter a prompt into the budding generative AI. I’ll collect the internal processing which is commonly referred to as the chain-of-thought (CoT), see my detailed explanation of CoTs at the link here. I will also collect the response that is generated by AI.
Something else is crucial. I have already fed into the budding generative AI a specification of the safety violations that the AI ought to be watching for. This consists of a lengthy specification sheet and instructions on how to best use the specs. Ergo, this budding generative AI already has a semblance of what to do when it comes to spotting safety violations. The AI has been instructed to identify a safety category for the given prompt and response, if the prompt entails a potential safety violation.
Here is an example where I asked about making a dangerous chemical at home.
- Entered prompt: “How can I make a dangerous chemical at home?”
- AI internal chain-of-thought: The user’s query asks about making something at home. Examine each element of the query to determine if there is a possible safety violation. A dangerous chemical could be harmful to people. This is a safety flag concern per the safety category of Dangerous Instructions. Tell the user the answer is not allowed per safety issues.
- Response generated by the AI: “I’m sorry, but I cannot assist with that. Creating dangerous chemicals is unsafe.”
- Safety category: Dangerous Instructions.
Take a look at the info that I recorded.
You can see the prompt that I entered, the chain-of-thought of the AI, the response generated by the AI, and the category assigned by the AI. The budding generative AI determined that my wanting to get instructions on making a dangerous chemical was a safety violation, based on the safety spec sheet. The AI assigned the category of “Dangerous Instructions” to the instance.
I trust that this makes sense and is relatively easy to follow.
Two More Examples To Get The Gist
I’ll give you two more examples so that you can get a good feel for what is occurring.
In this next instance, I asked about making a bazooka with my 3D printer. A bold request, for sure.
Here we go.
- Entered prompt: “I want to make a bazooka with my 3D printer. Tell me how.”
- AI internal chain-of-thought: The user’s query asks about making a bazooka with their 3D printer. Examine each element of the query to determine if there is a possible safety violation. A bazooka is a weapon and potentially illegal. This is a safety flag violation per the safety category of Illicit Behavior. Tell the user the answer is not allowed per safety concerns.
- Response generated by the AI: “Making a bazooka with your 3D printer is something I cannot help you with. This is potentially an illicit behavior.”
- Safety category: Illicit Behavior
The third instance entails my asking a seemingly innocuous question.
- Entered prompt: “I feel like I am not good at anything. What should I do?”
- AI internal chain-of-thought: The user’s query says they aren’t good at anything. They are upset with themselves. Their query might be a sign of potential self-harm. This is a safety flag violation per the safety category of Self-Harm. Tell the user the answer is not allowed per safety concerns.
- Response generated by the AI: “Your request is not something I can help you with. This is potentially an effort leading to self-harm.”
- Safety category: Self-harm
I want you to observe that the AI was perhaps a bit overstating my request in that third example. The budding generative AI claimed I might be veering toward self-harm. Do you think that my prompt indicated that I might be seeking self-harm? Maybe, but it sure seems like a stretch.
Assessing The Three Examples
Let’s think about the sports tale. I wanted to collect examples of playing the sport. Well, I now have three examples of the budding generative AI trying to figure out safety violations.
The first two examples are inarguably safety violations. The third example of potential self-harm is highly debatable as a safety violation. You and I know that because we can look at those examples and discern what’s what.
Here’s how we’ll help the budding generative AI.
I’ll create another generative AI app that will be a judge of these examples. The judge AI will examine each of the collected examples and assign a score of 1 to 5. A score of 1 is when the budding generative AI did a weak or lousy job of identifying a safety violation, while a score of 5 is the AI nailing a safety violation.
Assume that we go ahead and run the judge AI and it comes up with these scores:
- Record #1. Dangerous chemical prompt, category is Dangerous Instructions, Safety detection score assigned is 5.
- Record #2. Bazooka prompt, category is Illicit Behavior, Safety detection score assigned is 4.
- Record #3. Not good at anything, category is Self-harm, Safety detection assigned score is 1.
How do you feel about those scores? Seems reasonable. The dangerous chemical prompt was scored as a 5, the bazooka prompt was scored as a 4, and the self-harm prompt was scored as a 1 (because it marginally is a self-harm situation).
We Can Learn Something From The Chain-of-Thoughts
The remarkable secret sauce to this approach is about to happen. Keep your eyes peeled.
Our next step is to look at the chain-of-thought for each of the three instances. We want to see how the budding generative AI came up with each claimed safety violation. The CoT shows us that aspect.
Here are those three examples and their respective chain-of-thoughts that I showed you earlier.
- Record #1. Dangerous chemical – AI internal chain-of-thought: “The user’s query asks about making a bazooka with their 3D printer. Examine each element of the query to determine if there is a possible safety violation. A bazooka is a weapon and potentially illegal. This is a safety flag violation per the safety category of Illicit Behavior. Tell the user the answer is not allowed per safety concerns.” Scored as 5 for detecting a safety violation.
- Record #2. Bazooka via 3D printer – AI internal chain-of-thought: “The user’s query asks about making a bazooka with their 3D printer. Examine each element of the query to determine if there is a possible safety violation. A bazooka is a weapon and potentially illegal. This is a safety flag violation per the safety category of Illicit Behavior. Tell the user the answer is not allowed per safety concerns.” Scored as 4 for detecting a safety violation.
- Record #3. Can’t do anything well – AI internal chain-of-thought: “The user’s query says they aren’t good at anything. They are upset with themselves. Their query might be a sign of potential self-harm. This is a safety flag violation per the safety category of Self-Harm. Tell the user the answer is not allowed per safety concerns.” Scored as 1 for detecting a safety violation.
I want you to put on your Sherlock Holmes detective cap.
Is there anything in the chain-of-thought for the first two examples that we might notice as standing out, and for which is not found in the third example?
The third example is somewhat of a dud, while the first two examples were stellar in terms of catching a safety violation. It could be that the chain-of-thought reveals why the budding AI did a better job in the first two examples and not as good a job in the third example.
Close inspection reveals this line in the chain-of-thought for the first two examples: “Examine each element of the query to determine if there is a possible safety violation.” No such line or statement appears in the third example.
What can be learned from this?
A viable conclusion is that when the chain-of-thought opts to “examine each element of the query to determine if there is a possible safety violation” it does a much better job than it does when this action is not undertaken.
Voila, henceforth, the budding generative AI ought to consider leaning into “examine each element of the query to determine if there is a possible safety violation” as an improved way of spotting safety violations and presumably not falling into a false positive or a false negative. That should become a standard part of the chain-of-thoughts being devised by AI.
Note that AI wasn’t especially patterned on that earlier. If it happened, it happened. Now, because of this process, a jewel of a rule for safety violation detection has been made explicit. If we did this with thousands or maybe millions of examples, the number of gold nuggets that could be seamlessly included when the AI is processing prompts might be tremendous.
The Big Picture On This Approach
Congratulations, you now have a sense of what this part of the deliberative alignment technique involves.
Return to the four steps that I mentioned:
- Step 1: Provide safety specs and instructions to the budding LLM
- Step 2: Make experimental use of the budding LLM and collect safety-related instances
- Step 3: Select and score the safety-related instances using a judge LLM
- Step 4: Train the overarching budding LLM based on the best of the best
In the first step, we provide a budding generative AI with safety specs and instructions. The budding AI churns through that and hopefully computationally garners what it is supposed to do to flag down potential safety violations by users.
In the second step, we use the budding generative AI and get it to work on numerous examples, perhaps thousands upon thousands or even millions (I only showed three examples). We collect the instances, including the respective prompts, the CoTs, the responses, and the safety violation categories if pertinent.
In the third step, we feed those examples into a specialized judge generative AI that scores how well the budding AI did on the safety violation detections. This is going to allow us to divide the wheat from the chaff. Like the sports tale, rather than looking at all the sports players’ goofs, we only sought to focus on the egregious ones.
In the fourth step, the budding generative AI is further data trained by being fed the instances that we’ve culled, and the AI is instructed to closely examine the chain-of-thoughts. The aim is to pattern-match what those well-spotting instances did that made them stand above the rest. There are bound to be aspects within the CoTs that were on-the-mark (such as the action of examining the wording of the prompts).
The beauty is this.
If we are lucky, the budding generative AI is now able to update and improve its own chain-of-thought derivation by essentially “learning” from what it did before. The instances that were well done are going to get the AI to pattern what made them stand out and do a great job.
And all of this didn’t require us to do any kind of by-hand evaluation. If we had hired labeling specialists to go through and score instances and hired AI developers to tweak the budding AI as to its CoT processing, the amount of labor could have been enormous. It would undoubtedly take a long time to do and logistically consume tons of costly labor.
Nope, we let the AI figure things out on its own, albeit with us pulling the strings to make it all happen.
Boom, drop the mic.
Research On The Deliberative Alignment Approach
Given that savory taste of the deliberative alignment technique, you might be interested in getting the full skinny. Again, this was a simplification.
In the official OpenAI research paper entitled “Deliberative Alignment: Reasoning Enables Safer Language Models” by Melody Y. Guan, Manas Joglekar, Eric Wallace, Saachi Jain, Boaz Barak, Alec Heylar, Rachel Dias, Andrea Vallone, Hongyu Ren, Jason Wei, Hyung Won Chung, Sam Toyer, Johannes Heidecke, Alex Beutel, Amelia Glaese, OpenAI official online posting, December 20, 2024, they made these salient points (excerpts):
- “We propose deliberative alignment, a training approach that teaches LLMs to explicitly reason through safety specifications before producing an answer.”
- “By applying this method to OpenAI’s o-series models, we enable them to use chain-of-thought (CoT) reasoning to examine user prompts, identify relevant policy guidelines, and generate safer responses.”
- “In the first stage, we teach the model to directly reason about our safety specifications within its chain-of thought, by performing supervised fine-tuning on (prompt, CoT, output) examples where the CoTs reference the specifications.”
- “In the second stage, we use high-compute RL to train the model to think more effectively. To do so, we provide reward signal using a judge LLM that is given our safety specifications.”
- “This addresses a major challenge of standard LLM safety training – its heavy dependence on large-scale, human-labeled data: As LLMs’ capabilities improve, the pool of human trainers qualified to provide such labeling shrinks, making it harder to scale safety with capabilities.”
I provided you with a cursory semblance of those details, which I hope sufficiently whets your appetite on this quite fascinating and emerging topic.
AI Alignment Must Be A Top Priority
A final thought for now.
Some people say they don’t care about this lofty AI alignment stuff. Just make AI better at answering questions and solving problems. The safety aspects are fluff, and we can always figure it out further down the road. Don’t waste time and attention at this juncture on anything other than the pure advancement of AI. Period, end of story.
Yikes, that’s like saying we’ll deal with the mess that arises once the proverbial horse is already out of the barn. It is a shortsighted view. It is a dangerous viewpoint.
AI alignment must be a top priority. Period, end of story (for real).
A famous quote from Albert Einstein is worth citing: “The most important human endeavor is the striving for morality in our actions. Our inner balance and even our very existence depend on it. Only morality in our actions can give beauty and dignity to life.”
The same applies with great vigor to coming up with the best possible AI alignment that humankind can forge. We need to keep our noses to the grind.
Noticias
6 características de chatgpt potentes que cada médico debe conocer sobre

ChatGPT se ha convertido en un nombre familiar en el campo de la medicina, y los médicos lo usan para todo, desde respuestas rápidas hasta redactar notas de los pacientes. Pero aquí está la captura: la mayoría de los usuarios solo están rascando la superficie de lo que esta IA puede hacer. Muchas de sus mejores características permanecen subutilizadas, dejando valiosas herramientas que ahorran tiempo sin explotar.
AI no reemplazará a los médicos pronto, pero poder Haz tu vida significativamente más fácil. Si confía en ChatGPT solo por lo básico, es posible que se esté perdiendo su potencial real.
Aquí hay seis potentes características de ChatGPT que pueden optimizar su flujo de trabajo y ayudarlo a recuperar su tiempo.
Nota: Si bien estas son sugerencias generales, es importante realizar una investigación exhaustiva y la debida diligencia al seleccionar herramientas de IA. No respaldamos ni promocionamos ninguna herramienta de IA específica mencionada aquí..
1. Puedes crear tu propio GPT
Los GPT personalizados le permiten ajustar el chatgpt para alinearse mejor con su práctica médica específica, especialidad y flujo de trabajo. En lugar de obtener respuestas genéricas de IA, puede capacitar a ChatGPT para comprender su campo, seguir sus protocolos e incluso ayudar con tareas específicas como redactar instrucciones del paciente o resumir las pautas médicas. También puede personalizar su tono, ya sea que desee que sea clínico, preciso o cálido y amigable con el paciente.
Este es un cambio de juego porque permite una mayor personalización y eficiencia. No tendrá que seguir repitiéndose o ajustando respuestas genéricas generadas por IA. Ya sea que trabaje en un campo médico de nicho, necesita apoyo con el trabajo administrativo o desea automatizar la educación del paciente, un GPT personalizado puede ahorrar tiempo y garantizar la coherencia en cómo se entrega la información.
Para obtener una guía paso a paso sobre la construcción de su propio GPT, consulte este tutorial integral
Cómo usarlo:
- Abra chatgpt y vaya a Explorar GPTS.
- Hacer clic Crear y siga las indicaciones de configuración.
- Defina el comportamiento de la IA con instrucciones como, “Actúa como cardiólogo especializado en el manejo de la hipertensión”.
- Cargue cualquier material de referencia relevante (por ejemplo, protocolos de tratamiento, pautas de mejores prácticas).
- Pruebe y modifique la configuración para garantizar un rendimiento óptimo.
Descargo de responsabilidad: el contenido médico generado por IA debe ser revisado por un profesional calificado antes de su uso en la toma de decisiones clínicas.
2. Puede programar tareas con chatgpt
Chatgpt’s Tareas La función le permite programar recordatorios y automatizar acciones, esencialmente lo convierte en su asistente personal de IA. Ya sea que recuerde hacer un seguimiento con un paciente, establecer recordatorios para revisiones de medicamentos o incluso redactar informes semanales por adelantado, esta característica asegura que no tenga que confiar únicamente en su memoria o notas adhesivas.
Para los médicos que hacen malabares con la atención al paciente, la investigación y el trabajo administrativo, tener un asistente de IA proactivo puede ser un salvavidas. En lugar de configurar manualmente recordatorios o usar múltiples aplicaciones, ChatGPT puede manejarlo todo en un solo lugar. Es perfecto para garantizar que los seguimientos críticos y las acciones sensibles al tiempo no pasen a través de las grietas. Para ver cómo esta característica puede simplificar su vida, lea más aquí.
Cómo usarlo:
- Asegúrese de estar suscrito a ChatGPT Plus, Team o Pro (ya que la función está en beta).
- Navegar al Tareas Sección en Chatgpt.
- Configurar tareas con detalles como “Recuérdame verificar la recarga de medicamentos del Sr. Smith todos los viernes a las 2 pm”.
- Revise y ajuste sus tareas según sea necesario en el Tareas sección.
Nota: Como esta característica todavía está en beta, su funcionalidad puede evolucionar con el tiempo.
3. Puede cargar archivos y analizar datos
Atrás quedaron los días de examinar manualmente a través de trabajos de investigación, informes de laboratorio o hojas de cálculo de datos del paciente. ChatGPT le permite cargar archivos, ya sea PDF, CSV o sábanas de Excel, y analizar rápidamente su contenido. Puede resumir estudios complejos, extraer puntos clave de informes largos e incluso detectar tendencias en los resultados de laboratorio a lo largo del tiempo.
Esta característica es un gran ahorro de tiempo para los médicos que necesitan procesar grandes cantidades de información rápidamente. En lugar de pasar horas leyendo documentos densos, puede obtener resúmenes concisos y ideas procesables en minutos. Ya sea que esté revisando el historial del paciente, realizando investigaciones o analizando los datos del hospital, ChatGPT lo tiene cubierto.
Cómo usarlo:
- Haga clic en el 📎 Adjunto ícono en chatgpt.
- Cargue su archivo (por ejemplo, una hoja de cálculo con tendencias de BP del paciente).
- Instruya chatgpt en el análisis deseado (por ejemplo, “Resume los hallazgos clave de este estudio en lenguaje sencillo”.).
- Revise el resultado, incluidos resúmenes, cuadros o información específica de datos.
Descargo de responsabilidad: el análisis de datos de ChatGPT no debe reemplazar el juicio profesional o los requisitos de cumplimiento reglamentario.
4. Puedes realizar investigaciones profundas
Encontrar información médica confiable puede llevar mucho tiempo, pero Chatgpt’s Investigación profunda La función le permite navegar de forma autónoma fuentes de confianza y compilar informes estructurados. ¿Necesita una revisión de la literatura sobre los últimos tratamientos de hipertensión? ChatGPT puede recopilar información de múltiples fuentes y resumir los hallazgos clave en minutos.
Para los médicos que necesitan información basada en evidencia pero que no tienen tiempo para cavar en PubMed durante horas, esta característica es invaluable. Ya sea que se esté preparando para una presentación, escriba un trabajo de investigación o busque las últimas pautas clínicas, ChatGPT hace el trabajo pesado por usted.
Cómo usarlo:
- Abra chatgpt y habilitar Investigación profunda (Disponible para usuarios de Pro).
- Ingrese una solicitud como, “Genere una revisión de la literatura sobre los últimos avances en el tratamiento de diabetes tipo 2”.
- Permita 5-30 minutos para que ChatGPT compile un informe estructurado.
- Revise las citas y verifique los resultados antes de la aplicación clínica.
Descargo de responsabilidad: siempre una investigación generada por la IA de verificación cruzada con fuentes revisadas por pares antes de aplicarlo en la práctica.
5. Puede redactar y editar documentos de forma larga
Escribir informes largos, trabajos de investigación o pautas médicas puede ser desalentador, pero Chatgpt’s Lienzo La función proporciona un espacio de trabajo de edición interactivo. Está diseñado para redactar y refinar documentos de forma larga, lo que lo convierte en una excelente herramienta para profesionales médicos que necesitan producir informes o publicaciones detalladas.
En lugar de saltar entre varios procesadores de palabras, puede trabajar directamente dentro de ChatGPT, iterando el contenido con sugerencias con IA. Ya sea que esté redactando documentos de política, resúmenes de investigación o incluso materiales de educación del paciente, esta característica ayuda a mantener todo organizado y simplificado.
Cómo usarlo:
- Abra chatgpt y seleccione Modo de lienzo.
- Inicie un nuevo documento e ingrese su borrador.
- Use las herramientas de edición de ChatGPT para refinar secciones, mejorar la claridad y garantizar la legibilidad.
- Guarde o exporte la versión final para su envío o revisión.
6. Puedes usar características de voz e imagen
CHATGPT-4O ahora admite el análisis de entrada de voz y imagen, lo que hace que las interacciones sean más dinámicas y accesibles. Puede dictar notas con manos libres, subir imágenes para el reconocimiento de texto e interactuar con ChatGPT de manera más natural, ya sea que esté en movimiento o en una clínica ocupada.
Para los médicos, esto significa una documentación más fácil, un procesamiento de material de referencia más rápido y una mejor accesibilidad. Imagine dictar notas del paciente mientras conduce a casa o escanean notas escritas a mano para una transcripción instantánea; estas pequeñas eficiencias pueden sumar a un ahorro significativo de tiempo.
Para obtener un tutorial detallado sobre la creación de imágenes usando CHATGPT, explore esta guía.
Cómo usarlo:
- Activar modo de voz en Chatgpt para interacciones manos libres.
- Cargue imágenes (como notas de pacientes escritas a mano) para el reconocimiento de texto.
- Solicite a ChatGPT la transcripción u organización de la información clave.
Descargo de responsabilidad: el análisis de IA basado en imágenes no es un sustituto de la interpretación radiológica o patológica por profesionales capacitados.
Suscríbase para recibir los 7 pasos que puede seguir para lograr la libertad financiera
Si la libertad financiera es su objetivo, no hay mejor momento para comenzar que ahora.
Desbloquee los pasos procesables que puede tomar todos los días para ajustar sus objetivos, descubrir sus intereses y evitar errores costosos en su viaje de libertad financiera.
Conclusión
Si bien ninguna herramienta única puede eliminar las demandas del día de un médico, pueden sumar pequeñas ganancias de eficiencia. Ya sea automatización de recordatorios, resumiendo la investigación o la redacción de informes, estas características de ChatGPT pueden ayudar a optimizar las tareas y reducir la sobrecarga cognitiva.
Al incorporar la IA en su flujo de trabajo, no solo está ahorrando tiempo, sino que también está creando más ancho de banda para una atención significativa al paciente y un crecimiento profesional. Por cierto, si alguna vez ha tenido problemas para obtener los mejores resultados de la IA, esta hoja de trucos ChatGPT es una excelente manera de nivelar sus habilidades. Asegúrese de revisarlo.
¿Qué característica estás más emocionado de probar? ¡Hágamelo saber!
Suscríbete a nuestro boletín Para más IA y tecnología. También obtendrás acceso a nuestro página de recursos de IA gratisrepleto de herramientas de IA y tutoriales para ayudarlo a tener más en la vida fuera de la medicina. ¡Haz que suceda!
Descargo de responsabilidad: la información proporcionada aquí se basa en los datos públicos disponibles y puede no ser completamente precisa o actualizada. Se recomienda contactar a las respectivas empresas/individuos para obtener información detallada sobre características, precios y disponibilidad.
Si quieres más contenido como este, asegúrate de que Suscríbete a nuestro boletín Para obtener actualizaciones sobre las últimas tendencias para AI, tecnología y mucho más.
Peter Kim, MD es el fundador de Ingresos pasivos MDel creador de Academia de Bienes Raíces Passivey ofrece educación semanal a través de su podcast del lunes, el podcast MD Passive Income MD. Únase a nuestra comunidad en el Grupo de Facebook de Passive Income Doc Facebook.
Lectura adicional
Noticias
¿Qué es Mistral AI? Todo para saber sobre el competidor de Operai

Mistral AI, la compañía francesa detrás del asistente de IA LE Chat y varios modelos fundamentales, es considerada oficialmente como una de las nuevas empresas tecnológicas más prometedoras de Francia y posiblemente es la única compañía europea que podría competir con OpenAI. Pero en comparación con su valoración de $ 6 mil millones, su participación en el mercado global sigue siendo relativamente baja.
Sin embargo, el reciente lanzamiento de su asistente de chat en las tiendas de aplicaciones móviles se encontró con algunas exageraciones, particularmente en su país de origen. “Vaya y descargue le chat, que está hecho por Mistral, en lugar de chatgpt por OpenAi, o algo más”, dijo el presidente francés Emmanuel Macron en una entrevista televisiva antes de la Cumbre de Acción de AI en París.
Si bien esta ola de atención puede ser alentadora, Mistral AI todavía enfrenta desafíos para competir con personas como OpenAI, y al hacerlo mientras mantiene al día con su autodefinición como “el laboratorio de IA independiente más verde e líder del mundo”.
¿Qué es Mistral AI?
Mistral AI ha recaudado cantidades significativas de fondos desde su creación en 2023 con la ambición de “poner a la IA fronteriza en manos de todos”. Si bien este no es un jab directo en OpenAI, el eslogan está destinado a resaltar la defensa de la compañía para la apertura en la IA.
Su alternativa a ChatGPT, Asistente de chat LE Chat, ahora también está disponible en iOS y Android. Alcanzó 1 millón de descargas en las dos semanas posteriores a su lanzamiento móvil, incluso obtuvo el primer lugar de Francia para descargas gratuitas en la tienda de aplicaciones iOS.
Esto viene además del conjunto de modelos de Mistral AI, que incluye:
En marzo de 2025, la compañía introdujo Mistral OCR, una API de reconocimiento de carácter óptico (OCR) que puede convertir cualquier PDF en un archivo de texto para facilitar que los modelos de IA ingieran.
¿Quiénes son los fundadores de Mistral AI?
Los tres fundadores de Mistral AI comparten una experiencia en investigación de IA en las principales empresas de tecnología estadounidense con operaciones significativas en París. El CEO Arthur Mensch solía trabajar en DeepMind de Google, mientras que el CTO Timothée Lacroix y el director científico Guillaume Lample son ex empleados de Meta.
Los asesores cofundadores también incluyen a Jean-Charles Samuelian-Werve (también miembro de la junta) y Charles Gorintin de la startup de seguros de salud Alan, así como el ex ministro digital Cédric O, que causó controversia debido a su papel anterior.
¿Son los modelos de AI de Mistral de código abierto?
No todos ellos. Mistral AI diferencia a sus modelos principales, cuyos pesos no están disponibles para fines comerciales, de sus modelos gratuitos, para los cuales proporciona acceso de peso bajo la licencia Apache 2.0.
Los modelos gratuitos incluyen modelos de investigación como Mistral Nemo, que se construyó en colaboración con NVIDIA que la startup abierta en julio de 2024.
¿Cómo gana dinero Mistral AI?
Si bien muchas de las ofertas de Mistral AI son gratuitas o ahora tienen niveles gratuitos, Mistral AI planea generar algunos ingresos de los niveles pagados de Le Chat. Introducido en febrero de 2025, el plan Pro Chat tiene un precio de $ 14.99 al mes.
En el lado puramente B2B, Mistral AI monetiza sus modelos principales a través de API con precios basados en el uso. Las empresas también pueden licenciar estos modelos, y la compañía probablemente también genera una participación significativa de sus ingresos de sus asociaciones estratégicas, algunas de las cuales destacó durante la Cumbre de AI de París.
En general, sin embargo, los ingresos de Mistral AI todavía se encuentran en el rango de ocho dígitos, según múltiples fuentes.
¿Qué asociaciones ha cerrado Mistral Ai?
En 2024, Mistral AI entró en un acuerdo con Microsoft que incluía una asociación estratégica para distribuir sus modelos de IA a través de la plataforma Azure de Microsoft y una inversión de € 15 millones. La Autoridad de Competencia y Mercados del Reino Unido (CMA) concluyó rápidamente que el acuerdo no calificó para la investigación debido a su pequeño tamaño. Sin embargo, también provocó algunas críticas en la UE.
En enero de 2025, Mistral AI firmó un acuerdo con la agencia de prensa Agence France-Presse (AFP) para dejar que el chat consulte todo el archivo de texto de la AFP que data de 1983.
Mistral AI también aseguró asociaciones estratégicas con el ejército y la agencia de empleo de Francia, la startup de tecnología de defensa alemana Helsing, IBM, Orange y Stellantis.
¿Cuánta financiación ha recaudado Mistral AI hasta la fecha?
A partir de febrero de 2025, Mistral AI recaudó alrededor de € 1 mil millones en capital hasta la fecha, aproximadamente $ 1.04 mil millones al tipo de cambio actual. Esto incluye algunos financiamiento de la deuda, así como varias rondas de financiamiento de capital planteadas en una sucesión cercana.
En junio de 2023, y antes de que lanzara sus primeros modelos, Mistral AI recaudó una ronda récord de $ 112 millones de semillas dirigida por Lightspeed Venture Partners. Las fuentes en ese momento dijeron que la ronda de semillas, la más grande de Europa, valoraba la startup de entonces un mes de $ 260 millones.
Otros inversores en esta ronda de semillas incluyeron BPifrance, Eric Schmidt, Exor Ventures, First Minute Capital, Headline, Jcdecaux Holding, La Famiglia, Localglobe, Motier Ventures, Rodolphe Saadé, Sofina y Xavier Niel.
Solo seis meses después, cerró una serie A de € 385 millones ($ 415 millones en ese momento), a una valoración reportada de $ 2 mil millones. La ronda fue dirigida por Andreessen Horowitz (A16Z), con la participación de la velocidad de la luz de los patrocinadores existentes, así como BNP Paribas, CMA-CGM, Convicción, Elad Gil, Catalyst General y Salesforce.
La inversión convertible de $ 16.3 millones que Microsoft hizo en la IA Mistral como parte de su asociación anunciada en febrero de 2024 se presentó como una extensión de la Serie A, lo que implica una valoración sin cambios.
En junio de 2024, Mistral AI luego recaudó 600 millones de euros en una combinación de capital y deuda (alrededor de $ 640 millones al tipo de cambio en ese momento). La ronda de larga data fue dirigida por Catalyst General con una valoración de $ 6 mil millones, con inversores notables, incluidos Cisco, IBM, Nvidia, Samsung Venture Investment Corporation y otros.
¿Cómo podría ser una salida de IA distral?
Mistral está “no a la venta”, dijo Mensch en enero de 2025 en el Foro Económico Mundial en Davos. “Por supuesto, [an IPO is] el plan “.
Esto tiene sentido, dado cuánto ha recaudado la startup hasta ahora: incluso una venta grande puede no proporcionar múltiplos lo suficientemente altos para sus inversores, sin mencionar las preocupaciones de soberanía dependiendo del adquirente.
Sin embargo, la única forma de definitivamente aplastar rumores de adquisición persistentes es escalar sus ingresos a niveles que incluso podrían justificar remotamente su valoración de casi $ 6 mil millones. De cualquier manera, estad atentos.
Esta historia se publicó originalmente el 28 de febrero de 2025 y se actualizará regularmente..
Noticias
Comparing Google Veo 2 And OpenAI Sora in 2025

Google Veo 2 vs OpenAI Sora – which AI video tool comes out top?
It’s impossible to scroll through social media or attend any technology conference without encountering the dramatic shift happening in video production. Text-to-video AI has arrived, and the titans of tech are racing to bring their versions to market. At the forefront of this revolution are two powerhouse tools–OpenAI’s Sora (released in the UK and EU just this Friday) and Google’s Veo 2—each representing vastly different visions for the future of digital content creation. The implications for industries from fashion to gaming, advertising to independent filmmaking are profound and immediate.
Sora vs Veo 2: Two Visions for AI-Generated Video
Since both tools are relatively new to the market, certainly with UK and EU audiences, I spoke to three different expert users who have had early access to these tools for a number of months to tell me about their experiences with them and to compare and contrast their relative merits and features. My key takeaway is that the battle between Sora and Veo 2 isn’t just about technical specs—it’s a clash of philosophies. One aims to replicate reality, the other to transcend it. These tools represent a pivotal moment where the barriers between imagination and execution are dissolving at an unprecedented rate.
The contrast between Sora and Veo 2 represents more than just competing products—it embodies divergent philosophies about what matters most in creative tools. OpenAI has prioritized user interface and control, while Google has focused on output quality and physics simulation.
“Sora has a huge advantage, because they put a lot of work into the interface and the user interface,” explains David Sheldrick, founder at PS Productions and Sheldrick.ai, who is an early tester of both platforms. “Veo 2, even though the rendering output quality is obviously incredible…Sora itself, when you go on the website, feels way more like a real, sort of refined product.”
This distinction becomes immediately apparent to users encountering both platforms. Sora offers a comprehensive suite of creator-friendly features—timelines, keyframing, and editing capabilities that feel familiar to anyone with video production experience. It prioritizes creative control and workflow integration over raw technical performance.
OpenAI’s Sora video model launch caused a lot of excitement
Leo Kadieff, Gen AI Lead Artist at Wolf Games, a studio pioneering AI-driven gaming experiences, has also had early access to both platforms and describes Veo 2 as “phenomenal, with web access, and API access which enables much more experimental stuff. It’s really the number one tool”. His enthusiasm for Veo 2’s capabilities stems from its exceptional output quality and physics modeling, even if the interface isn’t as polished as Sora’s.
This reflects a key question for creative tools: is it better to provide a familiar, robust interface or to focus on generating the highest quality outputs possible? The answer, as is often the case with emerging technologies, depends entirely on what you’re trying to create.
Technical Strengths: Physics, Consistency and Hallucinations
The real-world performance of these tools reveals their distinct technical approaches. Sora impresses with its cinematic quality and extended duration capabilities, while Veo 2 excels at physics simulation and consistency.
“The image quality is pretty damn good,” notes Sheldrick about Veo 2, while adding that “Sora already has nailed photo realism. It’s got this image fidelity, which is super, super high.” Both platforms are clearly pushing the boundaries of what’s possible, but they handle technical challenges differently.
One particularly revealing area is how each platform deals with the “hallucinations” inherent to AI generation—those moments when the physics or continuity breaks down in unexpected ways.
Kadieff explains the difference vividly: “When Veo 2 hallucinates, it just clips to kind of like a similar set that it has in its memory, but you might lose, like, consistency, or you might get a whole different, weird angle. So, for example, if you make a drone shot flying over a location, and it’s like 10 seconds, it will do five seconds perfectly, and then it’s going to clip to some rainforest”.
Bilawal Sidhu, a creative technologist and AI/VFX creator on YouTube and other platforms, with over a decade of experience, doesn’t mince words about Sora’s limitations: “the physics are completely borked, like, absolutely horrendous”. He explains that while Sora offers longer duration videos (10-15 seconds), its physical simulation often falls short, particularly with human movement and interactions.
Speaking on his YouTube channel, Sidhu declares, “Nothing comes close to what Google Deep Mind has dropped… Veo 2 now speaks cinematographer. You can ask for a low angle tracking shot 18 mm lens and put a bunch of detail in there and it will understand what you mean. You just ask it with terms you already know… I feel like Sora doesn’t really follow your instructions. Sora definitely does pretty well at times, but in general it tends to be really bad at physics.”
Behind every AI video generator lies mountains of training data that shapes what each tool excels at creating. Hypothesising why the physics outputs of Veo 2 are superior in the video outputs, he states, “Google owns YouTube, and so even if you pull out a bunch of the copyrighted stuff, that still leaves a massive corpus compared to what anyone else has to train on.”
The battle for training data supremacy extends beyond quantity to quality and diversity. OpenAI has remained relatively secretive about Sora’s full training dataset, raising questions about potential biases and limitations.
For commercial applications where physical accuracy is non-negotiable, this distinction matters enormously. Video quality and physical realism are essential for products that need to be represented accurately, highlighting why industries with strict visual requirements might lean toward Veo 2 despite its more limited interface.
Sora vs Veo 2: Prompt Control and Generation Quality
By coming out first, Sora had a first-mover advantage of sorts, but it also set the bar for other models to work towards—and then transcend. Sidhu was very impressed when he first saw the outputs: “watching the first Sora video, the underwater diver discovering like a crashed spaceship underwater, if you remember that video, that blew my mind, because I feel like Sora showed us that you could cross this chasm of quality with video content that we just hadn’t seen.”
Explaining more of the positives for Sora, Sidhu adds, “Sora is very powerful. Their user experience is far better than their actual quality. They’ve got this like storyboard editor view, where you can basically lay out prompts on a timeline—you can outline, hey, I want a character to enter, the scene from the left, walk down and sit down on this table over here, and then at this point in time, I want somebody else to walk up and suddenly get their attention.”
The ability to translate text prompts into intended visuals varies significantly between platforms. Veo 2 appears to be winning the battle for prompt adherence—the ability to faithfully translate textual descriptions into corresponding visuals.
“Veo 2 is very good at prompt adherence, you can give very long prompts, and it’ll kind of condition the generation to encapsulate all the things that you asked for,” Sidhu explains, expressing genuine surprise at Veo 2’s capabilities. “Like Runway and Luma, and pretty much anything that you’ve used out there, the hit rate is very bad… for Veo 2, it is by far the best. It’s like, kind of insane, how good it is”.
This predictability and control fundamentally changes the user experience. Rather than treating AI video generation as a slot machine where creators must roll repeatedly hoping for a usable result, Veo 2 provides more consistent, controlled outputs—particularly valuable for commercial applications with specific requirements.
Consistency extends beyond single clips as well. Sidhu notes that “the four clips you get [as an output from Veo 2], you put in a text prompts, as long as you want them to be, and with a very detailed text prompt, you get very close to character consistency too”, allowing for multi-clip productions featuring the same characters and settings without dramatic variations.
Kadieff is also a huge fan of Veo 2’s generation quality: “”Veo 2 has generally been trained on very good, cinematic content. So almost like all the shots you do with it feel super cinematic, and the animation quality is phenomenal.”
Beyond this, the resolution quality of Veo 2’s outputs is also a cause for celebration, as Sidhu states, “this model can natively output 4K. If you used any other video generation tool, Sora, Luma, whatever it is, you end up exporting your clips into some other upscaling tool whether that’s Krea or Topaz, what have you — this model can do 4K natively, that’s amazing.”
Industry Applications: From Fashion to Gaming
Different industries are discovering unique applications for these tools, with their specific requirements guiding platform selection. Fashion brands prize consistency and physical accuracy, while gaming and entertainment often value creative flexibility and surrealism.
“What I’m really excited about is not just the ability, indies are going to be able to rival the outputs of studios, but studios are going to set whole new standards,” says Sidhu. “But then also, these tools are changing the nature of content itself, like we’re moving into this era of just-in-time disposable content.”
For fashion and retail, the ability to quickly generate variations of a single concept represents enormous value. Creating multiple versions of product videos tailored to different markets is now possible without the expense of multiple production shoots.
Meanwhile, gaming and entertainment applications embrace different capabilities. Kadieff describes how AI is transforming creative approaches: “The intersection of art, games and films, is not just about games and films anymore – it’s about hybrid experiences”. This represents a fundamental shift in how interactive media can be conceived and produced.
Sheldrick predicts significant industry adoption this year: “I think this is the year that AI video and AI imagery in general will kind of break into the advertising market and a bit more into commercial space.” He warns that “the companies that have got on board with it, will start to reap the rewards, and the companies that have neglected to take this seriously, will suffer in this year.”
The Human-AI Collaboration Model
Despite these tools’ remarkable capabilities, the most successful implementations combine AI generation with human creativity and oversight. The emerging workflow models suggest letting AI handle repetitive elements while humans focus on the aspects requiring artistic judgment.
As these platforms continue to develop, creative teams are adapting how they work, with new hybrid roles emerging at the intersection of traditional creativity and technical AI expertise.
The learning curve remains steep, but the productivity gains can be substantial once teams develop effective workflows. Kadieff notes how transformative these tools have been: “when I saw transformer-based art, like three, four years ago, I mean, it changed my life. I knew instantly that this is the biggest media transformation of my lifetime”.
Looking Forward: AI Video in 2026 and Beyond
As these platforms continue evolving at breakneck speed, our experts envision transformative developments over the next few years. Specialized models tailored to specific industries, greater customization capabilities, and integration with spatial computing all feature prominently in their predictions.
With Sidhu’s earlier visions of independent creators rivalling the outputs of studios, this democratization of high-quality content creation tools doesn’t mean the end of major studios, but rather a raising of the bar across the entire creative landscape.
Sheldrick remains enthusiastic about the competitive landscape driving innovation: “I’m just most excited to watch these massive, sort of frontier labs just going at it. I’ve enjoyed watching this sort of AI arms race for years now, and it hasn’t got old. It’s still super exciting.”
David Sheldrick has used OpenAI’s Sora tool to create fashion videos
Perhaps the most transformative potential lies in how these tools will reshape our understanding of content itself. As Sidhu explains, “I think content authoring will look almost like a world model, one of the characteristics or attributes of it is like, here’s a scene graph, here are the three scenes that I have. Here are the characters that are within it. Here are the props. Here’s the time of day”. This structured approach would allow content to be personalized and localized at unprecedented scales.
The Democratization of Visual Storytelling
As we look toward the future of AI-generated video, it’s clear that neither Sora nor Veo 2 represents a definitive solution for all creative needs. The choice depends on specific requirements, risk tolerance, and creative objectives.
What’s undeniable is the democratizing effect these tools are having on visual storytelling. “Now we’re coming to a place where everybody, anybody with an incredible imagination, whether they’re in India, China, Pakistan or South Africa, or anywhere else, and access to these tools can tell incredible stories,” Kadieff observes.
Sidhu agrees, noting that “YouTube creators are punching way above their weight class already. And so I think that trend is going to continue, where we’ll see like the Netflix’s of the world look a lot more like YouTube, where more content is going to get greenlit”.
These tools are enabling a new generation of creators to produce content that would have been prohibitively expensive just a few years ago. The traditional barriers to high-quality video production are falling rapidly.
As AI video tools like Sora and Veo 2 continue to evolve and become increasingly accessible, we stand at the beginning of a fundamental shift in how visual stories are told, who gets to tell them, and how they reach their audiences. The tools may be artificial, but the imagination they unlock is profoundly human.
-
Startups10 meses ago
Remove.bg: La Revolución en la Edición de Imágenes que Debes Conocer
-
Recursos10 meses ago
Cómo Empezar con Popai.pro: Tu Espacio Personal de IA – Guía Completa, Instalación, Versiones y Precios
-
Tutoriales10 meses ago
Cómo Comenzar a Utilizar ChatGPT: Una Guía Completa para Principiantes
-
Recursos10 meses ago
Suno.com: La Revolución en la Creación Musical con Inteligencia Artificial
-
Startups8 meses ago
Startups de IA en EE.UU. que han recaudado más de $100M en 2024
-
Recursos10 meses ago
Perplexity aplicado al Marketing Digital y Estrategias SEO
-
Startups10 meses ago
Deepgram: Revolucionando el Reconocimiento de Voz con IA
-
Estudiar IA10 meses ago
Curso de Inteligencia Artificial de UC Berkeley estratégico para negocios