Noticias
Tackling Insomnia Via Generative AI And ChatGPT
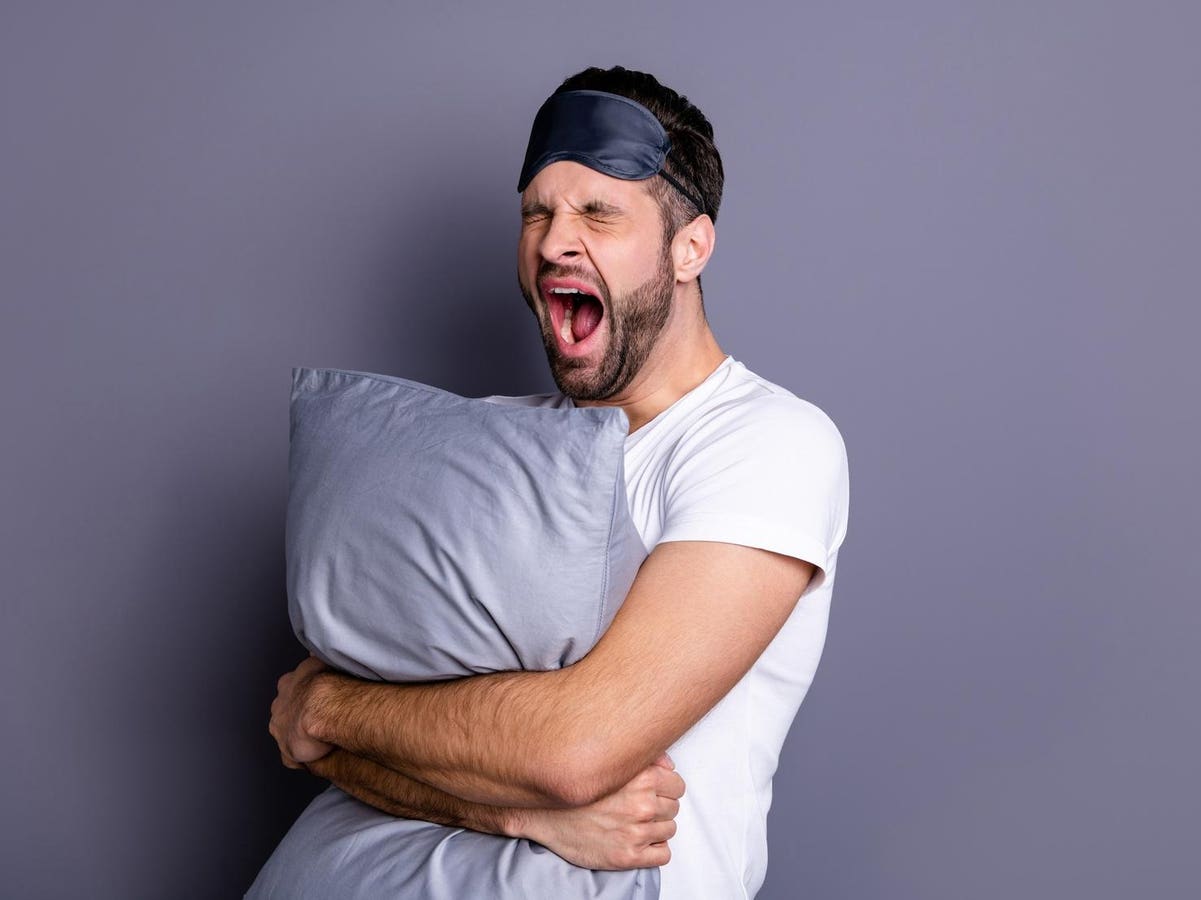
Insomnia is quite a beast — modern-times generative AI is seeking to tame the sleeping difficulties … [+]
Insomnia is an eye-opening problem (pardon the pun!).
There is no doubt that insomnia is a highly serious challenge. Being unable to sleep is certainly a disconcerting and most pressing issue that we all have faced. From time to time, it seems that bouts of sleep deprivation are bound to strike any of us in this hectic world we live in. Work pressures, family issues, and the general sense of the planet being on edge are enough to wreck our sleep patterns.
What you might not be aware of is that an estimated 10% to possibly 30% of adults are at some point in time dealing with chronic insomnia. This is far beyond occasional or episodic insomnia. Chronic insomnia is typically classified as a disorder, reflecting the seriousness and severity that it can inflict on your physical body and your mental well-being.
There are lots of suggested ways to cope with insomnia.
I bet you’ve heard, seen, and possibly tried many. Some say that you can mentally will yourself out of insomnia. Various therapeutic techniques purport to do so. Rarely are you able to do this entirely on your own. It is customary to seek out professional mental health guidance. In addition, medications sometimes can play an important role too, though you should be cautious about taking medications unless you’ve got a suitably prescribed approach.
Let’s go ahead and add another option for tackling insomnia.
Are you sitting down and ready for what it is?
Okay, the option is generative AI.
That’s right, include generative AI such as ChatGPT, GPT-4, Claude, Gemini, and other popular generative AI apps on your list of presumed solution possibilities for conquering insomnia. A vital point to clarify is that generative AI should not be overstated or classified as a remedy or cure per se. It is simply another tool in the toolbox of ways to combat and defeat insomnia. No silver bullet on this front.
I trust that you are intrigued about how generative AI in some sensible manner can be utilized to cope with insomnia. I am quite glad that you asked, thanks.
I will walk you through essential background about insomnia and dovetail how generative AI enters newly into the picture. The aim is to be informative, reveal something you probably didn’t know, and showcase that modern-day generative AI is worthy of being included in any regimen or method of coping with insomnia.
Before we leap into the details, allow me to go into my customary opening remarks.
For my ongoing readers, in today’s column, I am continuing my in-depth series about the impact of generative AI in the health and medical realm. The focus this time is once again on the mental health domain and examines the use of generative AI for coping with insomnia.
I have previously examined numerous interleaving facets of generative AI and mental health, see my comprehensive overview at the link here. You might also find of notable interest a CBS 60 Minutes episode that recently examined crucial facets of this evolving topic, see the link here (I am honored and pleased to indicate that I was featured in the episode, see the link here).
Other vital background includes my in-depth coverage of mental health chatbots which have been bolstered by generative AI (see the link here) and the rapidly changing nature of the client-therapist relationship due to generative AI at the link here. I explored where things are headed regarding the levels of AI-based mental therapy autonomous guidance at the link here, and showcased the importance of the World Health Organization (WHO) report on global health and generative AI at the link here, and so on.
Research On Insomnia Tells Quite A Story
We shall begin by taking a quick glimpse at some key timely research covering insomnia.
An esteemed medical journal recently explored the latest research and insights about insomnia, doing so in a published paper entitled “Management of Insomnia” by Charles Morin and Daniel J. Buysse, The New England Journal Of Medicine, July 2024. Here are some key points (excerpts):
- “Insomnia disorder is characterized by dissatisfaction with sleep quality or duration associated with difficulty falling or staying asleep and substantial distress or daytime impairments.”
- “The disorder is a sleep disturbance that occurs 3 nights or more per week, persists for more than 3 months, and is not the result of inadequate opportunities for sleep.”
- “It frequently co-occurs with other medical conditions (e.g., pain) and psychiatric disorders (e.g., depression), as well as other sleep disorders (e.g., restless legs syndrome and sleep apnea).”
- “Insomnia is the most prevalent sleep disorder in the general population and among the most frequent issues raised by patients during primary care visits, although it often goes untreated. Approximately 10% of adults meet the criteria for insomnia disorder and another 15 to 20% report occasional insomnia symptoms.”
- “Current treatment options for insomnia include prescribed and over-the-counter medications, psychological and behavioral therapies (also referred to as cognitive behavioral therapy for insomnia [CBT-I]), and complementary and alternative therapies.”
You might closely observe that when sleep is a disorder there is a qualitative and quantitative measurement involved, such as the second point above noting that a considered severe or chronic sleep disturbance occurs at least 3 nights per week and persists for 3 months or more. That’s the really rough-and-tumble side of insomnia.
The fourth bullet point reveals an aspect that you might find surprising.
According to the researchers, when patients visit their primary care physician, sleep issues are a frequently cited concern by the patient. Have you done the same? Meanwhile, lamentedly, sleep difficulties tend to be less likely viewed as something for treatment, unless the lack of sleep has already risen to a debilitating level. Some would argue that this is the classic oversight of trying to catch the horse after it is already out of the barn.
The fifth bullet point mentions that besides medications, various psychological and behavioral therapies are often employed. One that gets the most attention is known as CBT-I, cognitive behavioral therapy for insomnia. I’ll be saying more about this in a moment.
CBT-I used to be mainly done via in-person visits with a mental health professional or clinician. Nowadays, in our online world, the use of remote options exists, including apps or digital CBT-I which is typically referred to as eCBT-I.
There are specialized apps that are built specifically to guide you via the precepts of CBT-I. Other times, a mental health app will cover a variety of behavioral realms and happen to include CBT-I amid the many others via the app. Another possibility is using eCBT-I in conjunction with a mental health professional, such that you are presumably getting the best of both worlds.
The cited research paper provides a handy set of details about the range of psychological and behavioral therapies for patients with insomnia, and identifies these major areas:
- Sleep restriction
- Stimulus control
- Relaxation training
- Cognitive therapy
- Sleep hygiene education
- Acceptance and commitment therapy
- Mindfulness
- Brief behavioral treatments for insomnia
Not only do eCBT-I specialized apps tend to cover those areas, but you might be pleasantly surprised to know that generic generative AI can usually provide similar capabilities. For my extensive coverage of how generic generative AI for mental health use is different from and at times similar to specialized mental health apps, see the link here and the link here, just to mention a few.
I will in a moment walk you through the use of modern-day generative AI for serving as a handy tool for coping with insomnia.
First, let’s talk in general about generative AI and large language models (LLMs), doing so to make sure we are on the same page when it comes to discussing the matter at hand.
Generative AI And Tackling Of Insomnia
I’m sure you’ve heard of generative AI, the darling of the tech field these days.
Perhaps you’ve used a generative AI app, such as the popular ones of ChatGPT, GPT-4o, Gemini, Bard, Claude, etc. The crux is that generative AI can take input from your text-entered prompts and produce or generate a response that seems quite fluent. This is a vast overturning of the old-time natural language processing (NLP) that used to be stilted and awkward to use, which has been shifted into a new version of NLP fluency of an at times startling or amazing caliber.
The customary means of achieving modern generative AI involves using a large language model or LLM as the key underpinning.
In brief, a computer-based model of human language is established that in the large has a large-scale data structure and does massive-scale pattern-matching via a large volume of data used for initial data training. The data is typically found by extensively scanning the Internet for lots and lots of essays, blogs, poems, narratives, and the like. The mathematical and computational pattern-matching homes in on how humans write, and then henceforth generates responses to posed questions by leveraging those identified patterns. It is said to be mimicking the writing of humans.
I think that is sufficient for the moment as a quickie backgrounder. Take a look at my extensive coverage of the technical underpinnings of generative AI and LLMs at the link here and the link here, just to name a few.
Back to the crux of things.
As you might imagine, generative AI can be handy for aiding those who are concerned about insomnia overall. This includes a wide array of stakeholders, including adults, children, therapists, policymakers, regulators, and many others.
Here are some significant ways that generative AI can be useful:
- (1) Generative AI – Insomnia Detection. Generative AI can potentially detect that someone seems to have insomnia or might be heading in that direction.
- (2) Generative AI – Insomnia Mental Health Advisement. Generative AI can conversationally guide someone through the mental health dynamics of insomnia and serve as a kind of therapist in a limited way.
- (3) Generative AI – Insomnia Education. Generative AI can educate people about insomnia, such as explaining what insomnia is, what to do about insomnia, etc.
- (4) Generative AI – Scenario Simulation Of Insomnia Impacts. Generative AI can showcase what insomnia can do in terms of mental health ramifications arising due to insomnia, doing so by taking on or simulating a persona, see examples of generative AI persona usage at the link here.
- (5) Generative AI – Policymaking On Insomnia. Generative AI can contribute to the policymaking sphere about insomnia from a medical/legal perspective. This can be done by aiding in crafting policies, reviewing policies, and serving as a handy tool throughout the policymaking process.
- (6) Generative AI – Other Insomnia Aspects. Generative AI can be used in a variety of other ways that might aid in dealing with insomnia, though there is always a chance that generative AI won’t be helpful, or worse still, might be misapplied and misinterpreted.
That’s an impressive array of potential uses.
Allow me a brief moment to stand on a soapbox and make some important remarks about the mental health uses of generative AI. It is a topic I’ve been covering extensively, such as the link here and the link here.
We are right now in a somewhat wanton grand experiment of using generic generative AI for mental health purposes. Insomnia is one instance of how generative AI can be applied for mental health advisement. The thing is, no one can say whether using generic generative AI for mental health uses will ultimately be for the good or the bad. In a sense, we have mental health advisement via the auspices of generative AI that is taking place under our noses at a massive scale, and we can’t say how it will pan out.
The population-level impacts are either amazing or chilling.
Okay, I provided my warnings, so I’ll get down from the soapbox, and we can proceed with considering the upsides and downsides of generative AI in this realm.
Using ChatGPT To Showcase The Use Of Generative AI
I will next proceed to examine further the use of generative AI for insomnia.
This will consist of a series of dialogues with ChatGPT. ChatGPT is a logical choice in this case due to its immense popularity as a generative AI app. As noted, an estimated one hundred million weekly active users are said to be utilizing ChatGPT. That’s a lot of people and a lot of generative AI usage underway.
A few quick comments before we launch into using ChatGPT.
If you are going to try to do the same prompts that I show here, realize that the probabilistic and statistical properties will likely produce slightly different results than what I show here. That’s the nature of generative AI and how it is devised.
You also should expect that different generative AI apps will respond in different ways. Not all generative AI apps are the same. The key is that sometimes a particular prompt will work in one generative AI app and not another. Nonetheless, the odds are relatively high that you will get roughly similar responses from all the major generative AI apps such as GPT-4, Gemini, Bard, Claude, etc.
There is also a timing factor to be considered. A prompt that worked today might not work the same way tomorrow. Most of the generative AI apps are continually being updated. The updates might alter internal mechanisms that could change how the generative AI reacts to your prompting.
We are ready to get underway with ChatGPT.
I opted to start by asking ChatGPT what insomnia is.
As an aside, whenever you are starting a conversation with generative AI, I recommend as a prompt engineering technique to begin by asking a question that will establish if the AI has been data-trained on the topic at hand. A generated response that is vacuous will give you a heads-up that you might be barking up the wrong tree with that generative AI app.
Here we go.
- My entered prompt: “What is insomnia?”
- ChatGPT generated response: “Insomnia is a sleep disorder characterized by difficulty falling asleep, staying asleep, or getting restful sleep, leading to daytime fatigue and impairment.”
I’d say that the response by ChatGPT was an apt description, short and to the point.
That’s an encouraging sign that ChatGPT has likely scanned the topic during the initial data training phase of development. This makes indubitable sense. The odds are that while scanning the Internet for content to pattern match on, invariably content associated with the popular topic would have been encountered.
Moving on, let’s see what ChatGPT has to say about some deeper facets.
- My entered prompt: “How prevalent is insomnia? And what are potential mental health problems that can arise due to insomnia?”
- ChatGPT generated response:
“Insomnia is a common sleep disorder affecting a significant portion of the population. Research indicates that approximately 10-30% of adults experience chronic insomnia, with some studies suggesting prevalence rates as high as 50-60% for occasional insomnia. Insomnia is more prevalent among women and older adults. For instance, up to 50% of elderly people may suffer from insomnia.”
“Insomnia can have several negative impacts on mental health, including:”
“Depression: Chronic insomnia is strongly associated with an increased risk of developing depression. Individuals with insomnia are ten times more likely to develop depression compared to those without sleep issues.”
“Anxiety: Insomnia can exacerbate anxiety disorders. Lack of sleep can heighten anxiety symptoms and make it more difficult for individuals to cope with stress.”
“Cognitive Impairments: Persistent insomnia can lead to problems with concentration, memory, and decision-making, which can affect daily functioning and work performance.”
“Mood Disorders: Insomnia can cause mood swings and irritability. The lack of restorative sleep can affect emotional regulation and lead to mood disorders.”
“Substance Abuse: People with insomnia might turn to alcohol or other substances as a means to induce sleep, leading to potential substance abuse and dependency issues.”
“Addressing insomnia through behavioral interventions, cognitive-behavioral therapy for insomnia (CBT-I), and, when necessary, medication can help mitigate these mental health risks.”
End of ChatGPT response
The response by ChatGPT is solid.
I say this because it is vital to always double-check any output from generative AI. There is a chance that generative AI can make up fictitious facts, often referred to as AI hallucinations, see my coverage at the link here. In this case, based on the literature on insomnia, the indications by ChatGPT are sound.
We are now ready to ask the zillion-dollar question.
- My entered prompt: “Can generative AI help someone cope with insomnia?”
- ChatGPT generated response:
“Yes, generative AI can help someone cope with insomnia by providing personalized relaxation techniques, sleep hygiene tips, and generating calming content such as bedtime stories or soothing sounds. It can also offer cognitive behavioral therapy (CBT) strategies to address underlying thoughts and behaviors contributing to insomnia.”
“Here are some specific ways generative AI can help cope with insomnia:”
“Personalized Sleep Hygiene Tips: AI can analyze individual sleep patterns and suggest personalized routines to improve sleep quality, such as optimal bedtime, wake-up times, and pre-sleep activities.”
“Guided Relaxation and Meditation: Generative AI can create custom guided meditations or relaxation exercises to help calm the mind before sleep.”
“Calming Content Generation: AI can generate bedtime stories, white noise, or soothing music tailored to individual preferences, promoting a more relaxing sleep environment.”
“Cognitive Behavioral Therapy for Insomnia (CBT-I): AI can provide interactive CBT-I sessions, helping users identify and change thoughts and behaviors that negatively impact sleep.”
“Sleep Tracking and Analysis: AI-powered apps can track sleep patterns and provide insights and recommendations based on the data collected.”
“Stress and Anxiety Management: AI can offer tools and exercises to manage stress and anxiety, which are common contributors to insomnia.”
“Virtual Sleep Coaches: AI can act as a virtual sleep coach, offering ongoing support and adjustments to sleep strategies as needed.”
End of ChatGPT response
I believe that to be a handy list of the ways that generative AI can be beneficial in coping with insomnia. The list generally comports with my list, shown earlier, though providing a more detailed look at the topic.
Doing A Therapeutic Dialogue With ChatGPT About Insomnia
I’ve used generative AI for nearly all the listed approaches, having done so not for personally having insomnia but as a tryout of generative AI for said therapeutic purposes. I will go ahead and show you a sample dialogue to give you a sense of what this kind of usage consists of. Note that I will be pretending to have insomnia.
Here we go.
- My entered prompt: “I’ve been having trouble sleeping lately. Can you help me?”
- ChatGPT generated response: “Of course! I’m here to help. Can you describe your sleep difficulties? Are you having trouble falling asleep, staying asleep, or both?”
- My entered prompt: “Mostly falling asleep. I just can’t seem to shut my mind off at night.”
- ChatGPT generated response: “That sounds frustrating. Let’s start with some relaxation techniques. Would you like to try a guided meditation or some breathing exercises?”
I’d like you to observe some key facets shown via this dialogue.
First, you might have noticed that generative AI goes along with my remark that I am having trouble sleeping. A fellow human might right away doubt you or otherwise not take seriously your expressed concern. In a sense, you could say I am being heard by ChatGPT. Happy face.
Second, I was right away asked useful probing questions.
The initial question was a broad one. The accompanying question was more specific, namely asking about whether I had trouble falling asleep, staying asleep, or both. This one-two punch using a general question in combination with a more specific question is a great way to get someone to suitably respond. You see, if you ask only a general question, you are bound to get a general answer. If you ask only a more specific question, you might be diving too fast into the depths of the matter. Having both questions posed is a good approach.
Third, after I gave my answer, an expression of empathy was given (“That sounds frustrating”), which is an important means of getting the person to further open up. For my analysis of how generative AI can appear to be empathetic, see my analyses at the link here and the link here.
Let’s see what happens next.
- My entered prompt: “A guided meditation sounds good.”
- ChatGPT generated response: “Great! Here’s a short, guided meditation to help you relax and prepare for sleep…” [Note: I’ve clipped the dialogue for space purposes].
- My entered prompt: “Thanks, that was helpful. What else do you advise?”
- ChatGPT generated response: “Next, let’s work on your bedtime routine. Do you have any habits before bed, like using screens or consuming caffeine?”
- My entered response: “I often use my smartphone before bed, and I drink coffee in the afternoon.”
- ChatGPT generated response: “Using screens can interfere with sleep. Try to put away your smartphone at least an hour before bed. Also, consider switching to a decaf coffee or avoiding caffeine after noon. How does that sound?”
- My entered response: “I can give that a try.”
The dialogue went on for quite a while.
I am cutting it there for space purposes herein. You can readily try the same type of conversation by creating an account in a generative AI app and proceeding as I’ve demonstrated.
Be Mindful And Cautious In Your Use Of Generative AI
Do not falsely assume that generative AI will be a silver bullet for dealing with insomnia.
Besides the fact that you are interacting with a generic AI system rather than a mental health professional, there are other potential issues to keep in mind.
Let’s get generative AI to fess up to those concerns.
- My entered prompt: Might the use of generative AI for coping with insomnia have any drawbacks or downsides?”
- ChatGPT generated response:
“Yes, there are potential drawbacks and downsides to using generative AI for coping with insomnia.”
“Over-Reliance: Users might become too dependent on AI for sleep support, which could hinder their ability to develop and maintain healthy sleep habits independently.”
“Privacy Concerns: AI systems often require personal data to provide tailored advice. This data collection can raise privacy and security issues if not properly managed.”
“Effectiveness: AI interventions might not be as effective as personalized treatment from a healthcare professional, especially for complex or severe cases of insomnia.”
“Generalization: AI-generated advice may not always account for individual nuances and might offer generalized solutions that don’t fully address specific needs.”
“Lack of Human Touch: AI lacks the empathy and understanding that a human therapist or counselor can provide, which may be crucial for some individuals dealing with insomnia.”
“Potential Misinformation: AI systems can sometimes provide incorrect or outdated information, which could negatively impact sleep hygiene or overall health.”
End of ChatGPT response
I wholeheartedly agree with those stated downsides.
Consider this mindfully.
For example, please do not tell someone to use generative AI for insomnia without also mentioning and cautioning about the downsides. People going on this path need to be fully informed about what generative AI can and cannot do.
One aspect that was mentioned consists of privacy or the lack thereof.
A lot of people seem to think that when they use generative AI, they are guaranteed total privacy and confidentiality. This is a highly unlikely assumption. The licensing agreements usually say that the AI maker can readily access your prompts and anything else that you’ve entered into the generative AI app. Furthermore, the licensing typically indicates that they can use your entered content as an additional form of data training for the AI. See my detailed discussion on this disconcerting matter of privacy intrusions and what to watch out for, at the link here.
Conclusion
Congratulations, you are now up-to-speed about the use of generative AI for coping with insomnia.
There is still a long way to go on this topic. The clever idea of applying modern-day generative AI to aid in overcoming insomnia is only in its infancy. Join in and help advance the research in this budding and promising realm. You can make a difference.
There are controversies afoot and grand challenges ahead.
For example, some would fervently insist that no one should ever turn to generative AI for this purpose unless they are doing so under the vaunted guidance of a mental health professional. What do you think? For now, you get to be the judge. See my discussion on the latest in coupling human-based therapy with AI-based therapy to know more about the ins and outs involved, at the link here.
A final remark or two for now.
F. Scott Fitzgerald famously said, “The worst thing in the world is to try to sleep and not to.” I dare say that most of us have learned that lesson the hard way. Even if you don’t have chronic insomnia, the occasional episodic insomnia due to say jet lag can be seemingly unbearable. Sleep is vital.
Let’s end the discussion with some revered Shakespeare: “O sleep, O gentle sleep, Nature’s soft nurse, how have I frightened thee. That thou no more will weigh my eyelids down.”
Maybe a bit of poetry will help with this, and I wish you all the best in your everyday and much-needed sleeping endeavors.
Noticias
OpenAI elimina datos clave y se intensifica la demanda por derechos de autor

Un acontecimiento reciente en la batalla legal en curso entre OpenAI y los principales medios de comunicación, incluidos Los New York Times y Noticias diariasha llamado la atención en las comunidades tecnológica y legal.
Los abogados que representan a estos editores afirman que los ingenieros de OpenAI eliminaron accidentalmente datos cruciales que podrían haber respaldado su caso en una demanda que acusa a la empresa de inteligencia artificial de extraer contenido protegido por derechos de autor para entrenar sus modelos sin permiso.
Este incidente pone de relieve la tensión entre las tecnologías emergentes de IA y los derechos de propiedad intelectual. Esto es lo que sucedió y por qué es importante.
¿Qué pasó? El incidente de eliminación de datos
En un giro inusual, los ingenieros de OpenAI borraron los datos almacenados en una máquina virtual configurada para que los editores buscaran su material protegido por derechos de autor.
La demanda gira en torno a afirmaciones de que OpenAI utilizó artículos con derechos de autor, incluidos los de The New York Times y Daily News, para entrenar sus modelos de IA, como GPT-4, sin obtener los permisos o licencias necesarios.
En otoño, OpenAI acordó proporcionar dos máquinas virtuales a los equipos legales de los demandantes. Estas máquinas permitirían a los expertos buscar en los vastos conjuntos de entrenamiento de IA de OpenAI para ver si el contenido de las empresas se había utilizado sin consentimiento.
Después de semanas de búsqueda, los expertos habían acumulado más de 150 horas de trabajo, hasta que los datos fueron eliminados el 14 de noviembre.
Los abogados de los editores presentaron una carta en el Tribunal de Distrito de EE. UU. para el Distrito Sur de Nueva York, explicando que los ingenieros de OpenAI habían borrado accidentalmente datos críticos que podrían haber ayudado a rastrear dónde y cómo se utilizaron los artículos de los editores en el entrenamiento de los modelos.
Si bien se recuperaron algunos datos, las estructuras de carpetas y los nombres de los archivos se perdieron “irremediablemente”, lo que hizo que los datos recuperados fueran inútiles para la investigación. Este error obliga a los demandantes a recrear su trabajo desde cero.
¿Por qué es importante esto?
Esta eliminación podría tener un impacto significativo en el caso legal. Si los demandantes no pueden probar su afirmación de que OpenAI utilizó sus artículos sin permiso, puede resultar difícil responsabilizar a la empresa.
Aunque OpenAI intentó recuperar los datos, el incidente generó preocupaciones sobre la integridad de la gestión de datos de la empresa y su capacidad para cumplir con las obligaciones legales en lo que respecta al respeto de los derechos de propiedad intelectual.
Además, el equipo legal de los editores enfatizó que, si bien no creen que la eliminación haya sido intencional, subraya una cuestión crítica: OpenAI es la única entidad con acceso a sus propios conjuntos de datos.
La empresa podría estar mejor posicionada para realizar búsquedas exhaustivas para localizar cualquier contenido infractor. Sin embargo, sin una cooperación transparente, el proceso podría verse gravemente obstaculizado.
La defensa de OpenAI: uso legítimo frente a infracción de derechos de autor
En respuesta a estas demandas, OpenAI ha defendido constantemente su posición, argumentando que utilizar datos disponibles públicamente, incluidos artículos de noticias, para entrenar modelos de IA es una forma de “uso justo”.
Según esta doctrina legal, las empresas pueden utilizar material protegido por derechos de autor sin permiso si se utiliza con fines como investigación, educación o comentarios.
OpenAI cree que, dado que sus modelos, como GPT-4, se basan en grandes cantidades de contenido disponible públicamente, no necesita compensar a los titulares de derechos de autor, incluso si su tecnología genera ingresos al proporcionar servicios basados en IA.
Sin embargo, la legalidad de esta defensa sigue siendo un área gris. Si bien OpenAI ha firmado acuerdos de licencia con varios editores destacados, incluidos La prensa asociada, Información privilegiada sobre negociosy Tiempos financieroslos términos de estos acuerdos no han sido revelados.
Los informes sugieren que editores como Dotdash Meredith, la empresa matriz de Gentepodría estar recibiendo pagos sustanciales y, según se informa, Dotdash consiguió un acuerdo anual de 16 millones de dólares.
Estas asociaciones parecen ser parte de la estrategia de OpenAI para mitigar posibles riesgos de infracción de derechos de autor, pero la cuestión más amplia del uso de contenido protegido por derechos de autor sin consentimiento sigue siendo importante.
Las implicaciones legales y éticas
El caso OpenAI es un recordatorio de que los límites entre la innovación y la ley de propiedad intelectual siguen siendo turbios, especialmente en el campo de la IA en rápida evolución.
También destaca una preocupación más amplia: a medida que las tecnologías de inteligencia artificial se vuelven más sofisticadas, la línea entre lo que constituye “uso legítimo” y la infracción de derechos de autor es cada vez más difícil de trazar.
Para los editores, este caso plantea preguntas importantes sobre si deberían ser compensados por su trabajo utilizado en la capacitación en IA o si las empresas de IA deberían tener más libertad para construir modelos utilizando la gran cantidad de datos disponibles en Internet.
Si el argumento de OpenAI de que extraer datos disponibles públicamente constituye un uso legítimo se sostiene en los tribunales, podría sentar un precedente que cambie el panorama tanto para los creadores de contenido como para las empresas de tecnología.
¿Qué sigue en el caso?
Por ahora, la batalla legal está lejos de terminar. Si bien OpenAI no ha confirmado ni negado que haya utilizado ninguna obra específica protegida por derechos de autor, se espera que la demanda se prolongue durante algún tiempo.
Los próximos meses probablemente serán críticos para determinar cómo las empresas de IA manejarán las cuestiones de derechos de autor en el futuro. Los editores están siguiendo de cerca este caso, ya que su resultado podría remodelar la forma en que se utiliza el contenido para entrenar la IA y cómo se compensa a los creadores por su trabajo.
Un debate creciente sobre la IA y los derechos de autor
Este caso es parte de un debate más amplio sobre cómo debería evolucionar la ley de propiedad intelectual en la era de la inteligencia artificial.
Muchos creadores y editores de contenido están preocupados por las implicaciones de que la IA elimine su trabajo sin permiso, mientras que los desarrolladores de IA argumentan que tales prácticas son esenciales para construir tecnologías innovadoras que beneficien a la sociedad.
En los próximos años, es posible que veamos más casos como este, a medida que el mundo de la IA siga expandiéndose. Es probable que los tribunales tengan que decidir cómo equilibrar los intereses de los creadores, los consumidores y las empresas de tecnología de una manera que respalde tanto la innovación como la equidad.
Ejemplos del mundo real: ¿quién se ve afectado?
El impacto de este caso no se limita a las grandes editoriales como Los New York Times y Noticias diarias. Los creadores de contenidos más pequeños, los blogueros y los periodistas independientes también pueden sentir los efectos dominó del resultado.
Si a las empresas de inteligencia artificial se les permite extraer contenido sin licenciarlo, podría socavar el valor del trabajo original y la propiedad intelectual para todos los creadores.
Por otro lado, si los tribunales deciden que las empresas de inteligencia artificial deben pagar por el contenido que utilizan, esto podría proporcionar nuevas fuentes de ingresos para aquellos cuyo trabajo impulsa los algoritmos. Este es un momento crítico en la intersección de la tecnología, el derecho y la creatividad.
Al profundizar en esta disputa legal, podemos ver que el camino a seguir requerirá una cuidadosa consideración tanto de los principios legales como de las consideraciones éticas que rodean a la IA. Queda por ver si se exigirá a OpenAI que compense a los medios de comunicación por su contenido o si sus acciones se considerarán “uso justo”.
Pero una cosa está clara: la relación entre las empresas de IA y los creadores de contenido está entrando en un territorio inexplorado, y su resolución podría sentar las bases para la forma en que las futuras generaciones de IA interactuarán con el contenido que da forma a nuestro mundo digital.
Noticias
¿Quién será el próximo presidente del Comité Nacional Demócrata? Le preguntamos a ChatGPT

La carrera por el liderazgo del Comité Nacional Demócrata (DNC) ha comenzado oficialmente. El ex gobernador de Maryland, Martin O’Malley, y Ken Martin, presidente del Partido Demócrata-Campesino-Laborista de Minnesota, han surgido como los primeros candidatos oficiales.
La selección del nuevo presidente será fundamental para dar forma a la estrategia y dirección del partido de cara a las elecciones presidenciales de 2028.
Pero, ¿quién terminará reemplazando al actual presidente del Comité Nacional Demócrata, Jaime Harrison, quien ocupa el cargo desde enero de 2021? Semana de noticias preguntó la herramienta de IA generativa, ChatGPT, qué candidato cree que saldrá victorioso.
Ilustración fotográfica de Newsweek/Getty Images/AP
¿Quién ganará la carrera por el liderazgo del Comité Nacional Demócrata?
Semana de noticias preguntó Chat GPT “La carrera por el liderazgo del Comité Nacional Demócrata (DNC) ha comenzado oficialmente. El puesto quedará vacante en marzo cuando el actual presidente Jaime Harrison se prepara para dimitir. ¿Quién será el próximo presidente del DNC?”
Se pidió a la herramienta de IA generativa que viera una lista de los posibles candidatos, explicara por qué serían buenas opciones y sugiriera quién tenía probabilidades de ganar.
“El Comité Nacional Demócrata (DNC) elegirá un nuevo presidente para marzo de 2025, tras la decisión de Jaime Harrison de dimitir”, respondió Chat GPT. “Varios demócratas destacados han surgido como candidatos potenciales para este papel fundamental”.
Candidatos declarados

Ashlee Rezin/Chicago Sun-Times vía AP
Ken Martín
Martin, que actualmente se desempeña como presidente del Partido Demócrata-Campesino-Laborista de Minnesota y vicepresidente del Comité Nacional Demócrata, anunció su candidatura el 19 de noviembre. Aporta una amplia experiencia en la organización del partido y ha obtenido el apoyo de varios líderes y progresistas del partido estatal.
Semana de noticias se ha puesto en contacto con Martin por correo electrónico para solicitar comentarios.

Kevin Dietsch/Getty Images
Martín O’Malley
El exgobernador de Maryland y candidato presidencial de 2016 declaró su candidatura a la presidencia del Comité Nacional Demócrata el 18 de noviembre. O’Malley enfatiza la necesidad de que el partido se reconecte con los estadounidenses comunes y corrientes y se centre en la seguridad económica.
Semana de noticias se ha comunicado con Martin a través de un comentario en un formulario en línea.
Candidatos potenciales

Daniel Boczarski/Getty Images
Ben Wikler
Como presidente del Partido Demócrata de Wisconsin, Wikler ha desempeñado un papel decisivo en la movilización de votantes en un estado clave en el campo de batalla. Sus habilidades organizativas y su éxito en Wisconsin lo convierten en un fuerte contendiente.
Semana de noticias se ha puesto en contacto con Wikler por correo electrónico para hacer comentarios
Emanuel Rahm
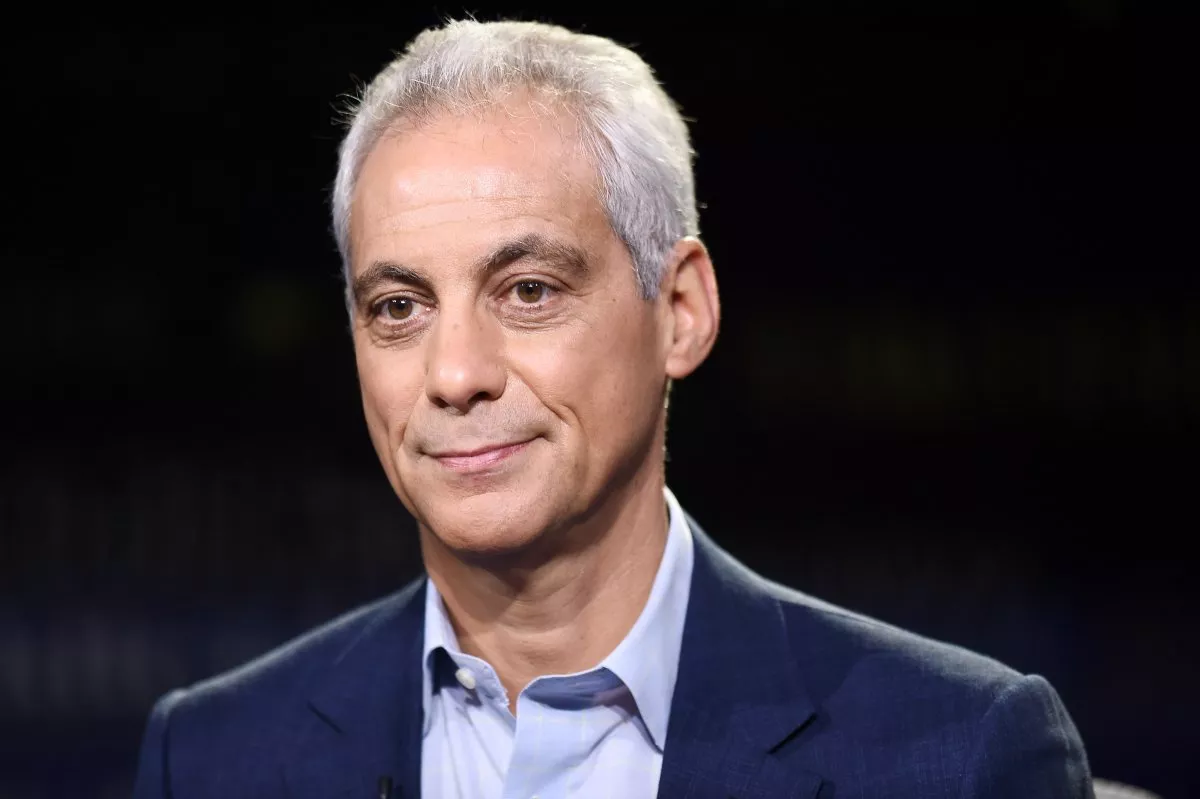
Steven Ferdman/Getty Images
Según se informa, el actual embajador de Estados Unidos en Japón y ex alcalde de Chicago está considerando postularse para presidente del Comité Nacional Demócrata. La amplia experiencia política de Emanuel y su capacidad de recaudación de fondos podrían ser activos valiosos para el partido.
Semana de noticias se ha comunicado con Emanuel por correo electrónico para solicitar comentarios.
Mitch Landrieu

Anna Moneymaker/Getty Images
El ex alcalde de Nueva Orleans y zar de infraestructura de la Casa Blanca ha sido mencionado como candidato potencial. La experiencia de Landrieu en proyectos de infraestructura y gobernanza urbana podría proporcionar una ventaja estratégica.
Semana de noticias se ha comunicado con Landrieu por correo electrónico para solicitar comentarios.
Resultado probable
Si bien es prematuro predecir el resultado definitivo, la entrada temprana de Ken Martin en la carrera y su apoyo sustancial de los miembros del Comité Nacional Demócrata lo posicionan como un candidato fuerte. Sin embargo, la dinámica podría cambiar a medida que otros candidatos formalicen sus candidaturas y presenten sus visiones para el futuro del partido.
La selección del próximo presidente del Comité Nacional Demócrata influirá significativamente en la estrategia y dirección del Partido Demócrata en los próximos años, especialmente después de los recientes resultados electorales.
¿Cuándo se decidirá el próximo presidente del Comité Nacional Demócrata?
Se espera que el Comité Nacional Demócrata elija a su próximo presidente antes del 1 de marzo de 2025. Las reglas del partido exigen que la elección de liderazgo se lleve a cabo antes de esta fecha, según Ballotpedia.
Aunque aún no se ha anunciado la fecha exacta, las elecciones generalmente tienen lugar durante la reunión de invierno del comité.
¿Tienes una historia? Semana de noticias debería estar cubriendo? ¿Tienes alguna pregunta sobre esta historia? Póngase en contacto con [email protected].
Noticias
13 sencillos consejos y trucos de Google Gemini para lograr la máxima productividad

Mucho más que un simple chatbot talentoso, Google Gemini ofrece funcionalidad de IA multimodal y una interactividad potente e indolora. El nivel más alto de Google One, uno de los mejores servicios de almacenamiento en la nube de la actualidad, incluye convenientemente acceso a su versión más potente.
Emitir indicaciones sencillas y generar texto escrito competente resulta fácil para los nuevos usuarios de Gemini, pero su conjunto de herramientas en constante expansión proporciona impresionantes mejoras en la productividad. Estas son algunas de las formas más útiles de utilizar el agente de inteligencia artificial de Google en constante mejora para ahorrar tiempo y aumentar el rendimiento.
Relacionado
Primeros pasos con Google Gemini: conceptos básicos del último asistente de Android
Si quieres probar Géminis, estos consejos y trucos te ayudarán
13 Actualizar la gestión del tiempo y las rutinas de autocuidado
Aprenda hábitos eficientes y prácticas efectivas.
Alimentar a Géminis con una lista completa de tareas pendientes, completa con tareas recurrentes y actividades únicas, puede generar un cronograma conciso que priorice su rutina personal, las próximas fechas límite importantes o los nuevos hábitos que está tratando de adquirir. Alternativamente, brinde al agente de IA un resumen detallado de cómo pasó los últimos días, y podrá brindarle consejos sobre cómo optimizar su agenda para que todo se ajuste más cómodamente.
En su vida profesional, delinear las necesidades y los recursos de un proyecto le permite a Gemini analizar las formas más rentables y rentables de lograr sus objetivos. Después de todo, nada ayuda más a desarrollar la eficiencia de una máquina que preguntarle a una máquina.
12 Obtenga esquemas sencillos de videos de YouTube
No pierdas el tiempo sentado viendo vídeos extendidos
A veces, los creadores de información se van por la tangente y los expertos educados se vuelven prolijos. En lugar de esperar vídeos largos para ir al grano, o intentar mantener todos los elementos en orden en tu cabeza, pídele a Gemini un resumen. Puede seleccionar los puntos más importantes a lo largo de una producción y presentarlos en un formato fácil de leer.
Funciona especialmente bien con vídeos instructivos, como tutoriales de reparación y cocina, pero resulta útil con todo tipo de contenido. Incluso puede ayudar a evitar anuncios tediosos para aquellos que aún no están suscritos a YouTube Premium.
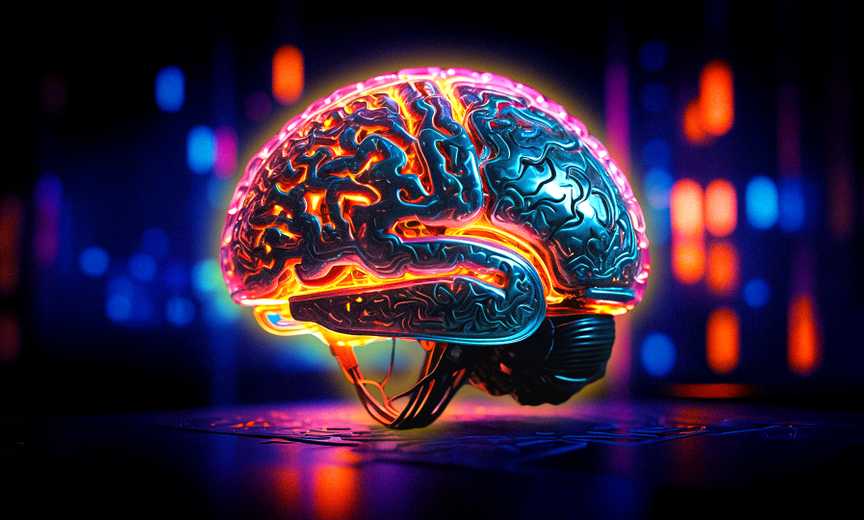
Relacionado
El vicepresidente senior de Arm explica el verdadero impacto de la IA, y no es lo que piensas
Nuestra entrevista con el ejecutivo de Arm, Chris Bergey
11 Resumir conjuntos de datos grandes y complejos
Analizar cantidades abrumadoras de información
Si tiene una hoja de cálculo con demasiadas páginas y columnas para entenderla rápidamente, pídale a Gemini que la desglose por usted. Puede extraer inferencias generales de toda la colección de datos, investigar tendencias dentro de categorías individuales e incluso ayudar a desarrollar gráficos para visualizar la información. Puede solicitar resultados más simples o más complejos si todavía tiene problemas para comprender un documento o necesita información más detallada.
10 Autocompletar hojas de cálculo según el contexto
Complete rápidamente la entrada de datos repetitivos
Fuente: Google
La función mejorada Smart Fill de Gemini en Google Sheets detecta patrones y proporciona soluciones automatizadas para la tediosa entrada de texto. Transfiere rápidamente datos relativamente simples de una columna o formato a otro. Gemini Autofill agrega una capa de integración de IA, identifica relaciones más complejas y sugiere formas de registrarlas de manera clara y efectiva. Estas dos características ejemplifican la utilidad de lo que algunos llaman un “autocompletado glorificado”.
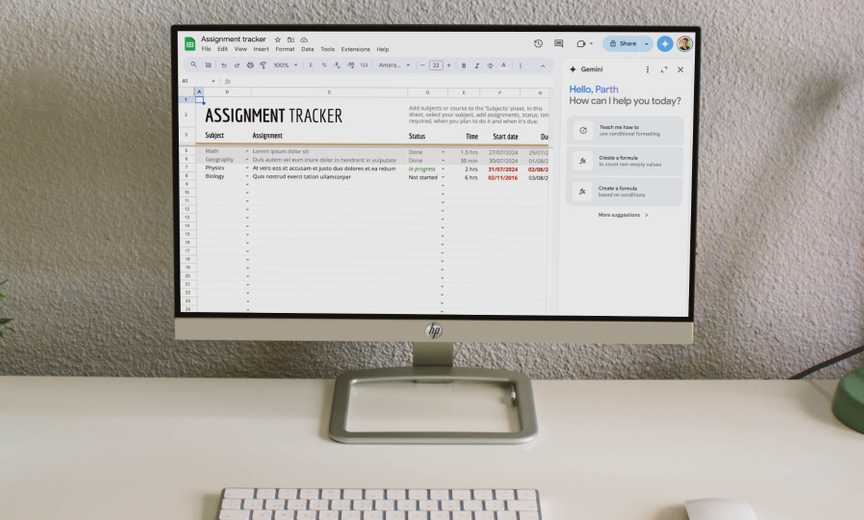
Relacionado
8 formas de usar Gemini en Google Sheets
Google Sheets con Gemini garantiza que tu día sea más fácil
9 Analice fácilmente la calidad SEO de los sitios web
Consulte las entradas del blog y los editoriales para comprobar su exhaustividad.
Un mensaje rápido le permite a Gemini examinar qué tan bien usted o un medio crearon un sitio. Puede compilar un informe sobre la cohesión de encabezados, meta descripciones, palabras clave y enlaces entrelazados. También puede usarlo junto con la página de resultados del motor de búsqueda para ver qué tan bien se está conectando con lo que quiere el público. Sobresale especialmente en generar preguntas de personas que también hacen y fragmentos destacados.
Con acceso a la herramienta de prueba de datos estructurados de Google, pocos agentes de inteligencia artificial pueden igualar la capacidad de Gemini para analizar la estructura y navegación de una página. Sin embargo, al igual que con otras solicitudes directas de análisis, asegúrese de comprobar los sentidos de las respuestas de Géminis. En lugar de confiar en que sus consejos de SEO sean correctos, utilice sus afirmaciones para comparar la página con las técnicas de SEO actuales y establecidas, y prepárese para solicitar revisiones o una segunda opinión.
8 Solucionar problemas y verificar el código
Rebotar ideas de programación de un modelo programado.
Los programadores han utilizado durante mucho tiempo patos de goma como oyentes inanimados para ayudar a encontrar problemas que se escapan de las grietas. Géminis puede responder, lo que lo hace aún más efectivo. Puede brindarle numerosos puntos de partida y métodos para abordar una tarea de programación y evaluar la eficiencia con la que se ejecutará su código ya escrito.
Querrá comparar sus sugerencias con sus conocimientos y recursos y, a veces, se topa con callejones sin salida o alucina, pero es mucho más efectivo que un juguete de baño.
7 Crea itinerarios de viaje inspiradores
Deje que Géminis actúe como trampolín para sus planes de vacaciones
Utilice Gemini para Google Sheets para crear planes de viaje perfectos, ya sea para un viaje de trabajo o recreativo. Puede dirigirlo a los sitios más famosos, mostrarle más atracciones que pasan desapercibidas e incluso presentarle algunos lugares locales oscuros. Naturalmente, siempre puedes utilizar sus itinerarios de muestra para ayudarte a pensar dónde quieres ir y qué quieres hacer, eliminando el estrés de planificar salidas de larga distancia.
6 Diseñe indicaciones de IA más efectivas
Cómo Gemini te ayuda a usar Gemini
Decirle al asistente de inteligencia artificial que necesita ayuda con el mensaje de Géminis correcto puede desbloquear estrategias que de otro modo tendría que investigar y adivinar. Esto tiene sentido, porque ¿qué conoce mejor a Géminis que él? Describir sus necesidades, incluido el contexto, el formato y el grado de especificidad, puede reducir la cantidad de tiempo necesario para obtener información útil. También puede adaptar el resultado al tono adecuado para comunicarse con grupos específicos de personas.
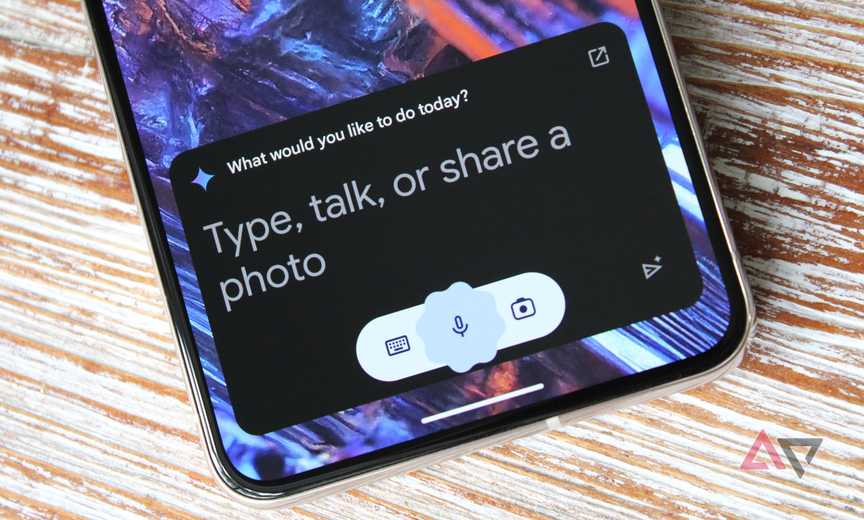
Relacionado
Aquí se explica cómo avisar a Géminis, según Google
Google publicó una hoja de trucos para aprovechar al máximo su herramienta de IA
5 Simplifique la redacción técnica para lectores generales
Reduzca la jerga y optimice los temas complicados
Para un escritor técnico que explica temas avanzados a generalistas, o para un profano que intenta comprender las complejidades de un campo de alta tecnología, Gemini hace maravillas al simplificar temas confusos. Reemplaza la terminología específica de un campo con una redacción sencilla y ayuda a lectores y escritores de ambos extremos del espectro a mantenerse en sintonía.
4 Encuentre respuestas a preguntas oscuras
Cuando la Búsqueda de Google no es suficiente
Es difícil obtener las respuestas exactas a algunas preguntas, pero el vasto Gráfico de conocimiento de Google vincula su enorme variedad de información de maneras poderosas. Mejora su capacidad para investigar y crear contenido informativo en profundidad, especialmente cuando utiliza Gemini en Google Docs.
Gemini y otros LLM cometen errores y siempre necesitarás volver a verificar sus respuestas, pero copiar y pegar rápidamente lo hace fácil. Al menos, buscar en la web a través de Gemini le brinda el trampolín perfecto para responder consultas extremadamente específicas.
3 Implementar fórmulas avanzadas de hojas de cálculo
Omita el proceso de dominar Google Sheets
Ya no necesitas pasar incontables horas dominando hojas de cálculo para implementar las fórmulas más efectivas. Gemini para Google Sheets ya hace un trabajo fantástico al sugerir las operaciones correctas y siempre está mejorando. La gestión competente de hojas de cálculo puede hacer o deshacer un equipo, lo que la convierte en una poderosa herramienta profesional.
2 Prepárese para entrevistas y reuniones importantes
Haga que Géminis haga las preguntas para evitar que lo tomen por sorpresa
Fuente: Policía de Android
No hay nada mejor que practicar para entrevistas individuales y paneles de discusión grupales. Proporcione a Gemini su tesis, currículum o tema y recursos de discusión, y pídale que le interrogue sobre contenido relacionado. Rápidamente desarrollarás una comprensión más profunda de tu propio material e incluso podría ayudarte a conseguir un trabajo o un ascenso.
1 Ajuste los agentes de IA de Gems personalizados
Tenga a mano asistentes de IA personalizados en todo momento
Fuente: Google
Los agentes de IA de Google Gems le permiten personalizar un agente específico para los temas, formatos y tonos que necesita habitualmente. Desarrolla el tuyo propio y mantenlo a tu lado para tus tareas más habituales. Esto puede reducir en gran medida la cantidad de tiempo y esfuerzo que se necesita para usar la IA, de otra manera que Gemini te ayuda a usarla.
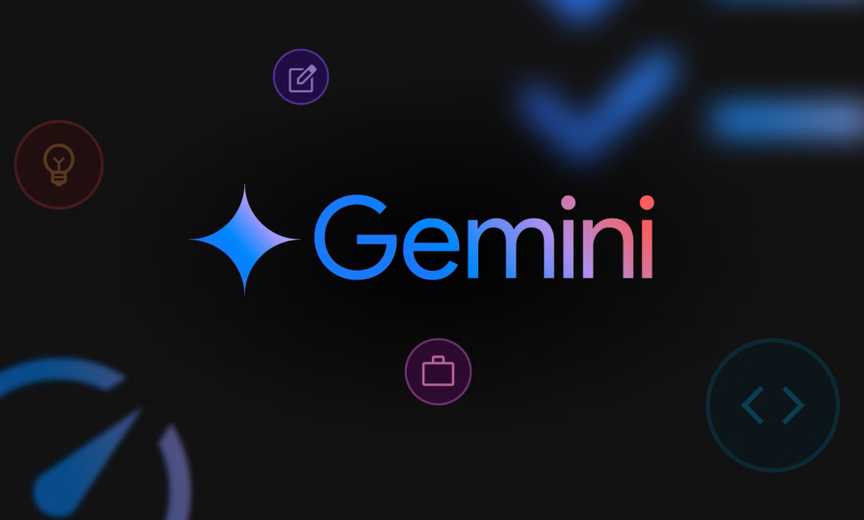
Relacionado
Cómo crear chatbots de IA personalizados con Gemini Gems
Crea un chatbot personalizado usando Gemini Gems
Las posibilidades casi infinitas siguen aumentando
A medida que Google integre aún más Gemini en servicios potentes como la aplicación Workspace, su efectividad solo mejorará, especialmente considerando el procesamiento de IA en el dispositivo que permiten los mejores teléfonos inteligentes de la actualidad. Reduce la complejidad de la capacitación, la comunicación, el resumen y la creación, y puede ayudarlo a mantener sus pensamientos en orden cuando aparentemente tiene demasiada información que manejar.
Si utiliza estos u otros consejos de productividad con frecuencia, probablemente se beneficiará de una suscripción Gemini Advanced, que brinda acceso a un procesamiento más potente que el Gemini estándar.
-
Recursos6 meses ago
Suno.com: La Revolución en la Creación Musical con Inteligencia Artificial
-
Startups6 meses ago
Remove.bg: La Revolución en la Edición de Imágenes que Debes Conocer
-
Recursos6 meses ago
Cómo Empezar con Popai.pro: Tu Espacio Personal de IA – Guía Completa, Instalación, Versiones y Precios
-
Recursos6 meses ago
Perplexity aplicado al Marketing Digital y Estrategias SEO
-
Eventos6 meses ago
La nueva era de la inteligencia artificial por el Washington Post – Mayo 2024
-
Noticias4 meses ago
Dos periodistas octogenarios deman a ChatGPT por robar su trabajo
-
Estudiar IA6 meses ago
Curso de Inteligencia Artificial de UC Berkeley estratégico para negocios
-
Tutoriales6 meses ago
Cómo Comenzar a Utilizar ChatGPT: Una Guía Completa para Principiantes